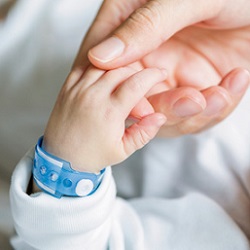
There is a growing consensus among policymakers that bringing high-quality evidence to bear on public policy decisions is essential to supporting the effective and efficient government their constituencies want and need. At the U.S. federal level, this view is reflected in a recent Congressional report by the Commission on Evidence-Based Policymaking, which recommends creating a data infrastructure that enables “a future in which rigorous evidence is created efficiently, as a routine part of government operations, and used to construct effective public policy.”4
Key Insights
- Fact-based policymaking—the practice of using data and research to guide policy decisions—is a promising solution to improving the effectiveness and efficiency of government programs.
- Administrative data can provide new facts to guide policy makers. However, understanding the quality of administrative records, and integrating, transforming, and optimizing them for policy insights present many challenges.
- To overcome these challenges, we developed an integrated database of administrative records from multiple Rhode Island state agencies with more than 800 tables and 2.7 billion records related to more than 4 million anonymous individuals. This data supports econometric and machine-learning research into policies with promise to deliver higher impact per dollar and better serve Rhode Island families.
This article describes a new approach to data infrastructure for fact-based policy, developed through a partnership between our interdisciplinary organization Research Improving People’s Livesa and the State of Rhode Island.13 Together, we constructed RI 360, an anonymized database that integrates administrative records from siloed databases across nearly every Rhode Island state agency. The comprehensive scope of RI 360 has enabled new insights across a wide range of policy areas, and supports ongoing research into improving policies to alleviate poverty and increase economic opportunity for all Rhode Island residents (see the sidebar “Policy Areas in which RI 360 Has Contributed Insights”). Our approach can guide other policymakers and researchers seeking to similarly transform and integrate administrative data to guide and improve policy.
The role of administrative data in policymaking. Administrative data can be collected from the computer systems used by government agencies to run their programs. When transformed into databases that are more suitable for insights, these anonymized records provide new sources of facts for policymakers to benchmark goals and measure the successes and shortcomings of existing and future programs. Often classified as “big data”10 due to their volume, variety, and availability, administrative records are also an increasingly valuable source for empirical social science research.5 Research with administrative records can contribute new data-driven insights to inform important policy decisions (see the side-bar “Recent Data-Driven Insights from Administrative Records”), and add objectivity and scientific rigor to measuring program impact and designing effective program changes. Moreover, scientists can inform how data from administrative systems, which are primarily designed around operational needs and often not suitable for analysis, can be transformed effectively to support research and insights.
Although the idea of guiding policy with data dates back to the 1970s and 1980s, early studies only considered isolated data sources and come from a time when data was scarce. It was not until recently that advances in data collection, storage, and scale provided the opportunity to integrate data across nearly every facet of government. Early case studies and survey studies highlight how the process of data modeling can facilitate negotiation and consensus-building among policymakers,8 but also how the unmet promises of new information technologies prompted frustration among government leaders at that time.9
An important lesson is to engage policymakers and leaders to fully understand their needs, which is why we formed extensive partnerships with state government leaders while building RI 360. Integrated administrative data can support not only academic research, but also the analytics requirements of government itself. Like researchers, government analysts need access to data that has been transformed to provide insights and integrated across programs that serve what are often overlapping populations. For these reasons, RI 360 was selected as the primary data source for the Rhode Island Executive Office of Health and Human Service’s Data Ecosystem project, to empower its data analysts and partners with data optimized for insights.
An example policy for low-birth-weight newborns. Throughout this article, we will describe our process for building RI 360 in the context of a specific policy: determining the optimal weight threshold for providing additional medical care and resources to low-birthweight newborns and their mothers.3 Children born with low birth-weight tend to have more health difficulties and worse outcomes later in life compared to their peers. They also tend to be at higher risk, coming from dis-advantaged backgrounds where mothers are more likely to be teen mothers or have reported alcohol or drug abuse. Programs to support these infants and mothers may increase equity of opportunity and reduce state and federal expenditures for support programs and anti-poverty programs later in life. Currently, the threshold for additional resources is set at 1,500 grams.1 We use this threshold to measure the causal impact of these additional resources to determine if increasing this threshold could be a low-cost, high-return policy change that could improve lives, increase equity of opportunity, and save state and federal funds in the long run.
Using integrated data from RI 360, we can examine a wide range of outcomes, including educational test scores, college enrollment, use of social programs and Medicaid, and maternal care and stress. The data allows for a holistic view of policy impact; measuring gains to education and well-being from the immediate to the longer-term, and also measuring expenditure savings to government-funded social safety-net programs from early-life investments so that government can incorporate concepts of return on investment when considering how to get the most impact per dollar spent.
