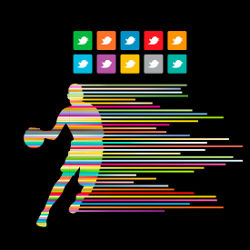
The sports industry is big business globally and domestically;10 for example, the New York Knicks of the National Basketball Association (NBA) generated $287 million in revenue in 2013.2 In order for sports organizations to maximize their financial performance, they must win on the court. The operational staffs, including coaches and general managers, must consistently make the right decisions despite many constraints, including a league-imposed salary cap and team budgets. Sports analytics plays an increasingly important role in such decisions.
Key Insights
- Sentiment analysis, an emerging text-mining technique, can help analyze tweets of NBA players.
- Coaches, general managers, and other staff of NBA teams can use it to discern and address players’ moods before games.
- Our study shows players’ before-game mood, as captured through the sentiment they reveal in their tweets, is positively associated with on-court performance.
Sports analytics traditionally involves statistical techniques for analyzing historical player performance. General managers have used it to build their rosters and coaches have used it in conjunction with their domain knowledge to adjust lineups and improve players’ on-court performance. Though ongoing sports analytics research and practices center mostly on the structured data of player profiles and historical performance,1 this article explores the extent NBA teams can use “unstructured” social media data to further their sports analytics efforts. This novel focus is motivated by the prevalence of social media analytics in all kinds of business domains over the past five years. Specifically, our objective is to show how NBA players’ pre-game emotional state, as captured through their tweets, or the messages they post on Twitter, before a game can help predict on-court performance in the game.
The framework we propose here can be used to inform decisions regarding game-day player assessment, lineup changes, and on-court strategy. Given NBA teams’ longstanding positive attitude toward and practice of sports analytics, the league is an appropriate context for illustrating the new role we envision for sports analytics.
We begin by exploring the widespread use of Twitter among NBA players, then how our framework could benefit sports analysts in the NBA and their potential motivation for adopting the approach. This discussion includes justification for why the framework and potential future relevant work could interest analysts and motivate software developers to add processing of unstructured data (such as social media content) to their commercial products. We next detail how social media analytics can be incorporated into analysts’ decision-making processes and follow with a case study implementing the core techniques of the framework.
Twitter and NBA Players
The past few years have seen an explosion of tweets by NBA players. With the unique features of Twitter compared to other social media applications and its worldwide user base, NBA players have used this emerging technology to communicate with fans, journalists, peers, friends, and others. They actively voice their opinions, thoughts, and feelings through short, direct messages of 140 characters or less on Twitter. According to data from http://Tweeting-Athletes.com and http://www.Basketball-Reference.com, 353 active players, or approximately 78.4% of the total, had Twitter accounts during the 2012-2013 season, posting a total of 91,659 tweets.
Table 1 includes several tweets by NBA players expressing their views and reactions to major NBA-related events. NBA players’ spontaneous use of Twitter renders Twitter a natural environment for spreading information. Players’ tweets thus provide insight into their unfiltered thoughts that may otherwise be unavailable. The tweets in Table 2 show players’ use of Twitter is not as uniform as their use of conventional media, producing unpredictable consequences for the league, teams, and the players themselves.
Along with the widespread use of Twitter by NBA players, what effect does the large number of players’ brief messages have on their teams? Compared to most non-sports organizations, which frown on employees’ using social networking sites for personal purposes, the NBA and its teams are even less friendly toward players’ use of Twitter, concerned it might distract from winning games;9 for example, in 2009, the league established a policy saying coaches and players are not allowed to use social networking sites beginning 45 minutes before game time until after post-game interviews.17 The Miami Heat, Toronto Raptors, Milwaukee Bucks, Los Angeles Clippers, and other teams have expanded the ban to “team times,” including practices and meetings.17 Still, players are avid users of Twitter, and the number of tweets they send shows no sign of decreasing.
