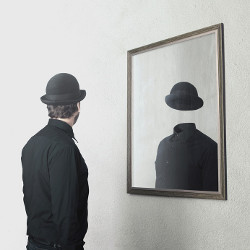
Many scholars and educators argue the antidote to some of the ethical problems with artificial intelligence (AI) is to integrate ethics and AI or embed ethics in AI.2,12,14 The product of this combining is supposed to lead to Ethical AI, a term that is both frequently used and seemingly elusive.5,9,13 Although attempts to make AI ethical are to be lauded, too little attention has been given to what it means to "integrate" or "embed," be it integrating ethics and AI or embedding ethics in AI.
A rather simple idea of additivity seems to be behind these proposals. That is, the efforts are directed toward figuring out how ethical principles can be "injected into"11 AI or how an ethical dimension can be "added to" machines1 or, if the focus is on the latest wave of machine learning, how to "teach" machines to act in an ethical way.10 The common vision of such proposals is ethical components can and should be added to existing AI systems. Representing this vision with an equation gives us Ethics + AI = Ethical AI.
However, the truth of this equation is questionable. Additivity between two entities requires ontological likeness. Adding ethics and AI is based on ontological assumptions about what AI is and what ethics is, namely that the two entities are of the same nature or, at least, some of their components are.
As a discipline, AI was born as a subfield of computer science, so naturally AI artifacts, such as algorithms (for example, resolution for automated reasoning), models (for example, artificial neural networks for machine learning), software (for example, the Eliza chatbot) or hardware (for example, neuromorphic chips) are all computational in nature. Among the founders of the discipline, we may even find computational stances regarding human intelligence.8 Questions on the nature of human intelligence aside, all the artifacts that ethics is supposed to be "injected into" or "added to" or "taught to" are software running on computers, that is, bona fide computational entities. If the nature of AI in the Ethics + AI addition is computational, then this seems to entail that ethics or, at least, the ethics that we add to AI must be computational as well. In other words, if AI is a piece of software, then for that software to become ethical it will have to include instructions that express ethics in computational language.
Whether ethics is, at its core, computational is a deep question and there are good reasons to be skeptical. Those who are now trying to code ethics tend to think of it as rules or principles or theories. This way of thinking is attractive because rules, principles and theories are amenable to formalization, and formalized systems can be translated into computational ones, some theoretical boundaries on the completeness of such translation notwithstanding.4 However, people (individuals, societies, cultures) do not generally act on the basis of adherence to philosophical moral theories, and although individuals may acknowledge and embrace moral rules and principles (for example, respect human life, treat others fairly), rules and principles must always be interpreted and applied.
Ethics eludes computation because ethics is social. The concepts at the heart of ethics are not fixed or determinate in their precise meaning. To be applied they must be interpreted, and interpretations vary among individuals and groups, from context to context, and may change over time. Yes, we all agree that respect for persons, fairness, and keeping promises are good or even essential to ethical behavior, but in real-world situations and particular domains, these concepts require interpretative specification. For example, in medicine, analysis is required to figure out what respect for persons could mean for doctor-patient relationships. It was not until the 1970s that respect for persons was translated into informed consent and doctors later were prohibited from experimenting on patients without it. Privacy is an even more complex concept with a good deal of controversy about how it can or should be protected in practice. Many companies have interpreted it to mean they must have privacy policies containing elaborate details that customers never read but agree to by default; others argue that this interpretation is not an adequate understanding of privacy. Sometimes social consensus on an interpretation leads to a law, for example, equality and justice mean (among other things) non-discrimination; other times social consensus leads to informal social conventions, for example, keeping one's promises is implicit in many interpersonal relationships; and yet other times, the interpretation of a social value continues to be contested and unsettled, for example, how is universal suffrage to be achieved.
Even though ethical concepts are not amenable to computational expression, there are often computable dimensions to ethical problems.
Because ethical concepts require social interpretations, they are subject to disagreement, contestation, and change. No computational model can capture all the possible interpretations that can constitute the social meaning of an ethical concept. As such, ethical concepts are not conducive to computational expression. Some may argue that a computational model could count as a particular interpretation of an ethical concept, but even that would only be the case if there were social understanding and acceptance of the meaning of that interpretation.
Nevertheless, even though ethical concepts are not amenable to computational expression, there are often computable dimensions to ethical problems. Computability is the characteristic of problems that can be described in a mathematical form that is compatible with the operations of a computer. Computable problems can be given to a computer as input, and computation can provide solutions to them. Such solutions can solve part of an ethical problem, but not all of it, since the problem will have aspects that elude computation. In other words, the role of computation in solving ethical problems will be limited in scope; it will not be able to incorporate ethical notions which depend on variable social agreement or ethical ideas that have been interpreted in diverse and contested ways or may be in flux.