Our study finds that newborns just below the threshold who receive additional medical care fare significantly better later in life compared to those just above the threshold. Crossing the threshold is associated with increases in standardized test scores in elementary and middle school of 0.34 standard deviations, increases in college enrollment rates by 17.1 percentage points of a base rate of 53.6%, and decreases in social program expenditures of $27,291 by age 10 and $66,997 by age 14. Because the average cost of the additional medical services provided in the hospital at birth is approximately $4,000,1 this study provides new facts to help policymakers evaluate the educational impact and potential financial returns of adjusting the threshold. We conclude that moving the threshold is a potential low-cost, high-impact policy lever for helping children at the margin to achieve better outcomes later in life.
To conduct this comprehensive study of outcomes for low-birthweight newborns, we access data in RI 360 that originates from several Rhode Island agencies. Three decades of birth records from the RI Department of Health define the study population of newborns with low birthweight. The RI Department of Education provides test scores from third-, fifth-, and eighth-grade standardized tests, the PSAT, the SAT, and Advanced Placement exams; records of grade repetition, Individualized Education Programs, and disciplinary actions; and college enrollment records from the National Student Clearinghouse. The RI Department of Human Services provides enrollment and benefit payment records for Supplemental Security Income, the Supplemental Nutrition Assistance Program, Medicaid, and Temporary Assistance for Needy Families. The RI Department of Labor and Training provides quarterly wage records that measure maternal employment rates and earnings following birth. The Centers for Disease Control provide survey responses from the Pregnancy Risk Assessment Monitoring System that measure maternal attitudes and experiences following birth.
Securing the data. Figure 1 summarizes our approach and highlights the first challenge when working with administrative records: deploying security controls that protect the data. Security is our first and foremost concern because the risks of improperly securing administrative data is great. Unauthorized access or data leakage have the potential for invasions of individual’s privacy, identity theft, financial fraud, or even interference with our democratic institutions, including elections. Moreover, irresponsible handling of data can have spillover effects that hinder scientific progress and policy improvement, as data owners perceive great risks of using data and partnering with scientists, even if the uses and partnerships are legitimate and secure.
Figure 1. Overview of the processing steps to secure, integrate, and conduct anonymized research with administrative data.
We mitigate these risks by isolating all data ingest and processing within an encrypted tank (Figure 1a) inside a secure computing environment called a data enclave.16 The enclave’s key features are that it is physically secure and isolated from the Internet, data transfers in and out are restricted and subject to a documented approval process, all access is comprehensively audited, and access is granted to only a limited group of approved researchers. These security controls protect against unauthorized access and ensure researchers access the data in compliance with the data-sharing agreements governing its use.
Our implementation of the data enclave uses a locally hosted system. However, modern cloud computing can help governments implement similar data enclaves using best practices for security and compliance. An additional benefit of a cloud solution is that government can own and operate the enclave, retain possession of the administrative data, and directly manage researchers’ access, which removes the need for data transfers and data sharing agreements.
As an additional security measure, we restrict access to the encrypted tank using a two-party password, known only by senior leadership. A two-party password means two people each know a different half of the password, and both of the senior parties must be present and consent to access the encrypted tank. This ensures no individual researcher can access data that may reveal personally identifiable information.
Once the original data has been successfully transferred into the encrypted tank, we run an automated pipeline to separate out personally identifiable information (Figure 1b). Sensitive identification numbers—such as Social Security numbers or other identifiers deemed sensitive by the agency—are flagged ahead of time and automatically replaced with irreversible hashes, a technique that is widely used for protecting passwords.11 Following this separation, the remaining data contains no personally identifiable information and is de-identified (Figure 1c).
Anonymizing the data. Once the data is secured, the next challenge is developing a method for identifying the same individual across datasets, while also preserving their anonymity so researchers cannot discover their identity, even inadvertently. Although many of the data sources for the birthweight study identify records by Social Security number, an exception is the RI Department of Education, which identifies students by name and an internal identification number. Therefore, we require an automated method to find matches among individual records based on hashed Social Security number when available, or else based on other fields like name and date of birth—all without revealing these fields to the researcher.
Our solution is to assign a global anonymous identifier (Figure 1d) to records right after separating out personally identifiable information. An automated script identifies matches among all hashed social security numbers, phonetic representations of names (using the Soundex algorithm18), and dates of birth. Using the global identifier, we can join information on outcomes to low-birthweight newborns and their parents in the birth records without knowing any personally identifiable information for any of the individuals.
