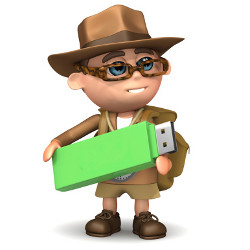
The world is facing enormous challenges, ranging from climate change to extreme poverty. The 2030 Agenda for Sustainable Development and its 17 Sustainable Development Goals (SDGs)a were adopted by United Nations Member States in 2015 as an operational framework to address these challenges. The SDGs include No Poverty, Quality Education, Gender Equality, Peace, Justice and Strong Institutions, among others, as well as a meta goal on Partnerships for the Goals. Despite limitations,7 the SDGs form a rare global consensus of all 193 UN member states on where we should collectively be heading.
Goals are meaningless without a way to track their progress. Data on the SDGs and the associated indicatorsb are often outdated or unavailable, hindering progress during the Decade of Action leading up to 2030.c Challenges around rapid access to data have also become apparent in the context of, for example, the Sudan revolution (public sentiment) or the Beirut explosion in August 2020 (infrastructure damage). The paucity of data has been highlighted during the COVID-19 pandemic, with its sudden impact on all aspects of life, most of which have yet to be quantified. Going beyond availability, accessibility, and timeliness, there is a need for more disaggregated data, such as by gender and town.
These challenges provide an opportunity to use non-traditional data sources as a complement to existing data and approaches, in particular through the use of artificial intelligence (AI). At the same time, a naive belief in AI as a savior risks ignoring complex, structural root causes. Furthermore, a reliance on digital traces, such as mobile phone data, risks excluding the most vulnerable—and often least connected—further aggravating inequalities. Lastly, there is a risk of taking a reductionist one-size-fits-all approach, often with a Western lens and without understanding local context, in particular in the Arab region with its diverse cultures and languages.
Here, we showcase regionally developed projects that explore the use of non-traditional data sources and AI to help measure progress toward the SDGs. Some of these projects also support countries in other parts of the world, demonstrating that the Arab world is not only a consumer of, but a contributor to, world-leading innovation.
Leveraging Behavioral and Humanitarian Data Sources to Analyze Development Challenges Faced by Syrian Refugees and Host Communities in Lebanon
Project stakeholders: UNESCWA, Data-Pop Alliance, Qatar Computing Research Institute, Lebanese Central Administration of Statistics, Lebanese Ministry of Telecommunications, UNHCR
The need to produce timely, granular, and cost-effective estimates for the vulnerabilities faced by refugees and host communities in Lebanon remains an important priority. These estimates are normally generated through official government data and the UN High Commissioner for Refugees’ register of refugees. However, families eligible for services are often not captured in the data; for example, according to the Vulnerability Assessment of Syrian Refugees (VASyR) report, only 44%16 of Syrian refugee families eligible for multipurpose cash assistance were provided with help.
In this project, UN ESCWA, in partnership with the Qatar Computing Institute (QCRI) and the Data-Pop Alliance,d explores the potential of non-traditional data sources to generate higher quality data that can lead to more targeted service provision by hosting governments, international organizations, and NGOs, particularly at the nexus of SDG1 (No Poverty), SDG8 (Decent Work and Economic Growth), and SDG10 (Reduced Inequalities).
This project covers several data sources: call detail records (CDRs), Facebook advertising data,6 the Global Database of Events Language and Tone (the GDELT project),11 and Twitter data. Here, we describe findings derived from CDRs.
Mobile phone metadata, such as the number and timing of outgoing calls, mobility patterns, and data consumption behavior, can be good predictors of socioeconomic status.2,e In our case, CDRs from two mobile operators, Alfa and Touch, were analyzed through the Ministry of Telecommunications. Data was obtained for 12 kazas (districts), spanning the two muhafazahs (zones) Bekaa and North, for the period April-June 2016 through 2019. Data from Touch consisted of the number of outgoing and incoming calls, disaggregated by age and gender; data from Alfa contained statistics about data consumption.
Overall, the spatial distribution of calls matched well with the population distribution in the CASLFS (R2 = 0.9).f Concerning spatial variation in socioeconomics, the ratio of dial-out-duration to receiving-in-duration in Touch data turned out to be a good predictor with an R2 of 0.36 with the percentage of Syrian refugee families with debt greater than USD $600 (using Tawasol data and VASyR ground truthg), and an R2 of 0.72 with the percentage of self-declared poor in the Lebanese host community (using general Touch data and CASLFS ground truth). Details can be found in the forthcoming full report.
