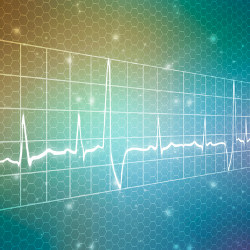
There is demonstrable appeal in consumer-wearable devices like activity trackers, having now been used by approximately 10% of American adults to track measures of their fitness or well-being.4 Because activity trackers are most commonly used for motivating a change in behavior toward modest personal fitness goals or healthy activity levels over time,8 it is easy to forget they are also used to inform more critical decision making and serious investigations of self, including tracking ongoing health conditions and disease progression;24 tracking mood, with potential implications for mental-health treatment;4 and self-diagnosing problems involving health or other concerns.22
Key Insights
- Unlike popular use scenarios involving today’s consumers, more ambitious future use of health wearable data can be expected to involve even less tolerance for data uncertainty.
- Here, we explore the effect of uncertainty on several forward-looking scenarios in which health-wearable data is used to guide critical health decision making.
- Areas in need of further research include how to provide users access to confirmatory evidence of reliability, preserve provenance of uncertainties, and tailor communication about uncertainties to users.
These popular uses expose the potential variability of “uncertainty tolerance” among multiple users.12 Those undertaking a serious investigation of self require a certain level of precision and data accuracy, as well as details regarding correlations between variables, whereas salient information for those with a casual interest in their fitness may simply want to know whether they have met some target or are generally improving over time. Technological advances, both recent and on the horizon for health wearables, are predicted by some experts to enable breakthroughs in disease prevention, prediction, and management, areas for which uncertainty tolerance differs significantly from that of the wearable consumer.10 In addition to existing health wearables that claim to measure blood pressure, breathing rate, and mood or emotions and stress through galvanic skin response, wearables may soon be able to measure or infer health indicators like blood glucose, calories consumed, hydration, and heart strain (for details, see https://www.wareable.com/fitness-trackers).
Here, we explore the implications of, and difficulties in designing for, uncertainties regarding health wearables. We begin with the relatively minimal negative impact of uncertainty in current consumer uses of these gadgets as a way to demonstrate the known-but-as-yet-unresolved challenges in communicating health data to users. We next argue that seemingly innocuous uncertainties emerging in the present use of wearables need attending to, as they are likely to produce important consequences in the future. We raise three concerns in particular: First, advances in wearable technology will enable measurement of physiological data of which the user has little or no access to verifiable evidence (see the section in this article on emergency medical intervention and disease prevention). Second, low-level uncertainties are compounded by the interdependency between various data systems and their implications (such as for disease prevention, prediction, and management) (see the section on life coaching). And third, near-future scenarios involving external use of personal health data introduce new stakeholders whose tolerance for and ability to understand uncertainties will vary, requiring deeper research into ways to deal with uncertainties (see the section on patient compliance monitoring).
Known Uncertainties of Consumer Wearables
For this purpose, we use the term “uncertainty” to mean a lack of understanding about the reliability of a particular input, output, or function of a system that could affect its trustworthiness. With wearable activity trackers, uncertainties arise in various forms and affect user trust to varying degrees. The consequences, while not always apparent to the user, also differ. Here, we explore some of the salient uncertainties that will be relevant to the discussion later in the article.
The old engineering principle says, “garbage in, garbage out,” but it can be difficult to know whether the data coming into a system is sufficiently accurate to produce meaningful output—where “meaningful” is defined in relation to the user’s needs; we call it “input uncertainty.” Inaccuracies in data can be introduced by wearable users in various ways. For example, diagnostic tracking,20 may require users to manually record instances of symptoms, food they have eaten, or medications they have taken. In such cases, the reliability of system outputs depends on users’ ability to correctly infer what data their tracker is capable of automatically collecting23 and their vigilance in manually collecting the rest, as well as the degree they are able to understand the standards for entering data and the importance of the precision of their input. Users often lack knowledge of how algorithms process their data and may thus fail to appreciate how imprecision in a single input could affect the overall system’s ability to make appropriate recommendations. Supporting users’ understanding of these impacts is difficult,18 as few people have the requisite knowledge or interest in interrogating an algorithm. However, we suggest that supporting understanding and reducing input inaccuracies may be helped by following three practical guidelines: enable users to engage in a trial-interaction phase, where they are able to play around with different inputs to see the effects on calculated outputs; provide simple tips on the inputs that explain data-collection standards and the importance of precision; and/or provide some window into the underlying model and calculations.