Our deterministic algorithm is designed to minimize false matches (incorrectly matching two different individuals) at the expense of having more missed-matches (in which two records of the same individual are not matched). Some records are missing too many fields and are considered too ambiguous to assign a global identifier, but this occurs for only 3.9% of records. As an alternative to the deterministic approach, the identifier could be constructed with probabilistic record-linkage methods that would likely have fewer missed-matches, but would also carry higher costs for computation and manual curation, as well as a higher likelihood of false-matches.12
Integrated administrative data can support not only academic research, but also the analytics requirements of government itself.
Integrating the data. We receive data extracts from administrative systems in various formats. The raw records used in the birthweight study arrive in the encrypted tank as comma-separated text (with varying delimiters and quoting conventions), fixed-width text, XML, and Excel files. Our approach has been to meet government data partners where they are, and to accommodate data extracts in the format they can most easily produce. Most agencies have perpetual operational demands on their administrative systems, and they are not resourced to support additional development for data warehousing or analytics.
Since there is no universal format or data dictionary across agencies, we normalize the data into a consistent format and typing structure with a lightweight and open source integration tool called Secure Infrastructure for Research with Administrative Data. We developed this tool using an agile approach to meet the evolving needs of researchers and analysts as we built RI 360. Our GitHub repositoryb provides additional technical detail about our integration methods, as well as a worked example based on simulated data.
We chose an Extract Load Transform approach over the more typical Extract Transform Load approach.7 In practice, this means the de-identified data is loaded into RI 360 in as close to its original format as possible. The majority of transformations are added later after researchers have a chance to perform preliminary analyses to assess data quality and understand the data-generating processes underlying the administrative systems.
As an example, birthweight is an essential variable for defining our study population. However, it has been measured in different units (grams and ounces) over the three decades of birth records. Therefore, we construct a birth derived table that normalizes weight, as well as several other categorical variables measured at birth that switch from using numeric to character codes in the records over time. A derived table is a materialized view that aggregates, normalizes, and/or combines data from multiple original tables in RI 360 into a single table that facilitates a specific analysis need—in this case, determining birthweight in a consistent way for all births.
A more complex example in RI 360 is the Supplemental Nutrition Assistance Program-derived table. It combines records on applications, eligibility, benefit payments, and household structure to determine all individuals enrolled in the program at a given month and their household-level benefits.
At the highest level, we roll up all the derived tables into a single RI 360 summary table, which spans 20 years of history for the state’s most important programs and outcomes, as well as demographic information about anonymized individuals (for example, age, race, ethnicity, and sex). Most of the outcomes in the birth-weight study, including educational outcomes and benefit payments, are found in the RI 360 summary table, which reduced the effort needed to launch the study. Creating derived tables also ensures all studies using RI 360 draw from common variable constructions and definitions that are robust and reproducible.
Supporting research integrity. A fundamental requirement of scientific findings is that they can be independently replicated by other investigators.17 Similarly, fact-based policy should be based on robust findings that are peer-reviewed and replicable. To facilitate future replication, we update and snapshot RI 360 approximately three times a year, creating what we call a research version. (Figure 1f). The research versions are de-identified data and become the permanent archive of RI 360. We have generated 11 such versions. Once a research version has been validated, the encrypted original data used to create that version is wiped from the encrypted tank and destroyed. Every analysis is tied to a fixed research version of the database, and can be rerun against the research version at a later time to replicate the results. Additionally, to encourage reproducibility, analysis projects use a common project template to organize code and research results in a standardized way.c
Even through RI 360 has been de-identified, our data-sharing agreements restrict all research with anonymized individual-level records to the data enclave. Only aggregated or statistical results such as summary tables, plots, and regression coefficients can be exported from the enclave. All statistics must be aggregated such that they represent 11 or more distinct individuals. To ensure compliance with these agreements, no individual researcher has the ability to export files from the enclave. Copy and paste functionality has also been disabled within the enclave’s user interface. Exports are subject to review and documentation to ensure exported results conform to usage agreements (Figure 1g), and they trigger real-time alerting to senior leadership. A read-only snapshot of each export is archived in the enclave to facilitate future audits.
Conclusion
The insights gained from research with administrative data have the potential to transform the way policymakers approach some of society’s most important policy decisions. Robust evidence on previous policy outcomes and predictive modeling of future outcomes can guide policymakers to smarter policies with greater benefits at lower cost. We have described a comprehensive approach to overcoming the many challenges faced when integrating siloed statewide databases into a data infrastructure for fact-based policy, which is the first system of its kind in the U.S. In the future, we hope more systems of this kind will provide policymakers at all levels of government—and in many countries across the world—with a rich ecosystem and evidence base for the important decisions they make on behalf of their constituents.
Acknowledgments. This work was supported by the Smith Richardson Foundation and Laura & John Arnold Foundation.
Join the Discussion (0)
Become a Member or Sign In to Post a Comment