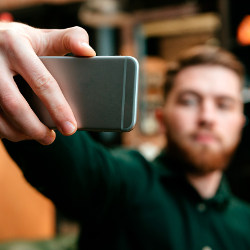
Self-portraiture has become ubiquitous. Once an awkward feat, the “selfie”—a picture of one’s self taken by one’s self, typically at arm’s length—is now easily accomplished with any smartphone, and often shared with others through social media. A 2013 poll indicated selfies accounted for one-third of photos taken within the 18-to-24 age group. Google estimated in 2014 that 93 billion selfies were taken per day just by Android users alone.10 More recently, selfie taking has begun to influence human behavior in the physical world. Museums26 have started to develop environments that cater specifically to Instagram and Snapchat users. Even facial plastic surgeons have observed an increase in the number of patients that seek plastic surgery specifically to look better in selfies (55% of surgeons had such patients in 2017, up 13% from 2016).2 Perhaps most strikingly, plastic surgeons have begun reporting a new phenomenon termed “Snapchat dysmorphia,” where patients seek surgery to adjust their features to correspond to those achieved through digital filters.28
Key Insights
- Nowadays, anyone with a sufficiently powerful smartphone is able to make sophisticated edits to their photos and videos.
- By editing selfies, we change the way people perceive us and the way we perceive ourselves.
- The change can be positive or negative, and we must be mindful how we edit and evaluate photos.
- Researchers and software developers in the field should have a broad view that considers not only technology, but also cognitive science, psychology, and ethics.
Photographs have long played a role in shaping our perception, and self-portraiture has existed almost as long as photography itself. Even early analog portrait photography offered powerful opportunities for personal identity formation and expression.35 Digital photography built on these opportunities by providing new ways of capturing, disseminating, and editing personal photos. Camera-equipped smartphones greatly increased the number of people who could photograph themselves. Similarly, social media platforms amplified the ability to share personal portraits with others. Selfies represent a culmination of the personal and social dimensions of digital photography. Yet, while the selfie phenomenon demonstrated the ease of capturing and sharing self-portraits, until recently, the process of editing self-portraits has required extensive professional experience and skill.
This is beginning to change. A new class of digital photo manipulation technologies has begun to emerge—ones that enable complex, realistic, and automatic edits to digital portraits. The speed and ease offered by these new tools means that anyone with a sufficiently powerful smartphone is able to make sophisticated edits to their image. These editing technologies have implications, not only for the photos people share, but also for how the takers of those photos see themselves. As the Snapchat dysmorphia phenomenon illustrates, the act of editing one’s selfie can change one’s expectations for physical appearance in real life.
Our objective in this article is two-fold: to provide an overview of state-of-the-art techniques for portrait manipulation, and to explore the implications of widespread use of these techniques on self-perception. In doing so, we seek to start a dialog on how potential consequences of these technologies—both positive and negative—should factor into decisions about how and why we choose to develop similar technologies in the future.
We discuss six categories of automated portrait editing technologies and the impact these approaches can have on self-perception. The ability to adjust the perspective and pose of a portrait will enable people to disguise the fact they have taken a selfie. Digital makeup suggests ways to increase self-esteem and one’s professional appearance, but it could also increase the narcissistic perception of selfies in general. Facial adjustment algorithms offer ways to improve people’s satisfaction with their digital portraits while also suggesting the potential to normalize certain facial proportions and features. Technologies for automatically swapping hair and wardrobe in photographs provide a new form of online identity exploration while also opening new risks for appropriation. Algorithms for shifting the age of a person’s photograph will enable people to selectively choose how old they appear for different contexts online and change peoples’ expectations for how they will look in the future. Techniques that turn still photos into video portraits can enhance photos with dynamic expressions, but the expressions might be taken from other people, raising questions about the authenticity of the emotions in the video. We follow with a discussion of the broader impacts of widespread portrait editing on media consumption, trust, and personal appearance.
