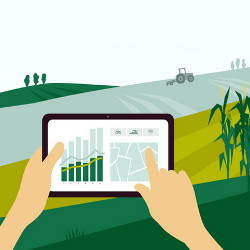
The Food and Agriculture Organization of the United Nations (FAO) reports that, compared to 2010 levels, global food production needs to increase by 70% prior to 2050 to feed the world’s growing population, which is expected to reach between 9.4 and 10.2 billion by then.12 We need to achieve this goal in spite of the fact that the amount of arable land is not increasing, diets are changing, water demand is rising, the climate is changing, and both the environment and soil health are under pressure. These problems are most alarming in low-and middle-income countries (LMICs), which are expected to see the highest population increases,19 leading to a growing demand for food and more diversified diets. In many LMICs, most of the population is rural, and more than 70% of farmers are small-scale producers (SSPs).16 As has been seen in more developed nations, economic growth in LMICs can reduce population growth and potentially improve livelihoods. LMICs need an agricultural transformation to help grow their economies, a daunting task made more difficult by such enormous obstacles.
Key Insights
- 72% of the world’s 570 million farms operate on less than 1 hectare.
- The average smallholder in sub-Saharan Africa lives on less than $2 USD per day.
- Digital solutions can improve access to finance, advisory, insurance, and market services for millions of smallholders.
- Only 13% of SSPs in sub-Saharan Africa have registered for digital services and less than 5% are active.
- Innovations in connectivity, sensors, AI, digital infrastructure, and usability are needed to drive the adoption of digital agriculture for smallholder farmers.
Digital agriculture promises to help address many of these global challenges. Digitization of the food system can enable greater efficiency, transparency, profitability, and equity. The use of digital technology has seen rapid growth and investment, which has spurred many new innovations in Smart Farming.3 These include sensors, Internet of Things (IoT), automation, Blockchain, artificial intelligence (AI), and computer vision. However, most innovation of this kind has been designed for high-income countries (HICs) and large commercial farming systems. Few digital innovations are designed specifically for LMICs and SSPs. Additionally, while digital solutions can potentially improve the lives of millions of rural poor, there are fundamental barriers to adoption. For example, mobile Internet for SSPs is not available to all, with sub-Saharan Africa and South Asia seeing some of the largest gaps.17 In Figure 1, coverage gap represents the population living in areas with no mobile broadband. Usage gap represents people who live in areas with mobile coverage but do not have access, often due to handset or subscription costs, digital skills, literacy, trust, and safety, which are even bigger barriers to adoption amongst women.26 Such barriers must be overcome to realize the potential of digital agriculture for SSPs.
Figure 1. Mobile Internet connectivity by region 2020.27
In this article, we discuss how digital technologies can benefit small-scale producers, smallholder farmers, and small livestock operators. We highlight how digital agriculture innovations must be developed differently for these users, and, consequently, the need for new approaches to make those innovations feasible for LMIC value chains. Investing in and focusing on the right technologies for smallholder farmers could have enormous impact at scale. We contend that the increased use of digital technologies can not only help address global food-production challenges, but also improve the livelihoods of millions of SSP households.
The Promise of Digital Agriculture
Digital agriculture—that is, using digital technology and data to drive agricultural processes and decisions—can help the entire agricultural sector be more efficient, transparent, productive, profitable, and responsible. Sensors in the field, combined with automated farm equipment and data from drones and satellites, can provide new insights and better advisories to farmers.9 While today it may just be an aspiration for SSPs, the entire operation of the farm can be automated. Better data and understanding of farm operation risks can help improve targeting of inputs and tailoring of finance and insurance products, as well as give buyers, off-takers, and commodity traders the insights they need to invest in agriculture.7 Some of the key trends in digital agriculture, based on recent reports on agricultural investments, include:3
- Sensors: Existing sensors measure weather or basic soil properties. New sensor types are being developed, such as for nutrients or more accurate sensing of plants and livestock. There is also new research on in-plant sensing.
- IoT: The connection of small, cheap, and disposable sensors throughout IoT platforms enables real-time monitoring and cloud or edge computing, providing greater visibility and traceability of food throughout the supply chain. For example, if fresh produce exceeds temperature thresholds at any point during transit this can be recorded and flagged in real time. There is also a greater demand to learn where food comes from. Several startups are using IoT to build technologies that trace and monitor produce in storage units from the farm to the retail store.