Accessing CDRs carries with it several questions about privacy and data governance. The collaboration between UN ESCWA and the Central Administration of Statistics was critical to ensuring the accountability necessary for the Ministry of Telecommunications to grant data access. This project acts as a building block to consolidate the trust and necessary mechanisms to further explore the usefulness of this data source for the analysis of the living conditions of Syrian refugees and host communities.
Creating the Sudan Horizon Scanner for Detecting Real-Time Change
Project stakeholders: UNDP Sudan, Republic of Sudan’s Ministry of Labour and Social Development, Republic of Sudan’s Ministry of Trade, Republic of Sudan’s Prime Minister’s Office
Over the past 18 months, Sudan has “witnessed the people’s revolution and history-making transition process.”h Along with this, Sudan has experienced a rapid change in public sentiment, a narrative that has been difficult to capture using traditional data, which were detecting neither the dynamics nor drivers of this change. As part of its sensemaking efforts, UNDP Sudan’s Accelerator Labi developed the Sudan Horizon Scanner (SHS), a system to monitor a changing public narrative through real-time change detection, topic identification, sentiment classification, and summarization (see Figure 1).
Figure 1. Sudan Horizon Scanner: A Web application that collects different types of data and media files, then applies machine learning algorithms to detect early signs of opportunities or challenges in Sudan’s development.
The Accelerator Lab is exploring whether the system will be able to eliminate noise with minimal intervention and to explain changes in public sentiment by connecting different types of data, including socioeconomic and health data, Facebook posts, and newspaper headlines. The Accelerator Lab is also including in its analysis popular underground songs, radio shows and call-ins, and Friday prayer sermons—unusual sources of data that have captured the attention of other UNDP country offices as they look to analyze rapidly changing public sentiment in their countries.
The songs and sermons have been the most effective thick datasets for detecting signals of change. A challenge in analyzing this data is the fast rate at which vocabulary and lyrics are changing, with no existing training data for the use of these songs’ sub-languages that are colloquially called Randook. To develop the required natural language processing (NLP) functionalities, we are therefore building our own thesaurus and training data.
Mobile phone metadata, such as the number and timing of outgoing calls, mobility patterns, and data consumption behavior, can be good predictors of socioeconomic status.
Two preliminary insights derived from the Sudan Horizon Scanner include:
- There is a strong positive correlation between higher COVID-19 infection rates and frequent water cuts in Khartoum State.
- COVID-19 is more likely to be perceived as a conspiracy in areas with higher consumption of Kaftans, a piece of white cloth used to cover the diseased within the Muslim tradition. These areas also had higher death rates during the first peak of COVID-19.
Currently we are extending the SHS data capture and processing methods to help fill data gaps on the SDGs, particularly in the areas SDG2 (Zero Hunger), SDG6 (Clean Water and Sanitation), and SDG16 (Peace, Justice and Strong Institutions). The data will be translated into a real-time country performance tracker and monitor.
Using Social Media for Sentiment Analysis to Overcome COVID-19 Lockdown in Tunisia
Project stakeholders: UNDP Tunisia
With the onset of COVID-19, our collective reality changed irrevocably and along with it our approach to development. Values of transparency and accountability, participation and ownership should not, however, be compromised. To overcome lockdown and social distancing constraints, the United Nations Development Programme (UNDP) in Tunisia is using digital tools to inform priorities for its upcoming five-year plan through social sentiment sensing (see Figure 2).
Figure 2. Sentiment analysis process.
As of January 2020, there were 6.5M Facebook users in Tunisia,j equivalent to 55% of the population. This observation led us to study the behavior of Tunisians on social networks, particularly Facebook, to gauge trends and sentiments relating to development challenges. We collected two datasets related to Mosaïque FM,k a private radio station in Tunisia. The first consists of 99k Arabic news headlines with their descriptions, dates, and categories. The second consists of 221k comments posted on the Mosa’que FM Facebook page, including information on titles, authors, and comments. The data are further organized by topic, such as education, politics, economy, transportation, and health.
Tunisians communicate in French, standard Arabic, dialect, Roman numerals, and emoticons. Content on social networks is characterized by orthographic heterogeneity and lack of normalization particularly in written dialects that complicate the use of NLP tools. Additionally, Arabic users often use code-switching with Latin script to communicate in Arabic, a language rich in rhetorical characteristics, vocabulary, and implicit meanings. Due to these challenges, we annotated a corpus of 22,025 training instances, including dialectical Arabic, to train a sentiment classification model.