Here, we suggest NBA teams consider the value of players’ tweets through a sports analytics perspective. When governments can listen to, analyze, and understand their citizens’ opinions through such channels as social networking sites and bulletin-board systems and use it in their administrative decision-making processes,6 NBA coaches and general managers can use players’ tweets to identify player moods before games and their potential effect on the court. To this end, our proposed framework offers a solution.
The Framework as a Solution
Baskerville and Myers3 wrote that in knowledge-creation networks, motivations and qualifications are the paramount issues to be considered when one domain consumes ideas produced by another domain. Since social media analytics is a novel creation combining information systems and computer science, sports analysts should welcome our framework, which is grounded in social media analytics, only if they are motivated and the framework itself demonstrates enough practical benefit.
Motivations. Analysts must first understand the demand shaping the markets likely to adopt the framework. First, organizations in non-sports domains have benefited from social media. The value of social media is presumably transferrable to sports organizations like the NBA and should align with their interest in achieving superior on-court, as well as related business, performance. Anecdotes throughout the business and statistical literature cite how organizations of all types take advantage of social media to gain a competitive edge. Social media can be used as an R&D tool to collect customer thoughts and feedback for product and service innovation and improvement, branding and sales, and customer support.5 While an increasing number of organizations have begun to deploy social media as an operational tool for managing employees, facilitating knowledge-management systems, and enhancing cross-functional collaboration,13 only a few sources report NBA teams have explored social media for operational purposes. Such dormancy is a surprise, given NBA teams demonstrate the same enthusiasm for social media as every other organization; all NBA teams have created Twitter accounts and actively use them year-round to interact with fans, aiming to fill seats and boost TV ratings. In this sense, the framework, which helps observe, understand, and manage player performance, should attract teams’ interest. On the other hand, in 2011, Alamar and Mehrotra1 wrote “… the most significant structural barrier to the growth of sports analytics is not only the absence of a clear doorway for teams … but also the lack a clear process for developing the skills needed to open that doorway.”1 Though preliminary, the framework we propose here could serve as a good starting point for NBA teams use of social media as an operational tool within their own organizations.
Given the popularity of sports analytics among NBA teams, social media analytics should also motivate them to adopt these methods. NBA team managements have long been disciples of sports analytics. Before sports analytics, domain experts, including scouts, coaches, and general managers, were the sole members of sports teams qualified (and authorized) to make sports decisions.16 However, with data from through a variety of conventional and multimedia sources, domain experts can quickly be overwhelmed processing a growing mountain of data. Moreover, they are less capable than statistical analysts at identifying the hidden patterns and trends behind the data. As a result, an increasing number of sports organizations turn to specialized analysts with advanced statistical know-how to make sense of it, leading to the current rise of sports analytics.
The spontaneous use of Twitter among NBA players raises the issue of whether these players even consider the consequences of their posted messages.
Sports analytics is well regarded; for example, the Player Efficiency Rating, devised by John Hollinger, a former ESPN writer and analyst, is a widely used indicator of NBA players’ overall performance. Many NBA teams now have statisticians on their payrolls; for example, the Dallas Mavericks have worked with Wayne Winston, a professor of decision science at Indiana University, assisting coaching staff with on- and off-court decisions. Since 2010, a new breed of management has become more prominent in the NBA, and an increasing number of statisticians, including John Hollinger of the Memphis Grizzlies and Darly Morey of the Houston Rockets have begun to assume key roles in the front office. Some NBA teams contract with third-party companies for specialized analytics services, as between, say, the Portland Trailblazers and Citizen Sports in the league’s player draft. Pursuing the potential benefit available through sports analytics, NBA teams are active proponents and participants in the annual MIT Sloan Sports Analytics Conference (http://www.sloansportsconference.com), looking to promote analytics tools for better on-court team performance.
While conventional sports analytics focuses primarily on structured data, we propose a framework that uses social media analytics to process unstructured textual data in sports analytics. Given widespread recognition of existing sports analytics, NBA teams should welcome the enhancement.
