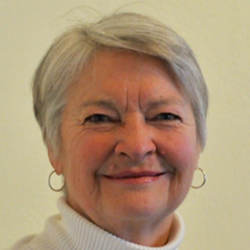
The high-tech business frets about how to encourage trust in artificial intelligence [Rossi2019, RAND2021]. This campaign worries me [Hill2019, Hill2021], due to the prevalence of AI-enhanced algorithmic decision systems in employment, financial, and criminal-justice decisions, systems that sometimes deliver palpably faulty results. Those are instances of too much trust, along with "self-driving" cars where, yes, I know they're not self-driving; the clumsy irony emphasizes the lending of too much trust to the vehicle.
On the topic of AI systems that make life-changing decisions, say, loan applications, I detect many versions of this argument:
AI systems show bias.
People show bias.
——
Therefore we should use AI systems.
A reader in computer science hardly needs to be told that the conclusion doesn't follow. Certainly we grant both statements shown as premises, but that is not enough. More solid reasoning has to rely on assumptions such as:
- AI systems draw fewer biased conclusions than people do.
- AI systems can be freed of bias, if we just work on them for a while.
Neither is incontrovertible. Neither is well substantiated. Both are aspirational. Either may be true. Neither is known to hold at the moment. While the two original premises can be stipulated, the conclusion given only follows on appeal to faith in the free-floating beneficence of technology.
My own assessment is that these AI systems simply cannot do the decision-making job, and should not take over from the people that are currently doing those jobs. If only, I think to myself, the public, lawmakers, and tech experts could see that far-superior reasoning. And I can show it! I can easily distill my version of the argument, as follows.
AI systems show bias.
People show bias.
——
Therefore we should use people.
Well. Not quite the killer arg I anticipated… Same structure, same flaw. Yet the author remains convinced. By what? What exactly is presumed? The answer that lurks in there is the notion that we can tell when our practices and conventions go off the rails. We can tell, with difficulty. We come to know when things are not working out. We come to know, painfully. With fits and starts, but without overt programming prompts, we recognize and rectify obstacles to well-being.
All those qualifications weaken the scaffolding of the additional premises on which to build a sound deduction. We must acknowledge long histories of bias, acted upon with confidence and approval. In the face of that, our reasoning can only appeal to this: eventual corrective meta-observation. In this country, we endured a protracted struggle for civil rights in order for that sort of unfairness to come to the full attention of the public, let alone come to resolution (ongoing). Yet we humans are the observers that finally figured it out in the past and continue to do so in the present.
Here are the additional premises, in short:
- AI systems don't recognize their own bias.
- People sometimes recognize their own bias.
By "people," I mean individuals working in clerical jobs reviewing paperwork, society noting the collective results of decisions, institutions investigating their own practices, AND the intellects that apply critical thinking to those observations. We may not notice soon enough that some people are excluded unfairly from some rights and privileges, but we do notice.
References
[Hill2019] Hill, R. The Artificialistic Fallacy. Blog@CACM, March 30, 2019.
[Hill2021] Hill, R. Misnomer and Malgorithm. Blog@CACM, March 27, 2021.
[RAND2021] RAND Europe, Salil Gunashekar, Project Associate Director. Exploring ways to regulate and build trust in AI. April 25, 2022.
[Rossi2019] Rossi, Francesca. Building Trust In Artificial Intelligence. Journal of International Affairs. School of International and Public Affairs at Columbia University. February 06, 2019.
Robin K. Hill is a lecturer in the Department of Computer Science and an affiliate of both the Department of Philosophy and Religious Studies and the Wyoming Institute for Humanities Research at the University of Wyoming. She has been a member of ACM since 1978.
Join the Discussion (0)
Become a Member or Sign In to Post a Comment