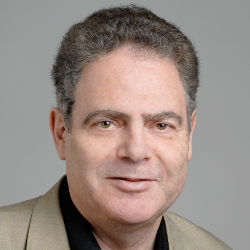
Since the release of the ChatGPT interactive AI assistant it has been surprising to see some of the snide, passive-aggressive reactions from some (not all) members of the software engineering community, in the style of “it’s just inference from bad data”. Let’s get real, folks, it is truly game-changing. The kind of thing that you witness once in a generation. (The last two times were object-oriented programming and the World-Wide Web.)
Basically, if you need a program element and can describe that need, the assistant will generate it for you. There is no particular restriction on the programming language that you choose, as long as its description and enough examples are available somewhere. The code will be pretty good. (More on the semantics of “pretty” below.) You can ask the assistant for a test suite and various other adornments.
Programmers no longer needed?
Trying this tool seriously is guaranteed to produce a “Wow” effect and for a software engineer or software engineering educator, as the immediately following step, a shock: “Do I still have a job?“. At first sight, you don’t. Especially if you are a programmer, there is not much that you can do and ChatGPT cannot.
In assessing this observation, it is important to separate the essential from the auxiliary. Any beta release of a new technology is bound to suffer from a few pimples. Instructive in this respect is a look at some of the early reviews of the iPhone (for example those on CNET and on PCMag), lamenting such horrible deficiencies as the lack of Bluetooth stereo. I could complain that the generated code will not compile out-of-the-box, since ChatGPT believes that Eiffel has a “do” keyword for loops (it’s loop) and enumerated types introduced by “type” (it doesn’t). These bugs do not matter; the tool will learn. What does matter is that if I ask, for example, for a Levenshtein edit distance program in Eiffel, I get something that is essentially right. Plus well-formatted, equipped at the start of every routine (per good Eiffel style rules) with a header comment explaining clearly and correctly the purpose of the routine, and producing the right results. Far beyond the Turing test. (To be more precise: as readers of this blog undoubtedly know, a tool passes the Turing test if a typical user would not be able to determine whether answers come from a human or a program. In this case, actually, you will need to add a delay to the responses of ChatGPT to have it pass the test, since no human could conceivably blurt out such impressive answers in a few seconds.)
What comes after the bedazzlement? The natural question is: “What can I do with this?”. The answer — for a programmer, for a manager — is not so clear. The problem is that ChatGPT, in spite of its cocky self-assurance (This is your result! It will work! No ifs and buts!) gives you, for a non-trivial problem, an answer that may work but may also almost work. I am no longer talking here about growing pains or bugs that will be fixed, but about essential limitations.
Exact, or almost exact?
Here is an example that illustrates the phenomenon vividly.
In discussion of use cases and other requirements techniques, I like to use the example of a function that starts with explicit values: 0 for 0, 1 for 1, 4 for 2, 9 for 3, 16 for 4, 25 for 5. At this point almost everyone (and ChatGPT) will say sure, you don’t need to go on, I get it: the square function. As a specification technique (and that was my point in an earlier article in this blog, already 10 years ago, A Fundamental Duality of Software Engineering), this approach is terrible: an example or any number of examples do not provide a specification; I had some fun, in preparing that article, running the values through a curve-fitting algorithm that provided several other reasonable matching functions, along with a few unreasonable ones.
This time I fed the above values to ChatGPT and for good measure added that the result for 6 is 35. Yes, 35, not a typo. Here is the start of the iteration.
Now, lo and behold, ChatGPT still infers the square root function!
Obligingly adding instructions of how to use the function and examples of results (including 36 for 6!).
It does not stop there. The tool is truly an assistant, to which (one has to resist writing “whom”) you can talk:
It will correct itself, but by resorting to the kind of case-by-case programming reminiscent (as my colleague Jean-Michel Bruel pointed out) of the code that an an undergraduate student will enthusiastically produce just after discovering TDD:
(In an earlier attempt, I did get an if-then-else with n2 by default and 35 for the special case, but I was not able to reproduce it.) Asking as the next question what value the function gives for 7 elicits a disappointing response, but things becomes amazing again, in fact more amazing than before, when I point out my dissatisfaction with the above style:
The inferred function is rather impressive. What human would come up with that function in less time than it takes to say “Turing test”? Of course it would also help (as respondents to this article have pointed out) if the function matched all the data points. The algorithms will improve, but the cocky self-assurance remains scary, reinforcing the need for specification and verification.
