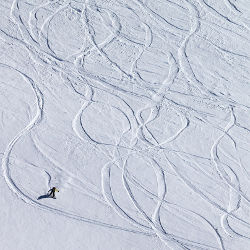
We hear a lot about how big data, smart devices, and all the ‘-omics’ (for example, genomics, proteomics, metabolomics, and so forth) are going to transform medicine—and they will. But there is another force that is going to change the way we think about and practice health, and that is our small data—small data derived from our individual digital traces.
Consider a new kind of cloud-based app that would create a picture of your health over time by continuously, securely, and privately analyzing the digital traces you generate as you work, shop, sleep, eat, exercise, and communicate. While there are personal devices and Internet services specifically designed for self-tracking (Fitbit, Patients like me, http://quantifiedself.com, and so forth), digital traces include a much richer corpus of data that we generate every day, just by virtue of our normal activities. And while the use of electronic health records is increasing, today’s systems capture data reported by clinicians, not patients; and data about clinical treatment, not day-to-day activities.
We generate these data because most of us mediate, or at least accompany, our lives with mobile technologies. As a result, we all leave a continuously updated "trail of data breadcrumbs" behind us, which together make up our digital traces. You all are generating such traces now, as you do when you wake up and perhaps read email before you even got out of bed, or when you decide to take a walk after work instead of staying home and frequenting your refrigerator and couch.
The social networks, search engines, mobile operators, online games, and e-commerce sites we access every hour of most every day extensively use these digital traces we leave behind. They aggregate and analyze these traces to target advertisements and tailor service offerings and to improve system performance. But most services do not make these individual traces available to the person who generated them; they do not yet have a ready-made vehicle to repackage their data about you in a useful format for you and provide it to you. But they should, because this broad but highly personalized data set can be analyzed to draw powerful inferences about your health and well-being from your "digital behavior."
To be clear, I am not talking about apps doing detailed medical diagnosis, and I am not talking about replacing the insight and role of doctors or loved ones, nor am I discounting the importance of our own self-awareness. Instead, use of these traces could serve to greatly enhance all of those with personalized data-driven insights—insights ranging from early warning signs of a problem, to indicators of gradual improvement. Ginger. io (http://ginger.io) refers to this sort of services as a check engine light. Another way to think of it is as a personalized "behavioral pulse." A signal that can indicate subtle but significant changes in a person’s well-being by representing changes in day-to-day behavior, in a manner that is comfortable to share with a select number of friends or family.
Once I, as a patient and consumer, can access the data that service providers have collected and stored about me, I can then use these data to fuel apps I choose to subscribe to. For example, imagine an app that helps my doctor determine whether the new medication dosage I have been taking for the last two weeks is better for me than the previous dosage. The app could create a comparative picture of my daily function this month relative to last month by automatically analyzing motion, location, and vocabulary data plucked from my digital traces. Or, I could see, from an app running over my location traces that I get back from AT&T or Verizon, if the supplement I am taking for my early-stage arthritis is actually helping me get out and about more quickly most days; and if overall I am less sedentary than I was previously.
From chronic pain to depression to memory enhancement and Crohn’s Disease—many chronic conditions have a lot of day-to-day variability, with confounding factors. Moreover, both good and bad changes are gradual. Consequently, it is difficult for me as an individual to reliably and precisely track the effect of a new treatment based only on my subjective and selective memory. But these same health conditions have symptoms and side effects that show in our functional, everyday, behaviors—and for the first time really, our everyday behaviors are becoming data. While that might be disconcerting at times, it is the case; and what I am arguing for is that we as individuals should have access to our digital traces so that we can mine them for our own purposes.
You will be the customer for the data about you; I will be the customer for the data about me.
And we can do this for the young and old alike, because while we do not usually think of elders as digital natives, they do increasingly carry cellphones (even if only simple phones); and they increasingly use the Internet (even if only via their TV). Both simple phones and cable TV boxes are potential sources of digital traces! And, of course, as we become the elders of tomorrow we will carry with us our existing digital practices and addictions into our senior years. When I think back to my father’s final few months of life, I can identify signals that indicated that something was wrong, signals that could have shown up in his digital behavioral pulse if one had been available. He suddenly stopped sending email (and this was a man who had been using email on the Arpanet since the mid-1970s), and his daily patterns gradually changed so that he no longer shopped at the supermarket to prepare food at home for my mother, and he took shorter and shorter neighborhood walks. His declining condition was not detectable on his regular visits to his cardiologist since it did not show up in his EKGs, or traditional exchanges about how he felt, and he like others "pulled it together" for his favorite doctor. On an emergency room visit one day, the attending doctor observed nothing atypical for a 90-year-old man; nothing in his vitals or his electronic health record communicated to the emergency room doctor that this 90-year-old man was behaving entirely differently than he was just a few weeks earlier—a behavioral pulse graph, derived from his digital traces, could have. Having access to my father’s ‘digital behavioral pulse’ would not have changed the outcome; but it would have given us the tools to track these changes and communicate them objectively to members of his medical team.