Input uncertainties also arise through onboard sensors. Notably, while guidelines for effective sensor placement are typically provided to users, user estimation of sensor accuracy is not. The reliability of fitness-tracker data has long been a source of concern in human-computer Interaction (HCI), and comparative evaluations of activity tracker brands reveal minimal though potentially significant differences in reliability.3 While users of these tools are highly cognizant of their lack of reliability (such as with step counting6 and sleep monitoring16), attempts to test devices for inaccuracies and calibrate use accordingly often fail.18 Prevailing advice from designers is to enable users to annotate or amend their data if deemed inaccurate,6,20 but users’ ability to correct sensor errors is limited only to readings they are able to verify independently. As wearables begin to measure physiological data (such as heart strain) not otherwise accessible to the user, new design solutions will be needed to address input uncertainties.
Another type of uncertainty we call “output uncertainty” is apparent when users are unable to determine the significance of the inferences or recommendations produced by a system (see the sidebar “Understanding Health Wearables Data”). For example, many users of activity trackers struggle to understand how they compare with others (such as whether their readings are normal, exceptional, or worrying)16 or whether they can claim to be “fit.”14 Even if users are able to determine their readings are outside what would be considered by medical doctors in the normal range, they routinely ask for guidance about what to do with the information.14,15 Current tools do not provide the support users need to understand the significance of their data3 and without it cannot determine the significance of uncertainties in that data.
While some evidence suggests providing users information about why a system behaved a certain way can increase trust17 and not doing so (such as not providing uncertainty information) can lead to reduced trust,11 a recent study found algorithm and system transparency does not necessarily yield more trust21 and greater intelligibility tends to reduce trust when there are significant output uncertainties.17 These points suggest questions that deserve further research; for example, when—or indeed for what users—is it appropriate to communicate how the systems collect and process data and how confident the systems are in their outputs? And, moreover, how should these uncertainties be communicated to maximize user trust?
A final notable concern is what we call “functional uncertainty” that emerges when users are unable to understand how, why, and by whom their data is being used. Concerns about privacy and security are manifestations of this uncertainty. It is not always apparent to users exactly what data is being collected from their devices, as well as the duration, location, or security level of their storage. For example, Epstein et al.7 found that nearly half of the participants in their study turned off location tracking, fearing friends might be able to see where they were at all times or their location information might be sold to companies to better target ads. In certain contexts, a lack of location information might reduce the precision of other calculated metrics that depend on it. Further, consent terms and conditions being notoriously verbose and inaccessible, consumers may not fully understand the implications of the consent given when signing up with their devices.1 This, in turn, can influence user compliance with recommended usage, introducing further input uncertainties.
We argue that for general fitness and well-being, the effect of the uncertainties we have just outlined are limited. They may contribute to loss of trust and high rates of device abandonment,5 but while these consequences may be a concern for companies producing the gadgets, it is not especially problematic otherwise. However, our interest throughout the rest of this article is how the effect of these uncertainties could intensify in more ambitious uses of health-wearables data.
Uncertainties in Future Uses
Here, we introduce three areas where we anticipate increased use of commercial activity-tracker data and explore how they may further affect uncertainty tolerance and thus implications in designing for uncertainty. We focus on these scenarios as a way to draw out three distinct concerns that require attending to in future research:
Emergency medical intervention and disease prevention. Health wearables allow users to make sense of past events—what activities they have done and what effect they are likely to have on their well-being—to prompt positive behavior change, as discussed by Fritz et al.8 The next stage of development might be for health wearables to predict health crises; examples include alerting a hospital of early signs of a heart attack or warning users of how likely it is they will develop, say, breast cancer.
