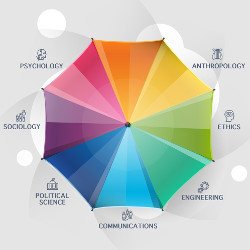
On October 23, 2008, Alan Greenspan, the Chair of the U.S. Federal Reserve, was testifying before Congress in the immediate aftermath of the September 2008 financial crash. Undoubtedly the high point of the proceedings occurred when Representative Henry Waxman pressed the Chair to admit “that your view of the world, your ideology, was not right,” to which Greenspan admitted “Absolutely, precisely.”17 Fast forward 10 years to another famous mea culpa moment in front of Congress, that of Mark Zuckerberg on April 11, 2018. In light of both the Cambridge Analytica scandal and revelations of Russian interference in the 2016 U.S. election, Zuckerberg also admitted to wrong: “It’s clear now that we didn’t do enough to prevent these tools from being used for harm. That goes for fake news, foreign interference in elections, and hate speech, as well as developers and data privacy.”15
Key Insights
- The social ills of computing will not go away simply by integrating more ethics instruction or codes of conduct into computing curricula.
- A better approach to addressing these problems would be to move the academic discipline of computing away from engineering-inspired curricular models and supplement it with the methods, theories, and perspectives of the social sciences.
- In practice, computing is already moving tentatively into the methodological and theoretical pluralism of the social sciences, but this movement has not been fully recognized within academic computing.
As far as mea culpas go, Greenspan’s was considerably more concise, but also much more insightful as to the root problem. Greenspan admitted the problem was not due to misguided user expectations, or to poorly worded license agreements, or to rogue developers. Instead he recognized the problem lay in a worldview that seemed to work for a while … until it didn’t. In the immediate after-math of the financial crisis, there were calls for reforms, not only of the financial services industry, but also within universities, where it was thought that unrealistic models and assumptions within economics departments20 and business schools11 were also responsible for inculcating a worldview that led to the crisis. It is time for us in computing departments to do some comparable soul searching.
This article is one attempt at this task. It argues the well-publicized social ills of computing will not go away simply by integrating ethics instruction or codes of conduct into computing curricula. The remedy to these ills instead lies less in philosophy and more in fields such as sociology, psychology, anthropology, communications, and political science. That is, because computing as a discipline is becoming progressively more entangled within the human and social lifeworld, computing as an academic discipline must move away from engineering-inspired curricular models and integrate the analytic lenses supplied by social science theories and methodologies. To this end, the article concludes by presenting three realistic recommendations for transforming academic computing in light of this recognition.
The World View of Computing
Academic departments are not one-dimensional monoliths, so right at the start I must acknowledge there is a wide range of perspectives and beliefs at play in any individual computing department. It’s also true that computing, like any academic discipline, has somewhat arbitrary intellectual demarcations: its boundaries are less like high fences and more like a series of irregular stones that mark the rough borderlands around its domain. Computing, as a new field, initially laid out a very preliminary series of markers to help distinguish it from mathematics and engineering. Perhaps the most important of these was computing’s unique disciplinary way of thinking and practicing, which, as narrated by Tedre and Denning,22 was variously labeled as “algorithmizing,” “algorithmic thinking,” “algorithmics,” and, more recently, as “computational thinking.” Over the decades, the claims made about the utility and power of this mode of thinking became increasingly ambitious and by the 2000s it became common to argue that everyone can benefit from thinking like a computer scientist.23 Analogous movements, such as Computer Science for All, Can-Code, and Computing at School, are all motivated by the premise that computational thinking will be a necessary part of all future work and thus it is essential that children learn it in school.
Within academia, computing and computational thinking has also followed an expansionist arc. The Digital Humanities—that is, using computational approaches and technologies within the humanities—was seen by its advocates as a way to refresh the humanities by modernizing its methods, moving it out of dusty dark libraries and into the clean, bright air of the datacenter.5 Computational social science is another recent curricular experiment in adopting the methodologies and techniques of computing.19 Finally, within computing itself, research has expanded significantly beyond both the analysis and creation of algorithms and the design and implementation of hardware and software architectures, to now include using these computational lenses to examine social, psychological, and cultural phenomenon more generally. ACM’s Transactions series now covers health care, computing education, economics and computation, and social computing, and are just a sampling of this new research growth within academic computing.