Portrait Manipulation
Portrait photography is a complex process, for which many elements determine the final result. First and foremost, the subject of the photograph, their head pose and expression, their makeup and their clothes are all reflected in the photograph. Scene elements such as lighting conditions, camera location, and focus also play a substantial role. Capable photographers also take into account more “technical” details such as sensor sensitivity (ISO), aperture, and shutter speed in order to compose an effective shot.
With traditional print photography, these attributes of a portrait were largely baked into the photo at the time the shutter was closed. Afterward, skilled photographers could “dodge and burn” to locally modify exposure during printing, and for high-value shots like fashion photographs artists would even paint over a print using an airbrush in order to modify it. Today, with digital editing software like Adobe Photoshop, such operations are commonplace. But it may surprise some readers to learn that expression, makeup, pose, and even the identity of the subject can now, or in the near future, be easily modified in post processing, with no need for domain expertise or advanced image manipulation skills. Very soon, digital face and body editing will be as facile as Instagram filters are today—immediately accessible to anyone who can take a selfie.
Very soon, digital face and body editing will be as facile as Instagram filters are today—immediately accessible to anyone who can take a selfie.
Perspective and pose. Subtle details in how a photo is taken can have a substantial impact on how the subject of the photo is perceived by others. The distance between the camera and subject plays a key factor in perception. Faces imaged from closer distances appear to be more benevolent (good, peaceful, pleasant, approachable), while larger distances correlate with smart and strong appearance.27 Furthermore, people rate photographs of faces taken from within personal space (that is, “too close”) as less trustworthy, competent, and attractive.5 Selfies, one of the most prevalent forms of modern personal photography, are taken by definition at closer distances and exhibit noticeable perspective phenomena. As a result, while the convenience, affordability, and ease of selfies has allowed a broader range of people to participate in personal photography, the limits of photography at close distances means these same people are fundamentally constrained in the ways they can portray themselves to others.
We created a system that, given a single photograph as input, can virtually change the location of the camera to produce a new image, with different perspective.13 Our system produces photorealistic results through a combination of 2D and 3D techniques. We use commonalities in the appearance of heads to estimate the photo’s 3D structure, and then move pixels around on the 2D image plain to produce the final result. This approach allows for arbitrary pose changes, and the creation of 3-dimensional heads from 2-dimensional photos (Figure 1). The estimated 3D model is a rather weak approximation of the true head shape but is enough to describe a convincing 2D warp that produces a realistic result. This 2D-3D hybrid approach has also proved successful for other face manipulation tasks such as expression transfer.39 As capture hardware and algorithms improve, we expect better 3D models from single or multiple photos, which will further improve 3D-based photo editing.
Figure 1. Given a single input photograph (a) we can change perspective and pose. We remove the “selfie effect” caused by a short camera-to-subject distance (b), rotate the head (c), and create 3D anaglyphs from a 2D photo (d, use red-cyan glasses to view).
Automatic perspective adjustment will allow selfie takers to distinguish between the impacts of the camera lens, angle, and distance, and their actual facial proportions. Individuals who assume that they have undesirable facial characteristics can now view their pictures from multiple perspectives and get a more accurate sense of how their faces appear to others. These techniques will also increase the expressiveness of the selfie as a tool for self-presentation. From minor changes, such as shifting ones’ pose to a more attractive angle, to major changes like adjusting the perspective of one’s face to appear more competent and intelligent for a LinkedIn profile, more people will be able to make perspective adjustments that align with how they wish to be perceived in different online environments.
Makeup. Physical makeup can alter our own perceptions of ourselves, and also change how others see us. Bloch and Richins demonstrated that makeup can temporarily increase the wearer’s self-esteem4 and Etcoff et al. showed that people wearing minimal amounts of physical makeup are often perceived as more likeable and competent.11 Today, makeup use has become prevalent among the general public as cosmetic products have become cheaper and more widely available.17 Yet successfully applying physical makeup can be difficult—requiring skill in both selecting the right products and applying them correctly.