The scenario involving predictive emergency medical intervention raises the question of who ought to have access to personal health data. While it would be helpful to link one’s health data directly to the closest hospital in order to set the long chain of care in motion as early as possible in an emergency, there would be highly sensible consumer pushback around the access various parties might want to have to personal health data and that functional uncertainty in this arena would likely not be tolerated. Alternatively, if a wearable device alerted a user to hurry to a hospital at the start of a possible medical crisis, how certain does the device have to be? Should gadgets err on the side of caution, possibly provoking a false alarm? While not alerting a user due to insufficient certainty may lead to preventable deaths, so might causing alarm when alarm is not absolutely necessary, thus leading users to ignore or even reject subsequent alerts, with the gadget turning the user into “the boy who cried wolf.”
The very notion of a health wearable alerting a user to an otherwise imperceptible impending crisis demonstrates the insufficiency of solutions for addressing uncertainty that rely on manual data correction by the user, as suggested by Consolvo et al.6 and Packer et al.20 Explaining the data collected and the ways it is processed by the algorithm may be more appropriate for assisting a user determining whether the device output is certain enough to warrant seeking medical attention. At the same time, this information must be delivered in ways that can be evaluated rationally by a person who just received an anxiety-provoking output (see the sidebar “Communicating Uncertainty”). Both parts of this solution are non-trivial and require further research.
Life coaching. Tracking data points through one’s personal history is of limited value for individuals seeking improvements in and maintenance of their well-being, in contrast to information about dependencies and correlations among multiple variables5 (such as the effect of certain foods on an individual’s blood sugars). Given that users are often not rational data scientists22 and consistent in asking for greater analytical capabilities than their devices are capable of providing, it seems inevitable that device manufacturers will introduce systems that purport to provide more definitive answers for users. The danger would be doing so without properly attending to the uncertainties highlighted earlier.
Users’ inability to appreciate uncertainty is made especially clear in the case of wearables that claim to identify correlations between mood and activities (such as ZENTA, https://www.indiegogo.com/projects/zenta-stress-emotion-management-on-your-wrist). It is conceivable that wearable life coaches may soon draw from other pervasive technologies to provide indications of, say, toxic relationships between the user and other individuals and encouraging them to cut unhealthy social ties. While such revelations could have benefits, the implications of inaccuracies of one’s data or of the data being drawn from other sources to determine correlations would begin to extend beyond the individual user, affecting others in the user’s social circle who did not necessarily consent to such analysis. Additionally, the consequences to individuals deciding to cut a person out of their lives are not necessarily knowable to a system (such as how cutting ties might introduce undue financial instability into their lives). How certain would one have to be of the toxicity of a relationship to be willing to end it? It might indeed be the case that people would more readily accept diagnoses of their problems in the form of a scapegoat than that their unhappiness is a result of their own behaviors they find difficult to change. This is all the more reason why tools that claim deep insight into users’ lives must be very clear about the uncertainties they are juggling in their algorithms.
For advanced diagnostic tracking in the form of life coaching, new techniques are needed to identify potential triggers from relevant contextual information; and to the extent that doing so entails drawing data from other pervasive devices, such a filter might introduce further uncertainties that need to be reflected in overall measures of uncertainty. Additional research is needed to understand how best to communicate these uncertainties to users. In particular, tools are needed for capturing users’ cognitive and affective responses to these uncertainties (as covered in the sidebar “Communicating Uncertainty”) and for capturing information regarding subsequent actions taken by users in order to improve uncertainty feedback visualizations and interfaces, as in Morris and Klentz11 and Kay et al.12
Patient compliance monitoring. It has been argued by some technology experts that the commercial appeal of activity trackers for relatively affluent and active individuals has obscured the true potential of the devices for helping manage chronic illnesses—given that those with a true health need are significantly less likely to abandon their gadgets when the novelty has worn off.10 If the degree of certainty in the reliability of activity-tracking data were to be better understood, such devices might be more readily accepted in the doctor’s office as a way of inferring compliance with exercise plans and dietary advice, as discussed by Swan.24 With this end in mind, we anticipate commercial wearables will advance to the point of being able to determine whether and when a patient is taking prescribed medication and at what dosage; the effects of such medication on their physiologies; and what other behavioral factors might be affecting symptoms.