- Automation: Robotic milling stations already exist for dairy and autonomous tractor applications. Startups are developing new applications around sowing, chemical application, irrigation, and weeding. Autonomous vehicles equipped with high-resolution cameras continually monitor crops as they grow through a process known as rapid phenotyping, which promises to increase the pace of new crop variety development.
- Imagery: Remote sensing, which in agriculture typically refers to analyzing images from satellite data, has been around for several decades. New trends center on either using low Earth orbit (LEO) satellites to access images more frequently or using higher-resolution imagery from drones—flying beneath clouds and available on demand—to monitor crop health or pest and disease outbreaks.
- Blockchain: Smart contracts and traceability can be ensured with secure blockchain technology, so that sellers, buyers, and consumers can be certain that information and data about the source and transit of food products and shipments is trustworthy and has not been compromised.
- Artificial intelligence (AI): As new data sources become available in larger quantity, resolution, timeliness, and quality, new techniques using distributed computing are required to process that data and convert it into actionable information. AI holds promise and machine learning (ML) is already being used to some degree—from crop prediction to chatbots—and will continue to be applied in more sophisticated ways. Augmented intelligence may be even more feasible to support SSPs’ decision-making in the near-term.
- Computer vision: Algorithms are rapidly improving to take advantage of the larger amounts of available imagery and photos. An AI model analyzing a photograph of a leaf, for example, can quickly identify specific plant pests and diseases, and make real-time treatment recommendations. Farm field boundaries can also be automatically extracted from satellite images.
These technologies are in various stages of readiness for agriculture, and many are still at the peak of expectations for precision agriculture20 in HICs. The Gartner Hype Cycle reflects the visibility of technologies as they mature. Technologies at the peak indicate a lack of clear, evidence-based applications—that is, they may be overhyped and confusing the landscape. We present a version of the Gartner Hype Curve for SSPs (see Figure 2), adapted and updated from Rakestraw et al.20 and Gray et al.,13 because the same assumptions about readiness are not necessarily consistent across HIC and LMIC contexts. We also propose that an improved innovation assessment framework may be required, such as the Technology Readiness Levels created by NASA6 and previously adopted for crop research23 or another innovation-scaling framework.14 Regardless, the advantages of digitization and application of the right technologies are likely to bring SSPs enormous benefit. We describe some of these in subsequent sections.
Figure 2. Digital agriculture hype curve for SSPs.
Small-Scale Producers and Farming in the Developing World
The agriculture sector is one of the primary employers in LMICs, employing more than 80% of the rural population in some countries.15 The majority of the world’s farmers are smallholders, with 72% of the world’s 570 million farms operating on less than 1 hectare.16 Agriculture is a significant driver of economic growth in these countries and critical to overall gross domestic product (GDP).18 Many smallholder farmers are producing for their own consumption, but for agriculture to contribute to economic growth and help bridge looming food gaps, farmers must invest in their operations, produce more, and sell into markets.
Who are smallholder farmers? According to AGRA’s 2017 Africa Agriculture Status Report,2 smallholder farmers provide livelihoods for more than two billion people and produce about 80% of the food in sub-Saharan Africa and Asia. While a generalized definition of a smallholder farmer is based on area (less than 1 hectare or 2.5 acres), a more nuanced typology is based on the share of crop production value that is sold, and the share of non-farm income to total-household income, as shown in Figure 3. Based on this definition, the general categories are:
Figure 3. Smallholder farmer typologies.2
- Subsistence-oriented small farms, which sell less than 5% of their agricultural output and obtain less than 33% of their total income from non-farm sources.
- Transition farms, which obtain 33% or more of their income from non-farm sources and sell up to 50% of their crop output.
- Pre-commercial small farms, which sell 5% to 50% of their production and earn less than 33% of their income from non-farm sources.
- Commercial small farms, which sell 50% or more of their production. Commercial small farms sub-divide further, into specialized commercial farms, if their non-farm income share is less than 33%, or into diversified commercial farms otherwise.
