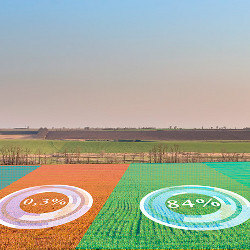
According to the World Health Organization (WHO), the world is going hungry. WHO data shows that in 2018, the most recent year for which data is available, 820 million people lacked enough food to eat, an increase of nine million people over the year before.
Hunger kills plenty of people worldwide. It also impacts those who survive, causing serious childhood development issues like stunting, where children are too short for their age, and wasting, where they’re too thin for their age.
The explosion in our planet’s population is a major factor in there not being enough food to go around. Since 1950, the global population has grown from 2.6 billion people to almost eight billion people in 2021, according to the United Nations. That growth is continuing apace, with the world’s population estimated to increase by another two billion people by 2050.
To solve problems such as explosive population growth, new solutions are required.
Artificial intelligence (AI) is one of those solutions. AI systems—including machine learning and deep learning systems—are increasingly using large datasets and robust computer science techniques to improve crop yields, boost farm productivity, and prevent disease from destroying crops.
The result is what Ananth Kalyanaraman calls a “revolution” in agriculture. Kalyanaraman is the director of the USDA-NIFA Institute for Agricultural AI for Transforming Workforce and Decision Support at Washington State University, a research institute dedicated to developing AI to solve tough agricultural problems. Known familiarly as the AgAID Institute, it is one of 11 AI-focused research institutes launched by the National Science Foundation (NSF) to figure out how AI, machine learning, deep learning, and other next-generation technologies can solve hunger issues worldwide.
The AgAID Institute is funded by the U.S. Department of Agriculture’s National Institute of Food and Agriculture. Federal investment in outfits like AgAID signals the war on hunger will not be won by people alone; it will be won through a creative combination of human and artificial intelligence.
Agrobots, Crop Surveillance, and Digital Twins
A key application of AI in agriculture is the use of intelligent and autonomous robots, says George Papakostas, a professor in the Department of Computer and Informatics Engineering of the Eastern Macedonia and Thrace Institute of Technology, Greece, and an acknowledged expert on the use of computer vision in agriculture. “Agrobots” use computer vision to execute agricultural tasks like planting and harvesting without human supervision. They increase crop yields by working both faster and longer than humans.
“Agrobots are able to make decisions based on the knowledge they have acquired through the training process with big data and to perform tasks seamlessly with precision and speed,” says Papakostas.
These robots are not just better at such agricultural jobs than humans; they also do jobs humans don’t want. “The lack of experienced and specialized staff [in the agricultural sector] due to urbanization” is a huge problem, says Papakostas, who adds that autonomous robots directed by AI can address this problem by providing a workforce able to plant, weed, and harvest 24 hours a day, seven days a week.
Automation is one area where AI makes agriculture better able to feed the world’s hungry people. Prediction is another.
AI is used to detect and predict diseases in crops. AI techniques process the data received from Internet of Things (IoT) sensors that monitor climatic, environmental, and visual information from the surface, soil, and microclimate of crops. This data is then used to train deep learning models that can forecast the likelihood of disease developing in certain types of crops, says Papakostas.
The result? Intelligent crop disease surveillance systems that make it much easier to predict yields and spot diseases before they wipe out entire crops in which farmers have invested a fortune, says George Kantor, a researcher at the Robotics Institute of Carnegie Mellon University.
“Early disease detection capabilities can feed into precision spraying equipment for targeted application of the necessary treatment,” says Kantor. “This can dramatically reduce chemical inputs used by farmers, which both saves on production costs and reduces environmental impact.”
AI also makes it possible to go one step further in disease prevention: we no longer need to grow certain crops to predict what could affect them, says James Schnable, an associate professor and Dr. Charles O. Gardner Professor of Agronomy in the Department of Agronomy & Horticulture at the University of Nebraska-Lincoln. “I’m most excited about the development and deployment of digital twins: virtual counterparts of real-world plants that allow us to experiment and predict how plants will respond in different environments,” he says.
Intelligence crop disease surveillance systems can make it easier to predict yields and spot diseases before they wipe out crops in which farmers have invested fortunes.
Schnable’s work on digital twins also is funded by the NSF. These virtual creations allow farmers to game out the outcomes of different crop management decisions, such as when to apply fertilizer or how much irrigation water to use. Digital twins help farmers both increase current crop efficiency and anticipate future growing conditions before they become a problem.
This is important because today’s plant breeders are producing tomorrow’s crops.
“A plant breeder working today is developing new varieties for farmers to grow in 2030,” says Schnable. “Yet, we don’t have access to the environments of Nebraska in 2030, or Rwanda in 2030. In order to overcome this challenge, we need to be able to predict how crop varieties will perform in environments that won’t exist for another 10 to 15 years. That’s where artificial intelligence and machine learning come in.”
