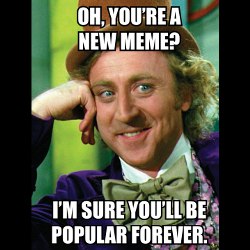
A meme is a concept introduced by Dawkins12 as an equivalent in cultural studies of a gene in biology. A meme is a cultural unit, perhaps a joke, musical tune, or behavior, that can replicate in people’s minds, spreading from person to person. During the replication process, memes can mutate and compete with each other for attention, because people’s consciousness has finite capacity. Meme viral spreading causes behavioral change, for the better, as when, say, the “ALS Bucket Challenge” meme caused a cascade of humanitarian donations,a and for the worse, as when researchers proved obesity7 and smoking8 are socially transmittable diseases. A better theory of meme spreading could help prevent an outbreak of bad behaviors and favor positive ones.
Key Insights
- We test the hypothesis that protomemes in social media are less likely to be popular when they are too similar to other protomemes in a large, variegated dataset.
- We calculate the canonicity of each specific use of a protomeme, or how typical or common is its use.
- We show that canonicity has a non-linear relationship with protomeme popularity, increasing the similarity between protomemes and genes, in that not all mutations are beneficial for protomemes.
Studying memes in general is difficult because detecting and measuring them objectively is difficult. The inception of the web 30 years ago made it easier to focus on a subtype of meme—the one shared through social media. Researchers have since focused on the effect of timing, social networks, and limited user attention rather than on meme content.6,15,23 Being timely and shared by actors in key positions of a social network explains a large portion of a meme’s virality. While these factors can help explain the meme ecosystem at large, both are exogenous to the meme itself. Even if endogenous meme characteristics have less compelling predictive power over meme popularity, it is still important to understand their relationship with virality, as such understanding can be applied case by case to specific memes, rather than produce only a description of the general mechanics of the overall system. For example, we11 showed that success eschews similarity; successful memes are generally found at the periphery of meme-similarity research. The more a meme is imitated, the less the original meme (and all its imitations) will be successful in going viral in the future.
Here, we focus on protomemes, or all catchphrases used frequently on social media that may or may not end up being adopted as memes. We aim to extend our earlier work11 by expanding the scope of the dissimilarity-driven success theory; providing new evidence of the effects of the theory in a dynamic environment; and introducing canonicity to evaluate protomeme content.
First, we test the dissimilarity-driven success theory on a larger data source and on shifting scholarly attention from memes to protomemes. The original work11 focused on a small site and very specific subtype of Internet meme. Here, we focus on Reddit and Hacker News, two online social media websites, and consider any kind of protomeme in the form of frequent and regularly used n-grams that can be shared on these websites, rather than limit ourselves to the image-macro meme subtype studied previously.11
Second, we test the effect of popularity spikes on the future popularity of a protomeme, following Weng et al.,21 as we did not study spikes in the earlier work.11 When a protomeme suddenly becomes very popular, or when it places highly in a ranking of user appreciation, many participants in social media use it in their own posts. The increased number of posts that include the protomeme will make it more similar to the average post of the day. As a consequence, as reported by Lakkaraju et al.,17 its expected popularity will decrease. The new posts that include the popular protomeme are then poorly ranked, as they are stealing each other’s chance for success.
Lastly, we introduce a measure of “canonicity,” or capturing the amount of change introduced by a post compared to previous posts with the same protomeme, showing that the more different a post is from the canonical usage of a popular protomeme, the greater its odds of going viral. However, the effect of canonicity is not linear. High canonicity lowers the overall success of viral posts at the same time it helps non-viral posts be appreciated; that is, we correct the earlier work,11 arguing here that there is a non-linear relationship between canonicity and meme propagation. This is why other research did not find content to be a powerful predictor of success; while true that success eschews similarity, it is not true that with dissimilarity comes success. One explanation might be that protomemes follow the same dynamics as genes. Not all mutations are beneficial; some are irrelevant and some harmful. Future research will test this theory.