Since the advent of portrait photo editing, makeup has also been applied to photos—first through physical retouching methods and later through digital tools like Photoshop. Like physical makeup application, digital makeup creation has, until recently, also required specialized skill and expertise. Recent developments in automated portrait editing have greatly lowered the effort necessary to apply digital makeup. In one example, we introduced a system that can apply and remove makeup.9 Given a pair of photos—a source photo s without makeup and a reference photo
showing a makeup style—we automatically generate a new picture
showing s wearing makeup in the style of
(Figure 2). The approach leverages recent advances in image style transfer based on deep learning. As is typical in machine learning projects, a good data set is essential. However, for this project it would be very difficult to acquire ground truth triplets (s,
,
). Our approach instead learns two functions: makeup transfer function T(s,
) →
, and makeup removal function R(
) → r that can remove makeup. The key insight that permits us to train these functions is that we can actually apply them twice sequentially, yielding the original image pair. This insight relies on the observation that T(r,
) →
and R(
) → s. This allows us to train with image pairs of different people.
Figure 2. Source photos (top row) are each modified to match reference makeup styles (left column) to produce nine different outputs (3 x 3 lower right).9
Given the impacts that physical makeup has on self-image, it is likely that digital makeup will also have an effect on how we view ourselves. The professional edge offered by physical makeup is arguably easier to attain (for digital contexts) through automated makeup filters. These same filters may offer smartphone users quick self-esteem boosts at the touch of a button. More broadly, the ease of digital makeup transfer will make it easy for people to experiment with a range of different makeup styles. This flexibility could have multiple benefits. It suggests an opportunity for more people to engage in playful experimentation with their appearance and build confidence in their online portrayal. Furthermore, digital makeup could provide an opportunity to preview an effect before investing the time and money to recreate it in real life. The benefits of moderate amounts of physical makeup suggest that automated makeup filters may lower the threshold for presenting oneself as competent and confident when online. Conversely, large amounts of makeup, while increasing attractiveness, can also lead to perceptions that a person is untrustworthy or narcissistic.11 People already view posting selfies as a narcissistic act,10 therefore increasing prevalence of digital makeup in selfies may perpetuate negative attitudes towards selfie takers.
Facial features. The shape and relative location of facial features define how we look. Characteristics such as a pointy nose, big eyes or an elongated face are all derived from facial features. Some features can be changed at will (a smile), some can be changed over time (a skinny face) and some are tightly coupled with bone structure (weak jaw). In the physical world, the latter can only be changed via plastic surgery, and not all results are achievable.
Until recently, major digital edits to the face, like reshaping the eyes and nose, required substantial skill and knowledge. Whereas previous digital editing paradigms required users to select from low-level, general tools like digital paintbrushes, and skillfully apply the tools to produce believable results, new automated digital approaches make it possible to immediately transform individual features with believable results. Unwanted eye-blinks and sideways glances are common in photos of individuals, and even more likely to appear in group photos. Shu et al.32 automatically edit eyes in photographs by leveraging a user’s personal photo collection. They find good reference eyes in the personal collection and transfer them to the target (Figure 3 top). Transferring features between photos is not limited to eyes, nor to portraits.1 Yang et al.39 transfer facial expressions from one photo to another (Figure 3 bottom). In addition to making local edits to the target feature, this method has the important effect of also enacting subtle adjustments to adjacent features and face shape.
