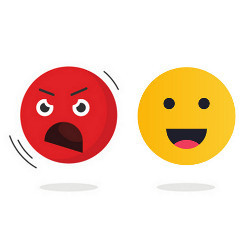
Ishaan and Elizabeth, both graduate students in business, are attending a marketing strategy lecture at a business school in the Northeast. While learning about the principles of market segmentation, Ishaan texts “outdated” followed by three thinking—face emojis to Elizabeth. He wonders how demographic-, geographic-, or psychographic-based segmentation—the topic of the lecture—can help his family’s franchise restaurant deal with the hundreds of sometimes-not-so-positive online reviews and social media posts. Meanwhile, Elizabeth hopes that the fast-food restaurant where she ordered her lunch understands that she now belongs to the segment of ‘extremely displeased’ customers. Earlier, she used the restaurant’s new app to order a burrito without cheese and sour cream, only to discover that the meal included both offending ingredients. Her lunch went straight into the trash can and she angrily tweeted her disappointment to the restaurant. Elizabeth replies to Ishaan’s text, “that is so passé,” followed by a face_with_ rolling_eyes.
Key Insights
- Market segmentation faces important challenges and opportunities, including the impact of big data. This study offers a promising approach to implementing market segmentation using unstructured data, illustrating how firms can develop specific actions or adjust their marketing mix based on insights derived from this type of data.
- Unstructured data segmentation also implies a shift in the current market-segmentation paradigm, as it is based on consumer-generated data. Practitioners do not delineate the variables included in the analysis to perform segmentation. Instead, the analysis is implemented on what matters to consumers.
- The suggested unstructured data segmentation can complement or supplant traditional segmentation approaches, as it provides unique advantages, such as real-time market segmentation.
This simple vignette illustrates an important point. Organizations of every size are challenged with capitalizing on enormous amounts of unstructured organizational data—for instance, from social media posts—particularly for applications such as market segmentation. The purpose of this article is to give the reader an idea of the challenges and opportunities faced by businesses using market segmentation, including the impacts of big data. Our research will demonstrate what market segmentation might look like in the near future, as we also offer a promising approach to implementing market segmentation using unstructured data. With this demonstration, the article also illustrates how firms can develop specific actions or adjust their marketing mix based on unstructured data segmentation.
Big Data and Market Segmentation
Market segmentation plays an essential role in strategic management, grouping customers who share similar preferences, behaviors, or attitudes into segments.32,42 Grouping customers together in a meaningful way allows organizations to more efficiently allocate resources and to strategically focus on fairly homogeneous consumer segments.12,39 Traditional segmentation has relied heavily on costly data collection methods, of which surveys are the most prevalent.15 Aside from having to justify the sometimes high financial costs of data collection, the use of surveys adds at least two more potential problems. First, surveys used in market segmentation projects only capture a snapshot of the market itself, and the dynamism of current markets leads to data quickly becoming obsolete. Second, any survey design issue cannot be fixed once data collection has begun.14 Unstructured data potentially solves some of the limitations of traditional data collection methods in market segmentation but creates a new set of challenges. These challenges may be the root of an emerging, but still very scarce, collection of literature on unstructured data segmentation.
Ahani et al. developed the first study of market segmentation by employing online consumer review numerical ratings,1 while Fresneda et al. implemented the first segmentation study using unstructured data.16 To the best of our knowledge, this is the first study to incorporate different types of unstructured data for market segmentation.4 Adding a second type of unstructured data—emojis—uniquely contributes to obtaining more nuanced and multi-faceted results, as illustrated in the empirical example of the study.
Explaining what constitutes unstructured data is simple: data that cannot be structured for spreadsheet analysis. This type of data can take the form of text, audio, video, images, or even combinations of these types. Emojis are arguably the epitome of unstructured data. The successor of earlier “emoticons,” emojis are graphic representations of facial expressions (such as a smiley_face), animals, objects, etc. that constitute a popular and simple way to express ideas or feelings.21 Emojis can complement or highlight those ideas or feelings, especially for young consumers, as illustrated in the introductory vignette.
