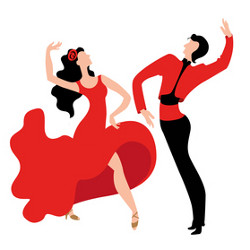
Latin America, with its rich and varied cultural heritage, is a region widely known by its diverse musical rhythms. Indeed, music and dance constitute an important part of Latin American cultural assets and identity.2 Some of these rhythms, although famous worldwide, belong to specific regions; for example, samba is from Brazil, tango is from Argentina, merengue is from the Dominican Republic, corrido is from Mexico and vallenato is from Colombia, among many other examples. Most of them were created by the cultural interaction between people from African, Native American, and European cultures that shared their music and instruments. Those heterogeneous cultural characteristics made these music styles appealing to an international audience.
One of the most well-known Latin musical genres is salsa—a genre considered a fusion between several popular Latin music styles such as son montuno, guaracha, mambo, bolero, and chachacha, to mention a few. However, most of the research on the salsa musical genre is based on ethnomusicology, which has revealed salsa's complex and diverse social and cultural aspects. Indeed, salsa is an amalgamation of Latin traditions and musical styles representative of the Afro-Hispanic culture in the urban-industrial working class. Salsa songs voice concerns of scarcity, violence, inequality, and desperation, which are musically translated using violent orchestration.3
Computational research on intangible cultural heritage contributes to the legitimation of local music as a valuable part of the identity of a region.
From a computational perspective, there is an interdisciplinary research field called music information retrieval (MIR) that seeks to develop computational data search and retrieval techniques applied to music. MIR combines various engineering techniques such as signal processing, informatics, machine learning, and computational intelligence with the results of careful experimentation in psychoacoustic and musicology research. MIR focuses on developing a variety of efficient computational algorithms; for example, for genre or instrument recognition, for temporal pattern detection, or even for song structure detection and composition. These state-of-the-art algorithms enable the discovery, organization, and monetization of continuously evolving and growing media collections.
However, most of the research has been focused on popular western music such as pop, rock, and classical music, which usually follow simple and regular melodic and temporal patterns. Unfortunately, ethnic and folk non-western music from Asia, Africa, and Latin America has been underrepresented in the MIR community9 by grouping them into a single category labeled World Music. This particular over-simplification undermines the diversity, richness, and complexity of music in these regions. In particular, the salsa musical genre was not included in rigorous musical research due to two main reasons: the unavailability of salsa musical information on music research datasets and its complex heterogeneity. To address these difficulties, recent MIR research is beginning to include salsa songs in research datasets. In 2008, the Latin Music Database8 did the first attempt by sharing 311 salsa songs. Also, the Proud Music record labela provided 66 entries of salsa songs with features such as tempo, mood, character, instruments, arrangement, and composer.
Latin American researchers created the Salsa Dataset with more than 20,000 salsa songs, containing metadata and acoustic features, including the analysis of the entire wave for each song.
Nearly a decade later, Latin American researchers created the Salsa Dataset6,7 with more than 20,000 salsa songs, containing metadata and acoustic features, including the analysis of the entire wave for each song. Later, the dataset was modified to contain only 10 seconds of each song in order to improve its share-ability. Those 10 seconds corresponded to the most representative part of the song, which in salsa is the chorus. To find the chorus of each song, a chorus extraction algorithm that follows a sequence alignment process was used.1 This type of algorithm is widely used in bioinformatics to find repeated chains of amino acids. In this sense, by replacing the nomenclature of DNA ACTG (C: cytosine, G: guanine, A: adenine and T: thymine) with the 12 musical notes (C, C#, D, D#, E, F, F#, G, G#, A, A#, B), the sequence alignment algorithm could search similarities between several parts of the song until the chorus was found. This approach achieved a 74% accuracy in chorus detection and revealed that the mambo (the instrumental part of a salsa song) has a similar behavior with respect to the chorus.1
The Salsa Dataset was further modified by conducting an analysis to understand the topology of the songs.6 The analysis, performed using unsupervised machine learning techniques such as k-means and self-organizing maps, yielded unsatisfactory results, meaning that the dataset needed an extension that included annotations. The annotations were done manually by an expert in the salsa genre. The expert rated nearly 10,000 10-second audio fragments by associating the audio with its ability to enable dancing the way salsa songs are danced. This rating resulted in a percentage of salsa for each song fragment. Additionally, the expert identified different genres in the audio fragment (from a list of 40 musical genres and subgenres typically related to salsa, such as salsa itself, guaracha, son Cubano, bolero son, bolero tradicional, son montuno, pachanga, merengue and guajira). By combining these multi-labeled annotations with the acoustic features of the song, the Salsa Dataset can provide a complex testing ground for supervised machine learning algorithms.6
Besides music classification, another main task in MIR is the automatic composition of music. A formal system based on probabilistic parsers5 automatically generated salsa music inspired by songs from the Grupo Niche,4 a famous Colombian salsa band. A Web-based tool to visualize and listen to the possible outcomes of the model was also implemented. This tool allowed the recognition of the patterns used by the band in their songs with the bass and piano scores of the generated song.
Computational research on intangible cultural heritage contributes to the legitimation of local music as a valuable part of the identity of a region. Furthermore, the proliferation of audio streaming platforms has encouraged studies concerning the automatic classification of musical genres and the successful development of recommender systems for a global audience. The inclusion of the salsa musical genre on the MIR research community represents an opportunity for Latin American researchers to showcase their culture using computational techniques and formal models.
Join the Discussion (0)
Become a Member or Sign In to Post a Comment