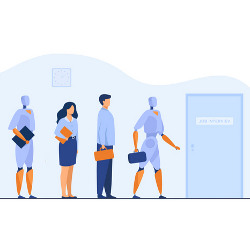
Anxiety about automation is prevalent in this era of rapid technological advances, especially in artificial intelligence (AI), machine learning (ML), and robotics. Accordingly, how human labor competes, or cooperates, with machines in performing a range of tasks (what we term “the race between human labor and machines”) has attracted a great deal of attention among the public, policymakers, and researchers.14,15,18 While there have been persistent concerns about new technology and automation replacing human tasks at least since the Industrial Revolution,8 recent technological advances in executing sophisticated and complex tasks—enabled by a combinatorial innovation of new techniques and algorithms, advances in computational power, and exponential increases in data—differentiate the 21st century from previous ones.14
Key Insights
- A full picture of automation and the future of work requires an understanding of not only technological change (the extent to which a human task is automated} but also labor demand for occupations performing a task.
- Based on changes in degree of automation and employment share from 2008 to 2020, task types are classified into five distinct categories. Our findings highlight that increased automation levels do not always lead to decreased labor demands for the task.
- Task-level automation indexes for representative occupations and U.S. cities are proposed, which allow for a systematic tracking of the labor market implications of automation technology over time.
For instance, recent advances in autonomous self-driving cars demonstrate the way a wide range of human tasks that have been considered least susceptible to automation may no longer be safe from automation and computerization. Another case in point is human competition against machines, such as IBM’s Watson on the TV game show “Jeopardy!” and DeepMind’s AlphaGo in the board game go. Both cases imply that some tasks, such as pattern recognition and information processing, are being rapidly computerized. Furthermore, recent studies suggest that robotics also plays a role in automating manual tasks and decreasing employment of low-wage workers.3,22
However, the oft-overlooked reality is that technology directly affects the demand for specific skills or tasks, instead of acting on occupations as a whole.1 Furthermore, technological advances do not always cut the labor demand for a task:2,8 Technology can automate and substitute for some human tasks, but it can also augment human intelligence and complement specific types of tasks in ways that boost demand for occupations in which those tasks are performed. The tension between automation and augmentation has been discussed in research and industry alike.29 For example, in her speech at the World Economic Forum 2017, Ginny Rometty, chairman and CEO of IBM, suggested that AI should stand for “augmented intelligence” not “artificial intelligence.”30 Thus, a full picture of automation and the future of work requires a task-level, rather than an occupation-level, understanding of not only technological change—the extent to which a human task is automated—but also labor demand for a task. To this end, we propose a data-driven methodology to scrutinize which tasks are more susceptible or complementary to automation in the 21st century, with respect to (i) changes in degree of automation and (ii) changes in employment share.
A large strand of literature on skill-biased technological change has segmented the workforce into the task types or skill clusters that are required to carry them out to assess the role of technological changes in employment and wages. For instance, Autor et al.7 classify tasks along two dimensions, routine versus non-routine and cognitive versus manual, predicting that IT contributes to automating and substituting routine tasks, which are mainly executed by middle-skilled occupations (see Acemoglu and Autor1 for details on the task-based model). Acemoglu and Restrepo2 argue that tasks are the fundamental unit of production and that the future of work depends on “the mixture of new technologies and how [they change] the task content of production.”
Frey and Osborne20 suggest that “engineering bottlenecks” create the following three categories of tasks, which are not susceptible to automation: perception and manipulation tasks, creative intelligence tasks, and social intelligence tasks. Moreover, recent studies also examined within-occupation structures of skills and human capital to provide a more granular understanding of how technology affects jobs;4,5 note that we use occupation and job interchangeably in this article. However, prior studies did not gauge the extent to which a human task is automated; at best, they have attempted to estimate an occupation’s possibility of automation and computerization.6,20 In addition, we have a limited understanding of a dynamic view of the degree of automation, despite a call for tracking the status of technological advances over time to measure and prepare for the unpredictable impact of technological advances28 (see Frank et al.18 for a discussion on the impact of AI and automation technologies on labor). Hence, the primary purpose of this article is to propose a data-driven approach to quantitatively measure the task-level degree of automation, so that researchers and policymakers can systematically track and compare it over time.
The oft-overlooked reality is that technology directly affects the demand for specific skills or tasks, instead of acting on occupations as a whole.
