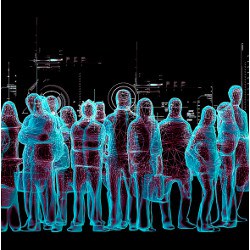
As we finalize this article November 11, 2018, and consider current and future directions for computing in Europe and across the globe, we remember the end of World War I exactly 100 years ago: the end to a war of atrocities at a scale previously unseen and the culmination of a series of events that European nations had allowed themselves to ‘sleepwalk’ into, with little thought for the consequences.10
When this article appears in spring 2019, we will remember the first proposal for a new global information sharing system written by Tim Berners-Lee 30 years ago at CERN,4 the European organization for nuclear research. This proposal marked the beginning of the World Wide Web, which now pervades every facet of modern life for over four billion users. However, the Web 30 years on, is not the land of free information and discussion, or an egalitarian space that supports the interests of all, as originally imagined.4 Rather, egotisms, nationalisms, and fundamentalisms freewheel on a landscape that is increasingly dominated by powerful corporate actors, often silencing other voices, including democratically elected representatives.
For seven decades Europe has been a political and social project, seeking to integrate what has been divisive historically and to make citizens more equal. While the proponents of the Web were driven by similar values, there is now increasing concern in Europe—and beyond—that the Web has become a vehicle of disintegration, polarization, and exploitation. What is more, since the Web operates at a global scale, beyond nation-states and with little formal regulation, we lack both the understanding and the means to avoid sleepwalking into another catastrophe.
Web Science seeks to investigate, analyze, and intervene in the Web from a sociotechnical perspective, integrating our understanding of the mathematical properties, engineering principles, and the social processes that shape its past, present, and future.7 Over the past 10 years, Web Science has made remarkable progress, providing the building blocks to face the challenges described here. And yet there is more do to. In this article, we offer a more detailed definition of Web Science and outline its achievements to date. We consider how Web Science frames and addresses key sociotechnical challenges facing the Web now and for the near future, emphasizing the importance of this as new artificial intelligences start to shape the Web (and Web Science) in significant new directions. Arising from this, we outline some of the practical strategies Web Science is developing to integrate knowledge across disciplinary boundaries and build collaboration with Web stakeholders. Web Science equips us to understand the past and present of the Web and the skills and tools to shape a positive future.
What Is Web Science?
Web Science in Europe begins from the premise that the Web is both technical and social. From this perspective, it is so difficult to disentangle the social from the technical that we describe the Web as ‘sociotechnical.’ The Web has been built on layers of communication at different levels of abstraction, from physical link layers (such as Ethernet) over Internet and transport layers (such as TCP/IP). It started as a Web of Documents (HTML), which served as the nucleus that other Webs would piggyback on: a Web of Data (RDF, SPARQL), a Web of Services (REST, JSON), a Web of Things.a
All these layers are defined by underlying technical standards and are the result of sophisticated engineering. And they are also deeply social, in two key ways. First, they have been developed in particular social contexts, with social goals in mind. For example, CERN was established to ensure a European nuclear capacity after the devastation of the research infrastructure in World War II.13 Similarly, the original intentions for the Web were to allow physicists to share data across teams underpinned by an intellectual commitment that information ‘wants to be free.’8 Second, the Web merely offered a set of opportunities for humans to develop and populate information constructs and link with each other. Over time we have seen multiple and competing rationalities drive the take-up of these opportunities. For example, information sharing and community building dominated academic and countercultural use in the early days. As new users began to embrace the opportunities on offer—for government and commerce in particular—content began to change. More than this, new users began to shape Web technologies—for example enabling user-generated content, video streaming, and secure online payments—in ways that, in turn, opened up new possibilities both positive, and less so.
The Web has changed the world and the world has changed the Web. And this is only set to continue, as the platform economy, the Internet of Things and new artificial intelligences offer new opportunities and shape the Web into the future.
For the past decade, Web Science has been building the interdisciplinary expertise to face the challenges and realize the value of this rapidly growing and diversifying Web. This task transcends the work of any single academic discipline.7 While our universities continue—overwhelmingly—to be organized in siloes established in the 20th century, or much earlier, the Web demands expertise from computer science, sociology, business, mathematics, law, economics, politics, psychology engineering, geography, and more. Web Science exists to integrate knowledge and expertise from across fields, integrating this into systematic, robust, and reliable research that provides an action base for the future of the Web.
