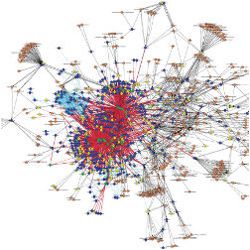
Health organizations in Europe and the U.S. are pushing ahead with plans to compile massive databases of patient information, which they anticipate will not just improve treatments and survival chances for cancer sufferers, but will drive the development of new therapies. These moves will involve many more participants in massive clinical trials than today, and will allow medical centers in the developed world to expand their reach globally.
One organization, the American Society of Clinical Oncology (ASCO), aims to have ready by 2015 the production version of its CancerLinQ ‘learning health system,’ building on the experience of a 170,000-record prototype unveiled in 2013. At a White House event in November 2013, Thomas Kalil, deputy director for technology and innovation at the White House Office of Science and Technology Policy, identified CancerLinQ as one of a number of healthcare systems that would aggregate large quantities of data to improve treatment.
“There are huge opportunities to both improve health outcomes and lower costs,” Kalil said, claiming CancerLinQ would allow every patient’s experience to help inform future cancer care. “Currently only 3% of patients participate in clinical trials. ASCO is committed to figuring out how to use the data, while protecting patient confidentiality of the other 97% to make our healthcare system much more of a learning system, to improve the quality of care and accelerate the development of new therapeutics.”
Dr. Peter Campbell, head of cancer genetics and genomics at the Wellcome Trust Sanger Institute, said at the Oncopolicy Forum 2013 held in Amsterdam, The Netherlands, last autumn that aggregating data into large, online databases would help drive the advent of personalized medicine, providing an opportunity to treat serious illnesses more effectively. “We are standing on the cusp of an era where we can characterize all patients and what drives their cancer.”
Cancer is the primary target, partly because of its prevalence. According to the American Cancer Society, cancer remains the second most common cause of death in the U.S., accounting for almost one in four deaths. The European situation is similar; Tonio Borg, European Union commissioner for health, says, “We expect that in the EU, one in three men and one in four women will be affected by cancer before reaching the age of 75. Cancer is not something that only affects others; it happens to everybody.”
The other reason for using population-scale databases to collate and process patient information is due to cancer’s nature. Campbell says cancers have huge variations that result from the many different ways in which the DNA of tumor cells can mutate in different patients. In breast cancers, for example, analysis of tumors reveals the most commonly mutated or deleted genes were found in just 10% of affected patents. “There is a long tail of other mutations, each affecting just a few percent or less of patients,” Campbell explains.
With diseases such as cancer, genetic changes cause some biological feedback loops and other processes to break down in unpredictable ways. Researchers have found that even within the same tumor, cells may be altered in different ways, so a therapy that works for a large group of patients may be utterly ineffective for the one sitting in the doctor’s office.
The dream of personalized or stratified medicine, says Campbell, is to prescribe treatments for a patient’s specific condition and “melt away the cancer.” The problem is obtaining enough data to work out how different treatments fare under different conditions.
“I would say that interventional clinical trials are underpowered to detect gene-drug interactions. If a particular mutation is only found in one in 700 patients, you need to screen that many just to find one participant for a clinical trial for a drug that targets it,” Campbell explains. “We do not have enough patients to study. We need several thousand patients, maybe tens of thousands of patients. For any given tumor type, we need a database of 10,000 to 20,000 patients, and with 50 to 100 common tumor types, that means access to the records of at least one million patients.”
Dr. Sandra Swain, former ASCO president and medical director of the Washington Cancer Institute at MedStar Washington Hospital Center, says significant groups of patients are not represented well in the existing clinical-trial structure. Seniors, for example, “have diseases associated with getting older; that changes how we need to evaluate the patient in front of us.”
Another issue is how medical practitioners can sift through data available in principle, but inaccessible in practice.
To improve physicians’ access to relevant medical data, the University of Texas MD Anderson Cancer Center is using an IBM supercomputer running artificial intelligence software originally written to allow such a machine to compete on the “Jeopardy!” game show. IBM’s Watson supercomputer uses statistical natural-language processing techniques to work out which pieces of information are relevant to a problem. The Oncology Expert Adviser software is being used to analyze patient data, as well as research data from cancer trials at the Center.
Dr. Hagop Kantarjian, chair and professor in leukemia at MD Anderson, says the center’s Watson-based system “will be a transformational tool. Today when we see patients with cancer, we rely on our memory and our limited amount of knowledge to work out the next best step.” By presenting information from a wide range of sources, Kantarjian says, the Oncology Expert Adviser could be “a quantum leap in the way we treat patients and how we provide care and knowledge.”
For its CancerLinQ prototype, ASCO used an approach based on a combination of open-source software and the Galileo Analytics’ Galileo Cosmos data-mining software, which accessed and analyzed the preprocessed data. Preprocessing software uses statistical functions and an artificial neural network to learn, structure, and map data fields on the original records to the format the system needs.
Dr. Clifford Hudis, president of ASCO and chief of the breast cancer medicine service at New York’s Memorial Sloan-Kettering Cancer Center, said CancerLinQ lets physicians see the results of interventions on other patients who fit a given profile, to help them determine the most appropriate course of action. “The clinician isn’t just a robot doing what the computer says,” he stresses.