By representing occupational skill requirements as a network, we reveal 15 endogenous task types, which are clusters of related skills that are required to a similar extent by occupations. We then measure each task’s degree of automation in 2008 and 2020, respectively. Considering the changes in both degree of automation and employment share from 2008 to 2020, the task types are classified into five distinct categories, highlighting that the increase in automation level does not always lead to a decrease in labor demand for the task. Based on an occupation’s task content and a city’s occupational composition, we construct a task-level job automation index for representative occupations and a task-level city automation index for U.S. cities. The proposed automation indexes are found to be significantly correlated with occupational characteristics and regional characteristics for innovation capacity. Our data-driven approach to constructing task-level automation indexes helps to systematically track the trajectory of advances in automation technology, providing a granular understanding of how automation has affected occupations and communities over time.
A Data-Driven Approach to Measuring Task Automation
Endogenous task types. From the perspective of task content, we view occupation as a bundle of tasks to which workers apply skill endowments.2,7 In this regard, tasks performed by occupations are defined as clusters of related skills in occupational skill requirements.18 We employ the U.S. Department of Labor-sponsored O*NET database, which provides the importance of 182 skills, abilities, and activities (collectively referred to as “skills”) to representative occupations. In our analysis, we consider 650 occupations available consistently over the years, covering more than 96% of the total U.S. employment. Given that technological changes occur over the long term rather than annually, and the O*NET database updates about 10% to 15% of the occupations each year, we measure and compare the status of occupational task content and degree of automation as of 2008 and 2020, so that we can capture the impact of automation technology on occupations in the overall economy. With all skill ratings gradually completed across occupations from 2007 to 2009, we construct the 2008 measure by averaging those of the three years to include the most representative occupations in our analysis while minimizing any update-related biases.
To uncover the latent interdependence among skills in performing occupational tasks, we represent skill requirements by occupations as a two-mode network, namely a “job-skill network,” based on 2020 occupational data. In the job-skill network, jobs and skills are the two distinct classes, and nodes of each class are connected to those of the other class, where the weight of edge is how important a skill is to a job (denoted as w in Figure 1). Then, as illustrated in Figure 1, the two-mode network is projected onto a one-mode network, namely “skill network,” in which the nodes are skills and the edges connecting each pair of skills are weighted by the correlation between the two skills’ importance across all occupations. Thus, in the skill network, skills are connected to each other with a weight of how much they are required together.
Figure 1. Construction of a skill network.
By nature of job-skill representations in the O*NET database, every single skill is connected to each other with varying weights. Therefore, we construct a minimum spanning tree (MST) of the skill network, in which only significant edges remain connected to uncover its underlying structure.13,25 Given that occupations usually involve a task requiring a set of skills,26 to cluster the related skills, we employ an unsupervised clustering algorithm—the Louvain method12—which identifies 15 skill clusters in the skill network (hereafter referred to as “task types”). Task types are named based on their component skills (see the online appendix at https://dl.acm.org/doi/10.1145/3488376 for the detailed descriptions of materials and methods). Figure 2 illustrates the MST of the skill network, where the nodes are colored by task types and its size is proportional to each skill’s average importance across occupations.
Figure 2. Skill network and task types.
Task-level degree of automation. The O*NET database provides an aggregate measure of an occupation’s degree of automation, which could reflect not only the occupation’s task content, but also the overall impact of technological advances. Thus, our goal is to break down the occupation-level degree of automation into more granular skill- and task-level measures. If a skill is required by an occupation in proportion to the extent to which the occupation is automated, the skill is assumed to be highly automated. Relying on this assumption, we measure each skill’s degree of automation as the correlation between the importance of the focal skill to an occupation and the occupation’s degree of automation. Without loss of generalizability, we normalize the measure of automation between 0 and 1 by adding 1 and dividing by 2, for ease of interpretation.
For the task-level degree of automation, we look at the average of the measures of skill nodes belonging to a task type (a cluster of related skills). A higher (lower) task-level degree of automation indicates that the task tends to be performed to a greater extent by more (less) automated occupations (see the online appendix for validation of our measure of task-level degree of automation).
It is noteworthy that our correlation-based measures of degree of automation do not point to absolute criteria of high risk of automation, though they can provide useful guidance about relative automation levels of different occupations and tasks over time.