How do we engage the public in meaningful dialogue and decision making about the future of the Web?
Evidence of our endeavors includes the networks of Web science labs, a number of undergraduate and postgraduate educational programs across Europe, summer schools on Web Science, and an ACM conference series.b We have understood how we may target to build ‘objective’ technology, yet end up with social stereotypes we wanted to avoid.2 We have learned about the social and the technical processes that are needed to provide open data for the social good,c the methodological and epistemological challenges of using new forms of digital data and computational methods for social research,15,16 and Web Science has progressed Social Machines that let us collaborate, yet work independently in distributed fashion.23
And yet there is much more to do. As a topical and critical example, we need to understand how the Web influences our democracies. Democracy builds on pillars like the representation of all, the rule of law, publicity and quality of information, temporality of decisions, and autonomy of individuals. The Web affects these pillars: online intimidation may threaten individuals and, silence them. Groups may organize online to ignore the law. Misinformation in echo chambers lowers the publicity and quality of information. In light of too much online transparency, compromises—which are vital in democracy—become infeasible. And, autonomy may be jeopardized by intrusion into private spheres. For all that, the Web continues to offer positive opportunities—voice to the otherwise silenced, connections between fragmented populations, mobilization of those who lack other means or are repressed—it is clear that these opportunities have come at a cost and—more broadly—that we may need to reconsider the pillars of democracy in digital society. These questions make Web Science more important now than ever. While Europe strives to respond to them in EU projects.d and various national endeavors thrive (for example, the Alan Turing Institute in the U.K. and, the German Internet Institute) we have only begun to face the challenges.
The Sociotechnical Challenges
There is nothing inevitable about the future of the Web. Its history to date has been made at the intersection of technical innovation and everyday practice with wider social processes and power relations, defying any prediction of fixed or finished outcomes. While this poses profound challenges—we cannot simply engineer the Web into a preferred state—we must develop integrated and in-depth socio-technical understandings of the Web if we are to influence its future direction.
Here, we describe two key developments that characterize the opportunities and challenges we face.
Datafication refers to the development that our everyday activities are traced digitally at unprecedented scale and accuracy for commercialization and exploitation in a data economy. Datafication raises questions about how this situation can or should be managed and what might result out of its pervasiveness. The processes of datafication, their consequences and how we live with these are both social and technical. From the beginning, the question of what data is created depends both on human activities and technical devices.
How this data is used depends on configurations of ownership, markets, state authority, and citizens’ rights as well as the technical affordances for circulation through technical infrastructures and the computational possibilities for analysis. To even describe the processes of datafication demands expertise of the highest level from computer science, law, political science, sociology, and more. To consider if and how society might respond to this new landscape likewise. What are the opportunities to flip data ownership from the big tech companies to the individuals whose data fuels the data economy? Engineering solutions, as developed in the SoLiDe project, may be part of the response, but how can we be sure that people even want let alone will have the capacity to use these solutions? What new challenges might these solutions pose? How would this impact on the underlying business model for the Web?
The digital divide. Web access continues to rise rapidly but over three billion people worldwide have no access, and 1:8 of the European population does not use the Web regularly.f We should avoid normative claims that the Web is ‘good’ for everyone, we know now that this is not the case, yet at the same time this should be a matter of choice not constraint. Further, beyond the question of access alone, we see an increasing divide between those highly skilled users who are able to derive the greatest benefit and those less skilled who are less knowledgeable about privacy risks, less able to protect their security and may derive less economic benefit from the opportunities available online.17 So long as people are unaware of the technical mechanisms and social uses of datafication or the potential effects of this on their lives and life chances they will not be able to make effective choices about how to use the Web or join the public debate about the future of the Web. Web Science calls for new approaches to digital literacy, beyond the use of Web tools and beyond the extension of coding skills to schools (important as both these are) to build understanding of the Web as a sociotechnical system and drive toward greater empowerment of Web citizens. It engages, for example, through the Web We Want campaign, #fortheweb, and educational interventions.11
Both these examples are linked to wider practical, political, and philosophical questions. What are the checks and balances with regard to openness and privacy? What forms of transparency and accountability are appropriate and achievable, to balance individual privacy, fairness across social groups and a viable business model for the future of the Web? How do we engage the public in meaningful dialogue and decision making about the future of the Web?
