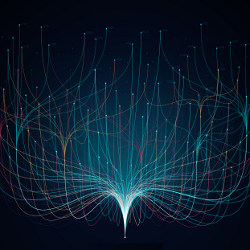
Large-scale Machine Learning (ML) algorithms are often iterative, using repeated read-only data access and I/O-bound matrix-vector multiplications. Hence, it is crucial for performance to fit the data into single-node or distributed main memory to enable fast matrix-vector operations. General-purpose compression struggles to achieve both good compression ratios and fast decompression for block-wise uncompressed operations. Therefore, we introduce Compressed Linear Algebra (CLA) for lossless matrix compression. CLA encodes matrices with lightweight, value-based compression techniques and executes linear algebra operations directly on the compressed representations. We contribute effective column compression schemes, cache-conscious operations, and an efficient sampling-based compression algorithm. Our experiments show good compression ratios and operations performance close to the uncompressed case, which enables fitting larger datasets into available memory. We thereby obtain significant end-to-end performance improvements.
1. Introduction
Large-scale ML leverages large data collections to find interesting patterns or build robust predictive models.7 Applications range from traditional regression, classification, and clustering to user recommendations and deep learning for unstructured data. The labeled data required to train these ML models is now abundant, thanks to feedback loops in data products and weak supervision techniques. Many ML systems exploit data-parallel frameworks such as Spark20 or Flink2 for parallel model training and scoring on commodity hardware. It remains challenging, however, to train ML models on massive labeled data sets in a cost-effective manner. We provide compression-based methods for accelerating the linear algebra operations that are central to training. The key ideas are to perform these operations directly on the compressed data, and to automatically determine the best lossless compression scheme, as required by declarative ML systems.
Declarative ML. State-of-the-art, large-scale ML systems provide high-level languages to express ML algorithms by means of linear algebra such as matrix multiplications, aggregations, element-wise and statistical operations. Examples at different abstraction levels are SystemML,4 Mahout Samsara,17 Spark MLlib,19 and TensorFlow.1 The high-level specification allows data scientists to create or customize ML algorithms without worrying about data and cluster characteristics, data representations (e.g., sparse or dense formats), and execution-plan generation.
Data-intensive ML algorithms. Many ML algorithms are iterative, with repeated read-only data access. These algorithms often rely on matrix-vector multiplications, which require one complete scan of the matrix with only two floating point operations per matrix element. This low operational intensity renders matrix-vector multiplication, even in-memory, I/O bound.18 Despite the adoption of flash-and NVM-based SSDs, disk bandwidth is usually 10x-100x slower than memory bandwidth, which is in turn 10x-40x slower than peak floating point performance. Hence, it is crucial for performance to fit the matrix into available memory without sacrificing operations performance. This challenge applies to single-node in-memory computations, data-parallel frameworks with distributed caching like Spark,20 and accelerators like GPUs with limited device memory. Even in the face of emerging memory and link technologies, the challenge persists due to increasing data sizes, different access costs in the memory hierarchy, and monetary costs.
Lossy versus lossless compression. Recently, lossy compression has received a lot of attention in ML. Many algorithms can tolerate a loss in accuracy because these algorithms are approximate in nature, and because compression introduces noise that can even improve the generalization of the model. Common techniques are (1) low- and ultra-low-precision storage and operations, (2) sparsification (which reduces the number of non-zero values), and (3) quantization (which reduces the value domain). However, these techniques require careful, manual application because they affect the accuracy in a data-and algorithm-specific manner. In contrast, declarative ML aims at physical data independence. Accordingly, we focus on lossless compression because it guarantees exact results and thus, it allows for automatic compression to fit large datasets in memory when needed.
Baseline solutions. The use of general-purpose compression techniques with block-wise decompression per operation is a common baseline solution. However, heavyweight techniques like Gzip are not applicable because decompression is too slow, while lightweight methods like Snappy or LZ4 achieve only modest compression ratios. Existing compressed matrix formats with good performance like CSR-VI15 similarly show only moderate compression ratios. In contrast, our approach builds upon research on lightweight database compression, such as compressed bitmaps and dictionary coding, as well as sparse matrix representations.
Contributions. We introduce value-based Compressed Linear Algebra (CLA),9,10 in which lightweight compression techniques are applied to matrices and then linear algebra operations are executed directly on the compressed representations. Figure 1 shows the goals of this approach: we want to widen the sweet spot for compression by achieving both (1) performance close to uncompressed in-memory operations, and (2) good compression ratios to fit larger datasets into memory. Our contributions include:
Figure 1. Goals of compressed linear algebra.
- Adapted column-oriented compression schemes for numeric matrices, and cache-conscious linear algebra operations over these compressed matrices (Section 3).
- A sampling-based algorithm for selecting a good compression plan, including techniques for compressed-size estimation and column grouping (Section 4).
Our CLA framework is available open source in Apache SystemML, where CLA is enabled by default for matrices that are larger than aggregate cluster memory.
2. Background and Motivation
After giving an overview of SystemML as a representative ML system, we discuss common workload characteristics that directly motivate the design of our CLA framework.