Related Approaches
Here, we follow the rich literature studying memes as they spread through the web. In our previous work,11 we observed traditional meme dynamics (such as competition and collaboration in the context of the web10) and provided evidence for the theory that similarity with existing content penalizes a meme’s odds of viral success.11 The origin of this line of research is due to questions raised in the broad distribution of meme popularity observed multiple times in different contexts,15,23 consistent with the dynamics of fads.2
Many research projects have sought to model and predict meme success, and we are unable to mention them all here. The most successful research track focuses on the relationship between memes and the social media through which they spread. For example, social media researchers can predict how wide the cascade spread of a meme will be by observing its temporal and structural features; for example, a cascade’s initial breadth, rather than its depth, is a better predictor of larger cascades.6 The community structure of a social network, or its tendency to form densely connected groups, influences the dynamics of news and meme spread.19,24
The general conclusion of other work is that content is a secondary explanatory factor for meme propagation. However, none of them has effectively ruled out meme characteristics as partial explanation for their success. Further, it is interested in using networks to explain the shape of popularity distributions, not what makes individual memes more or less fit to go viral, which was the focus of our earlier work,11 as well as of this article. Different hashtags in Twitter reflect different degrees of persistence, showing evidence that meme propagation is a complex form of contagion, rather than a simple epidemic.20 Beyond computer science, social science researchers have found links between a meme’s content and its virality. Emotionally positive content is more viral than negative content, although valence alone is just one of many factors driving propagation.3 All such studies inevitably face the challenges of complex spread dynamics, as content that eventually becomes popular might be over-looked when it first appears.14
Our research perspective focuses on the role of mutation and innovation in meme usage and their relationship with success spikes;21 for example, Adamic et al.1 explored an example showing the dynamics of meme mutation. Some results in the literature support the role of novelty in content diffusion,5 while others questioned this result,16 though in neither case was “novelty” strictly defined. Here, we build on the evidence of a negative relationship between title similarity and success on Reddit.17 The difference is that Lakkaraju et al.17 considered how similar an instance of a meme is to the past submission history of the thematic community in which it was shared. By introducing the measure of canonicity, we focus here instead on the similarity of the meme instantiation with the meme’s own submission history, regardless of the context in which it was previously observed. Canonicity is closely related to the Newsjunkie framework,13 which is based on an information-theoretic background. However, Newsjunkie was developed with a different application—ranking novelty of full-text news articles significantly longer than Reddit and Hacker News post titles. For this reason, Newsjunkie is not applicable to this research scenario concerning novelty.
Protomemes
We now reflect on data collected by Weninger et al.25 from Reddit, a social-bookmarking website where users are encouraged to post interesting content. Every post can be upvoted (or downvoted) if the user likes (or dislikes) it. The upvote/downvote ratio is used to highlight high-quality content. In addition, there is a time discount; that is, no matter how many upvotes a post manages to attract, it cannot be highly visible forever. The most popular (highest upvote/downvote ratio) posts appear on Reddit’s “front page,” giving them a further boost in visibility. By default, the front page hosts 25 posts. Each entry in the dataset we studied consisted of a post, its title, and its number of upvotes/downvotes that were combined in a post score by Reddit’s sorting algorithm. Note our research can observe only the final score of a post, not its full upvote timeline. This might introduce bias when looking to establish whether or not the post hit the front page. We assume the final post score is highly correlated with the post score on its first day of life. We base this assumption on the fact that the vast majority of upvotes come within 24 hours following post submission.
Note the terms “score” and “popularity” are not interchangeable, as they refer to related but different concepts. Score is the one-off measurement of a single instance of a protomeme in a day, and popularity is the overall success of all instances of all memes over a longer period of time.
All 22,329,506 posts added to Reddit from April 5, 2012 to April 26, 2013 were part of the dataset. To cross-test our results, we also used a similar dataset from Hacker News, which uses the same dynamics as Reddit though focuses on a more specialized technical audience and has a much smaller user base. The Hacker News dataset included 1,194,436 posts from January 7, 2010 to May 29, 2014.
Here, we consider protomemes, or a catchphrase with the potential for going viral. Note there are more possible types of memes (such as pictures and videos), but given the nature of the data we limit ourselves here to catchphrases. Catch-phrases were also used as meme proxy in Memetracker18 and Nifty.22 We extracted them using information taken exclusively from the post title.
The more a meme is imitated, the less the original meme (and all its imitations) will be successful in going viral in the future.
We apply our definition by borrowing the bag-of-words methodology from the text-mining literature, meaning a protomeme is seen as a set of at least two “tokens” (also called an “n-gram” with n ≥ 2). A token is a word that is stemmed whereby “stop words” are filtered out and are not tokens. To be classified as a protomeme, an n-gram must have been used frequently and constantly over the observation period. We used the frequent-itemset-mining algorithm Eclat4 to extract the frequent n-grams and discard an n-gram if it has not been used for a certain number of days. We also discarded all n-grams that are proper subsets of another n-gram.