Next, we investigate another most prominent sociotechnical challenge in more detail that today is most often characterized as a technical challenge alone, whereas it is deeply entrenched into the way that we as individuals or as society interact with each other and with the artifacts we create.
Web and Artificial Intelligence
The Web and its infrastructure has become interwoven not only with documents, but also with data, services, things—and artificial intelligences. Initially, the Web was a field of application for artificial intelligence. Knowledge-based systems and machine learning were used to provide intelligent access to information on the Web, to enhance search, to facilitate browsing or to negotiate in electronics market. In hindsight, this may be considered to have been a very useful, but a shallow, piecemeal interaction between Web and AI.
Yet since the end of its first decade, there was a vision to build a Web that was intelligent in itself, that included agents that would assist its users.6 As this objective was beyond reach then, the Semantic Web community increasingly refocused on what became a proverb that data with a little semantics goes a long way. When researchers started to properly understand and use the social motivation of Web developers and Web content managers, some European researchers developed what now has become the two most popular Semantic Web applications, Wikidata27 and Schema.org.g At the same time Web Science was coined as a field that would address the systematic understanding of these socio-technical interactions between Web and humans.7
At the end of the second decade of the Web, artificial intelligence took several major turns. Big data, which frequently came from the Web directly or from crowdsourcing on the Web, became the foundation for human-like performance on some tasks such as image annotations.19 At the same time chatbots and virtual assistants have been developed and are now widely found on our PCs, smartphones, and in our homes. The latest developments let these virtual assistants acquire their knowledge from the Web, from archived dialogues,12 or from live interaction.
Microsoft researchers were pushing the edge and put their AI chatbot “Tay” online to interact with and learn from human encounters. Humans quickly taught it to go <<from “humans are super cool” to full nazi in <24hrs>>.h While there was a wide discussion that the technology was inadequate, there seemed to have been little understanding that it was the social context and the social processes that determined the fate of Tay. While in the initial Semantic Web, the lack of such understanding led to a simple, but not very problematic non-adoption, in the case of Tay being an active agent the lack of insight led to malbehavior.
The Web as a social medium, whether considering past contributions or ongoing interaction, is prone to misguide artificial intelligences. Indeed, the question arises, what the social values are that an AI on the Web should embed and how this should be realized? Efforts to censor the successor of Tay by ruling out topics like religion and politics hamper the chatbot leaving it socially awkward.25 Notions of social biases2 and data representativeness are interwoven, but who decides whether or when the answers are ‘right’? Several researcher communities (for example, Semantic Web, Computer-Human Interaction) and institutions have decided to actively tackle some underlying problems, for example, addressing the underrepresentation of women on Wikipedia by Edit-a-thons.
Finally, in two decades the Web has produced a range of highly valuable companies that did not play a major role before, or were not even founded when the Web started. Many of them benefit from first-mover and network effects that are difficult if not impossible to imitate by new companies. Will few big AI companies use their intellectual and computational power to rule the world using AI in the future? Or can society draw close, organizing the many and by sharing the necessary data and computation power bring AI to everyone’s fingertips? The CommonVoice projecti is certainly a project of developing AI on the Web in a direction that benefits more than a few of the already wealthy.
Extending Web Science
Web Science in Europe has begun the task of building up a body of knowledge to address these challenges. (Further information on the Web Science conference, educational programs and summer schools can found at http://www.webscience.org/.) Yet we have more work to do in extending Web Science, both within and beyond the academy. We classify the challenges by considering the interaction between various stakeholders involved, as illustrated in the accompanying figure.
Figure. Web Science methods must remain incomplete, if lacking interaction between scientists (i), or if not involving all of the Web’s stakeholders.
Interdisciplinary methods. To the present day, the vast majority of Web research is disciplinary. Web Science in Europe has been at the forefront of developing interdisciplinary approaches to describing, analyzing and intervening in the Web. Our experience over the past decade shows that working across disciplines brings a depth of analysis and level of confidence in research outcomes that is much needed to address the very real challenges facing the Web—and society—as we move forward into the 21st century. Our experience also allows us to see where we can and should extend Web Science research through the novel application and development of research collaboration.
We are the first to recognize that this is challenging. Academic disciplines work with different objectives and have crafted a range of epistemologies, methodologies, and methods that have distinct professional standards. This is particularly noticeable across the computational and social sciences, where there are some profound differences in what counts as knowledge, science, and method. This is evident in the majority of—otherwise exciting—conferences between the social sciences and computer science, which tend to start from one ‘side’ or the other, and to privilege that body of knowledge, rather than opening it to revision and reconstruction through engagement from beyond.