Figure 3. Top: Closed eyes (left) are automatically opened (right, showing three example results).32 Bottom: a photos with no smile (a) is enhanced by using a smile from another photo (b) to create a final composition (c).39
An alternative approach holistically considers all face features simultaneously. Leyvand et al.23 created a data-driven technique for face beautification. Their system is trained to warp images so the relative location of facial features matches images of faces that people rated as more appealing. The warp is trained to stay close to the input and users can adjust the modification amount, resulting in portraits that preserve characteristics of the original face. However, because Leyvand’s approach does not use a physically based model, it can produce facial transformations that are either impossible, or would require extensive facial surgery to achieve in real life. Leyvand’s approach is also distinguished from Shu and Yang’s; rather than optimizing portraits based on features drawn from images of the same person, Leyvand’s algorithm adjusts images according to optimum derived from images of other people. The automated nature of facial-feature editing also means that facial editing can now be directly integrated into the camera viewfinder.24 In some cases, a suite of effects is applied by default in real time, meaning that from the moment the user opens the application, they are presented with an adjusted image of their face.
The integration of automated facial adjustment algorithms with personal cameras will affect our perception of self attractiveness. Dissatisfaction with aspects of one’s appearance is part of being human, and cultural beauty ideals existed well before digital photography. In one sense, tools that enable people to optimize their portraits by combining personal images are poised to broaden the range of people who can produce photos that represent them at their best. The use of tools that adjust facial features according to the photos of others presents a less clear-cut outcome with regards to self-image. Flipping between an untouched image of their face, and one adjusted to some external standard could lead to people identifying “flaws” in their appearance that they were previously unaware of.
Hess argues that beautification filters create a situation where people compare their image to an idealized version of themselves, rather than to external ideals like celebrities or models.16 The before and after comparison afforded by these technologies may also refine people’s understanding of how far their individual facial features are from an idealized norm. Rather than having a vague sense that one’s chin is too big, a person can now immediately see how small an algorithm thinks their chin should be. All algorithms, by definition, contain built-in biases determined either by the preferences of the algorithm designers or by the data used to train the algorithm. Whereas previously beauty norms were influenced by people in the fashion and marketing industries, new norms will be determined by the algorithms themselves. It’s important to recognize that, like human biases, algorithmic biases can unfairly discriminate against minority groups and can reinforce or amplify existing racial and gender stereotypes.6
Age. Age shapes both how we perceive others, and the way we perceive ourselves. Age can affect attitudes toward a person’s competence as demonstrated in one study where younger raters rated older workers as less qualified and as having less potential for development in comparison to younger workers.12 Age also affects how we perceive attractiveness. Culturally, we often associate beauty with youth, identifying attractive people as younger than they actually are, or characterizing young people as more beautiful than older people.22 Until now, personal photos have primarily reflected the physical age of the person relative to date they were taken. This quality has largely defined the role portraits have served in family life, by providing a way to document family members’ age progression over time and mark key moments of coming of age. The act of reviewing personal portraits from different stages in one’s life plays an important function in personal commemoration and memory.35
Altering the age of a person in a digital photo, even by a few years, is a difficult task. A person’s future self depends on their current appearance, but also on invisible genetic traits and unforeseeable environmental conditions. Nevertheless, recent tools for automatic age adjustment have emerged that make it feasible for anyone to make extreme shifts in age of their portrait. Most notably, Kemelmacher-Shlizerman et al.20 use a large dataset of photos of various ages to calculate typical differences between age groups. They then apply the differences to a new photo of a baby, producing age-progressed result from childhood to old age.
Automatic age adjustment fundamentally broadens the nature of personal photography. Whereas photos previously served as a tool to document a person’s appearance at a specific moment, they will now provide a starting point for projecting how a person looks across multiple points in time. Photographic age will become something anyone can actively manipulate and control in a digital context. Just as people currently falsify their age on online dating sites to appear more desirable,15 people will now be able to alter their photographic age to appear more attractive, professional, mature, or youthful, depending on the context. Automated portrait aging will also affect young people in important ways. Children who transform their own portraits will have a different understanding of how their appearance will change as they grow older. They will be able to preview the effects of aging immediately, rather than experience them gradually over time, and have different expectations about how their features will change as they age.