The upper and lower graphs of Figure 3 present the task-level degree of automation in 2020 and its changes from 2008 to 2020, respectively. Figure 3 demonstrates that the degree of automation of each task has been largely altered between 2008 and 2020. While routine problem solving has remained the most automated task throughout the past decade, machine control and hazardous tasks have experienced a large increase in the use of automation over the same period. Interestingly, task types that were relatively less automated in 2008—such as dynamic/general physical tasks, vehicle and equipment operations, conflict resolution, management and supervision, and interpersonal tasks—have become more automated between 2008 and 2020, although their automation levels are still not high as of 2020. In contrast, systems analysis and creative and critical thinking have seen a decrease in automation levels, making them least susceptible to automation.
Figure 3. Task-level degree of automation.
Labor demand for tasks. Automation technology can complement the jobs in which some tasks are performed but can substitute for others. The real effects of automation manifest in the labor markets, which are influenced by several factors, such as complementarity to technology, labor supply availability, and changing labor demand due to increased worker efficiency.8 Acemoglu and Restrepo2 show that the effect of automation on labor demand is determined by the balance between automation technology’s productivity and displacement effects. Therefore, for a comprehensive understanding of the impacts of automation technology on human tasks, this study also takes into consideration labor demand for occupations in which a task is performed.
To this end, we proxy the change in labor demand with change in employment share, as consistent with prior work.9 Specifically, labor demand for each task is measured by weighting occupational employment by the proportion of task content in each occupation. Data on occupational employment is obtained from the U.S. Bureau of Labor Statistics (BLS). However, the nationwide changes in occupational employment could be confounded by the overall economy’s sectoral/industrial changes during the past decade. To minimize the industry-composition effects, we compute within-industry change in employment share for each task from 2008 to 2020 at a three-digit NAICS level and then average the with-in-industry changes in employment share for each task.
A Taxonomy of the Race between Human Tasks and Automation
Taking the task-level degree of automation and the labor demand together into account, we plot the 15 task types by two dimensions in Figure 4: (i) technological change on the x-axis, measured as the changes in degree of automation from 2008 to 2020, and (ii) labor demand for occupations in which a task is performed on the y-axis, measured as average within-industry changes in employment share for each task during the same period. We consider the increase (decrease) in degree of automation on the x-axis as technological advance (stagnation), and the increase (decrease) in labor demand on the y-axis as labor complementarity (substitutability).
Figure 4. Task automation taxonomy.
As depicted in Figure 4, we classify the revealed task types into five distinct categories based on how they either complete or cooperate with the machine. The first category, which we term race with the machine, comprises relationship-oriented tasks—Interpersonal tasks, Group tasks, Management and Supervision, and Conflict Resolution—which have been in demand in the labor market during the past decade despite the increase in their degree of automation. The second category, which we call race ahead of the machine, comprises Communication, Systems Analysis, and Creative and Critical Thinking—several tasks associated with lower automation levels but higher employment numbers between 2008 to 2020. A third category, dubbed changing the course of the race, includes Routine Problem Solving and Information Processing, tasks that have already been highly automated in both 2008 and 2020 but have experienced decreased employment and automation levels from 2008 to 2020. General and dynamic physical tasks, which we term race against the machine, have experienced a large increase in automation levels from 2008 to 2020, while employment for them has decreased. Finally, despite an increase in automation levels, the employment share for handling equipment/machinery tasks—Equipment Maintenance, Machine Control, Hazardous Tasks, and Vehicle and Equipment Operation—has remained relatively stable. We categorize these as running a different race.
It is noteworthy that three task categories—race with the machine, race ahead of the machine, and changing the course of the race—are composed of cognitive tasks. The other two categories—race against the machine and running a different race—consist of manual tasks. This is consistent with prior work that classifies task types largely into cognitive and manual categories.4 Routine problem solving and information processing are the tasks that have been suggested to be most automated and computerized in the past two decades,7 whereas physical tasks have been considered as less susceptible to automation so far. In this regard, the decreasing level of automation for the already highly automated tasks may imply that they are subject to task redefinition and rebundling in response to task automation—that is, changing the course of the race. Our data-driven task taxonomy sheds additional light on how cognitive and manual tasks have taken divergent paths in terms of automation level and labor demand in the 21st century.