We can continue to think about AI as merely computational artifacts, but we should acknowledge there is another, more complex notion of AI.
Consider the following example. Accusations of bias in algorithmic systems are usually based on ideas about equity, fairness, and non-discrimination. One context in which this arises is in recruitment and hiring and within this context, one area that is often thought to be solvable by algorithms is in the distribution of job advertisements.7 The basic idea is that an equal distribution of ads among all demographic categories ensures a fairer job market for example, one that does not discriminate. However, although an algorithm can produce a distribution of data (ads) that represents an interpretation of equality, this does not necessarily make the hiring system fair. For one thing, whether or what kind of equity is achieved depends on the demographic categories and venues used in the computational distribution. For another, the distribution of ads is only one part of fairness of job markets; the rest requires non-computational strategies, be they organizational, governance, design and use practices, social change or all of these factors.
This means ethics cannot be added to AI—if AI is understood to be computational artifacts—because ethics cannot be understood as purely computational. However, there is another way to conceptualize AI. AI artifacts are generally used in contexts in which they are part of social practices that involve human behavior, goals, and norms. An important dimension of this is that the output of an algorithm computed by a machine has significance and efficacy only insofar as human beings attach meaning to it and use it.
Borrowing a concept that is a staple of Science, Technology and Society (STS) studies, technologies, including AI are more productively understood as sociotechnical systems, that is, systems in which artifactual behavior is combined with human, social, and organizational behavior3 to produce results. Viewing AI as sociotechnical systems provides a broader scope to our understanding of AI. It does not deny or ignore the fundamental and defining contribution of computation to AI, but it takes into consideration the important relations that hold between AI artifacts and the people who design them, those who deploy them, those who make policies about them and, ultimately, those who use them. Neglecting these relationships is an oversight that we named sociotechnical blindness.6
Of course, we can continue to think about AI as merely computational artifacts, but we should acknowledge there is another, more complex notion of AI. With the sociotechnical systems understanding of AI, AI artifacts are understood to be components in systems that are constituted by social practices, social norms, and social meanings as well as the computational artifacts. With the sociotechnical systems concept of AI, AI has ethical significance. Returning to our job recruiting example, the algorithm may distribute ads equally but whether "equally" constitutes fairness or non-discrimination depends, as mentioned earlier, on the nature of the categories as well as such factors as the content of the ad, how many likely qualified individuals have internet access, how people and disciplines think about discrimination, and so on and on and on.
The two concepts of AI—one narrow and only referring to the computational artifacts and the other broader and including the social arrangements in which AI artifacts operate—should not be conflated; the lens of ethics can only be turned to AI understood as sociotechnical systems. An AI artifact cannot be ethical or unethical, good or bad, biased or unbiased. With the broader concept of AI, AI artifacts can be understood as having an ethical dimension, not per se, but as part of a sociotechnical system.
The challenge for AI experts is to acknowledge that the organizations and industries in which algorithms operate are far from morally perfect.
Many of the current notions of Ethical AI miss the mark on this, not thinking about AI as sociotechnical and not acknowledging that AI has ethical dimension only insofar as it affects social relationships and arrangements and impedes or furthers social values. Ethics cannot be added to AI artifacts but such artifacts can be components in systems that have ethical significance, that is, systems that can be evaluated in ethical terms. Does the job recruiting system fairly distribute job advertisements? Does the parole system that determines which convicts are eligible for parole do so without discrimination? Do social services decide the eligibility of applicants accurately? The algorithms that these organizations use in making their decisions are part of the ethical question but they are only part of and only ethical in conjunction with other practices in the system.
The crux of the matter is that we cannot have ethical AI unless we have ethical domains or industries in which AI algorithms operate. If the norms by which a company or industry operates are unfair then the AI artifacts that instrument some of those activities will be unfair, and we will not be able to right that wrong only by means of computation.
The challenge for AI experts is to acknowledge that the organizations and industries in which algorithms operate are far from morally perfect. Indeed, there are no morally perfect systems (though some are better than others with respect to specific criteria). Seeking a better, fairer, more just and more humane world is a project, a project which AI experts can (and many already do) embrace, and a project for which they have a great deal to contribute. However, this generally involves more than working with machines, albeit intelligent machines. AI experts must pay attention to, and critically examine, the operations of the enterprises for which they are designing AI and try to improve on them. The interpretive fluidity and potential contestation of the ethical concepts make this especially challenging. Nevertheless, despite all difficulties and no promise of success, striving for Ethical AI is the right thing to do.
Join the Discussion (0)
Become a Member or Sign In to Post a Comment