This technology could also be used to motivate lifestyle change. With the right data, we could present alternate futures. A person could forecast how they might look in 10 years if they engage in healthy behaviors like regular exercise, or harmful behaviors like smoking.
Hair, wardrobe, and style. In the physical world, people experiment with different hairstyles and clothing choices to express different aspects of their identity. Psychologists have theorized that, particularly for younger people, low-risk experimentation with self-presentation can serve an important role in personality development. Digital communities have acted as an extension for physical forms of identity experimentation by providing a virtual environment where people can inhabit different avatars, or present different personas in online communities.34
Today people can also experiment with their wardrobe and hairstyle of their digital self-portraits. Kemelmacher-Shlizerman19 introduced a system to automatically swap the face of an existing photo with a target portrait (Figure 4). The inputs to the system are a photo of a person and a search term, such as “curly hair” or “1930.” The system retrieves Internet photos that match the search term and blends the input face to the Internet photos. The result is a photo with a style that matches the search term, containing the given face. The key here is that styles are often determined by hair or clothing, thus we can swap faces without drastically changing style.
Figure 4. Given an input photo and a target style (text string), the system of Kemelmacher-Shlizerman19 automatically retrieves Internet photos and swaps faces to produce the input person in the target style.
In some ways, the ability to digitally alter our clothing and hairstyle offers a new channel to extend benefits of fashion experimentation in the physical world by providing people with an easier, faster, and cheaper method to try out different looks. Furthermore, because photo-based methods of hair and clothing transfer enable styles to be transferred from photos found via Internet search, and applied to images of an actual person, rather than an avatar, this technique could avoid some of the limitations imposed by avatar based systems where either system designers or skilled users have control of the range of options available to users.18
This approach also has important constraints that can affect the self-perception of the people who use it. The facial transfer algorithm works best for images with similar looking faces. The effectiveness of a search for “movie star” or “scientist” will reflect the range and number of online images in these categories that most closely match the gender and ethnicity of the user, thereby reflecting and reproducing established trends and biases in online photo repositories. A similar issue emerged when Google released an app that matched people with similar faces within classical art and many non-white users found themselves matched with artworks reflecting racial stereotypes.31
Hairstyle and clothing transfer also have broader cultural and political implications for how we present and perceive identity. In countries with racial and ethnic diversity, trends in fashion often intersect with social tensions like racial stereotyping and cultural appropriation. Stereotyping and cultural appropriation in the real world can reduce self-esteem among disenfranchised minority groups who experience it.14 Digital techniques that enable people to experiment with clothing, hairstyles, and albeit unintentionally, skin-tone, from photographs will dramatically increase opportunities for people to represent themselves with styles of other subcultures. While this could prove empowering for the people doing the experimenting, it could have the opposite effect for the minority groups whose cultural styles are appropriated.
Video portraits. All the methods introduced thus far operate on photos—a moment frozen in time. Similar elements determine how we look in videos, with an added temporal dimension. For example, a smile is no longer just one photo taken at the apex of the smiling process, but a trajectory of motion, starting with a hint and ending with an ear-to-ear smile.
When considering videos, the added temporal dimension introduces both opportunity and challenge. Moving portraits can be more expressive, but more difficult to produce and manipulate compared to static photos. Averbuch-Elor et al.3 introduced a method that can animate an input photo, producing results akin to the moving portraits in the Harry Potter series (Figure 5). They took upon themselves the challenge of using only a single input photo of the person to animate. Their key contribution is in finding a way to transfer another person’s motion to the input photo, producing compelling results that can be applied to both current photos and historic figures, for which video footage is unavailable. Interestingly, since the driving video is of a different person, the result might couple the facial appearance of one person with the mannerisms of another.
Figure 5. The method of Averbuch-Elor et al.3 can create moving portraits from still photos. Given a single input photograph (top) and a reference video (not shown), a new video is created with dynamic expressions from the reference (selected frames shown, bottom 3 rows).