Challenges around rapid access to data provide an opportunity to use non-traditional data sources as a complement to existing data and approaches, in particular through the use of artificial intelligence.
This approach aims to guide planning and decision making through real-time analysis of public sentiment on various socioeconomic challenges and opportunities. For example, we identified strong signals about the health conditions in some regions where vulnerable populations expressed urgency in prioritizing action on SDG3 (Good Health and Well-Being). In addition, the sentiment analysis informed the development of UNDP Tunisia’s upcoming program (2021–2025). Going forward, the tool will be used by UNDP managers on a regular basis to monitor the evolution of trends and inform our work on SDGs (particularly No Poverty, Gender Equality, Reduced Inequalities, Climate Action, and Peace, Justice and Strong Institutions). UNDP Tunisia’s sentiment analysis framework has been shared with other UNDP country offices across the region and around the world as an effective example of gathering inputs and generating insights in a real-time manner when face-to-face consultations of regular citizens are disrupted, in this case due to COVID-19.
Monitoring Education Insecurities
Project stakeholders: Qatar Computing Research Institute, Education Above All,l United Nations Centre for Humanitarian Datam
Attacks on education, such as using force against students and educators to prevent their access to education, have intensified in recent years, especially in the global South,n slowing progress on SDG4 (Quality Education). Challenges that hinder authorities’ response to such incidents include missing and delayed data access. Despite the UN Security Council’s monitoring and reporting mechanisms to gather timely information on violations against access to education, most of the incidents remain unreported.1
Social networks, particularly Twitter, surface real-world events which otherwise receive limited coverage in traditional news media.17 This work seeks to capture reports of attacks on education from Twitter in Africa and the Middle East. Tweets are captured using language-specific keywords curated by domain experts. Over a period of 17 months (May 2019-September 2020), a total of 314K, 15.2M, and 161K tweets have been collected in Arabic, English, and French, respectively.
Keyword-based filtering alone is insufficient to identify pertinent reports from social media, particularly in the Arab region due to significant dialectal variations between nations. To overcome this issue, we trained three random forest binary classifiers to separate tweets “related to education insecurity” from “not related” using the Artificial Intelligence for Disaster Response (AIDR) system,8 which provides effective ways to collect, label, and train classifiers using active learning. Models trained achieved AUC scores of 0.85, 0.85, and 0.79 for Arabic, English, and French, respectively.
The models reduce irrelevant reports and retain only 26K (8.4%), 5M (34.2%), and 24K (15.2%) of Arabic, English, and French reports, respectively. We further discard tweets that are outside Africa and the Middle East, retweets, and duplicate tweets. This yields the final set of reports corresponding to 1.9K (0.62%), 11K (0.08%), and 236 (0.15%) of overall Arabic, English, and French tweets, respectively.
Extreme poverty will not be eradicated through more advanced technology, and lack of data is not the reason for lack of action on climate change.
We deployed the system to collect and identify attacks on education reports in real-time. Pertinent geotagged reports captured by the system are being continuously shared with the stakeholders using the UN Office for the Coordination of Humanitarian Affairs’s Humanitarian Data Exchange platformo and a public dashboard (Figure 3).p The trained models and the system can be deployed in other Arabic, English, and French speaking countries, noting that fine-tuning may be required depending on dialectal variations in the target language.
Figure 3. Public dashboard showing reports of attacks on education in Africa and the Middle East.
Mapping Digital Gender Gaps Using Advertising Data and Machine Learning
Project stakeholders: Qatar Computing Research Institute, University of Oxford, Data2X,q Sustainable Development Solutions Network
SDG5 (Gender Equality) includes a target to “enhance the use of enabling technology, in particular information and communications technology, to promote the empowerment of women.”r The measurement for this target is incomplete, with the latest 2018 data by the International Telecommunication Union (ITU)9 offering statistics for female-to-male ratios (f-to-m) of Internet users for only 83 countries.s To help fill this data gap, we launched Digital Gender Gaps, a collaboration between the Qatar Computing Research Institute and the University of Oxford, with support from Data2X.