We performed the analysis using different thresholds to ensure independence between parameter choice and results. From most- to least-restrictive threshold choices, we obtained 2,731 to 5,585 protomemes on Reddit and 817 to 2,538 protomemes on Hacker News; the preprocessed data we used is available for result replication.b
Results
We now first show how popularity spikes result in reduced future popularity for a meme, then introduce the concept of “canonicity” and how it can shed light on this phenomenon.
Popularity curse. Common sense tells us that popular ideas are likely to be imitated; a protomeme used in a very popular post today will be used in many posts tomorrow. Such intuition about the demand-supply relation on the web is corroborated by several studies, including Ciampaglia et al.9 However, dissimilarity-driven success theory would predict that flooding a system with imitations of a protomeme will cause the imitations to be less popular. At first glance, such a prediction might seem to find support in two observations: the average score of the posts containing a protomeme is less than expected the day after it experiences a popularity spike (see Figure 1a and Figure 1c), and the number of posts containing that protomeme increases (see Figure 1b and Figure 1d).
Figure 1. Distributions of score and number of posts per day of the observed memes, overall, and focusing on only those created the day after the meme was among the 25 top-scoring posts; included is the average and 95% confidence intervals.
These observations support our theory about viral connections but do not prove it. First, the total score awarded and the average score per post are not constant over time (see the online appendix, dl.acm.org/citation.cfm?doid=3158227&picked=for mats). The lower score might be just a relative change; if, for example, there are fewer upvotes awarded on that particular day, a lower absolute number could still represent an increase in upvote share for the day. Second, each protomeme is characterized by its own expected popularity;
scores cluster around each protomeme’s average. Third, a protomeme’s recent history might explain this variation. If, for example, a protomeme was getting declining scores, we might expect it to get even lower scores after a random popularity spike. Finally, there is a fat tail in score distributions, so the average alone is not meaningful.
To give more solid evidence for the theory about viral connections, we tested the median popularity of a protomeme on a particular day using the following mixed model, or “MED Model”
An observation (EWm,i (pm,i)) is the median popularity (pm,i) of the posts containing protomeme m on day i, or the score of the post. Since post scores are count data and distributed over a skewed distribution, we used a Poisson mixed model. Each observation has weight Wm,i, or the number of posts containing m on day i, thus giving less weight to the (presumably noisy) information on protomemes m that were not used very much on day i.
FPlm,i– 1 registers if protomeme m was on the front page on day i – 1. The parameter l is the number of posts hitting the front page each day. The default front page in Reddit includes 25 posts (30 for Hacker News). So, every day, at least 25 (30) posts hit the front page. However, users can increase the physical length of the front page. Moreover, as the day passes, front-page posts get replaced by other posts. As a result, there is no way to know how many posts hit any particular user’s front page on any given day (see the online appendix). For this reason, we ran the regression multiple times for different l values to test the robustness of our results for different front-page sizes.
E(pm,i-1) denotes the protomeme’s popularity on the day before i, controlling for existing trends; um is a random effect of protomeme we used to control for the fact that different observations can refer to the same protomeme; and ϵm,i is the error term.
Figure 2 outlines our estimates of β1 for different values of l. The effect of being on the front page is negative; the protomeme is expected to have a lower median score than on a business-as-usual day. This expectation confirms the theory of dissimilarity-driven success theory. Higher l values decrease the estimated effect, because we included lower-ranked posts that might not actually have hit the front page (all p-values are significant, with p < 0.001). Focusing on Reddit (see Figure 2a) values fall within the ] –0.1 : –0.02[interval. A value of –0.1 implies a score-reduction factor equal to e–0.1, which is close to 10%. This means that ranking in the top 25 posts on a particular day reduces the next day’s median score of a protomeme by almost 10%. The effect in Hacker News appears to be even stronger.
Figure 2. Evolution of β1 coefficients for increasing l in the model and in its associated null model; thin lines represent 95% confidence intervals.
We obtained these results with fixed frequency thresholds, using Table 1 and Table 2 to show the robustness of the results with different threshold choices. In them, we fix l = 25 for Reddit and l = 30 for Hacker News. For all threshold choices in Reddit and for most threshold choices in Hacker News, the results were consistently negative and significant with our main result: hitting the front page results in lower expected popularity on the following day.
Table 1. Effect on β1 of different threshold options for the Reddit dataset. Each row is a different frequency threshold, or minimum share of posts that must include the protomeme, or 0.004 = 0.4% of posts. Each column is the minimum share of days in which at least one post including the protomeme appeared, or 0.91 = 91% of days. Significant values with p < 0.01 are in bold. The threshold values included in Figure 2a are in italic.