SystemML compiler and runtime. In SystemML,4 ML algorithms are expressed in a high-level language with R-like syntax for linear algebra and statistical operations. These scripts are automatically compiled into hybrid runtime plans that combine single-node, in-memory operations and distributed operations on MapReduce or Spark. During this compilation step, the system also applies optimizations such as common subexpression elimination, optimization of matrix-multiplication chains, algebraic simplifications, physical operator selection, and rewrites for dataflow properties like caching and partitioning. Matrices are represented in a binary block matrix format with fixed-size blocks, where individual blocks can be in dense, sparse, or ultra-sparse formats. For single-node operations, the entire matrix is represented as a block, which ensures consistency without unnecessary overheads. CLA can be seamlessly integrated by adding a new derived block representation and operations.
Common operation characteristics. Two important classes of ML algorithms are (1) iterative algorithms with matrix-vector multiplications (or matrix-matrix with a small second matrix), and (2) closed-form algorithms with transpose-self matrix multiplication. For both classes, few matrix operations dominate the overall algorithm runtime, apart from the costs for the initial read from distributed file system or object storage. This is especially true with hybrid runtime plans, where operations over small data are executed in the driver and thus, incur no latency for distributed computation. Examples for class (1) are linear regression via a conjugate gradient method (LinregCG), L2-regularized support vector machines (L2SVM), multinomial logistic regression (MLogreg), Generalized Linear Models (GLM), and Kmeans, while examples for class (2) are linear regression via a direct solve method (LinregDS) and Principal Component Analysis (PCA). Besides matrix-vector multiplication, we have vector-matrix multiplication, which is often caused by the rewrite X⊤v → (v⊤X)⊤ to avoid transposing X because computing X⊤ is expensive, whereas computing v⊤ involves only a metadata update. Many systems also implement physical operators for matrix-vector chains with optional element-wise weighting X⊤(w⊙(Xv) ), and transpose-self matrix multiplication (tsmm
) X⊤X.4,17 Most of these operations are I/O-bound, except for tsmm
with m ≫ 1 features because its compute workload grows as O(m2). Other common operations over X are cbind
, unary aggregates like colSums
, and matrix-scalar operations.
Common data characteristics. The inputs to these algorithm classes often exhibit common data characteristics:
- Tall and skinny matrices: Matrices usually have significantly more rows (observations) than columns (features), especially in enterprise ML, where data often originates from data warehouses (see Table 1).
- Non-uniform sparsity: Sparse datasets usually have many features, often created via pre-processing such as dummy coding. Sparsity, however, is rarely uniform, but varies among features. For example, Figure 2 shows the skew of the Covtype and Mnist8m datasets.
- Low column cardinalities: Many datasets exhibit features with few distinct values, for example, encoded categorical, binned or dummy-coded features. For example, Figure 3 shows the ratio of column cardinality to the number of rows of the Higgs and Census datasets.
- Column correlations: Correlation among features is also very common and typically originates from natural data correlation, the use of composite features, or again pre-processing techniques like dummy coding. For example, exploiting column correlations improved the compression ratio for Census from 12.8x to 35.7x.
Table 1. Compression ratios of real datasets.
These data characteristics directly motivate the use of column-oriented compression schemes as well as heterogeneous encoding schemes and column co-coding.
3. Compression Schemes
We now describe the overall CLA compression framework, encoding formats for compressed column groups, and cache-conscious operations over compressed matrices.
3.1. Matrix compression framework
CLA compresses matrices column-wise to exploit two key characteristics: few distinct values per column and high cross-column correlations. Taking advantage of few distinct values, we encode a column as a dictionary of distinct values, and a list of offsets per value or value references. Offsets represent row indexes where a given value appears, while references encode values by their positions in the dictionary.
Column co-coding. We further exploit column correlation by partitioning columns into groups such that columns within each group are highly correlated. Each column group is then encoded as a single unit. Conceptually, each row of a column group comprising m columns is an m-tuple t of floating-point values that represent reals or integers.
Column encoding formats. The lists of offsets and references are then stored in a compressed representation. Inspired by database compression techniques and sparse matrix formats, we adapt four effective encoding formats:
- Offset-List Encoding (OLE) encodes the offset lists per value tuple as an ordered list of row indexes.
- Run-Length Encoding (RLE) encodes the offset lists as sequence of runs of begin row index and run length.
- Dense Dictionary Coding (DDC) stores tuple references to the dictionary including zeros.
- Uncompressed Columns (UC) is a fallback for incompressible columns, stored as a sparse or dense block.
Encoding may be heterogeneous, with different formats for different column groups. The decisions on co-coding and encoding formats are strongly data-dependent and thus, require automatic compression planning (Section 4).
Example compressed matrix. Figure 4 shows an example compressed matrix block in its logical representation. The 10 × 5 input matrix is encoded as four column groups, where we use 1-based indexes. Columns 2, 4, and 5 are represented as single-column groups and encoded via RLE, DDC, and UC, respectively. For Column 2 in RLE, we have two distinct non-zero values and hence two associated offset lists encoded as runs. Column 4 in DDC has three distinct values (including zero) and encodes the data as tuple references, whereas Column 5 is a UC group in dense format. Finally, there is a co-coded OLE column group for the correlated Columns 1 and 3, which encodes offset lists for all three distinct non-zero value-pairs as lists of row indexes.
Figure 4. Example compressed matrix block.
Notation. For the ith column group, denote by Ti = {ti1, ti2, …, tidi} the set of di distinct tuples, by
Join the Discussion (0)
Become a Member or Sign In to Post a Comment