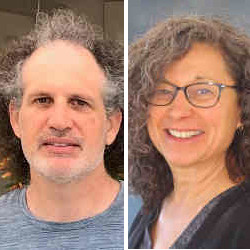
Introduction
If your research is in data science and you wish to present your insights and research about the teaching of data science, you most probably will have no choice but to try submitting your manuscript either to a journal that deals with a data science related topic and is dedicating one of its special issues to data science education (e.g., FODS – Foundations of Data Science's special issue on data science education or The Statistics Education Research Journal (SERJ)'s special issue of research on data science education) or to one of the educational conferences on a topic related to data science (e.g., SIGCSE – the special interest group on computer science education). One exception is the Journal of Statistics Education, published by The American Statistical Association, which in January 2021 changed its name to the Journal of Statistics and Data Science Education. The name change reflects the growing attention and importance attributed to data science education. This journal, however, still holds the statistics perspective and targets mainly the statisticians' community. For example, its first issue of 2021 is dedicated to Computing in the Statistics and Data Science Curriculum.
In other words, no journal exists today that deals exclusively with data science education, let alone highlights data science education from an interdisciplinary perspective. In this blog, we describe our vision for such a journal that would focus on data science education from the interdisciplinarity perceptive. For the purpose of this blog we will call it The Interdisciplinary Journal of Data Science Education (IJDSE).
Motivation
Data science is a new interdisciplinary field of research that focuses on extracting value from data and integrates knowledge and methods from computer science, mathematics and statistics, and the domain knowledge of the data. As an interdisciplinary field, it receives a lot of attention, mostly separately and independently, from each of the disciplines that comprise it, and so, the potential of its interdisciplinarity nature has not yet been fully exhausted and should be explored more deeply. We suggest that this statement applies also to the educational aspect of data science.
Accordingly, IJDSE would aim to explore the interdisciplinarity of data science from the education perspective. Such an examination would educate future learners better and more effectively than when the educational perspective is taken separately by each of the disciplines that make up data science. This statement relies on the working assumption that when data science education is explored from each angle separately, learners are not exposed to the comprehensive picture and interdisciplinary nature of data science needed in order to use it meaningfully in their personal and professional lives.
Target Audience
Since IJDSE would be the only journal (at least at its early stages) focusing on data science education from the interdisciplinary perspective, we anticipate that professionals and researchers from all disciplines — exact and life sciences, engineering, social sciences and humanities — would be interested in the subjects the journal would cover. In addition, since data science is relevant to all industries today and they all, therefore, need to educate all their employees on how to use data in a way that adds value to the firm, they would probably also find IJDSE relevant for their growth.
We anticipate that a data science education community would form and grow dramatically in coming years, a trend that is reflected, for example, by the growing number of data science programs established recently in K-12 (e.g., The International Data Science in Schools Project (IDSSP), a high school data science curriculum that focuses on the role of data in social issues (Heinemann et al., 2018), the Mobilize Introduction to Data Science program (Gould, 2018), as well as our own experience (Mike, Hazan, and Hazzan, 2020)), in undergraduate and graduate level studies (e.g., Anderson, Bowring, McCauley, Pothering and Starr, 2014; Havil, 2019; Khuri, Vanhoven and Khuri, 2017; Tartaro and Chosed, 2015), and in industry (e.g., Amazon Machine Learning University).
Accordingly, IJDSE would:
- target the three communities that comprise data science — computer science, math and statistics, and the various data domains (e.g., natural sciences, social science, and digital humanities) — and within these communities, scholars who are interested in data science education.
- explore data science education for all levels: from K-12, through undergraduate and graduate programs, to academic scholars and industry professionals.
Scope and Topics
To capture the interdisciplinarity of the field of data science education, IJDSE would encompasses a broad topic list and would welcome all research methods: qualitative and quantitative, data science methods, design-based research, action research, case studies, experience reports, etc. It would publish research papers, case study reports, opinion papers, work-in-progress papers, and commentaries in the following topics:
- Data science education for all levels: K-12, undergraduate as major or minor, graduate, research, and industry
- Data science curriculum and study programs
- Design of data science study programs
- The structure of data science study programs
- Connections between data science education and education in mathematics, statistics, computer science and the domain of the data
- Data science education pedagogy
- Pedagogies and approaches to teaching data science to diverse populations
- Pedagogy of interdisciplinary education that bridges the humanities, social sciences, sciences, and other disciplines
- Teaching methods, tools, and practices of data science education
- Assignments and tutorials
- Class activities
- Data visualization and animation tools
- Data science project management
- Assessment
- Applications in various domain areas
- Pedagogical challenges, opportunities and risks
- Learners' cognitive and social processes
- Cognitive and behavioral perspective on data science education
- Cognitive and social biases in data science
- Metacognition
- Learner difficulties
- Learning styles and models
- Diversity, representation, and equality; Recruitment and retention of underrepresented groups in data science
- Teacher preparation for data science
- Teacher training programs
- Communities of data science educators
- The essence of data science education
- Is data science education different from other educational fields?