Doctors could thus disambiguate factors that are affecting a patient’s health. This is important information for determining the accuracy of patient self-reports, which can be flawed for any number of reasons, ranging from innocuous memory failings to subjective interpretation of one’s experiences to intentional misrepresentation or deception. To the extent that patients understand noncompliance is detectable by their doctors, this may indeed promote greater compliance. On the other hand, the use of wearables as an objective (certain) measure may result in greater emphasis being placed on quantitative data than on the patient’s own anecdotal reports. Inconsistencies between the two accounts that arise as a result of uncertainties surrounding the wearable data or input uncertainties relating to sensor-error rates and the device having been used incorrectly by the patient could have negative implications for the dynamic of patient-doctor trust if the uncertainties are not clarified by both parties.
Seemingly innocuous uncertainties emerging in the present use of wearables need attending to, as they are likely to produce important consequences in the future.
Just as it is not always clear how to communicate uncertainties to the average consumer, it is also not clear how to communicate uncertainties to doctors. Effective communication of uncertainties may take different forms between these two groups. For example, doctors may be more comfortable interpreting raw data or graphs or need data in a certain form to be compatible and comparable with their existing patient records. It may take new training to be able to interpret results from commercial wearables within the standard assessment frameworks (these practices may need to evolve), as well as further training for dealing with patients who may have drawn their own (possibly false or irrelevant11) conclusions from their personal devices.
Entering the consumer health-wearables market also raises potential ethical questions, including whether patients want their doctors to know everything they do. If not, research is needed to determine how to strike the appropriate balance in data that supports serious medical decision making while preserving plausible deniability for the patient.
Conclusion
Future research is needed to address these questions and the trade-offs they imply:
How to provide access to confirmatory evidence of reliability. An inherent problem of many pervasive sensor technologies is the data recipient (whether doctor or patient) has little or no way to verify the data’s accuracy.13 In the case of health wearables, users might have some general sense of whether they are, say, dehydrated or have low blood sugar but are unlikely to be able to put an exact number on the measurements. So how might future health wearables provide access to confirmatory evidence of their precision? Doing so would be especially useful for enabling users to help with device calibration, as discussed by Mackinley,18 to help mitigate at least some potential input uncertainties.
How to preserve provenance of uncertainties. Due to the trend toward greater interdependence between data systems, with outputs from one system being churned through the algorithms of others,13 it is conceivable that data from individuals’ self-tracking devices will be used as bedrock data in other systems from which a range of inferences are made. Ensuring uncertainties are preserved and communicated throughout a long chain of systems whose developers and interpreters might have different readings of these uncertainties and tolerances for them is challenging but necessary if the systems are to be interpretable at scale, as discussed by Meyer et al.19 This requires development of mechanisms for ensuring important context is not lost, including, say, both the uncertainties and uncertainty tolerances at different points along the chain.
How to tailor communication of uncertainties. Designs must be flexible and/or customizable, presenting uncertainty information in ways that are understandable by the full range of end users with differing needs in data granularity and information presentation. Given that much of the value of health wearables for lay consumers comes from data being available at-a-glance, there is a need to balance important nuance with the interface usability, as discussed by Liu et al.16 Still, there are moments when even lay consumers could require access to uncertainty information, with systems perhaps allowing them to delve more deeply, as required. Contextual information (such as users’ intended and ongoing use of their wearable data) might also be useful for determining what kinds of uncertainty information the system ought to communicate. At the same time, designers must be cognizant of user variability in cognitive and affective responses to uncertainty-related information to design systems that can identify, learn from, and adapt to these responses to inform health-related decision making most effectively.
These design implications can be considered an open challenge to the health-wearables community without suggesting precise mechanisms for realizing them through design.
Acknowledgments
This article resulted from group work that was part of the CHI 2017 workshop “Designing for Uncertainty in HCI: When Does Uncertainty Help?”; http://visualization.ischool.uw.edu/hci_uncertainty/
We wish to thank our fellow workshop participants and keynote speaker, Susan Joslyn, for their feedback in developing these ideas. And in particular we thank the workshop’s organizers—Miriam Greis, Jessica Hullman, Michael Correll, Matthew Kay, and Orit Shaer—for providing a forum for discussing these ideas and bringing this team of authors together for ongoing collaboration post workshop.
Finally, we also thank the anonymous reviewers for their help shaping and improving this article.
Join the Discussion (0)
Become a Member or Sign In to Post a Comment