The data needed by the framework is easily obtained, further encouraging NBA teams to implement the framework. Sports analytics relies on the availability of raw data.10 Basketball aside, sports analytics is most widely applied in professional golf (http://www.pga.com/home and http://www.lpga.com) and Major League Baseball (http://mlb.mlb.com/home), given the ocean of data available through multiple sources. The proposed framework needs three sets of data: player/game profile (such as age, position, salary, and home/away game); player performance (such as field goals and percentage, plus/minus (+/−); and player tweets. The first two are available within NBA teams, as well as through outside databases compiled by third parties, including Basketball-Reference.com, ESPN (http://www.espn.com), and 82games.com (http://www.82games.com). Player tweets are easily viewed on Twitter, and analysts can use Web crawlers to gather data.
The social media analytics techniques needed by the framework are common features of existing commercial and open source software. Social media analytics includes a pool of established modeling and analytics techniques. The framework uses sentiment analysis to discern player mood from player tweets and regression, a type of trend analysis, to identify how that mood is associated with on-court performance. Both techniques are incorporated into existing software, including SAS Sentiment Analysis, SAS Enterprise Miner, and R. These resources free NBA teams from the technical detail of the framework. This low entry barrier to implementation could thus be further motivation for NBA teams to try it.
The framework’s concern with identification of players’ moods from their tweets has practical implications for three reasons: given the robust association between mood and performance in sports and psychology, NBA teams should want to discern player mood before games; mood adjustment has been an important part of team operation, especially player preparation; and incumbent methods for identifying player mood before games are limited. The framework thus represents a new way to manage player mood.
The consensus in the sports and the psychology literature is that mood affects athletes’ competitive performance in which they must closely anticipate and observe their opponents’ actions so they instinctively know how to respond. While positive mood helps athletes stay focused and vigilant, stimulating greater effort, motivation, and persistence, negative mood could be a major source of interference that distracts their attention.12,18
Players are heavy users of Twitter in that they update themselves frequently, providing much useful data for social media analytics.
NBA teams recognize the importance of tuning player mood before games, taking various approaches to offset negative mood or generate positive mood; for example, when the Indiana Pacers’ center Roy Hibbert struggled during the 2013-2014 NBA playoffs, his teammate Paul George invited him on a fishing trip to help him relax and regain his confidence. This apparently paid off in the form of Hibbert’s 28 points and nine rebounds in the next game, as well as in the Pacers’ victory over the Washington Wizards. At the same time, following a scandal involving Los Angeles Clippers’ owner Donald Sterling, there was a call through mainstream media for Clippers fans and players to boycott game five of the Clippers playoff series against the Golden State Warriors. Feeling his players were being distracted by related media coverage, Clippers coach Doc Rivers canceled an upcoming practice, giving his players a day off to refocus. Other anecdotes reflect how NBA teams work closely with psychologists who provide counseling services to address player mood before critical games.
Effectiveness and efficiency aside, these approaches cannot succeed without first discerning player mood. However, NBA teams cannot follow their players 24 hours a day, especially when off the court. Distributing a questionnaire designed to measure mood is not feasible, as it is time-consuming for professional athletes to complete and cannot be used to test mood before every game. As mentioned earlier, NBA players are active Twitter users, a stream of tweets that represents an enormous, unfiltered opportunity to discern their mood. In this sense, the framework could optimize NBA teams’ ongoing process of adjusting player mood by helping them discern mood more effectively.
Qualifications. The framework informs sports analytics in two main ways. First, it is rooted in social media analytics, a mature approach to processing social media data, developing and evaluating informatics tools and frameworks to collect, monitor, analyze, summarize, and visualize social media data to extract useful yet hidden patterns and intelligence.7 The rise of social media analytics could be attributed to three forces: growth of social media, with popular applications (such as Facebook, Twitter, and LinkedIn) spawning vast amounts of user-generated content; organizations recognizing the value of user-generated content, as well as other social media data, using it for competitive advantage; and business intelligence and analytics techniques and tools enabling collection, management, analysis, and presentation of social media data.