Kantarjian says of the Oncology Expert Adviser, “This kind of data gathering and analytical tool will allow discoveries that we cannot do today. Suppose a group of patients take a medicine that is not for cancer but is, say, a heart medicine, and that medicine also influences the sensitivity of their particular tumor to the effect of the cancer treatments. We are going to discover through these analytical tools very quickly that this helps the patients, and we can apply that to future treatment programs.”
Dr. Emile Voest, chair of the Center for Personalized Cancer Treatment at the medical research institute UMC Utrecht in The Netherlands, which has its own data analysis system, warns of putting too much faith in findings extracted from large-scale databases to prescribe customized treatments “off-label” without additional research.
“Some people say 75% of patients can now be treated with something because of the genetic information we now have. I tend to disagree because, frankly, we do not know; we can only postulate. It may work, but I am very much against treating patients off-label outside of clinical studies because we are going to make a mess of it,” says Voest.
An earlier attempt to use genetic data for treatment selection failed. Clinical trials started in 2007 after researchers at Duke University published results suggesting tests using arrays of chemical DNA probes could help select viable treatments. Later research found those results could not be reproduced, and a group of 30 bioinformaticians and statisticians urged the National Cancer Institute (NCI) to suspend those trials, which it did.
If not used directly to create customized off-label treatments in the doctor’s office, the mined data is likely to be utilized to inform basic medical research, particularly in the emerging field of systems biology, which uses computer modeling to predict biological behavior.
Says Andrea Califano, chair of the department of systems biology at Columbia University, “Instead of using statistical associations, we are starting to go towards a model-driven type of science where we create regulatory models of cells.”
Systems biology regards biological cells as a form of signaling network, a dynamic system in which proteins and genes interact in complex feedback loops. A number of cancer scientists believe combination therapies may provide the key to dealing with cancers currently difficult to treat directly.
Even within the same tumor, cells may be altered in different ways, so a therapy that works for many patients may be utterly ineffective for the one sitting in the doctor’s office.
The complex network inside a cell can provide a way to attack cancers more effectively. A single drug may target only one part of the network, perhaps inactivating a single protein, but that is only effective if the cancerous cell is disabled by that step. In many cases, changes made to the network by knocking a protein out of action will uncover new signaling pathways that help the cell to survive. Computer modeling can help identify the most productive targets, and combinations of them, to increase the probability of the treatment’s success.
Campbell argues, “Not only are many genes involved; many are not easily actionable. Many of them are essential: you cannot drug them without toxicity. For example, kidney cancer is almost entirely driven by tumor-supressor genes, and those are notoriously hard to drug.
“A further issue is that many of these genes interact with each other,” Campbell adds. “You can see patterns of genes that are co-mutated with each other. When pairs of genes are significantly mutated, they often interact strongly; that is clearly important and will probably play out in most cancers. These secondary mutations will probably have a strong impact on the patient’s treatment.”
Although the aim of large-scale medical learning systems is to have automated data collection and parsing, the reality is more complex. Dr. Peter Johnson, chief clinician at Cancer Research U.K., says full automation cannot be guaranteed, based on his experience with the UK pilot scheme. “What we are aiming to do is get automated data collection and extraction, but the complexity is such that a lot of the collection and extraction is manual,” says Johnson.
As CancerLinQ moves closer to production, ASCO expects some manual processing will be necessary, at least in early versions of the system. Says Dr. Robert Hauser, senior director of ASCO’s quality department, “Oncology is very complicated, and therefore will require a lot of manual interpretation and intervention in the beginning. We believe over time that manual intervention efforts will decrease as the system learns.”
As these systems grow to incorporate millions of patient records, organizations such as MD Anderson see them as a way to extend their reach globally. MD Anderson president Dr. Ronald DePinho points to a recent Institute of Medicine report that catalogued large disparities in cancer care across the U.S. Outside the U.S., DePinho says, “We have medical deserts on a global scale.
“Our mission is to end cancer, here and around the world. Our platform allows us to democratize MD Anderson care. We can go where the patients are, to places where a doctor hasn’t read a paper for 10 years.”
Collecting and processing the data is the beginning of a process that could link cancer treatment around the world.
Further Reading
Basso, K., Margolin, A.A., Stolovitzky, G., Klein, U., Dalla-Favera, R., Califano, A.
Reverse engineering of regulatory networks in human B cells, Nature Genetics 37, 382–390 (2005)
Gonzalez-Angulo, A.M., Hennessy, B.T.J., Mills, G.B.
Future of Personalized Medicine in Oncology: A Systems Biology Approach Journal of Clinical Oncology, vol 28, no. 16, June 1 (2010)
Tsimberidou, A.M., Ringborg, U., Schilsky, R.L.
Strategies to overcome clinical, regulatory, and financial challenges in the implementation of personalized medicine 2013 ASCO Educational Book http://meetinglibrary.asco.org/EdBookTracks/2013%20ASCO%20Annual%20Meeting [Open Access]
Godman, B., et al.
Personalizing health care: feasibility and future implications BMC Medicine, 2013, 11:179 http://www.biomedcentral.com/1741-7015/11/179 [Open Access]
Figures
Figure. In 2011, researchers at Columbia University Medical Center built this model of the gene regulation network in mammalian cells that they used for studies into the genetic variability of cancer.
Figure. The Kaplan-Meier plot of survival chances versus time, commonly used to gauge treatment efficacy for patients, shows how a cancer mutating from a frequently encountered form may develop increasing resistance to standard drug treatment.
Join the Discussion (0)
Become a Member or Sign In to Post a Comment