Table 2. Effect on β1 of different threshold choices for the Hacker News dataset. Rows and columns are interpreted, as in Table 1, and significant values with p < 0.01 are highlighted in bold. The threshold values in Figure 2b are in italic. We chose the different threshold values to accommodate the significant difference in size between the two datasets.
One could object to these results using the regression-toward-the-mean argument; that is, once the very visible front-page protomeme instance is copied many times, each copy tends to score approximately the protomeme’s average, which is lower than the spike. But the regression already corrects for this using the protomeme random effects. If the argument would be true, β1 would equal 0. In fact, the average is 0 calculated over 50 null models, where protomeme scores are generated randomly, preserving each protomeme’s average score and standard deviation (see Figure 2a and Figure 2b), disproving the regression-toward-the-mean argument.
To summarize, after hitting the front page, a protomeme will likely be used more frequently—15% more in Reddit, 8% more in Hacker News, as reported in Figure 1b, and Figure 1d. β1 values in the model suggest that posts including this protomeme will likely have a lower score (10% lower in Reddit, 23% lower in Hacker News), confirming the expectation. The effect is significant and independent of the recent overall history of the protomeme, changes in average post score, and front page size.
So, if hitting the front page is bad for subsequent protomeme posts, why does common sense tell us the opposite? We propose that a protomeme appearing on the front page two days in a row is very noticeable, and we just do not realize that, on average, the protomeme is doing poorly. We run the same regression, changing the target variable to the maximum score (MAX Model) instead of the median. In this model, for Reddit, the sign β1 is the opposite of the β1 sign for the MED Model (see the online appendix). If protomeme m hits the front page on day i – 1, the top-scoring post containing protomeme m on day i improves. This does not happen for Hacker News, and our hypothesis is that Hacker News is more resilient to fads, as it is used mostly for professional purposes, rather than humor, as with Reddit.
Hitting the front page is thus associated with a larger number of subsequent posts including protomeme m, which by itself is associated with less expected popularity for the same posts. However, in some scenarios, the best-ranked posts containing protomeme m can still hit the front page more easily than usual. We now turn our attention to this subset of special posts, explaining why they are able to overcome the popularity curse predicted by the theory.
The Canon Effect
Following the argument that success is associated with dissimilarity, we now hypothesize that a post including a widely used protomeme m the day after m hit the front page can still be successful if it is dissimilar from all other posts using m. In this way, the post is able to attract most of the attention network users are directing toward protomeme m.
To test this claim we first need a measure for the uniqueness of a post. In Coscia,11 we proposed a meme similarity measure that cannot be used here because it calculates meme-meme similarity, while here we consider only post-post similarity within the same protomeme. Moreover, our earlier11 measure applies only to a subtype of the memes shared on Reddit. We cannot use the measure developed by Lakkaraju et al.17 because it measures the similarity of a post to the subcommunity it is shared in, ignoring the meme it implements. Here, we focus on Reddit and expect a null result for Hacker News, as we showed it to be less prone to the fad effect.
We introduce the concept of canonicity of a post, measuring how much a post containing a protomeme m differs from the usual usage of m. A post is said to be canonic if it uses m as expected, without introducing elements not strongly associated with m itself. Consider, for example, a post M as a bag of words. Each word μ in the bag of words co-occurs with protomeme m with a given probability πm,μ. If m appears in 100 posts, and in 30 of them the post title also includes μ, then πm,μ = 0.3. The canonicity of M is calculated like this
The formula means the canonicity of a post M is the average probability of its words to appear with the meme it contains. Note some posts contain no other word than the words of the protomeme m itself. For this reason, the formula includes the m words in M. Otherwise, such posts will have Γ(M,m) = 0/0, which is unacceptable. Moreover, posts including only a protomeme’s words must have Γ(M,m) = 1 because they use m in its purest form. Since the protomeme’s μs always appear in posts containing the protomeme itself, their πm,μ always equals 1. Finally, a low canonicity score is obtained when there are many words in the post and they have low πm,μ. If a post includes only one unusual word, its canonicity score is still high, because it is still composed mostly of the protomeme itself.
