To what extent could artificial intelligence (AI) guide and help us in our efforts the maintain and strengthen democracy? In a recent article in Science, Tessler et al. (2024) offer an impressive exploration of how AI can improve human deliberation and help humans find common ground. Their findings are statistically sound, showing AI’s ability to facilitate consensus, even in contentious discussions.
However, beyond the empirical results, the article raises fundamental questions about deliberation’s nature and role in democracy as well as the limits of technology—even AI —for automating core democratic processes. Though the authors present the Habermas Machine as a way to improve deliberation, several conceptual issues arise, and they arguably propose an overly simplistic view of deliberation, potentially undermining key benefits of democratic deliberation should their solution be widely implemented.
What Counts as Deliberation?
The first issue concerns how deliberation is conceptualized. The article draws on Habermas’s theory of communicative action, claiming that AI helps participants reach consensus through rational dialogue. Yet, calling isolated, machine-mediated interaction “deliberation” seems misguided. Automated consensus-finding without human interaction cannot reasonably be called deliberation.
Deliberative democracy has evolved from Habermas’s focus on rational consensus to newer models emphasizing pluralism, conflict, and dissensus (Dryzek, 2000; Elstub et al., 2016). The ‘systemic’ turn stresses evaluating deliberation at a systems level, not isolated instances like mini-publics (Elstub et al., 2016). By focusing on maximizing agreement, the Habermas Machine reduces deliberation to optimizing language, not fostering the deep engagement vital to democracy. Deliberation goes beyond finding common ground—it must explore conflicting ideas and values.
The Problem with AI-Mediated Human Interaction
Imagine people isolated in pods, interacting only with the Habermas Machine to develop policy. Without direct interaction, Tessler et al. still consider this deliberation. This neglects the relational and interpersonal dynamics crucial to democratic deliberation. Physical human presence—imperfections and all—might be essential for realizing deliberation’s full benefits (Min, 2007).
Deliberation isn’t just exchanging opinions; it’s a social process involving trust, empathy, and understanding. Tessler et al. suggest their machine bypasses interpersonal frictions, but these are where the most meaningful democratic work occurs. Disagreements and emotional responses help participants understand underlying values. Such frictions may better satisfy deliberation criteria—mutual respect and acknowledgment (Mansbridge, 1999)—than mere support for AI-generated statements. These criteria require direct engagement between humans, not interaction with a machine.
The Habermas Machine turns deliberation into a process of optimizing statements for agreement, which may streamline discussions but at the cost of the deep, messy, and relational elements that define democratic participation. It also treats individuals as isolated information processors rather than engaged citizens.
Missing Variables: Social-Relational Factors
Another significant critique of Tessler et al.’s study is their failure to include key variables relevant to deliberative democracy. While the authors focus on agreement and endorsement as metrics of successful deliberation, they overlook other essential factors, such as mutual respect, trust, and the development of empathy between participants. These social-relational outcomes are critical components of democratic deliberation, and their absence in the study’s evaluation of AI-mediated deliberation is notable.
For example, Tessler et al. do not measure how participants’ attitudes toward each other change over the course of deliberation. Do participants come away with a greater understanding of opposing viewpoints? Do they develop respect for those with whom they initially disagreed? Or does the AI simply help them find a linguistic compromise without addressing the underlying tensions? Without answers to these questions, it is difficult to assess whether the Habermas Machine truly fosters democratic engagement or merely creates the illusion of consensus.
AI and the Perception of Neutrality
Finally, the study raises important questions about how AI is perceived in deliberative settings. Tessler et al. note that participants tended to view AI-generated statements as more neutral and less biased than those written by human mediators. AI’s perceived neutrality is both a strength and a risk. It may mitigate biases, but also lead to overreliance on AI as an objective arbiter, ignoring the political nature of AI systems.
If participants believe that AI-generated statements are inherently more neutral or fair, they may be less likely to critically engage with the substance of those statements. The danger here is that AI could be seen by the participants as a solution to political disagreement, rather than a tool for helping them engage deeply with their fellow citizens. In this sense, the Habermas Machine risks becoming not a deliberation tool, but a consensus machine.