Fortunately, I have a "real" doctor in the family—my eldest sister Margo—and her insight and vigilance in keeping detailed track of my parents’ medical history and day-to-day activities effectively created a behavioral pulse for my father, but most families do not have a ‘Margo’. So, what I am suggesting is that we begin to leverage our small data to bring more vigilance and insight to everyday care. We can think of this as new kind of medical evidence, evidence where n=me, because it complements traditional big-N population studies with data that are just about me (or you) over time. And what is so compelling about this approach is that these data already exist. It does not require deployment of any new hardware, so we can start leveraging our small, n=me, data now.
So, if the raw data are there, what is left to do to make small data and n=me become the standard of care? First and foremost, I do not in anyway want to trivialize the work that will be needed to convert these noisy sources of data into actual insight—that is where we will see much of the iterative innovation in the coming years from the computing community in particular. But it will not happen until we can start tapping into our own data. Therefore, our first step has to be what Todd Park refers to as data liberation: we need to liberate our data from mobile and Internet services, to you and me. We need a common (open) architecture so that a rich market of apps and services can grow around our n=me data in the same way the HTTP standard created the World Wide Web with its myriad apps and services.
Admittedly, some service providers are apprehensive about whether customers will be put off once they see how telling their digital traces are and worry it will create a public relations nightmare. But the data are already being captured for the most part, and in the long run consumers will know what is going on anyway. Perhaps transparency will lead to a more robust and sustainable basis for privacy. Assuming we overcome such disincentives, where are the positive incentives for commercial service providers to cooperate and make digital traces available to the individual? The economics of the market seem to be on our side. On the cost side, these digital traces are already recorded by the service providers so the added cost of providing small data to the customer can be quite low. In terms of benefits, if standard interfaces to personal digital traces spark a cottage industry of app makers who process small data and put it to work for subscribers, then implicitly they could increase the value of the consumers’ engagement with the underlying digital services; in the same way that mobiles apps greatly increased the value to consumers of smartphones. In other words, the business case for the service providers could be one of marketing and sustaining customer engagement, as well as in opening up new service offerings based on their own new, smalldata and personal data repository, offerings.
Again, it is never as simple as just getting the data. We face intriguing technical and design challenges in making sense of that data for the users, and we have regulatory challenges in navigating and adapting FDA, HIPAA, and privacy policies; for example, whether to treat this data as medical data or something more akin to personal diaries. But I do not think any of these are showstoppers; if we start the flow of n=me data, we can make the right things happen, and in the right way.
With my colleagues at Open mHealth (http://openmhealth.org) and Cornell Tech (http://tech.cornell.edu), we are building prototypes that demonstrate the power of small n=me data, and we are developing standard interfaces that service providers, app creators, and science researchers can use to build the applications that will process, fuse, and filter your small data for you. We have created a website to let you tell service providers we want our digital traces formatted and made available to us: http://smalldata.tech.cornell.edu. You will be the customer for the data about you; I will be the customer for the data about me. Let’s get our search engines, social networks, and mobile carriers, to start packaging our small data, for us.
Further Reading
Acquisti, A. and Heinz College.
The Economics of Personal Data and the Economics of Privacy. Background Report for The Economics of Personal Data and Privacy: 30 Years after the OECD Privacy Guidelines.
Becker, R. et al.
Human mobility characterization from cellular network data. Commun. ACM 56, 1 (Jan. 2013), 74–82
Campbell, A.T. et al.
The rise of people-centric sensing. IEEE Internet Computing 12, 4 (Apr. 2008), 12–21.
Estrin, D. and Sim, I.
Open mhealth architecture: An engine for health care innovation. Science 330, 6005 (2010), 759–760.
Kang, J. et al.
Self-surveillance privacy. Iowa Law Review 97 (2012), 809.
Kleinberg, J.
Bursty and hierarchical structure in streams. In Proceedings of the 8th ACM SIGKDD Intl. Conf. on Knowledge Discovery and Data Mining, 2002.
Kumar, S. et al.
Mobile health: Revolutionizing healthcare through transdisciplinary research. IEEE Computer 46, 1 (Jan. 2013), 28, 35.
Miller, G.
The smartphone psychology manifesto. Perspectives on Psychological Science 7, 3 (2012), 221–237.
Morris, M. et al.
PIXEE: Pictures, interaction and emotional expression, ACM CHI 2013 Extended Abstracts (Apr. 27–May 2, 2013, Paris, France).
The Organisation for Economic Cooperation and Development. Exploring the Economics of Personal Data: A Survey of Methodologies for Measuring Monetary Value.
Tucker, C.
The economics value of online customer data. Background report for The Economics of Personal Data and Privacy: 30 Years after the OECD Privacy Guidelines.
https://www.google.com/settings/activity
http://www.weforum.org/issues/rethinking-personal-data
http://blog.stephenwolfram.com/2012/03/the-personal-analytics-of-my-life/
http://web.media.mit.edu/~sandy/
http://www.amia.org/amia2011/keynotes
Join the Discussion (0)
Become a Member or Sign In to Post a Comment