Proposed Automation Indexes
Job automation index. The extent to which an occupation is subject to automating each task will be determined both by how automated the task is and how important the task is to the occupation. Thus, we constructed a task-level job automation index for representative occupations by multiplying the skill-level degree of automation by the percentage of each skill’s importance to the occupation, which is subsequently averaged at the task level. In counting the importance of a skill to an occupation, we consider the “effective use” of skills whose importance is higher than the mean across occupations, as is common in prior studies.4 The task-level decomposition of the degree of automation for each occupation provides a granular understanding of occupational susceptibility to automation, which has implications on the future of work. The proposed job automation index for every occupation is available at www.jobautomationindex.com.
City automation index. Cities and urban areas are typically the hubs of economic activity.24 Thus, we extend the job automation index to urban areas by taking regional occupational compositions into consideration following prior studies.19 Specifically, we construct a city automation index for U.S. metropolitan statistical areas (MSA) using a weighted average of the job automation index, where the weight is an occupation’s employment share in a city. Occupational employment at the MSA level is obtained from the U.S. BLS. The city automation index illustrates that U.S. cities are taking divergent paths in the race between human labor and automation technology, which can be attributed to changes in a city’s industrial and occupational mix. An interactive map based on the proposed city automation index is available at the website.
Relationships between automation indexes and occupational/regional characteristics. To demonstrate the informative value of the proposed automation indexes, we explore the association of task-level automation indexes of occupations and U.S. cities (as of 2020) with occupational and regional characteristics, respectively. Table S1 in the online appendix presents descriptions and summary statistics for the variables used in the correlation analysis. The results for the five task automation categories are reported in Table 1 (see Table S2 in the appendix for the results for 15 individual task types).
Table 1. Correlations between automation indexes and occupational and regional characteristics.
First, we find that occupations that perform cognitive tasks (race with the machine, race ahead of the machine, and changing the course of the race) are associated with higher annual wages, respectively. In contrast, racing-against-the-machine and running-a-different-race tasks are negatively associated with occupational wage levels.
Second, we correlate the job automation index with the suitability for machine learning (SML) across occupations, given that machine learning plays a central role in recent technical innovations. Brynjolfsson et al.16 suggest that “the types of tasks affected by machine learning will be quite different from those affected in past waves of automation.” We use occupation-level SML data provided by Brynjolfsson et al.,16 who measured it based on the rubric that evaluates what the current generation of machine-learning systems can and cannot potentially do in the near future.15 We find that occupations that perform cognitive tasks (race with the machine, race ahead of the machine, and changing the course of the race)—which have been considered complementary to automation technology in the last decade—appear to be more suitable for machine-learning applications.
Technology can automate and substitute for some human tasks, but it can also augment human intelligence and complement specific types of tasks in ways that boost demand for occupations in which those tasks are performed.
The examples of machine learning-powered applications mentioned in this article’s introduction, including IBM’s Watson, DeepMind’s AlphaGo, and self-driving cars, illustrate how cognitive tasks can be computerized by machine learning. Personal assistants such as Apple’s Siri offer another case in point where ML has been applied for communication tasks classified as race ahead of the machine. On the other hand, machine learning is less likely to be applied to tasks that have been sub-stitutable by automation in the last decade—that is, race against the machine and running a different race. These results highlight that the next wave of automation fueled by ML and its AI applications may impact different parts of the labor force from those of the last decade. Given that the SML measure represents the ex ante potential of machine learning,16 note that this might be different from actual implementation of ML in practice.
Finally, the job automation index is correlated with the suitability of telework and “offshorability,” proposed by Dingel and Neiman17 and Acemoglu and Autor1, respectively. The results demonstrate that occupations performing tasks that are least susceptible to automation (race ahead of the machine) appear to be best suited for telework and offshoring, whereas tasks that have been more automated over the past decade (race against the machine and running a different race) are less suitable for telework and offshoring. Given that workers who can work remotely are significantly less likely to have their labor market outcomes affected by COVID-19,10 the findings imply that automation could also play a role in job security during such shocks as pandemics, an implication that proposes a potentially fruitful avenue of future research.
Given that cities are hubs for innovation,11 we further investigate how a city automation index correlates to total employment (as a proxy of city size) and regional innovation capacity across U.S. metropolitan statistical areas. We proxy regional innovation capacity with three categories of innovation indexes developed by the Indiana Business Research Center:23 human capital index for a capacity of knowledge creation, business dynamics index for a capacity of creative destruction, and business profile index for local business conditions and resources.