In this project, we regularly collect data from Facebook’s Marketing API (free of charge) on the numbers of monthly active Facebook users in a given country, disaggregated by gender. We find that the f-to-m ratio of Facebook users in a given country is a good predictor of the f-to-m ratio of Internet users (adjusted R2 0.69, “online model”), better than relying on traditional offline indicators related to economic development or educational attainment (adjusted R2 0.62, “offline model”).4 Combining the two types of data improves the model fit (adjusted R2 0.79, “combined model”), but lowers the number of countries that predictions can be made for, as two different data sources need to be available (see Figure 4).
Figure 4. Female-to-male ratios of Internet users; left, taken from the most recent 2018 ITU data;9 right, predictions for the online model as of Nov. 11, 2020.
For the Arab region, we were expecting our model to be biased and underpredict the true f-to-m ratio as, due to cultural factors, women with Internet access might choose to refrain from Facebook more than men. We indeed observed such a gap for Oman, with an ITU reported f-to-m value of 0.93 vs. an online model prediction of 0.79 (on July 1, 2019 approximately the time point of the offline data collection), and Egypt (0.80 vs. 0.75). However, for Saudi Arabia (0.67 vs. 0.68), UAE (0.96 vs. 0.98), and Iraq (0.52 vs. 0.63) such gaps did not exist. As such our model does not seem to be systematically biased for the wider Arab region.
The project’s websitet regularly updates its predictions based on changes in the f-to-m ratio of Face-book users. The data is also included in SDGs Today, a global hub for realtime SDG datau that is used by both advocacy groups and policymakers. Going forward, we plan to extend our predictions to subnational regions.
Combining Data Sources for Mapping Poverty
Project stakeholders: Qatar Computing Research Institute, World Bank, UNICEF, Thinking Machinesv
Non-traditional data sources such as satellite imagery3,10 and CDRs2 have been used to map poverty at scale. We improve the state of the art by combining publicly accessible, anonymous advertising data with satellite imagery. Similar to the previous project, we use Facebook’s Marketing API to obtain estimates of the proportion of Facebook users utilizing a variety of network connections (3G, 4G, Wi-Fi), and mobile operating systems (iOS, Android) in a given location. We then combine this information with other variables such as population density and features extracted from daytime satellite imagery.5,15
We test our approach in the Philippines and India. As ground truth, we use the Wealth Index (WI), an asset-based measure of poverty derived from the Demographic and Health Surveys.w We apply ridge regression to predict WI using different combinations of Facebook and satellite imagery features (see the accompanying table).
Table. Wealth Index prediction performance of various models using different feature combinations of Facebook, satellite, regional indicators, and population density. All models are evaluated using R2 based on 10-fold cross validation.
In the Philippines, with relatively high Facebook penetration (>70%), we observe that a model using only Facebook features performs on par with a model using only satellite features. Both models improve when additional features such as population density and regional indicators are included, and the best performance is achieved when all features are combined. A geographic disaggregation shows that Facebook-only models perform better in urban locations, with satellite-only models performing better in rural locations. However, in India, Facebook features do not improve the performance of satellite-only models, neither in urban nor in rural areas.
Using Facebook advertising data for poverty estimation in countries in the Arab region with high Facebook penetration, such as Libya (>70%), appears promising. Furthermore, the advertising data affords the ability to obtain gender- or age-disaggregated poverty estimates.6 To encourage further evaluation of combining Facebook advertising data with other data sources for poverty mapping, this line of work has been presented at the UN World Data Forum and World Bank seminars.
Closing Thoughts
While striking a balance between case studies with a regional focus and those with a focus beyond the Arab region, all the initiatives presented here showcase regionally developed technology. Even for projects with a purely regional implementation, the lessons learned, and the knowledge obtained are disseminated throughout the global UN system, and together they offer an excellent demonstration of the opportunities that non-traditional data sources combined with AI provide for measuring and advancing the SDGs.
At the same time, these new approaches create challenges, including how to safeguard privacy and how not to exclude people without Internet connectivity, while amplifying the voices of the already-connected. More fundamentally, it is important to note that exclusion often extends beyond the data to the process of building and deploying technology. But any technology is only as good and as fair as the socio-political system it is embedded in. Put simply, extreme poverty will not be eradicated through more advanced technology, and lack of data is not the reason for lack of action on climate change. It is now more needed than ever to broaden the group of people who build technology for the SDGs, but also who get to decide what to build, and how it will be used.
Join the Discussion (0)
Become a Member or Sign In to Post a Comment