Social media analytics involves multiple informatics techniques, including sentiment analysis, or opinion mining, topic modeling, social network analysis, trend analysis, and visual analytics. All play an important role in the various phases of social media analytics and can work together to help decision makers extract intelligence from the social network data stream. Due to the maturity of these tools, social media analytics has been applied to explain, detect, and predict disease outbreaks, election results, macroeconomic processes (such as crime detection), movie box office performance, natural phenomena (such as earthquakes), product sales, and financial markets (such as stock price); see, for example, Feldman,8 Kalampokis,11 and Yu et al.21 Rui et al.15 drew on sentiment analysis, a technique that aims to distill mood underlying textual data, finding overall customer mood from tweets with regard to a movie has a direct effect on ticket sales. Along with the virtues and robustness of social media analytics, our framework can benefit NBA team management and players alike.
NBA players’ tweets represent high-quality text data for social media analytics because players use Twitter with spontaneous and genuine intent. Earlier research found athletes use social network sites to show affection and generally express themselves. Unlike conventional media, Twitter’s natural communications environment ensures NBA players’ unadulterated perspective on events and topics is expressed immediately. Moreover, the spontaneous use of Twitter among NBA players raises the issue of whether these players even consider the consequencesa of their posted messages.20 This problem is serious enough some ESPN analysts have suggested “maybe next time he’ll think before he Tweets,” motivating NBA teams and the league to institute regulations to curb “lack of thought.”20 Nevertheless, there is sufficient evidence to indicate the tweets communicated by NBA players are truly reflective of their opinions, thoughts, and feelings. As a result, Twitter could indeed be a credible channel for social media analytics to discern player mood.
Applying Sentiment Analysis
Determining and extracting a player’s mood from his tweets is not straightforward, as tweets include unstructured text and information. Tools are needed to analyze a sea of textual data to distill the hidden intelligence. The framework we recommend through which NBA teams can add information in players’ tweets to administrative decision making (see the figure here) involves analyzing players’ public tweets. The teams could thus know players’ mental status (such as negative moods) and introduce necessary interventions to address potentially less-than-optimal on-court performance beforehand. In the framework, NBA players post tweets as reactions to a number of things, including games, coaches’ on- and off-court decisions, or, more frequently, life encounters. At the core of the approach is sentiment analysis that could help sift through players’ tweets and identify their moods before games. The results are then summarized as a report to be presented to team management, which can then address players’ negative moods or look to inspire positive moods.
The distilled mood from players’ tweets is admittedly not free of bias due to a number of factors. First, bias can arise from self-selection. Certain players might simply post what they want seen on Twitter, hoping to promote a good though insincere image. While we cannot rule out the possibility of self-selection, its confounding effect is minimal due to players’ spontaneous and genuine use of Twitter. Second, the procedures for identifying player mood from mountains of textual data are themselves another potential source of bias. However, the virtues of sentiment analysis can compensate for bias. As noted earlier, this emerging text-mining technique has demonstrated robust validity in gauging customer mood through Twitter, and quantified mood has been used to help predict a number of future events.
The next section explores a case study of the process of applying sentiment analysis to identify NBA players’ moods underlying their tweets, further showing how mood is associated with performance in upcoming games.
Case Study
This case study serves two purposes: detail a process of applying sentiment analysis to identify NBA player mood before games and test whether a player’s identified mood is associated with on-court performance in the ensuing game. For the study, we built two datasets: Athletes Generated Content dataset (AGC), which collected the tweets of NBA players posted during the 2012-2013 season, and the sports performance dataset, which likewise collected player data in all games during the 2012-2013 season.