Across the groups that have farming as their main source of income—that is, subsistence, pre-commercial, and specialized commercial—the average annual farm income is around $780 per year. To be able to design solutions for them, it is critical to understand this in terms of the scale required and the resources available to smallholder farmers to access and use technology.
For agriculture to contribute to economic growth and help bridge looming food gaps, smallholder farmers must invest in their operations, produce more, and sell into markets.
Small-scale producer farm families lead a challenging existence. The average smallholder farmer in sub-Saharan Africa lives on less than $2 USD per day. They are often growing food for their own consumption and when they do have access to markets, they often don’t receive competitive market prices. With no way of storing produce post-harvest, most farmers in a region are selling simultaneously, driving prices down. Smallholders often don’t have access to the latest seed, inputs, and advice for maximizing yields—they are forced to rely on older, traditional, or counterfeit varieties and inputs, and to use antiquated practices. If farmers do have access to a government extension agent, visits are often infrequent, with agent-to-farmer ratios as low as one agent to 5,000 farmers.25
This means their crops are more vulnerable to the risks of climate change, weather variability, pests, and disease. Recent outbreaks in East Africa of fall armyworm and locusts have caused significant losses to production. Smallholders have limited ability to absorb these shocks because they have little or no access to affordable credit or insurance due to lack of collateral, ineffective land tenure systems, or the fact that they are landless contractors. With these challenges and barriers, and the looming persistence of poverty in LMICs, improvements to the livelihoods of the rural poor are difficult to realize. It is in this context that digital solutions tailored to smallholder farmers need to be designed.
Digitally Enabling Agricultural Transformation for Smallholder Farmers
Data about farms, coupled with insights from the latest advances in technology, can help transform the livelihoods of smallholder farmers and SSPs. Digitization of smallholder agriculture can reduce risk, improve productivity, increase incomes, empower women, and help solve the impending challenge of producing enough nutritious food for the world’s rapidly growing population. Some promising trends are enabling digital technology adoption, including:
- Smartphone costs are rapidly decreasing. A Jio phone in India, for example, costs less than $10 USD and data plans are becoming more affordable. More countries are reaching data costs of less than 2% of monthly income.27
- Social media usage is rapidly growing. Social media and tools such as WhatsApp and Facebook are seeing rapid adoption in LMIC’s.
- Mobile money has enabled growth of digital services. MPESA in Kenya, for example, has been attributed to a 2% decrease in poverty.23
- Mobile Internet coverage is increasing, enabling smartphone use, access to information, app usage, and two-way data flows. LMIC 4G coverage increased from 30% in 2014 to 75% in 2018.27
- Digitization of services, such as access to inputs; access to finance, insurance, and advisory services; and connections to markets, is taking place.10
- Voice and conversational AI, in the form of voice assistants or chat-bots, shows potential for providing farmers with automated advice and more streamlined access to information and services.
Furthermore, there is some evidence that the application of digital technologies for relevant use cases results in higher smallholder farmer productivity and income, particularly when those services are bundled. Figure 4, from the Digitalization of African Agriculture Report,10 shows the multiplier effect of bundling services. These use cases are outlined in Table 1 and summarized below:
Figure 4. Digital agriculture impact on smallholder farmers.10
Table 1. Digital services for smallholder producers, and technology needs, including a few examples.
Agricultural advisory services. If the data from a farm is captured, whether from in-field sensors or remote-sensed by satellite or drone, it can be combined with agronomic science to create digital advisories for:
- Planning: What crop to grow in a particular season based on expected weather, crop prices, and market demand.
- Planting: When to sow seed based on crop type and predicted weather.
- Management: When to irrigate, fertilize, and apply pesticide.
- Harvest: When to harvest the crop based on market prices, predicted weather, and drying and storage costs.
And, by digitizing extension and development agents, even farmers who do not directly interact with digital tools themselves can benefit from more accurate and timely information through such digitally enabled intermediaries.
Market linkages. Digital tools can help connect farmers to markets. On the input side, digital ordering services can help connect farmers to certified seeds and fertilizers—for example, DigiFarm and iProcure in Kenya. On the output (harvest) side, access to current market prices and digital connection to transport and aggregation centers—for example, Loop in India—can help farmers reduce costs and increase profit. Similarly, milk producers in India can receive instant feedback on quality through a digital scale and testing service and get rewarded for higher quality.