Web Science has made the case for interdisciplinarity at a high level but transcending these established knowledge frameworks to build new understandings is difficult, demanding creativity, risk taking, and generosity.
One of many examples we may envision is the use of interdisciplinary visual data analytics. Web data offer remarkable potential to analyze the things that people say and do, in real time, over time, rather than the things that they say they do when asked using conventional methods, for example, interviews and surveys.21,26 However, integrating understanding of the data and the computational methods required to interrogate this data with the domain-specific expertise required to address specific questions is challenging.17 Furthermore, developing robust methodological understanding of the data and the effects of applying particular computational methods to this data is, as yet, in its infancy. While the visualization community in computer science harbors a wealth of techniques and tools to interactively explore data and find patterns, joint research work that would give Web scientists the means to ‘interview’ Web data and trace the impact of computational methods on results are lacking. Visualizations approachable and understandable across Web scientist subcommunities might become ‘boundary objects’ enabling different forms of expertise focus on the same phenomenon.
Another example is participatory methods. Much has been said about the ignorance of researchers about what the broad public wants, as well as about the ignorance of the broad public about what the scientists deliver. Let us consider the example of privacy protection. While the public’s insight into understanding implications of privacy issues may have been limited, one might have acknowledged that the public’s attitude toward privacy protection did not only stem from lack of knowledge, but also from some nuanced degrees of willingness to share personal information. Such an ambiguous situation calls out for a two-way, participatory dialogue. Not content with only researching ‘on’ users, Web Science is committed to ensuring that the full range of voices is heard as we build our understanding of the Web and shape its future. Web Science seeks creative ways to build public understanding of the public about the threats, but also take on board, appreciate, and remark upon the personal values and attitudes of people. For instance, moral machines are one example where this is done now.3 We are committed to developing participatory methods that allow us to build insight to diverse perspectives and to build dialogues between these. These methods may include: citizen science—where non-experts are included in a variety of research projects, for example, to study local communitiesj or to contribute subjective, possibly diverging, point of views;1 online methods for deliberation; organizing face-to-face citizens’ assemblies; and the use of AI techniques (for example, for enhancing knowledge and understanding of the Web and extending dialogue). It is a priority for Web Science that we observe these processes in action to inform continuous improvement in public engagement, for the benefit of policy making and, more widely, the engineering of the Web.
A final example concerns how we observe the observers. Powerful corporate or governmental actors may determine the fate of Web users observing what we do22 and suggesting what we might do (or not), for instance, which accommodation to select, which job to apply to, or which person to befriend. Therefore, understanding what these actors do by tracking their activity and evaluating their algorithms has become an important activity. Researchers and NGOs like Algorithmwatchk pursue these tasks asking for data donations or crowdsourcing for getting insight into potentially discriminating or exploitative behavior. In other realms of life, corporate actors need to prove their carefulness by admitting to oversight of governmental agencies. In the Web we still lack such regulations, but the more that such actors become gatekeepers to our life, the less we can just rely on corporate slogans like “Don’t be evil” (originally used in Google’s corporate code of conduct).
Conclusion
The Web has grown from an idea in 1989 to become the largest sociotechnical assemblage in human history in a little under 30 years. It is implicated in the lives, livelihoods, and life chances of over half the world’s population already and connecting many more every day. While Europe embraces the Web and its opportunities for integration—perhaps more than other parts of the world—it discusses its risks of division. Rather than dystopian, and most likely false, predictions, what it needs is a scientific approach to understanding how the Web works and how it affects society. Web Science has been devised as a field to tackle these questions and we have highlighted a few aspects of where and how Web Science should proceed. In particular, computer science must look beyond its pasture and embrace the methodological experience and diversity by a broad set of fields—more than it has done until now. Funding and academic institutions need to welcome and reward such undertaking or it will not succeed.
Acknowledgment. This article benefited immensely from discussions we had with all the other participants at the Dagstuhl seminarl on “10 Years of Web Science: Closing The Loop.” In particular, we want to thank Bettina Berendt, Fabian Gandon, Katharina Kinder-Kurlanda, and Eirini Ntoutsi.
Join the Discussion (0)
Become a Member or Sign In to Post a Comment