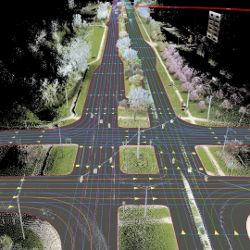
Real-time communication and collaboration lie at the heart of a new generation of high-definition (HD) digital maps that react quickly to changes in the real world. Autonomous vehicles and construction-site surveys are among the applications that are driving companies toward high-precision mapping performed almost in real time.
James Dean, founder and director of technology applications at London, U.K.-based startup SenSat, says, “Digitizing the world is incredibly important. We can make better, faster decisions from that digitized information than is possible with traditional means”
Early adopters of SenSat’s mapping technology come from the road-construction industry, a sector that today mainly relies on manual surveys conducted at ground level. Surveys can take as long as six weeks and, as a result, can only be performed infrequently during a project. A pillar built a foot out of place that is only discovered at a relatively late stage can set the project back weeks, but by sending out small, lightweight drones as flying cameras to scan the site on a daily basis, project managers can spot mistakes in the three-dimensional (3D) map long before they become a costly issue.
Regulations currently limit the area that drones can cover, which suits focused construction projects such as bridges over freeways and intersections. However, larger-scale applications, such as a proposed expansion of the capacity of one of the U.K.’s busiest roads—the M4 motorway—will require surveys to be conducted over many miles and months.
“Today, you have to keep the drone within the line of sight of the operator; that limits you to coverage of around 1km2 per hour. For economical use, you really want 20km2 per hour,” Dean says.
Still, the U.K. government is beginning to look favorably on changes to regulations that would give survey drones greater autonomy to support projects such as the M4 expansion, he says. “At the moment, it’s a supportive environment. We think it will only get better from here.”
Users of these roads, as vehicles become more autonomous, will need similarly detailed mapping to be carried out on a near-real-time basis. Although an autonomous vehicle could scan only its surroundings to see where it can drive, practical systems will use HD maps to perform the task of localization. “Using the map, we figure out where we are on the road,” says Jen-Hsun Huang, cofounder and CEO of graphics-processor company nVidia. “We want to test whether our understanding of the world is consistent with what is around the car.”
Filipe Mutz, associate professor of information systems at Federal University of Espírito Santo (IFES) in Vitória, Brazil, says the level of detail required in the map depends on its intended use: “For feature-based localization systems, a map can be represented by a sparse set of features, and it is not necessary to store a detailed 3D representation of the environment. For collision-free motion planning, on the other hand, a denser representation of the occupied and free areas is necessary.”
Mutz says the resolution of the grid used in the experimental vehicle-based mapping system built by a team at IFES is set to 20cm. “We plan to reduce the map resolution to 15cm in the near future, and our group considers 10cm the ideal resolution for safe operation in highly cluttered urban areas at reasonable speeds,” he adds.
Mapping companies such as Google, HERE, and TomTom are using fleets of similar vehicles armed with cameras and LIDAR sensors to map the roads they travel. Still, the level of detail needed for motion planning requires an immense effort.
Alain De Taeye, head of high-definition (HD) mapping at TomTom, said at the European leg of nVidia’s GTC technology conference in Amsterdam: “We have mapped 47.1 million kilometers of road, but only 120,000 kilometers is mapped in HD. And we are leading the pack. We have basic information for many of the roads today—that covers 70% of developed society—but we need to go much further and faster. You don’t want to miss centimeters in a self-driving car.
“At first, people believed a navigable map was unaffordable; now, they think HD mapping is unaffordable. It’s not unaffordable; you have to be clever about it,” De Taeye says.
Through a deal with nVidia, TomTom aims to accelerate HD map creation by applying more advanced artificial intelligence (AI) algorithms running on graphics processors in the vehicles themselves, as well as in the cloud. Huang says nVidia’s approach employs AI to work out the difference between trees, buildings, and other vehicles. “We detect all these for two reasons. Number one: we want to continuously update our map, and there are several different types of marker we can use to figure out where we are. Number two: we’re detecting where it’s safe to drive [in real time].”
With the front end of the mapping technology deployed in vehicles, TomTom and others want to gather mapping data from many vehicles to support a continuously updated map. “Crowdsourcing will be helpful. Otherwise, it would take many thousands of one’s own vehicles, like Google’s, and many miles of driving, resulting in high cost, to achieve these HD maps—and they would not be up to date, either,” says Kevin Mak, senior analyst for Strategy Analytics’ global automotive practice.