Traceability of agricultural products from farm to consumer for food safety and transparency can also unlock opportunities to enable small-scale producers to participate in regional and global marketplaces.
Financial services and insurance. Farming businesses get a return on their investment once they sell their produce to a buyer. This could be 90 days (from seed to harvest) after they obtain capital to purchase seeds, fertilizer, pest and disease treatments, and hire labor during the season for harvesting and transportation. Credit can be obtained from banks and other creditors if farmers have collateral, such as land or other assets. However, many smallholder farmers do not own the land they are farming and don’t own other forms of collateral. Farmers may not be identifiable, or their land ownership cannot be verified, making it hard to get credit at reasonable rates.
More data about the farmer and farm can provide financial services and insurance providers with valuable information to assess risk and provide smallholder farmers with more-tailored credit products, loans, and insurance policies. There are companies in Africa and South Asia—for example, Pula, Oko, Acre, Farmdrive, and Skymet—working to assess risk using satellite imagery and weather data to create credit-scoring algorithms and insurance risk assessments. If more data about a farmer and the farm field could be integrated to create trusted and verifiable credit scores and risk profiles, this could unlock finance and risk-mitigation tools that smallholder farmers need to invest in their farms to try new technologies.
Agricultural research and development. In the field of plant science, plant phenotyping refers to the set of methodologies and protocols used to accurately measure plant growth, architecture, and composition at different scales. Next-generation sequencing technology has greatly accelerated functional genomics, allowing for the identification of important genes and agronomic traits. The understanding of how genetics interact with the environment (GxE) through phenotyping is an essential part of crop breeding. Rapid phenotyping is now possible with digital technology—for example, high-resolution, multi-spectral imaging; drone imagery; in-ground sensors; and data platforms—enabling precise measurement, analytics, and digital twins to be generated. These solutions, however, are not yet adopted in LMICs, and development is still required, especially for below-ground measurement of root structures.28
Sustainability and climate. Climate change is one of the greatest threats facing humanity.1 For agriculture, climate change could depress crop yields up to 30% by 2050 without adaptation. Small farms around the world will be most affected (see Figure 5). Digital technologies will be essential to implementing the three action plans identified by the commission: increase research on agricultural climate adaptation; expand access to climate-informed digital advisory services; and expand small-scale food producers’ access to insurance, finance, markets, adaptive technologies, and agroecological practices.
Figure 5. Areas of high agricultural risk for different climate hazards in vulnerable areas.1
Long-term sustainability of farming systems is also dependent on improving and maintaining soil health. Unfortunately, soil health is in decline in many regions, limiting the soil’s ability to support higher productivity. Without investment in soil health, crop yields would decline. Digital soil spectroscopy sensors combined with satellite imagery can produce soil property maps and provide the information needed for tailored agronomic advisory. Similar application of technology to measure soil carbon could also help open new revenue streams for smallholder farmers, which many proponents argue may be possible through carbon markets.
Barriers to Adoption and Research Opportunities
The technologies discussed in the previous section, such as sensors, imagery, or automation, still aren’t widely adopted in smallholder farmer systems.22 Only 13% of SSPs in sub-Saha-ran Africa have registered for digital services and even fewer are active.10 There are several reasons for this. For example, despite improvements in mobile Internet coverage, there is still a staggering usage gap of 3.3 billion people who live in areas covered by mobile broadband but are not using mobile Internet services27—and the usage gaps are significant in sub-Saharan Africa and South Asia (Figure 1). These figures tell us that the factors creating the digital divide go beyond technology, such as:
Connectivity and access. Many farms and rural areas do not have quality Internet access. The ITU estimates that around half of the world’s population does not have Internet access, and many of them are in the developing world. The situation is improving; at the end of 2018, the mobile coverage gap represented 10% of the world population compared to 24% in 2014, but most of this gap exists in the marginal, rural areas of LMICs.27
Even with good connectivity, there are still adoption challenges to overcome. Significant digital gender gaps exist across LMICs—313 million fewer women than men use mobile Internet, representing a gender gap of 23%.26
What is needed? New technology solutions need to be developed to provide low-cost Internet access. To connect cameras or tractors, and support video, this connectivity needs to be broadband, which in the U.S. is defined as 25 Mbps downlink, and 3 Mbps uplink. We note that for precision agriculture technologies, uplink capacity needs to be greater than downlink, since most data is sent to the cloud. To address gender gaps and usage issues more broadly, there should be an emphasis on human-centered design that considers social context. Women, for example, may share a mobile handset, in which case separate accounts for personal access to services may be important.