Along with this expansion have come bold claims about computing’s ability to better understand and explain the social world without needing background in social theory, economic models, or psychological concepts.1,18 While there is no doubt that many fruitful insights into social phenomenon are being made and will continue to be made through the adoption of computational approaches, it is striking how the flow of ideas appear to be in just one direction. For instance, an article in Nature argued it is completely legitimate for a computer scientist to study social phenomenon even with little knowledge of traditional social science methods and theories due to the insights provided by large datasets.12 Another recent article wryly noted, it is telling that those in the traditional social sciences are often called upon to embrace computational approaches, but “computational experts dealing with social phenomenon are rarely called to conversely embrace traditional sociological thought.”2
This triumphalist, almost quasi-colonizing mentality that computing appears to have in relation to other disciplines, is, at least partially, to blame for computing’s current fraught relationship with other societal actors. This mentality perhaps made sense when the discipline was initially staking its academic claims in the 1970s and 1980s, or when the discipline was undergoing the harrowing student registration crisis of the first years of the 2000s. But a too-strong belief that computing provides a privileged insight, a methodologically superior set of techniques and approaches that can be applied universally and which supplies truth propositions unblemished by social institutions, human failings, or antiquated theories, is the ideology that leads to tech executives testifying to Congress about how it all went wrong. Not only is it academically arrogant, it’s short-sighted as well, because instead of replacing social science approaches, academic computing would be immeasurably improved by supplementing its own with the methods, theories, and perspectives of the social sciences. Indeed, one could even go further and make the claim that not only would computing be improved by more social science, but that computing today actually is a social science.
Why Computing Is a Social Science
Broadly speaking, the social sciences are a range of academic disciplines that studies human society and human individuals in the context of society. Long established fields such as sociology, economics, anthropology, psychology, and political science no doubt first come to mind when thinking about the social sciences. But disciplines such as education, law, linguistics, geography, gender studies, communications, archeology, and even business school fields such as management, marketing, and human resources can all potentially be categorized as falling under the broad net of social science. While this diversity of specialized fields can be an obstacle when it comes to generalizing about its nature, this diversity is both a strength and a reflection of the complexities of its domain of study. This is a point that needs to be reiterated. One of the key insights (and values) of the social science of the past half century is its embrace of complexity. That is, methodological and theoretical pluralism is what defines both the social sciences in general, but also its subject, humans in social, political, economic, and cultural contexts. This is seemingly quite different from the natural and engineering sciences, where the predictability of its subjects can be better assumed and thus a single methodological approach for making and evaluating knowledge claims is possible.
I would like to argue that in practice computing is already starting to move out of the methodologically singular natural/engineering sciences and moving tentatively into the methodological pluralism of the social sciences, but that this movement has not been fully recognized within academic computing.
One can get a preliminary sense of the social scientific nature of computing by looking at one manifestation of its social scientific nature, namely, how computing is already deeply implicated in relations of power. As renowned sociologist Manuel Castells noted, power relations are “the foundational relationship of society because they construct and shape the institutions and norms that regulate social life.”8 One of the key insights of contemporary social science has been its recognition of the role and influence of power and politics throughout our lives, our society, our institutions, and our technologies of knowledge.
In the contemporary world, power rarely relies on coercion, but instead is enacted through persuasion—that is, by the construction of meaning through knowledge production and distributed by communication systems. Scholars in the 1970s and 1980s, for instance, focused their power analysis on newspapers, radio, and TV, but in the past decade a wide range of scholars from fields as diverse as law, sociology, economics, and communications are now focused on the truth-and power-constructing regimes of data and the algorithms that process it. Power “is operationalized through the algorithm, in that the algorithmic output cements, maintains or produces certain truths.”3 Or, simply, “Data are a form of power.”14
As computing professionals, we often see ourselves as problem solvers in some manner. We might be using a type of algorithmic reasoning, to say, find a bug, document a process, design a data structure, or engineer a redundancy system. Very few of us would think that we are also doing politics. “I’m just creating something cool / solving a problem for my client / doing my job.” It is often true that one’s computing work is relatively innocuous in terms of its relationship to power. But it’s not always true.
“In the future, how we perceive the world will be determined more and more by what is revealed to us by digital systems … To control these is the essence of politics.”21 This has already been recognized within legal studies, where scholars such as Karen Yeung, Shoshana Zuboff, Anthony Casey, and Anthony Nisbett, have made compelling arguments that algorithms are already transforming the rule- and standard-based nature of law and justice, to a privatized and force-based one implemented via algorithms. Recommendation algorithms, automated sanctioning systems, reactive violation detection and prediction systems, and nudge architectures are replacing the human agency built into our legal and political systems with an architecture of unknowable black boxes allowing the one-way surveil and control of people without any corresponding contestation.24 In Casey and Niblett’s analogy,7 we are moving from a society of rooms, some of which have Do Not Enter signs (and thus can be ignored or violations forgiven), to a society of rooms with locked doors. As such, our range of possible action will no longer be controlled by law, but instead be controlled by code. That is, we will increasingly be disciplined by policies devised by cyber-security professionals, using algorithms implemented by computer scientists, making use of data analytics provided by data scientists, and engineered to run hyper-efficiently by software engineers. It’s no wonder that James Susskind ends his 2018 book on the future of politics with an exhortation to computer professionals: “The future of politics will depend, in large part, on how the current generation of technologists approaches its work. That is their burden whether they like it or not.”21 But this reckoning will not happen unless we also are willing to make changes to computing curricula that reflects computing’s expanding role in shaping the future of our societies.