Following the framework proposed by Matsudatira,14 we used Tweeting-Athletes.com to assemble a comprehensive list of NBA players (353 total) with Twitter accounts during the 2012-2013 season. The AGC dataset collected the tweets posted by these players during the season. For each tweet (content aside), the dataset also included descriptive attributes (such as timestamp when the tweet was posted). AGC found almost all active players in the league at the time had a Twitter account and posted 91,659 tweetsb that season, with most players, 266 of the 353, or 75.3%, posting at least 100 tweets for the season. The dataset also identified some noticeable “heavy users” of tweets; for example, Dwight Howard, star center of the Houston Rockets, alone posted 1,214 tweets.
To build the sports-performance dataset, we retrieved box-score data and player data for the 2012-2013 season from Basketball-Reference.com. We combined the information from each game with the information from each player who played the game as a single record in the dataset. Extracted game information included game date, game type, home/away, opponent and win/loss, with score. Player information included age (on the day the game was played), games started, minutes played, field goals (percentage), three-point field goals (percentage), free throws (percentage), and plus/minus (+/−).c
In order to represent all tweets accurately, we preprocessed their content by filtering out pure re-tweets and information-oriented tweets containing URL links. We also removed non-English tweets. Given the fact that tweets are replete with non-standard English, we applied a data-cleansing mechanism. Misspellings (such as “stealling” and “cuting”) represent a primary source of nonstandard English usage. We corrected misspellings through the minimum Hamming distance, an automatic error-correction algorithm. Non-standard English might have also originated from repeating letters (such as “Awwwwful,” “Awfuuuul,” and “Ruuuude”). We thus replaced letters occurring three or more times consecutively in the same word with two consecutive occurrences of the same letter. In the examples of repeating letters, the algorithm resulted in tokens “awful,” “awful,” and “Ruude,” respectively. We subsequently corrected the tokens as misspellings, if necessary, through the minimum Hamming distance.
We applied AFINN sentiment lexicons and an extended hand-assembled list of emoticons to detect the polarity of players’ sentiment in the tweets, or players’ moods behind the tweets. AFINN is a sentiment lexicon containing English words developed by Finn Årup Nielsen of the Technical University of Denmark. It rates words between −5 (negative) and +5 (positive), with a larger number representing a more positive sentiment. Though based on the Affective Norms for English Words lexicon proposed by Bradley and Lang,4 AFINN is a more refined and focused list based on the language used in microblogging platforms, including slang, obscene words, acronyms, and Web jargon prevalent on the Internet.4 We used an application based on R to automatically extract features related to the AFINN lexicon from a given tweet. We calculated an AFINN score for the tweet by summing the ratings of the positive and negative words matching the lexicon. The computed AFINN score reflects the mood of a player behind the tweet. Moreover, tweets are characterized by various emoticons that can express mood; for example, :) or :-) express positive mood, and :(expresses negative mood. We also accounted for the emoticons in our extended list by mapping them to either
or
,d as in Table 3; the examples in Table 4 represent a snapshot of the results of our overall sentiment analysis.
Recall the second goal of our study was to test the association between players’ identified moods before games and their on-court performance in subsequent games. A player might post several tweets before a game. Before we could simply aggregate the AFINN scores of the tweets to identify a player’s mood before a game, we also had to deal with timestamps. NBA games typically start at 8:00 P.M. on game day. Due to the league’s media policy prohibiting players, coaches, and officials from using Twitter and other social network sites from 45 minutes prior to game time until after post-game interviews, we then selected 7:00 P.M. (of game day) as the cutoff time to define “before game.” That is to say, for a particular game played by a particular player, we used his tweets back to 7:00 P.M. on game day until 7:00 P.M. one day before to discern his mood before the game. We then calculated the AFINN scores of the tweets submitted during this timeframe. The total score represents a player’s mood before a game. The higher the aggregated score, the more positive a player’s mood. Moreover, to enable comparison of mood among different players, we normalized the daily AFINN scores through the mean and standard deviation of all his seasonal available records.