Affordability. Smallholder farmers are financially constrained. Consequently, digital solutions for smallholder farmers need to be affordable. Existing on-farm solutions are cost-prohibitive. Sensors cost a few hundred dollars and can be prone to theft in these geographies. Drones cost a few thousand dollars, have limited battery life, and access to energy is scarce. Furthermore, tractors are often not a viable option for small-scale producers due to the high capital cost. Remote-sensing solutions require the processing of large amounts of satellite imagery in the cloud. AI techniques on this data require GPUs and further increase the cost of providing digital solutions.
What is needed? New techniques need to be invented to accomplish the same task as expensive devices, but at a low cost. In some cases, technology can be replaced by using less-costly manual components or by adopting creative service-delivery business models.
Literacy and skills. Many farmers in LMICs are not literate and technology skills are low. The GSMA Gender Report26 cites literacy and skills (linguistics and technology) as the highest barriers to digital-device adoption.
What is needed? Technologies to translate insights and to make them usable by smallholder farmers. In some cases, this may also require a new UI for smartphones or research into voice technology. For farmers with older-feature phones, new methods are needed to convey digital insights and design to meet users where they are on the digital and language-literacy spectrum.
Timely and relevant information. Relevance is one of the barriers to adoption of services. One way to ensure information services are more relevant is to base them on timely and spatially relevant data. With little in-field observations from the farm itself, existing solutions often rely on remotely sensed data from satellites. However, the small size of farms challenge existing solutions that are typically designed for farms in HICs, which span tens of acres and more. In LMICs, the farms are typically less than 1 hectare, and producers often plant multiple crops in that plot. Since each small farm is only a few pixels in a satellite image, it is difficult to extract intelligence for each crop in the field.
What is needed? Higher-resolution and more timely data, and information and insights at an affordable cost, are required to enable smallholder farmers to be more precise in their operations and more resilient to climate-induced and other shocks.
Data trust and security. Despite the need to obtain more data about smallholder farmers, there are stakeholders collecting information about agriculture in LMICs. Theoretically, if this information could be shared and aggregated, it could benefit the entire agriculture industry, including SSPs. However, one of the biggest barriers to sharing data is trust about data usage and consumer protection. Farmers, input suppliers, buyers, traders, financial companies, governments, and other entities all have varying incentives to collect and share data, yet there are few effective mechanisms for securely sharing data. Additionally, data breaches can have dramatic repercussions, yet securing data can be costly and complex.
There is still a staggering usage gap of 3.3 billion people who live in areas covered by mobile broadband but are not using mobile Internet services.
What is needed? Research into secure data platforms, peer-to-peer and privacy-preserving data-sharing models, and data marketplaces will provide stakeholders with choices about how to share data with appropriate protections against misuse, and within privacy and consumer protection laws.
Open Research Problems
For the digital technologies presented in the previous section to be adopted by small-scale producers, we need research in different areas of computer science. For example, to enable the adoption of sustainable agricultural practices, computer scientists need to develop low-cost carbon sensors; new AI techniques to estimate carbon, nitrogen, and water use from satellite imagery and other sources; and new user interfaces to convey the insights to smallholder farmers—all using low-cost techniques. We highlight some of these challenges for various digital agriculture scenarios listed in Table 2.
Table 2. Research problems that inhibit the deployment of technologies for smallholder producers.
Hardware and architecture innovation: Making solutions more affordable. Research is needed to design low-cost hardware architecture that is as functional as existing solutions. Smallholder producers can benefit from various types of hardware, including ground sensors that measure soil moisture and temperature; weather stations that measure abiotic properties; motion sensors that monitor livestock; cameras that identify pests and diseases; and drone imagery that helps to identify crop stress, predict yield, and recommend action to bridge the yield gap.
A few desired features of the hardware for smallholder farmers are:
- Lower cost: Technologies to sense or convey information at a lower price point.