Instead of limiting the input to a single photo, other methods try to learn what a person looks like in a video, and use that knowledge to generate synthetic head motion, expressions, and speech. Deep Video Portraits21 puppeteer one person using a video of another, allowing control over head pose, expressions, and eye gaze (Figure 6). They train a neural network to convert synthetic head renderings to a photo-realistic video frame. They then perform puppeteering by rendering heads with the identity of one person and other parameters (pose, expression) of another, producing the final video using their neural network. Input modalities other than head renderings can also be converted to video portraits. Wang et al.36 show sketch-to face video results, allowing a few brush strokes to control facial appearance. Suwajanakorn et al.33 convert an audio speech to a video of a person giving that speech. Improved controls for dynamic faces remains an opportunity for future research.
Figure 6. Deep Video Portraits21 transfer pose, expression and eye gaze from a source video (top) to a target video, producing convincing results (bottom). Resulting frames are generated by the method, and need not appear in the original video.
The emergence of automated video manipulation algorithms will make editing videos of our faces and bodies ubiquitous. At present, much of the attention on algorithmic video synthesis focuses on the risks this technology poses for information falsification, concerns we discuss later. However, it is also important to recognize the impact that ubiquitous video editing will have on self-perception. Each technique we described—adjusting pose, makeup, facial features, age, and style—will be adapted for video. Moreover, we will be able to alter temporal expressions of emotion. People may choose to amplify the emotional quality of a video, for example editing a karaoke video to correspond with the posture and poise of a professional pop star. Or, they may choose to replace the recorded emotions, swapping the disapproving head shake of a relative in a home movie with a nod and a smile.
Implications
As we demonstrate, most, if not all portrait elements can be digitally manipulated. A person in a photo might, in real life, be older, or have a different facial structure. A photograph of a person in an exotic location may, in reality, portray someone who never left their hometown. If the subject is moving, that does not mean that a real video was ever captured.
The emergence of automated video manipulation algorithms will make editing videos of our faces and bodies ubiquitous.
These forms of photo manipulation were possible before the development of the techniques we describe. More than 20 years ago the special effects team of Forrest Gump (1994) were able to create convincing videos of the movie’s eponymous protagonist sitting with John Lennon and shaking hands with President Kennedy. More recently, the actor Paul Walker was digitally inserted into Furious 7 scenes after his death (2015), and a young version of Arnold Schwarzenegger appeared in Terminator Genisys (2015). In fact, manipulation in the movie industry is now commonplace, producing convincing virtual characters or digitally de-aging famous actors. The important difference between visual effects in mainstream movie production and techniques presented here is the amount of labor and expertise necessary to achieve them. Rapid, automatic methods for portrait editing will broaden the range of people who can use these techniques, extend the domains and contexts in which they will be applied, and amplify impacts that digital manipulation has on self-image as a whole.
Democratization vs. distortion. As individuals, and as a society, we should strive to judge people for qualities beyond how they look. Unfortunately, at present, our physical appearance measurably impacts how we are treated by others. As we reflect on ways to change this, we must also recognize the desire to reshape personal appearance is a reasonable response in a world where beauty standards still exist. Moreover, the growing presence of social media and the Internet in daily life has created new expectations for how we present ourselves digitally, and new consequences for failing to adhere to cultural appearance standards. From this viewpoint, the democratization of tools to alter our digital appearance is important for individual empowerment. Yet making it easier to modify one’s digital self will increase the number of manipulated portraits people encounter overall. This, in turn, could increase dissatisfaction with one’s physical appearance and amplify the pressure to change it.