- Policy: Data science for all; Should everyone take an introductory data science course?
- The interdisciplinary challenges of data science education
- Characteristics of data scientists
- Ethical issues of data science
- Connection between data literacy, data science education, and other related topics
- University/public/profit and non-profit collaborations in data science education
- Specific topics in data science education – representative list
- Machine learning
- Artificial intelligence
- Data mining
- Bayesian statistics
- Historical perspective
- The data science cycle
Conclusion
In her 2020 article, Wing asks and answers: "What will data science be in 10 or 50 years? The answer to this question is in the hands of the next-generation researchers and educators" (Wing, 2020).
In this blog, we addressed the educational aspect of Wing's answer by proposing our vision for a journal dedicated to data science education — IJDSE — that would highlight the interdisciplinarity nature of data science and address the growing community of data science educators from a wide-range of disciplines, organizations and fields of research. IJDSE would be a place where such scholars would be able to share their practice and research-based expertise in data science education. Thus, beyond the contribution to the creation of a new interdisciplinary field of research, IJDSE would support the creation of an important and influential worldwide community of data science education researchers.
As an interdisciplinary journal, IJDSE would enable scholars from different fields of research to learn from each other, improve their professional work, and educate the 21st century professional data scientists as well as non-scientist citizens to use data in a responsible, ethical and meaningful way. Furthermore, since the potential contributors to IJDSE are the pioneers of data science education, they would have the potential to influence the creation of new curricula and study programs.
We note that the IJDSE would aim to address several of the challenges of data science education that we have already identified and published in our July 2020 blog, Ten Challenges of Data Science Education, including the interdisciplinarity nature of data science and its diverse body of learners and teachers.
To conclude, we suggest that it is urgent to indeed establish an educational journal for data science that approaches data science education from the interdisciplinary perspective and, to that end, we call all potential stakeholders to take up the gauntlet and establish such a platform for all data science educators. We would be happy to assist in any such initiative in its establishment process and to offer our comprehensive perspective on data science education.
References
P. Anderson, J. Bowring, R. McCauley, G. Pothering, and C. Starr, "An undergraduate degree in data science: Curriculum and a decade of implementation experience," in SIGCSE 2014 – Proceedings of the 45th ACM Technical Symposium on Computer Science Education, 2014, pp. 145–150, doi: 10.1145/2538862.2538936.
R. Gould, "Mobilize: a Data Science Curriculum for 16-Year-Old Students," vol. 10, 2018, [Online]. Available: https://sandbox.mobilizngcs.org/#demo.
B. Heinemann et al., "Drafting a Data Science Curriculum for Secondary Schools," ACM Int. Conf. Proceeding Ser., November, pp. 1–5, 2018, doi: 10.1145/3279720.3279737.
J. Havill, "Embracing the liberal arts in an interdisciplinary data analytics program," SIGCSE 2019 – Proc. 50th ACM Tech. Symp. Comput. Sci. Educ., pp. 9–14, 2019, doi: 10.1145/3287324.3287436.
S. Khuri, M. Vanhoven, and N. Khuri, "Increasing the capacity of STEM workforce: Minor in bioinformatics," in Proceedings of the Conference on Integrating Technology into Computer Science Education, ITiCSE, Mar. 2017, pp. 315–320, doi: 10.1145/3017680.3017721.
K. Mike, T. Hazan and O. Hazzan, "Equalizing Data Science Curriculum for Computer Science Pupils", Koli Calling – International Conference on Computing Education Research, November 2020, Article No.: 20, pp 1–5, doi: https://doi.org/10.1145/3428029.3428045
A. Tartaro and R. J. Chosed, "Computer scientists at the biology lab bench," SIGCSE 2015 – Proc. 46th ACM Tech. Symp. Comput. Sci. Educ., pp. 120–125, 2015, doi: 10.1145/2676723.2677246.
J. M. Wing, "Ten Research Challenge Areas in Data Science", Harvard Data Science Review, 2(3). September 2020, https://hdsr.mitpress.mit.edu/pub/d9j96ne4/release/2
Orit Hazzan is a professor at the Technion's Department of Education in Science and Technology. Her research focuses on computer science, software engineering and data science education. For additional details, see https://orithazzan.net.technion.ac.il/. Koby Mike is a Ph.D. student in the Technion's Department of Education in Science and Technology under the supervision of Orit Hazzan. Koby's research focuses on data science education.
Join the Discussion (0)
Become a Member or Sign In to Post a Comment