- Low power: Sensors or cameras should operate for long periods of time with low and intermittent power, perhaps using renewable energy sources or low-cost energy-storage solutions.
- Ruggedized: Technologies on a farm must operate in harsh conditions, including adverse weather, floods, and in the presence of wild animals.
- Theft-proof: Devices that can operate out of sight or can trigger an alarm may be more usable.
Achieving all the beneficial features is difficult. A few promising approaches are being explored by startups and academia. The Chameleon soil moisture sensor8 developed by CSIRO uses low-cost components to display different-colored lights, instead of sending values to the cloud, to indicate soil moisture content. Surrogate sensing is another promising approach for reducing cost. MEMS and audio technology can help build low-power sensors to measure agricultural parameters. Recent work leverages Wi-Fi chipsets on smartphones as a sensor for soil moisture and electrical conductivity.11 GroGuru has developed sensors that can be placed deep in the soil and are not visible to the human eye.
Other promising research has explored alternative imaging solutions. Plantix uses a smartphone’s camera to detect pests and diseases. TYE24 uses a camera (or smartphone) mounted on a helium balloon and transported by a human on a tractor or a bicycle as an aerial imaging alternative to drones. Further research, either on the use of low-cost components or alternative-sensing methods, can help make sensors and cameras more affordable.
These technology innovations can also lead to potentially new business models. Instead of deploying multiple sensors, a farmer could manually move a sensor to different parts of the farm to determine the soil map—for example, for nutrients. Alternatively, crop insurance, finance providers, or the government could subsidize the cost of hardware on the farm.
AI/ML, speech, and computer vision research: Deriving relevant data. Advances in computer vision and AI have already brought significant benefits to agriculture. Commercial tools, such as Climate Fieldview, Farmers Edge, and Land O’Lakes R7, can detect crop stress; predict weather, yields, and outcomes; and provide natural language and voice interfaces to growers. However, additional research is needed to realize these benefits for smallholder farmers.
Key research challenges include:
- AI on low-resolution satellite data: Each pixel in satellite imagery is a few meters, which is often too coarse for small farms.
- Multi-cropping systems: Several smallholder producers practice subsistence farming. They plant multiple crops in the same farm, which leads to challenges in the ways different crops interact and how digital systems can isolate the performance of each crop to provide appropriate advisories.
- AI on sparse data: There is a dearth of good, labeled data from small farms. Satellite data is limited, sensors are also sparsely deployed, and voice data is collected for the most-spoken languages. New AI techniques need to be developed to label the available data and augment data streams to train models for smallholder producers. While AI holds promise, more immediate solutions could leverage an augmented intelligence approach.
- Downscaled climate models: Accurate weather forecasts are available in HICs but less so in LMICs due to cost and complexity. Localized forecasts supported by low-cost sensors would improve a small-scale producer’s ability to adapt to climate change and be more resilient. High-resolution seasonal forecasts in the tropics are important for seed selection, insurance, subsidy programs, and food security.
There are a few promising approaches to address the sparse data challenges noted above. However, they have not yet been applied at scale for agriculture. For example, generational adversarial networks (GANs) can help generate new data to train the AI models. This can also be generated from 3D simulations of farms. Recent advances in graph neural networks can help incorporate human knowledge to overcome the limitations of sparse data. Self-supervised learning schemes can combine different data sources to generate more labeled data. Applying these and other AI techniques in agriculture can help bring the benefits of the latest in AI to smallholder farmers.
Networking, systems, and security research: Connectivity, edge, data platforms. Farmers need low-cost Internet connectivity on their devices. The sensors, cameras, and other devices need connectivity on farms. However, existing solutions are very expensive. A key challenge is how to make high-speed Internet more affordable.
To deliver broadband, an Internet service provider (ISP) typically uses a mixture of technologies, such as satellite communications, fixed-wireless, or fiber-optic cables. Wired technologies work best in densely populated areas, such as cities. For remote locations, satellite connectivity, in conjunction with some terrestrial technology, is more cost-effective. Among terrestrial technologies, fixed wireless in mmWave works well for high-speed, short-range access. Lower frequencies propagate further and are more suited to provide broadband in rural areas.