Take for example the interaction between social media use and adolescent body image. Salomon and Brown29 found that self-objectifying social media use predicted greater body shame among youth. Looking specifically at photo editing, McLean et al.25 found an association between self-photo editing and body dissatisfaction in adolescent girls. One explanation for this connection is that people who are already dissatisfied with their bodies naturally look for opportunities to digitally edit their online image. If true, this suggests that portrait editing tools can be empowering. They are a response to flawless fashion spreads, allowing everyone to compete in an ultra-Photoshopped society. This connection between photo editing and negative body image might also lead to an alternate conclusion: that the ability to edit one’s photos can increase body dissatisfaction by highlighting the gap between reality and the perceived ideal.
In the physical world, people must often walk a difficult line between being perceived as putting adequate effort into one’s appearance versus being perceived as deceptive. Similar challenges will present themselves when relying on digital forms of portrait manipulation. Algorithms that perform subtle adjustments may be more socially acceptable than those that produce realistic but dramatic differences between photo and reality. People who choose to substantially alter their appearance digitally may learn to portray such behavior as playful in an effort to avoid being seen as inauthentic or narcissistic.
Algorithms that perform subtle adjustments may be more socially acceptable than those that produce realistic but dramatic differences between photo and reality.
Synthesized storytelling. Automated portrait editing may also change the ways mainstream media delivers information to the public. News outlets have begun to experiment with virtual anchors to deliver news.38 The press release stated: “[The virtual anchor] has become a member of its reporting team and can work 24 hours a day on its official website and various social media platforms, reducing news production costs and improving efficiency.” Virtual anchors are still experimental, and it is not clear if an audience will find them engaging or trustworthy. Yet the potential advantages of such techniques are abundant; unlike traditional recording, synthesized anchors would enable dynamic changes to the news report to correct mistakes, translate a story into multiple languages, or respond on the fly as updates emerge. Such advantages could also transfer into other forms of information delivery including education and professional training.
Concerns over media manipulation are at a peak in many parts of the world, and the prospect of synthetic video has exacerbated fears that malicious actors will be able to deceive the public more easily.7 Given these concerns, and the fact synthetic video is one method among many existing means to manipulate information, it is useful to unpack the specific issues of video synthesis from the broader challenges of media falsification. The forms of portrait editing we describe in this article will undeniably expand the range of people who, if they choose to do so, can generate malicious false video content. It is the responsibility of the researchers who develop such technologies, ourselves included, to acknowledge this fact, and weigh the risks and benefits of developing such algorithms as we proceed in this research.
At the same time, the consequences of any malicious media creation, fake video or otherwise, are shaped by many different factors. Human editorial decisions, social media and search algorithms, and individual patterns of consumption determine the content people see. Cultural and political alignments, religion, education, family history, and many other complex factors shape what forms of media different people choose to trust. In our increasingly media-rich world, addressing the challenge of fake content will require systematic efforts to enact policy for how content is created, manipulated, and distributed. We must also get people to think critically about the media they see. There is already evidence that people have difficulty distinguishing between different types of media content—for example, an ad versus a news story.37 Distinguishing between “real” and manipulated photographs may pose an even greater challenge. This paper is one attempt to address this challenge by demystifying the state of the art in portrait manipulation. A broader solution might involve augmenting media studies curriculum, or even general education, with image processing techniques and algorithm design.
Conclusion
We have outlined emerging technologies for manipulating our facial structure, expressions, hair, makeup, clothing, and age, using state-of-the-art image and video synthesis methods. At an individual level, these techniques can enable one person to quickly and easily change their appearance. On a collective level, however, these technologies will fundamentally change the ways in which people present themselves to one another. As researchers continue to develop new technologies for manipulating the human face, it is critical to consider the magnitude of these changes and their impact on others. This requires considering the biases inherent in the data we rely on to drive these technologies. It necessitates constantly evaluating consequences of such technologies and be aware of the potential for unintentional harm. As we develop tools that are easier to use, we must also consider how automatically limiting some choices and enabling others will encourage some forms of self-expression and discourage others. One thing is clear, these technologies are bound to change the face of society.
Join the Discussion (0)
Become a Member or Sign In to Post a Comment