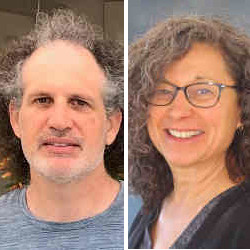
When the coronavirus broke out shortly before the beginning of the Spring 2020 semester, all of the higher education institutions in Israel and around the world had to transition overnight from traditional, frontal teaching to online teaching. After a period of learning and adaptation, both lecturers and students found their balance points, maximizing the advantages inherent in online learning, such as the possibility of recording lessons, working in groups using Zoom breakout rooms, and so on.
Towards the end of the semester, the pandemic began to wind down in Israel, and higher education institutions began preparing for their return to normal routine. Despite the decision to finish the semester online, the institutions intended to hold final exams in the traditional manner, including exam halls, forms, notebooks, and a hoard of supervisors. Unfortunately, with the beginning of the exam period, another outbreak of the coronavirus pandemic occurred, and the government re-imposed restrictions on gatherings. The question of the administration of exams by higher education institutions was discussed in various committees, and ultimately a decision was made to ban the institutions from holding end-of-semester exams on the various physical campuses.
These higher education institutions again faced a dramatic change that was forced upon them overnight; the solution that was adopted was to conduct home exams. To maintain academic integrity of the exams, the higher education institutions moved the existing, on-campus supervision mechanism to the students' homes, using Zoom cameras and other means. In addition to the concern about maintaining the exams' academic integrity, this mechanism proved ineffective in many cases, since it required considerable resources and was complicated to implement. In light of the challenges that holding home exams entails, it is clear that creative solutions to this problem must be found, both for the current semester exams and for the future.
One approach to the generation of new ideas is the provocation approach, which Edward de Bono described in his 1967 book The Use of Lateral Thinking. According to this approach, one way to think of creative, applicable ideas is first to think of radical ideas, even if they clearly cannot be implemented. Such radical thinking will encourage new directions of thought that differ from traditional directions and will enable the identification of applicable ideas that stem from those new directions of thought.
In this Blog, we wish to propose a radical solution to the student home exams problem. The proposed idea is based on data science, a new science that has emerged over recent years and offers models and tools for forecasting and predicting by studying big data. Many applications of data science have been developed in recent years. In science, industry, and a variety of other organizations, machine learning algorithms are being used today to identify diseases, to tailor pharmaceutical treatment plans for diseases, to detect credit card fraud, to create personalized learning environments for pupils, to predict the demand for goods and products, to predict crime, and so on. Autonomous driving, one of the more promising applications of machine learning and artificial intelligence today, is already undergoing field testing.
In light of the difficulties involved in conducting home exams and in light of the promising capabilities of data science in general and of machine learning algorithms in particular, we wish to propose the following radical idea: Instead of testing the students, let us predict their exam scores using a machine learning algorithm. To that end, we can train a learning machine using student data from previous semesters. We can provide the machine with complete data regarding the learning process, the learning products, the usage characteristics of e-learning systems, and graded exercises and homework. In addition, we can provide the algorithm with the students' exam scores from previous semesters, as well as with data on their achievements in other courses. Then, the machine could create a prediction model for the exam score based both on the student's learning process throughout the semester and on additional parameters. Thus, we could run this model on selected students in a certain semester and predict their exam scores without requiring them to even take the exam.
This idea is, as mentioned, radical and provocative and raises many questions: Do the data used to train the algorithm really exist? Are they reliable? Can a model be trained to predict success on an exam? How reliable would the model be? The idea also raises many ethical issues: Is it right to do so without studying for the exam? Is it not worth giving students the opportunity to study and improve their knowledge for the exam, even if they did not do that well during the semester? Will it be possible to influence the algorithm results in inappropriate ways?
These questions, and many others not mentioned here, are legitimate. Nevertheless, the objective of the proposal is to expose a new direction of thought that is intended to replace the direction of thought about replicating traditional exams. This direction of thought can raise additional ideas, some of which may be applicable already in the present or in the future. For example, a similar algorithm may possibly be used to detect cheating and impingement upon the academic integrity of the exams. The coming semester may be constructed in such a way as to enable the gathering of enough data to create an algorithmic grade. Students who would be interested in improving their algorithmic grade would be able to do so in a traditional, physical manner. If the number of students sitting for exams on campus decreases significantly, actual sitting for exams on campus would once again be possible.
In summary, this Blog presents an example of data science-based radical thinking about exams in higher education institutions whose objective is to encourage different ways of thinking about exams in general, and in particular about ending semester exams. As the coronavirus has transformed teaching in a way that will most probably also change the future of teaching, thought must be given to ways in which the evaluation of learning may be changed in corona times, and how such change may lead to a change also in future evaluation methods.
Koby Mike is a Ph.D. student at the Technion’s Department of Education in Science and Technology under the supervision of Orit Hazzan. His research focuses on data science education.
Orit Hazzan is a professor at the Technion’s Department of Education in Science and Technology. Her research focuses on computer science, software engineering and data science education. For additional details, see https://orithazzan.net.technion.ac.il/ .
Join the Discussion (0)
Become a Member or Sign In to Post a Comment