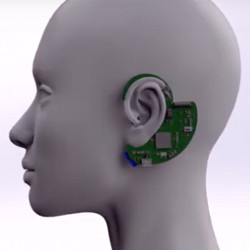
Imagine, for a second, that you suffer from hypertension (high blood pressure), diabetes, have a family history of heart disease, or have long battled with asthma. Unfortunately, most of you won't have to imagine this; according to the World Health Organization, over two billion people worldwide live with one of these chronic health conditions, so likely you or a close family member are afflicted. Treating these conditions requires frequent doctor visits and physical checks, both in and out of the clinic. Careful health maintenance and preventive care can lead to a happy and long life but is a lifelong burden on the patient and the doctor. Without it, however, these conditions can be deadly.
Why do they become deadly? The key problem, even in developed, rich countries, is access to continuous care; this can happen in a variety of ways, from inequitable healthcare systems, lack of trained professionals, distance from hospitals, lack of access to data, or patient non-adherence. These problems are now compounded by the COVID-19 pandemic, which has overloaded hospital systems and reduced health infrastructure's ability to prioritize preventive care. The bitter pill here is that preventive care is the best way to reduce this load long term, but it is just not feasible with available resources and exhausted clinicians.
For the past three decades, the computing community has asked if it can fill this preventive care gap. eBP—the automated blood pressure wearable system described in the following paper—is a sterling example of the third wave of mobile health tech to fill this gap. Where did it start? Well, in the 1960s, "Star Trek" showed the tricorder: a device that senses bio-signals, records them, and computes to prescribe treatment. In the 1980s and 1990s, before smartwatches, FitBits, or even iPods—researchers were building wearable prototypes enabling simple interaction with users and sensing of a few things like motion. Smart clothes, headbands, wristwatches, that sense, record, and compute like the tricorder. These were clunky—with poor battery life and limited ability—but proved the concept of mobile health and sparked the field. In the 2000s, everyone had a smartphone in their pocket, and the next wave of mobile health leveraged these modern versions of the "Star Trek" tricorder. Because of the phone, these mobile health approaches were the first to have broad usage. Researchers used the phone's camera, motion sensor, and significant computational ability to do things like screen for anemia and measure heart rate.
For the past decade, and especially in the last five years, the third wave of mobile health has been in full force. Taking advantage of the increases in computational power, accuracy, and efficiency of modern MEMS sensors, and major strides in applying machine learning and signal processing to biological data, a new class of wearable has emerged. These are unobtrusive and highly sophisticated devices that enable sensing of things we used to think were only possible in "Star Trek" and science fiction. More than just a FitBit, these devices have allowed us to identify and understand complex health-related human activities like eating, smoking, and sleeping, as well as accurately measure novel health markers like stress, respiration, pain, glucose levels—and in the case of eBP—blood pressure.
eBP and the third wave of mobile health devices will eventually replace expensive clinical machines without giving up accuracy.
Blood pressure is an important health marker for a variety of conditions. Accurate measurement requires a cuff which compresses the artery on your arm for a pulse signal. eBP supports continuous blood pressure measurement with clinical accuracy, outside the clinic. It is a small device that sits in and around a person's ear. A balloon inside the ear is automatically inflated to contact the artery in the ear, then a sensor on the balloon touching the skin can gather a weak signal. I greatly simplify here, but I don't want to spoil the fun! The paper details the significant challenges in making this system work, from weak and noisy signals, uneven contact, biocompatibility, battery lifetime, and user burden.
eBP is a wonderful example of this third wave of mobile health in two ways. First, the system achieves technical and computational sophistication within the constraints of modern medicine and telehealth. We can learn a lot from eBP on designing resource constrained computers. Second, the project leverages partnerships across health and computing disciplines, giving a laser focus on the actual needs of people, and a deep understanding of the issues that arise when trying to deploy health monitoring devices.
eBP and this third wave of mobile health devices will eventually replace expensive clinical machines without giving up accuracy. Soon these devices will provide automated interventions, suggesting exercises, dietary change, or mindfulness sessions. Preventive healthcare assisted by low-cost wearables will allow our medical workers to move from crisis care to long-term, data-driven health maintenance of their patients.
Join the Discussion (0)
Become a Member or Sign In to Post a Comment