Despite general crop yield gains, says Schnable, field tests are still needed to determine which crops will grow best using which methods in certain areas.
“Field tests conducted in Missouri won’t predict which crop varieties have the potential to be the most resilient or produce the most yield in Nebraska,” he says. “Field tests conducted in Kenya won’t predict which crop varieties have the potential to be the most resilient or produce the most yield in Rwanda.”
AI and machine learning models are critical to simulating different conditions at scale.
One application of this principle may be seen in work being done by Timothy Smith and Zhenong Jin at the University of Minnesota. In their most recent paper, the two researchers built a series of machine learning prediction models regarding the Corn Belt of the U.S., the premier corn-producing area of the country. The predictions simulated sustainability conditions across farms, allowing farmers to identify problem areas that reduce crop health, output, and yields.
Changing Climate, Changing Technologies
AI is critical to increasing agricultural yields to keep up with population growth. It also is key to solving core challenges related to climate change that could threaten the world’s food supply.
“Extreme weather events such as frost, extreme heat, or inversion can cause severe crop damage and loss in little time,” says Kalyanaraman. In the U.S., up to 50% of a state’s annual agricultural production losses may be attributed to a single weather event. AI can help by both collecting data from farms that experience such weather events through advanced sensors, and offering insights into site-specific decisions and risk mitigation.
That’s because part of the problem with climate uncertainty is that farmers just don’t generate sufficient data from their own personal experience to consider how best to respond to such extreme weather events. “A really successful farmer may get 40 total growing seasons in their career,” says Schnable, “but having only 40 chances also means that making the wrong call hurts a lot.”
As climate change increasingly disrupts traditional weather cycles, AI-powered predictive modeling gives farmers a source of information beyond their own individual experiences, which can potentially give them a leg up by modeling conditions they have not yet experienced.
“Future productivity improvements will be dependent on improved efficiencies in energy, water, and nutrient management under a changing climate.”
“Agriculture has achieved record levels of production in recent decades, but future productivity improvements will be dependent on improved efficiencies in energy, water, and nutrient management under a changing climate,” says Smith.
One creative solution to a changing climate is using AI to grow crops better indoors.
Vertical farming is a way to grow crops inside and vertically, rather than outdoors across wide tracts of land. AeroFarms, a leader in the vertical farming space, uses AI and machine learning to regulate the light each of its vertical farm crops receives. As a result of machine-augmented lighting and crop regulation, AeroFarms claims 390 times greater productivity per square foot when compared with traditional farming, as well as 95% less water usage and zero pesticide usage to grow its crops.
“We use AI and machine learning in concert to watch our crops for signs of problems of all types, including diseases, pests, and equipment failures,” says AeroFarms chief technology officer Roger Buelow, who adds that the company also images plants on a daily basis.
AI isn’t the only technology altering the playing field when it comes to sustainable farming. Super-high-resolution satellite imagery is becoming much more ubiquitous as the cost of launching satellites into orbit continues to decline.
“It is well within the realm of possibility that 10 or 20 years from now, every field in the world gets imaged from space at 30 centimeters per pixel resolution or less, and the results are used to predict which specific parts of each field will need irrigation or fertilizer,” says Schnable.
In all likelihood, says Kalyanaraman, the future of agriculture looks a lot like a partnership between humans and machines, and a worldwide one at that. Microsoft is deploying machine learning algorithms and smart sensors to increase crop yields in the developing world through its Azure FarmBeats technology. IBM offers global AI consulting to help agricultural producers use machine learning to boost profitability and yields.
Yet, “Due to the complexities of the labor tasks involved, it is unlikely that full automation is possible in these labor-intensive crop sectors,” says Kalyanaraman.
“Instead, collaborative and inclusive human-AI workflows that are able to augment and amplify the combined skills have the potential to lead to a whole that is greater than the sum of its components. Such a partnership can also help close critical skill gaps in the workforce.”
Ali, M.
Artificial intelligence could stop millions from going hungry by 2030, EuroNews, Jun. 2021, https://bit.ly/3CUuHy9
University of Minnesota Develops Tool to Help Farmers Make Crop Input Decisions, University of Minnesota News, Jul. 15, 2021, https://bit.ly/2VTxN57
World hunger is still not going down after three years and obesity is still growing, World Health Organization, Jul. 2019, https://bit.ly/3CWG5d9
The AgAID Institute: Agricultural AI for Transforming Workforce and Decision Support, https://agaid.wsu.edu
FarmBeats: AI, Edge, & IoT for Agriculture, May 2015, https://www.microsoft.com/en-us/research/project/farmbeats-iot-agriculture
IBM Agriculture, https://www.ibm.com/products/agriculture
Join the Discussion (0)
Become a Member or Sign In to Post a Comment