How canonicity is distributed in Reddit is reported in the online appendix. To test the connection between canonicity and popularity for posts using protomemes appearing on the front page the previous day, we create a rank binary variable φM that records whether or not the post was among the 5% best-scoring posts of the day. This is the target variable of the following logistic regression
This is the φ Model. Given a post M containing protomeme m, the φ Model estimates its probability of experiencing a popularity spike on day i, after m hit the front page on day i – 1. Note the set of posts we include in the model is still dependent on the l parameter. For different l values the set of posts included is different, because, for increasing front page size, more protomemes hit the front page, and more posts on the day after will thus be considered in the model.
Figure 3 reports φ Model’s βs for increasing l. For Reddit (see Figure 3a), β never takes values greater than –0.7, suggesting a noticeable and notable effect: high canonicity halves the odds of being a high-scoring post; for a deeper discussion see the online appendix. As we increase l, the canonicity effect gets weaker and weaker. This is expected, as we are considering the regression posts that might have not hit the front page. All β values in Figure 3a are significant (p < 0.0001). We thus expect a null result in Hacker News, given the result of the MAX Model covered earlier. Indeed, Figure 3b reports the effect of canonicity in Hacker News is zero, as no p-value reported for any l is less than 0.01.
Figure 3. Distribution of the φ Model’s β for varying l; thin lines represent the 95% confidence intervals.
We also run two Poisson mixed models with the same form of the φ Model, with the only difference being the dependent variable (in this case the post score) and the data included in them. In the Zero Model, we consider only the posts for which φMl = 0, while in the One Model we focus on the posts for which φMl = 1. In practice, the φ Model tells us the effect of canonicity on the odds of experiencing two popularity spikes in a row, while the One and Zero models reveal the score effect of canonicity on the posts that did and did not experience two popularity spikes in a row.
In the One Model, β has a negative sign (see Figure 4a); all βs are significant with p < 0.0001. If the φ Model told us that canonicity lowers the odds of experiencing two popularity spikes in a row, the One Model would tell us that if a post can nevertheless overcome those odds, it is additionally penalized with a worse score. In the Zero Model (see Figure 4b), β is positive and significant. For the unsuccessful posts in the Zero Model, canonicity has a positive effect. For robustness, we also ran a negative binomial model, resulting in similar estimates as the Poisson model (see the online appendix).
Figure 4. Distribution of the One Model’s and Zero Model’s β for varying l; thin lines represent the 95% confidence intervals.
The discordance of β signs in the Zero and One models can be interpreted as a similarity between protomeme and gene dynamics. Most mutations are harmful or irrelevant, while also lowering an organism’s fitness. In protomemes, if the change is not judged “suitable” for the protomeme by the user community, it will be selected against and gradually lose relevance. This is one of many possibilities and must be properly tested before it can be considered suitable. We leave such a test for future work. However, this result could explain why meme content is not a promising predictor of meme popularity.6 Since changing meme content can go both ways, increasing or decreasing meme fitness, the effects might cancel out.
Conclusion
We have tested some of the predictions of a theory that claims that meme success eschews similarity, because similar memes interfere with one another and get less attention.11 We tested the theory on Reddit and Hacker News, two popular social-bookmarking websites. Successful posts can hit each site’s highly visible front page and then be copied many times over by people who want to use them to be able to get their own posts to appear on the front page. The expected popularity of these posts should thus decrease. We showed that this is the case, though on Reddit some posts might still experience subsequent popularity spikes; Hacker News appears to be resilient to this phenomenon. We explain this apparent contradiction by showing these posts (with persistent popularity spikes on Reddit) have low canonicity; that is, they are usually dissimilar from the average post containing their protomeme. We showed that canonicity has a nonlinear effect.
These results open the way to future work. First, computational social scientists can now move the theory closer to practice, performing, say, a controlled experiment where they select front-page memes from Reddit and semiautomatically generate imitating posts with varying degrees of canonicity. By releasing the posts on Reddit, they should observe in which cases low-canonicity posts tend to garner more upvotes and in which cases high canonicity is helpful. And second, the theory makes claims that are not in line with another theory of meme popularity—the one giving factors other than meme content greater weight in predicting its success. In Cheng et al.,6 Gleeson et al.,15 and Weng et al.,23 meme content and structure were found to be a weaker explanatory factor for meme popularity. Better predictors are meme timing and the social network position of the meme creators. We thus recommend reuniting the two theories in a unified meme-analysis framework.
Finally, computational social scientists could extend the investigation of memes by studying the effect of negative votes: we expect it will show nontrivial dynamics; a vote, even if negative, still comes from a person paying attention to the concept, though its effect is to prevent other people from seeing it. This information was not available at the time of our study, but Reddit started to provide it in 2017.
Join the Discussion (0)
Become a Member or Sign In to Post a Comment