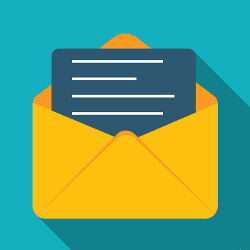
Both sides of the Point/Counterpoint "The Case for Banning Killer Robots" (Dec. 2015) over lethal autonomous weapons systems (LAWS) seemed to agree the argument concerns weapons "… that once activated, would, as Stephen Goose wrote in his "Point," be able to select and engage targets without further human involvement." Arguments for and against LAWS share this common foundation, but where Goose argued for a total ban on LAWS-related research, Ronald Arkin, in his "Counterpoint," favored a moratorium while research continues. Both sides accept international humanitarian law (IHL) as the definitive authority concerning whether or not LAWS represents a humane weapon.
If I read them correctly, Goose’s position was because LAWS would be able to kill on their own initiative they differ in kind from other technologically enhanced conventional weapons. That difference, he said, puts them outside the allowable scope of IHL and therefore ought to be banned. Arkin agreed LAWS differs from prior weapons systems but proposed the difference is largely their degree of autonomy and their lethal capability can be managed remotely when required. Arkin also said continued research will improve deficiencies in LAWS, thereby likely reducing the number of noncombatant casualties.
Stepping back from the debate about IHL and morality, LAWS appear to be the latest example of off-the-shelf (more-or-less) algorithms and hardware systems to be integrated into weapons systems. Given this, the debate over LAWS fundamentally concerns how far AI research should advance when it results in dual-use technologies. AI technologies clearly support driverless vehicles, aerial drones, facial recognition, and sensor-driven robotics, and are already in the public domain.
These technologies can be integrated into weapons of all sorts relatively cheaply and with only modest technical skills when equally modest levels of accuracy and reliability are acceptable. One must look only at the success of the AK-47 automatic assault rifle and Scud missiles to know relatively inexpensive weapons are often as useful as their higher-priced counterparts. A clear implication of the debate is AI research already enables development and use of LAWS-like weapons by rogue states and terrorists.
No one can expect AI researchers to stop work on what possibly could become dual-use technologies solely on the basis of such a possibility. LAWS may be excluded from national armories, but current AI technology almost assures their inevitable development and use by ungoverned actors.
Anthony Fedanzo, Corte Madera, CA
‘AI Summers’ Do Not Take Jobs
Artificial intelligence is a seasonal computer science field. Summers and winters appear every 15 years or so. Perhaps now we have reached an endless summer. Or not. A healthy discussion could keep expectations manageable. In his blog@cacm post "What Do We Do When the Jobs Are Gone?" (Dec. 2015), Moshe Y. Vardiwrote, "Herbert Simon was probably right when he wrote in 1956 that ‘machines will be capable … of doing any work a man can do.’" Simon was not right. Our admiration for Simon will not be lessened by considering his full statement: "Machines will be capable, within twenty years, of doing any work a man can do." But 20 years passed, and then 40, and now almost 60. Some people today say it will happen within the next 20 years. Want to bet? Even the most intelligent of us can underestimate the difficulty of creating an intelligent machine. Simon was not alone; every AI summer is marked by such pronouncements. AI advances will benefit everyone in small ways. Some jobs will be eliminated. Others will be created. More technology-driven solar and wind energy jobs are created than coal-mining jobs are lost. For more than five years the U.S. has added jobs every month, more than two million each year, despite the development of more capable machines. Humans are creative and resourceful.
Jonathan Grudin, Redmond, WA
What NBA Players’ Tweets Say About Emotion
The article "Hidden In-Game Intelligence in NBA Players’ Tweets" by Chenyan Xu et al. (Nov. 2015) lacked, in my opinion, a complete understanding of the topics it covered. The measures it cited were not adequately reported; for example, not clear was what the dependent variable consisted of, so readers were unable to judge what the coefficients mean or the adequacy of a 1% adjusted R2 in Table 5, an effect size that was most likely meaningless.
Moreover, the sample size was not explained clearly. There were initially 91,659 tweets in the sample, and 266 players tweeted at least 100 tweets during the season in question. Other than in a small note in Table 1, the article did not mention there are only 82 games in a regular NBA season, resulting in at least 1.22 tweets per game for those 266 players; this is not an appropriate sample size, and the distribution is most likely a long tail. With 353 players tweeting and 82 games, the sample size should be 28,946 player-games (the unit of analysis), yet the reported sample size was a fraction of that—3,443 or 3,344. That would be fewer than 10 games per player and not an adequate sample size.
Also unclear was if players with more tweets before a game can have higher emotion scores, as this measure seems to be an aggregate; the article said, "The total score represents a player’s mood … The higher the aggregated score, the more positive the player’s mood," emphasis added. More tweets do not mean more emotion. The article also did not address if there is a difference between original tweets and replies to other tweets.
The article also made a huge assumption about the truthfulness of tweets. NBA players are performers and know their tweets are public. The article dismissed this, saying, "Its confounding effect is minimal due to players’ spontaneous and genuine use of Twitter," yet offered no evidence, whether statistical, theoretical, or factual, that this is so.
The article coded angry emoticons (such as >:-o) to the negative mood condition, as in Table 3. This emoticon-mood mapping is incorrect, as anger can be positively channeled into focus and energy on the court. Smileys and frowns were given a weighting of +/−2 on a scale of +5 to −5, but not explained was why this is theoretically defensible.
NBA coaches do not seek to maximize performance at the level of an individual player but at the level of a team as a whole across an entire game and season. Bench players usually cannot replace starting players; the starters start for very good reasons.
The article’s conclusion said the authors had analyzed 91,659 tweets, yet footnote b said, "Of the 51,847 original posts, 47,468 were in English," implying they analyzed at most 87,280 tweets. Restating the number 91,659 was itself misleading, as tweets were not the unit of analysis—player-games were—and the authors had only 3,443 such observations, at most.
The one claim reviewers and editors should definitely have caught is in footnote c: A metric that can capture the unquantifiable? I am so speechless I might have to use an emoticon myself.
Nathaniel Poor, Brooklyn, NY
Authors’ Response:
Our study explored whether and how NBA players’ tweets can be used to extract information about players’ pre-game emotional state (X) based on the psychology and sports literature and how it might affect players’ in-game performance (Y). To generate X for a player before a game, we purged pure re-tweets, information-oriented tweets, and non-English tweets. Based on the remaining valid tweets, we then extracted, aggregated, and normalized the data, as in Table 5. We still find it intriguing X explains up to 1% of the total variations in Y, whereas other standard variables explain only 4%.
Chenyan Xu, Galloway, NJ, Yang Yu, Rochester, NY, and Chun K Hoi, Rochester, NY
Join the Discussion (0)
Become a Member or Sign In to Post a Comment