In addition to a danger of faith in the machine changing our approach to deliberation, is there a chance we also evaluate the output of machines differently from that of humans? Studies show that humans tend to morally evaluate the actions of humans and AI agents differently (Malle et al., 2019). There is a need to explore whether AI statements are perceived and evaluated differently than those from human mediators, even if identical. For example, humans may be less likely to assign blame or praise to a machine, and to endorse offers from machines than they might from humans, as they are perceived as neutral agents without emotion or strategic motives.
Reimagining AI’s Role in Deliberation
While Tessler et al.’s study contributes to AI-mediated deliberation, it raises questions about democracy’s future in the age of AI. AI should not replace all interpersonal aspects of human deliberation. AI should complement it and support it, but not replace the messy and seemingly inefficient—but quite essential—relational work of democratic engagement. As with similar efforts to “fix” or “solve” democracy with AI—such as “Democratic AI” (Koster et al., 2022)—the proposed deliberation machine risks diluting the concept by only dealing with parts of the underlying theories it purportedly builds on (Sætra et al., 2022).
Deliberation is not a process that can be optimized through technology alone. Consensus finding might, however, be. Deliberation requires empathy, trust, and a willingness to confront conflict—not just a mechanism for finding common ground. If AI is to play a role in the future of democratic deliberation, it must do so in a way that enhances, rather than diminishes, the human and relational elements of political life.
References:
Dryzek, J. S. (2000). Deliberative democracy and beyond: Liberals, critics, contestations, Oxford University Press.
Elstub, S., Ercan, S., and Mendonça, R. F. (2016). Editorial introduction: The fourth generation of deliberative democracy. Critical Policy Studies 10(2), 139-151. https://doi.org/https://doi.org/10.1080/19460171.2016.1175956
Koster, R., Balaguer, J., Tacchetti, A., Weinstein, A., Zhu, T., Hauser, O., Williams, D., Campbell-Gillingham, L., Thacker, P., Botvinick, M., and Summerfield, C. (2022). Human-centered mechanism design with Democratic AI. Nature Human Behaviour. https://doi.org/https://doi.org/10.1038/s41562-022-01383-x
Malle, B. F., Magar, S. T., and Scheutz, M. (2019). AI in the sky: How people morally evaluate human and machine decisions in a lethal strike dilemma. Robotics and Well-Being, 111-133.
Mansbridge, J. (1999). Everyday Talk in Deliberative Systems. In S. Macedo (Ed.), Deliberative politics: Essays on democracy and disagreement. Oxford University Press.
Min, S.-J. (2007). Online vs. face-to-face deliberation: Effects on civic engagement. Journal of Computer-Mediated Communication 12(4), 1369-1387. https://doi.org/https://doi.org/10.1111/j.1083-6101.2007.00377.x
Sætra, H. S., Borgebund, H., and Coeckelbergh, M. (2022). Avoid diluting democracy by algorithms, Nature Machine Intelligence 4(10), 804-806. https://doi.org/https://doi.org/10.1038/s42256-022-00537-w
Tessler, M. H., Bakker, M. A., Jarrett, D., Sheahan, H., Chadwick, M. J., Koster, R., Evans, G., Campbell-Gillingham, L., Collins, T., and Parkes, D. C. (2024). AI can help humans find common ground in democratic deliberation, Science 386(6719). https://doi.org/10.1126/science.adq2852
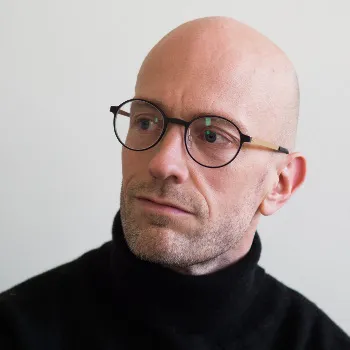
Henrik Skaug Sætra is a researcher in the field of the philosophy and ethics of technology. He focuses specifically on artificial intelligence, and much of his research entails interrogating the various linkages between technology and the environmental, social, and economic sustainability.
Join the Discussion (0)
Become a Member or Sign In to Post a Comment