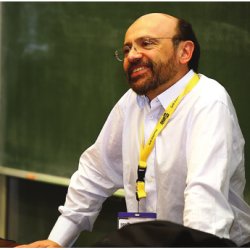
The rapidly changing advertisements that appear on Web pages are often chosen by sophisticated algorithms that match ad keywords to words on a Web page. Take the Chevy ad, for example, that frequently appears on your favorite news site. A real-time ad network at one of the major search engines—Google, MSN, and Yahoo!—might place it on a page of automotive news. But what if the news page’s featured article is about a tragic accident caused by a mechanical failure in a Chevy SUV? That’s not a page General Motors wants to be associated with, let alone pay good money to advertise on.
Costly mishaps like this could be avoided by a new discipline called computational advertising, which seeks to put the best ad in the best context before the right customer. It draws from numerous fields, including information retrieval, machine learning, natural-language processing, microeconomics, and game theory, and tries to match ads with a variety of user scenarios, such as querying a search engine, reading a Web page, watching a video on YouTube, or instant messaging a friend.
Computational advertising could spur the Web’s growth as a medium of mass customization. Better ad matching could quicken the trend toward personalization, making highly specialized magazines, Web sites, and TV channels more financially viable. “Advertising has been the engine that has powered the huge development of the Web,” says Andrei Broder, fellow and vice president for computational advertising at Yahoo! Research. “Without advertising, you would not have blogs and search engines.”
Computational advertising is a type of automation that tries to replicate what humans might do if they had the time to read Web pages to discern their content and find relevant ads among the millions available. “In the old world of advertising, they deal with few choices and large amounts of money for each choice,” Broder says. “We deal with maybe a hundred million potential ads, each worth a fraction of a cent.”
A Perfect Match
There are basically three kinds of Web ads. Sponsored search ads are matched to the results of search engine queries; banner ads target particular demographics and venues, typically without regard to a page’s content; and contextual advertising, also called context match, applies to other types of Web pages, such as the home page of a financial news site. Computational advertising addresses all three types of ads.
Google, MSN, and Yahoo! use electronic auctions to assign ads to their own results pages and the pages of other Web sites. “Google is a yenta,” or matchmaker, says Google chief economist Hal Varian. “The goal is to get a perfect match.”
In sponsored search, advertisers bid to place ads that contain keywords correlated to words in a user’s search string. For contextual advertising, the keywords are related to words on the entire page, and the search engine’s advertising service places the ads. For banner ads, online ad networks place ads on sites whose topics and audiences match the advertiser’s criteria.
Before the advent of computational advertising, ad engines could make mistakes more simple-minded than the Chevy SUV scenario. Suppose, for example, a news page contains the word “flowers.” If the article isn’t about flowers but instead revisits the Rolling Stones’ underrated 1967 record Flowers, the reader is unlikely to want ads from florists. The old method of analyzing co-occurring words and phrases doesn’t help much, and neither does frequency. “You could extract a word used many times in the article and it still is not what the article is about,” Broder says.
Therefore, Broder and the 30 researchers who work for him are finding ways to glean the meaning of a page. One promising avenue combines semantic and syntactic features. A semantic phrase categorizes the page and the ads into a 6,000-node topic taxonomy and compares the proximity of the two types of classes as a factor in ranking ads. The hierarchical taxonomy also improves the matching of ads that don’t fit a page’s exact topic. Keyword matching is still needed to capture more granular content, such as a specific brand of automobile. “We decided that what the article is about should count for about 80% and the words should count for 20%,” Broder says.
Another area of interest is using statistical analysis to measure the effect of exogenous events on browsing behavior and adjust the advertisements accordingly. Varian cites short-lived examples, such as this year’s rare snowfall in England, or longer-term ones such as the worldwide recession. “In the last few months, there is a big increase in interest in price-sensitive products,” Varian says. “The advertisers, in turn, are trying to respond.”
All three companies are close-lipped about which of their research has been commercialized, but say that new ideas for algorithms are quickly incorporated into their bidding mechanisms and advertiser tools. Bottom-line results are secret, but the search engines all collect metrics such as revenue per search.
Machine learning, another major focus, concentrates on training algorithms to scan pages for meaning, a technique employed successfully on single-topic documents with the aid of machine-generated labels, but trickier to perform on Web pages, with their assortment of graphics, text, and topics. Microsoft researchers have learned how to employ a type of multiple instance learning to automate classification of sub-documents on pages with incomplete labels and to detect the presence of certain types of content.
“Most of what we do can be boiled down to understanding intent,” says Eric Brill, general manager of Microsoft adCenter Labs. By analyzing search strings, for example, algorithms can predict if a person is interested in ads. Some strings are pure attempts at finding information, while others, such as “buy Canon digital camera,” have clear commercial intent. “When consumers don’t have commercial intent, you don’t want to put ads in front of them,” Brill says.
Much work focuses on ensuring that new bidding mechanisms don’t have incentives for advertisers to misrepresent click-through rates to get better ad placement. In the decentralized economy of the Internet, truthfulness is a currency reinforced by carefully crafted algorithms. “People are out there to make money,” says Thore Graepel, a senior researcher at Microsoft Research. “We need to build mechanisms where everyone benefits.”
One might expect the speed and volume of data to create a capacity problem, but the researchers express mixed opinions. Graepel says semantic analysis creates an extra burden. “You will hit a computational bottleneck, that’s pretty clear,” he says. To avoid this, researchers optimize algorithms to make the best decisions with the smallest possible data sets. But they also have faith in engineers’ ability to exploit techniques such as parallel processing. “It’s surprising how they are always able to scale to deal with these new algorithms,” Varian says.
Privacy regulations remain an obstacle to personalizing ads, says Graepel. The existing opt-in, opt-out model lets users choose to reveal personal data in exchange for discounts and other incentives. Researchers are also investigating aggregating data on Web traffic to more accurately match ad categories with coarsely defined groups of users who identify their interests simply by visiting certain types of Web sites.
Fortunately, there is hope for avoiding embarrassments like the ill-placed Chevy ad. Researchers at Microsoft adCenter Labs claim their sub-document classification methods can prevent incompatible ads and Web sites from ever hooking up. You might call it a reverse matchmaker, just the sort of odd little entity the Internet’s inventors might never have imagined.
Join the Discussion (0)
Become a Member or Sign In to Post a Comment