We can only say “nice try”. Should we also head for the employment office in search of mid-career retraining sessions for professions with a future?
Not so fast!
Let us come to the basic question of “What do we do with this?”, assuming for focus that “we” is an IT project manager who has been tasked with the development of some system. Even assuming “we” only use an AI-based assistant for individual modules (part of an overall architecture that we still devise using traditional means), are we going to stake our reputation on automatically generated software?
The program generation approach is based on the analysis of myriad existing code, not on logical deduction or any kind of formal methods. It will generate programs that, as the above example and many others that anyone can try demonstrate, are almost right. This property is not a temporary limitation (such as a tool’s imperfect understanding of the syntax of a programming language, cited above, which will inevitably correct itself); it is built into the very definition of modern AI approaches, based on inference from statistical models. The previous major success of these approaches, modern automatic translation tools relying on statistics rather than just structural linguistic, is a good illustration of this phenomenon: while deepl.com reached an unbelievable level of quality, it still makes a serious translation error once in a while (even if an increasingly rare while).
Being almost correct, though, is not very useful in software. We need correct answers. Of course hand-produced programs have bugs too, but these programs are developed at a human pace and the corresponging tests also get produced with matching techniques. We saw above that even with a precise specification we can still get an incorrect answer. The likelihood of this situation will decrease, but the possibility will remain.
Who is ready to make business processes contingent to such uncertainty?
What will happen?
Anyone who has looked into the history of software engineering (or witnessed some of it) knows that the phrase “automatic programming” has been around all along, to denote any approach that was a level of abstraction just a bit higher than the then current standard. One of the first applications of the term was to … Cobol, presented as a replacement for programming and a way to, yes, get rid of the need for programmers! Users would just describe their needs and the Cobol compiler would generate the programs for them. A large part of software engineering is, of course, devoted to understanding the semantics of this “just.“
This time, things are different. We do have a technology that can generate programs from very high-level descriptions in natural language. As in previous cases, however, the advance does not resolve the problem but pushes it further, or, more precisely, higher (in the sense of abstraction).
The AI-based-assistant revolution, of which ChatGPT is the first salvo, will change the landscape of programming. Much low-level coding — the kind, for example, that led to the initial push for outsourcing (whose software engineering implications I discussed in a 2005 IEEE Computer article) — can be handled automatically. But then? The need remains for requirements, specification and verification.
Not the end of programming, then, but a revival — which I take the risk of predicting — of these good old mainstays of software engineering. For the past few years, in the competition with remarkable new subjects such as (surprise) machine learning, these disciplines of requirements analysis, precise specification, and software verification (both dynamic tests and static analyses including proofs) have taken a second seat. The phenomenon that is bound to happen (as previewed by two recent and separate comic strips, here and here) is a renewed interest in these fundamental techniques, without which no “automatic programming” can succeed.
Final note
Just as much as software development, software education will fundamentally be affected by what is happening now. As a simple example, I just ran an exam for a software course, including a programming exercise, for which (as a result of a general policy developed over years of reflecting about educational issues) we allowed the students to browse the Web. This time the exam happened a few days after the release of ChatGPT; we did not change the policy, and do not know whether any students were aware of the possibility. One thing is for sure, though: there is no way we can ignore such tools in devising future policies.
The example of programming exams is just one case of the general issue of what effect the emergence of AI-based assistants will have on the teaching of computer science and software engineering. In my latest article on this blog, just two days ago, I mentioned the FISEE workshop on teaching to be held in the South of France on Jan. 23-25. The organizers (A. Capozzucca, J-M Bruel, S. Ebersold and I) have defined as one of the goals of the workshop to produce a white paper on exactly this topic. If you are interested in participating, go through the workshop page or contact me directly. We look forward to discussing in depth the impact of AI assistants on teaching, and producing a constructive contribution to this important debate.
Bertrand Meyer is a professor at the Constructor Institute (Schaffhausen, Switzerland) and chief technology officer of Eiffel Software (Goleta, CA).
Join the Discussion (0)
Become a Member or Sign In to Post a Comment