Long-range, affordable broadband access can be provided using unlicensed spectrum and open-source technologies, and can bring down the cost of Internet access. A few promising technologies include:
- TV white spaces: Unused TV spectrum can be leveraged to opportunistically send and receive data. Since most TV channels are unused in rural areas, this provides large amounts of available capacity for broadband connectivity.
- LEO satellite-based Internet access, such as from SpaceX, is another promising technology. It is relatively inexpensive to launch a swarm of cube-sats, such as those being launched by SpaceX, which can then provide Internet access worldwide.
- Private LTE: Private cellular networks, such as Endaga, use community cellular networks to bring connectivity to rural areas.
There has been other recent work on leveraging airplanes4 and Google’s Loon balloons for delivering connectivity. However, any connectivity technology solution must be accompanied by innovative business models to make them economically viable for smallholder farmers. For example, an ISP might provide digital agricultural advisories in addition to Internet access to offset the cost of deploying a communication tower and infrastructure.
Another promising technology is edge computing. Not all data needs to be sent to the cloud. Instead, large amounts of it can be processed on a computing device closer to the farm. The edge could gather imagery, perform analytics, and provide a digital advisory service, which can also run offline. However, edge compute devices need to be low-power, ruggedized, and may, in some cases, need to run an app store for completely disconnected operation.5
A privacy-preserving, secure, and sovereign data-sharing platform can help aggregate data, both for creating market linkages and for delivering AI-based advisories. However, sharing geolocation attributes, such as farm parameters, weather, or farm management, might reveal the producer’s identity. Technologies such as Confidential Compute Framework and homomorphic encryption are promising, but need to be adapted for geo-spatial data.
Computer human interfaces: Improving usability. To reach producers who are not very tech savvy, who typically have a feature phone, and who have literacy challenges, we need to look beyond a graphical user interface (GUI).15 There has been work with using non-GUI interfaces, such as those based on speech, haptics, and gestures. The two most common non-GUI user interfaces for agriculture that have been explored are speech and haptics/gestures. The latter is useful for robotics and autonomous vehicles, while speech has been studied as an interface for farmers in India and Africa.
Startups use different user interfaces to reach smallholder producers. Digital Green uses video to educate growers about farming techniques. Awaaz De uses audio, missed calls, and text messages to send notifications and alerts to producers. Many others use either SMS messages, voice calls, or both.
The latest advances in voice technology, which automates and personalizes messaging apps; bots; and speech-based agents, such as Alexa, Cortana, Siri, or Google Voice, can help reduce barriers to digital service access. However, these speech AI models must be customized to learn local dialects and mannerisms. This is not trivial; there might not be enough data to train AI models. Research challenges include:
- Support for regional languages: Growers in rural communities typically only know their regional languages; therefore, the user interface must be trained for every region.
- Two-way interactions: Several interactions, such as price discovery and digital advisories, require two-way communication between the grower and the service provider. Text messages are too short, while speech is often used only to convey information to the grower.
- Geo-spatial insights over text messages or speech: A digital advisory might have a geo-spatial component, such as the geo-coordinates of crop stress, or a micro-scale nitrogen map of the farm. In the developed world, this insight is conveyed using 2D or 3D maps. Conveying the same information over text messages or speech is challenging.
Summary
This paper highlights the innovations that are needed across different areas of data science and computer science to enable digital agriculture for small-scale producers. This includes research across various ACM Special Interest Groups (SIGs), such as SIGCOMM, SIGOPS, SIGARCH, SIGCHI,
SIGGRAPH, and many more, to devise affordable hardware for cloud and networking solutions, with innovations in AI to handle sparse and incomplete data.
As technology solutions emerge, it is also important to understand the path to market and scalable adoption. As such, we are working on a Digital Agriculture Technology Readiness Index6 and calculator to evaluate the maturity of different digital technologies for small-scale agriculture in Table 2. The output of this index will guide our efforts towards accelerating research in technologies that are not yet ready for adoption and investing in technologies as they mature. We are excited to work with the computer science community to address some of the hardest problems, so that we may help accelerate the adoption of digital agriculture for smallholder producers, improve the livelihoods of millions of families, and ensure greater global food security.
Join the Discussion (0)
Become a Member or Sign In to Post a Comment