Three Recommendations for Transforming Computing
Despite the title of this essay, I’m not actually advocating for the institutionalized transfer of computing departments into social science faculties—such a move is no doubt highly impractical and implausible—but rather for a change in mentality, a recognition that the field now and in the future will have more affinities with the concerns of the academic social sciences, and fewer with the natural sciences or engineering. To get there, I have three recommendations:
Recommendation 1: Embrace other disciplines’ insight. First, computing must divest itself of its colonizing mentality toward other disciplines and to instead recognize that theoretic frameworks from outside computing have value and would indeed improve computing. Take, for instance, the subfield of data science. It has been especially good at identifying patterns in heterogeneous data sets. But to explain patterns and correlations “requires social theory and deep contextual knowledge.”16 Computer scientists are also increasingly finding themselves working in social and psychological domains. This work can be improved by theories and approaches already in place in those fields. A better understanding of human psychology, power, and the incentive structures in society, may have allowed us to avoid some of the socio-technical problems we face today. The lack of deep security measures in the initial Internet protocols, for instance, betrays the hopeful, but naïve understanding of human motivation held by the early pioneers of the Internet. The legitimation crises facing democracies today is at least partly a consequence of the social fragmentation enabled by digital platforms created by programmers with a minimalist understanding of what new communications modalities can do to an unprepared audience.4 Finally, consider the relatively newfound appreciation within AI research about how pre-existing human biases can pollute the training data using within machine learning. Perhaps less surprise would have been encountered had those working within AI been required to take, say, a course in anthropology. For almost 50 years, the most introductory anthropology training has endeavored to instill a recognition that cultural differences and perceptions of otherness biases the observations of researchers. And, yet, in AI research, we are now only starting to recognize this fact because of an institutionalized blindness to the accumulated insights of a century of social research.
The triumphalist, almost quasi-colonizing mentality that computing appears to have in relation to other disciplines, is, at least partially, to blame for computing’s current fraught relationship with other societal actors.
For too long within computing we have instead a tendency to rely on pop-culture theories about inevitable technology-driven social change that painted an attractive and self-satisfied veneer over our work. Moving forward, we need to do better, and be willing to inform both our work and our thinking, with the more nuanced, historically grounded, empirically supported thinking of the social sciences. We would all benefit from remembering the perspective articulated by Peter Denning: “I am now wary of believing what looks good to me as a computer scientist is good for everyone.”10
Recommendation 2: Replace some computing courses with social science ones. The best way to achieve my first recommendation is to embrace my second recommendation: modestly reduce the number of computing courses in our programs and in the ACM curricular recommendations in order to accommodate some mandatory social science courses.
I can already hear the rebuttal. “Surely there is no room for additional non-computing courses … we don’t have enough curricular room even to cover all the essential computing topics!” I have been actively involved in the design of two of my university’s computing programs, and I too remember well that feeling of having too many topics and not enough course spots. Regardless, the perception that topic X and topic Y absolutely must make it into the curriculum are sometimes more a reflection of individual faculty desires rather than a reflection of informed pedagogy or the hireability of students.
Take, for instance, the topic of Web development. By far, it is the main source of employment for CS graduates, and yet Web development has shrunk to being just one of several sub-areas within the elective-only Platform-Based Knowledge area in the ACM 2013 CS curricula guidelines. Indeed, many CS programs do not include any Web topics in their curricula, a point of some astonishment by those outside the CS academy.9 So if we, as computing curricula experts, are willing to let our students graduate without what are arguably the most important skills needed for successful employment because we think they can learn it on their own in the workforce, then surely there are one or two other computing topics that can also be learned after graduation, thereby opening up potential space in the curricula for non-computing courses.
We need to do more to fully educate our computing graduates than simply teach them deontological vs. utilitarian algorithms for ethical trolley problems.