First, we find larger cities tend to have more jobs with tasks that have experienced a decrease in automation levels, whereas smaller cities accommodate a variety of jobs in terms of susceptibility to automation. This is consistent with prior findings that smaller cities are more vulnerable to automation,19,27 and our analysis also portrays a more nuanced picture of urbanization and automation. Specifically, though the overall automation level for major tasks of popular occupations in large cities has decreased, the labor demand for some tasks—that is, the race ahead of the machine category—has also increased. However, the labor demand for others—that is, the changing the course of the race category—has decreased. Thus, large cities may need to reinvent and reorient human labor and jobs in a way that outperforms machines. In contrast, small cities may need different automation adaptation strategies by fostering more jobs and industries that complement automation and work together with machinery and equipment—for example, race with the machine.
Second, we reveal that a city’s capacity for innovation (particularly, human capital and business dynamics) is significantly associated with task content and degree of automation of occupations in the city. The result implies that human capital, knowledge creation, and creative destruction in a city may play a role in building regional structures of industries and occupation mixes that are complementary and resilient to automation technology.
Some tasks, such as pattern recognition and information processing, are being rapidly computerized.
Taken together, the correlation analysis in Table 1 illustrates how task-level measures of automation can provide greater insight into the granular implications of automation at the occupation and city levels. Given that it is beyond the scope of this article, we leave the identification of causal relationships between degree of automation and occupational and regional characteristics to future research.
Discussion and Conclusion
To explore the race between human labor and machines in the 21st century, we proposed a data-driven approach to reveal endogenous task types performed by representative occupations and quantitatively measure their degree of automation. Our findings demonstrated that many, but not all, tasks have experienced an increase in the degree of automation between 2008 and 2020. This study highlights that a priori assumptions on the impact of automation may not hold over time, calling for dynamic and data-driven approaches that reflect recent changes in the task content of occupations and technological capabilities to automate different types of tasks. A major contribution of this study lies in its introduction of measurements for the task-level degree of automation, which allows practitioners and researchers to measure and track them in a consistent manner over time. As we use publicly available datasets compiled or sponsored by federal agencies, this work can provide a solid foundation for future empirical work and practice if the source datasets continue to be updated.
Our work calls for a dynamic view of technological advances, changes in task content, and labor demand. Our findings suggest that an increase in automation levels (through technological advances) does not always lead to a decrease in labor demand for the task. As new technologies, such as machine learning, require redesigning of the task content of jobs,16,26 some tasks that are subject to automation could be unbundled and rebundled within occupations. Indeed, we find that some tasks that were already automated to a great extent have also experienced a decrease in automation level, implying that task content of occupations may be redefined and repositioned in a way that favors human labor over machines. Moreover, technological changes can create new tasks for production in which labor has a comparative advantage over machines.2 The implications of automation vary by task type, which means the task content of occupations, the way in which tasks are redesigned and repositioned, and the way new tasks are created should be at the center of debates on the future of work, rather than full automation and displacement of occupations as a whole.
In this regard, it is imperative to track the evolution of an occupation’s task content and its automation level, based on up-to-date data. Our data-driven approach has an advantage in reconfiguring the endogenous task types on an ongoing basis and updating their degree of automation to date—see Figure 2, and Figure S7 in the Online appendix, for the skill networks constructed using the 2020 and 2008 datasets, respectively.
Policymakers and practitioners can use our data-driven approach as a tool to evaluate and track the impact of task automation and thereby cope with the challenges of labor automation caused by technological progress. Prior research emphasizes the importance of education in helping workers adapt to such technological advances. For instance, Autor8 argues that “the issue is not that middle-class workers are doomed by automation and technology, but instead that human capital investment must be at the heart of any long-term strategy for producing skills that are complemented by rather than substituted for by technological change.” For worker retraining programs, a granular understanding of task-level automation can speak to which jobs need to be retrained, and which tasks or skills they should learn in order to adapt existing skills/tasks to evolving demands in the era of automation.
We view this study as the first step to forming a comprehensive picture of the race between human labor and machines and the future of work. Our explanation for the correlation between automation and occupational and regional characteristics should be complemented by more theoretical and empirical works to reveal something of what currently seems to be a black box. We hope that our study will spur discussion and communications on the impacts of automation and the future of work in the era of rapid technological changes.
Join the Discussion (0)
Become a Member or Sign In to Post a Comment