Marco Lisi, engineering manager for global navigation systems at the European Space Agency (ESA), points to handheld gadgets as rich sources of mapping data. “Whenever we carry around a smartphone, we are carrying around several sensors: a compass, accelerometer, gyroscope, and GPS. We are collecting data on our location all the time with several sensors at once,” Lisi says.
Such real-time data streams already feed back into location-driven applications such as the StreetBump app used by the City of Boston, which collects data on potholes from users—the app forwards data from the motion sensors in smartphone handsets when the vehicle they are riding in hits a bump. The Waze app uses regular updates on the location of smartphones carried by its clients as they drive around in their cars; data from the app not only highlights traffic jams, but lets the host servers estimate the number of lanes on a freeway based on the geographic spread of pings across the road collected over time.
Eric Gunderson, CEO of MapBox, sees similar data aggregated on a large scale being used to perform precision mapmaking. “What we’re getting every single day is data that represents 100 million miles of traveling. As it comes in, it just looks like noise, but as I analyze the data, the algorithm can discern the actual lanes on the highway. You can drill down this crowdsourced data all the way to put center lines on the road so I can drive the car as if it was on rails.”
A lack of standards for representing sensor data even for dedicated vehicle systems will make crowdsourcing more difficult in the short term, Mutz says. “Nowadays, most vehicles have different sensor setups and there are no established standards for the storage and distribution of that data.”
Although mapping organizations are likely to embrace standards to allow them to incorporate data from many sources, a fully open ecosystem seems unlikely, says Strategy Analytics’ Mak, who says the companies involved will prefer to maintain semi-closed ecosystems.
Some of the standards used to exchange mapping data will be mandated by government: agencies such as the U.S. National Highway Transportation Safety Administration (NHTSA) see the potential for crowdsourced data to improve traffic safety, as well as map accuracy. A car can send messages to nearby vehicles if it detects a pedestrian moving toward the road, or passes a vehicle signaling that it intends to turn across oncoming traffic.
Working with the U.S. Department of Transportation, the NHTSA said in 2015 it was speeding up plans to mandate the adoption of vehicle-to-vehicle (V2V) communication. Based on a version of the Wi-Fi local-area network protocol adapted to work in a dedicated frequency band around 5.9GHz to limit interference, V2V allows cars to share data on the environment around them. If a car detects a pedestrian moving toward the road or passes a vehicle signaling that it intends to turn across oncoming traffic, it can send messages to the vehicles following behind.
The communication need not be limited to vehicles. Says Maurice Geraets, senior director of chipmaker NXP Semiconductors, “There will be cameras at intersections that send V2X signals.” Such smart intersections will be able to indicate whether nearby cars need to slow down for a red signal or warn that a car has blocked an exit. Temporary roadside beacons will alert vehicles to the presence of roadside workers, and that can be added to local maps temporarily.
“Collaboration across many technologies is very important,” says Dean.
Yet the vehicles and their mapping software will need to be alert to the possibility of hacking, and of collaborators in data being less than honest. Lars Reger, chief technology officer of NXP’s automotive division, says without effective security, “I could put a little beacon in front of my house and transmit to the world that an accident has happened, and clear the road outside.”
Seif, H.G, and Hu, X.
Autonomous Driving in the iCity – HD Maps as a Key Challenge of the Automotive Industry, Engineering: The Official Journal of the Chinese Academy of Engineering and Higher Education Press, Vol. 2, Issue 2, June 2015, pp159–162
Carrera, F., Guerin, S., and Thorp, J.
By the People, for the People: the Crowdsourcing of ‘StreetBump,’ an Automatic Pothole Mapping App, International Archives of the Photogrammetry, Remote Sensing and Spatial Information Sciences (ISPRS), Volume XL-4/W1, 29th Urban Data Management Symposium (2013)
Harding, J., Powell, G., R., Yoon, R., Fikentscher, J., Doyle, C., Sade, D., Lukuc, M., Simons, J., and Wang, J.
Vehicle-to-vehicle communications: Readiness of V2V technology for application, National Highway Traffic Safety Administration Report No. DOT HS 812 014.
Mutz, F., Veronese, L.P., Oliveira-Santos, T., de Aguiar, E., Auat Cheein, F.A., and De Souza, A.F.
Large-Scale Mapping in Complex Field Scenarios Using an Autonomous Car, Expert Systems with Applications. Vol. 46, 15 March 2016, pp439–462
Join the Discussion (0)
Become a Member or Sign In to Post a Comment