To test the mood-performance relationship, we developed a model relating a player’s mood before a game to his performance in the game. We began with a standard baseline model, including mood variable only, then extended the baseline model to a more comprehensive one consisting of variables commonly included in the sports analytics literature.
We thus adopted the following model
where i, t indexes a player-game-day’s combination, Pi,t represented the performance of player i for a game at day t, M represented a player’s mood, and β was the coefficient of the regression model. Here, εi,t was the unobserved player/game-specific effect reflecting the idiosyncratic characteristics associated with each player and with the game itself; Table 5 includes estimates under the column heading “Baseline Model.”
The baseline model might be subject to the “endogeneity” influence common in sports analytics. To limit it, we added control variables that are potential predictors of sports performance. As suggested in the sports analytics literature, we devised the following extended model
where Pi,t−1 is one game-day-lagged performance of player i for a game at day t, Salaryi,t is the natural logarithm of the player’s salary in the game season, Agei,t2 is the player’s age on game day, Homei,t is the game if a home game (1) or away game (0), PosCi and PosGi are two position dummy variables (0 or 1) identifying the player’s position (center, forward, or guard), and β1 through β8 are the coefficients of the extended regression model; the estimates are listed in Table 5 under the heading “Extended Model.”
Coaches, players, fans, and researchers alike share a well-founded belief that athletes’ emotional states affect their on-court performance.
Consistent with the psychology and sports literature, we found mood does affect player performance—β = 0.17, p < 0.01 in the baseline model, and β = 0.16, p < 0.01 in the extended model. This result confirms a player’s mood, as captured by his tweets posted prior to a game, is positively associated with his performance in the game. Such a finding is essential because it highlights the informational value of players’ tweets. Team management could draw on sentiment analysis to identify players’ moods by examining their tweets, detecting potential concerns regarding on-court performance. Coaching staff could then address players’ negative moods or help inspire positive moods. In this sense, with the proposed framework, coaches and management staff are able to keep pace with players’ emotional status by following their tweets. The extended model takes a step further by considering player attributes, including position, age, and salary, as well as an important game attribute, home or away game. This analysis supports the robustness of the results we found in the baseline model using Twitter as the sole intelligence source. The extended model suggests other applications of the framework for future work and users of sports analytics; for example, an analyst would be able to explore how the mood-performance relationship varies with different player factors and game factors.
Conclusion
Business intelligence involves using technologies to transform data into meaningful information for business analysis and decision making. Against this backdrop, our proposed framework could be regarded as providing a new avenue for sports intelligence for coaches, general managers, and various sports organizations with the potential for expanding the sports analytics horizon. Along with the use of tweets among NBA players, social media analytics has emerged as a practical means for gathering intelligence about players and their performance. Players’ tweets provide insight into their thoughts and opinions, especially what cannot be obtained through other sources. Moreover, players are heavy users of Twitter in that they update themselves frequently, providing much useful data for social media analytics. Our framework demonstrates the value of players’ tweets as a source of direct game-performance intelligence.
Using sentiment analysis on players’ tweets, coaches and general managers can unleash the power of the unstructured data in the tweets, transforming it into valuable information concerning players’ pre-game moods and likely performance outcomes. Coaches, players, fans, and researchers alike share a well-founded belief that athletes’ emotional states affect their on-court performance. Following this logic, we analyzed 91,659 tweets posted by NBA players during the 2012-2013 season, finding their pre-game emotional states, as captured through sentiment analysis on their tweets, was directly related to on-court performance after controlling for other factors affecting performance.
Acknowledgments
We thank the reviewers, as well as Robert Barbato, John Ettlie, Sean Hansen, Victor Perotti, Hao Zhang, and participants of the Saunders College of Business at the Rochester Institute of Technology research colloquium (2014-2015) for their insightful comments, suggestions, and advice.
Figures
Figure. Framework of our recommended administrative process that listens to and transforms NBA players’ tweets into in-game intelligence.
Join the Discussion (0)
Become a Member or Sign In to Post a Comment