But for this to happen, ACM curricular recommendations must lead the way. Future ACM curricula must acknowledge that computing students need more than just computer and mathematics courses. They must acknowledge that graduates in the 2020s will face greater responsibilities and the intellectual worldview of graduates must broaden as a recognition of how computing is both shaping and shaped by political, social, economic, and cultural institutions. If the ACM is unwilling to make these changes, the current social structure of the discipline will endlessly recreate itself, and the social ills enabled by computing will continue to surprise its creators.
Another rebuttal to this recommendation might be that “we already have a computer ethics course.” While an important first step for sure, we need to do more to fully educate our computing graduates than simply teach them deontological vs. utilitarian algorithms for ethical trolley problems. I’m not minimizing the vital work done by groups such as the ACM Committee on Professional Ethics, Computing Professionals for Social Responsibility, and Computing for the Social Good.13 The problem with computing ethics is that at present it stands by itself in the computing curricula. By only having a single mandated course about the relationship of computing to the wider human and social world, how can it not but strike a student that this is peripheral (and hence irrelevant) knowledge? Just one look at the curriculum and a student will no doubt get the impression that the ethics course is not all that important in comparison to courses such as numeric theory, algorithm evaluation, and programming.
This is the natural consequence of the engineering model that computing curricula seems to inhabit. That is, the belief there is so much computing and mathematics content to be learned that there is no room for anything else. As a result, we normalized the belief that the world is irrelevant next to computing precisely through the structure of our curriculum. It is sometimes said that workers of organizations adopt a world view that is a reflection of the organizational structure of their workplace. Our students do so as well, except in this case, it’s their academic discipline’s organization. This is a problem though that we can fix … or at the very least make an attempt at doing it better.
Recommendation 3: Embrace multidisciplinarity through faculty hiring. My final recommendation involves more boldly moving our discipline toward multidisciplinarity. Computing has sometimes struggled with maintaining a balance between academic disciplinary coherence on the one hand, with career-oriented students, on the other, who are mainly interested in the professionally relevant topics. In this regard, computing is quite similar to its cousin in the social sciences, the discipline of communications. That field weathered a series of crises brought on by technological change and by the contrasting pulls of faculty and student interests, by embracing multi-disciplinary opportunities.
Craig Calhoun, in his 2011 plenary address on communications as a social science, argued using a metaphor from ecology that porous edges are better than sharp boundaries when it comes to newer academic disciplines such as communications. Edges are zones where ecosystems overlap, and where biodiversity and biodensity are much higher than in the central areas of any one ecosystem. “There are more song-birds at the edges of forests than in the middle.”6 This is what computing also needs as an academic discipline: to move to the edge and to participate in the rich academic biodiversity that happens where computing interacts with other disciplines. Some researchers in CS are already there. But rather than make this an exotic vacation, it should be our discipline’s home flora and fauna. And we should not inhabit this edge with a colonizing ideology that sees computational thinking as the best way to understand and inhabit this world. Indeed, the whole point of inhabiting an edge is to take strength from multiple sources, from multiple world views, from multiple methodologies and theoretic angles, and not to pave it over with a single approach.
How can this be achieved? One way would be to hire more tenurable faculty into computing departments who are specialists in the human and social side of computing. We don’t need to limit ourselves to CS Ph.D.s. There are communications, sociology, law, psychology, anthropology, and other Ph.D.s out there whose dissertation topics are clearly computing related or even computational in approach.
Conclusion
Computing professionals and academics have helped create something awesome over the past half century. Awesome is truly the appropriate word, especially if we are cognizant of its etymological heritage. “Awesome” is derived from the ancient Greek word deinon, and this word captures better the full dimensions of computing’s awesomeness. To be deinon is to be both wondrous and terrifying at the same time. “There are many deinon creatures on the earth, but none more so than man” sings the chorus in Sophocles’ tragedy Antigone.
Within computing we have generally only focused on the wondrous and have ignored the terrifying or delegated its reporting to other disciplines. Now, with algorithmic governance replacing legal codes, with Web platform enabled surveillance capitalism transforming economics, with machine learning automating more of the labor market, and with unexplainable, non-transparent algorithms challenging the very possibility of human agency, computing has never been more deinon. The consequences of these changes will not be fully faced by us but will be by our children and our students in the decades to come. We must be willing to face the realities of the future and embrace our responsibility as computing professionals and academics to change and renew our computing curricula (and the worldview it propagates). This is the task we have been given by history and for which the future will judge us.
Figure. Watch the author discuss this work in the exclusive Communications video. https://cacm.acm.org/videos/computing-social-sciences
Join the Discussion (0)
Become a Member or Sign In to Post a Comment