Firms already rely on data and analytics to make complex business decisions.42 In this respect, organizations can use unstructured data to overcome many limitations of traditional market-segmentation approaches. Unstructured data accounts for 80% to 90% of the total amount of data available in the digital world, seemingly mitigating the problem of data availability.11 Moreover, many organizations have partially or totally migrated their customer service to social media platforms because of the customer interaction capabilities and flexibility.17 Online reviews are another popular way in which consumers can interact with companies and other consumers. Social media posts and online reviews are freely accessible, which eliminates the cost of data collection. Plus, unstructured data is extremely dynamic, which solves the static nature—the “snapshot issue”—of traditional data collection methods as well.41
Why, then, is unstructured data not used in every market-segmentation project? There are several reasons. Probably the most important is that analyzing unstructured data is difficult, and it is even more challenging to integrate different types of unstructured data in the same analysis—for instance, text and images together, such as “memes,” or emojis embedded in text. Additional obstacles arise due to the nature of the data itself and the selection of the unit of analysis. Employing unstructured data from social media for market segmentation implies using secondary data, which is data that was not generated specifically for the project at hand. No Tweet is posted with the purpose of helping an organization in its effort to segment a market. This lack of specificity can sometimes be problematic for narrowly focused researchers and practitioners, and so is deciding on the unit of analysis.
Traditional segmentation operates at the individual level—customers, potential customers, and so forth—as individuals can be identified in the physical, offline world, though not without some effort. That is not often an easy task in the digital world. Individuals can not only hide behind nicknames or made-up usernames on social media or online reviews, but they can also have different profiles or accounts on the same platform at the same time. This gives rise to murky questions such as: What exactly should be segmented if unstructured data were to be used? What should be the unit of analysis? User profiles on a platform? Individual posts or individual online reviews? Traditional offline segmentation would be inclined to select profiles or any kind of connection with individuals, whatever that means in the digital world. However, this approach would not fully capitalize on the dynamism of unstructured data and that can be illustrated with an example.
Elizabeth, the student previously referred to in the vignette, might be pleased with the fast-food restaurant on Monday night, when she received a coupon (tweet number 1), but entirely dissatisfied by Thursday afternoon, when her order was botched (tweet number 2). Segmenting individual social media posts and subsequently grouping those posts or reviews by user profile can allow managers to track a user’s migration from segment ‘pleased’ to segment ‘displeased’ and act accordingly. The ability to capture this change, or temporal dimension, is one of the major advantages of using unstructured data for market segmentation, but unstructured data segmentation has additional advantages. Organizations can learn a lot from the segments identified by using unstructured data. In social media, online reviews, chats, or blogs, users freely generate content on what really matters to them and not on what market researchers prompt them to answer.6 Nobody asked Ishaan for his opinion about the relevancy of demographic-, geographic-, or psychographic-based segmentation in the introductory vignette. Yet, Ishaan texted, “outdated” and three thinking_face emojis to his classmate Elizabeth.
Noteworthy, much of the unstructured data that can potentially be used for market segmentation is easily accessible or even publicly available. The reader can imagine not only the possibilities, but also the menace of competition accessing many of a company’s customer interactions and, cleverly enough, even competition implementing market segmentation on a company’s own customers. Uncovering which segment is ‘displeased’ with a company—and why—is tempting for any competitor. Remember that in the introductory vignette Elizabeth was ‘displeased’ with the fast-food restaurant because cheese and sour cream were included on her burrito. Thanks to Elizabeth’s tweet number 2, the restaurant knows this, but so does the restaurant’s competition. Furthermore, social media platforms or online review sites are such open public forums that practitioners may observe that extremely unsatisfied customers sometimes try to boycott a company with very negative posts that are often reposted several times (this was observed in the data used in the empirical example shown in the final portion of this article.)
Then, the question that arises is: How can organizations implement market segmentation based on unstructured data? Once the challenges and advantages of employing an organization’s unstructured data for market segmentation are clear, there are several approaches to implement this task. By combining a set of already existing analytics tools into a novel process flow, this study presents our recommended approach for unstructured data segmentation. This ensemble of analytical tools is customized to capitalize on the previously noted advantages of this new type of market segmentation. This article will show the reader what market segmentation might look like, with an empirical example of the application of its methodology on real data.
Implementing Market Segmentation with Unstructured Data
The core type of unstructured data used in this study’s segmentation approach is text, but it also includes embedded emojis. There is a simple reason to choose textual data over other forms of unstructured data: Text content created by customers is often the only data available for market segmentation, and there is no link to other customer information—at least until the customer provides it. This is the case with social media posts, company chats, and, in many cases, online reviews. In a study among 5,000 participants, Microsoft found that 34% of consumers worldwide and 28% of consumers in the U.S. use social media for customer service assistance.29 The popularity of digitally based customer support services, such as social media, continues to increase.
The suggested approach to market segmentation using textual data employs two primary tools—topic modeling and cluster analysis—and two secondary tools—sentiment analysis and emoji detection. The analysis uses “documents,” the text analysis terminology which refers to the unit of text analysis. Documents can refer to an individual social media post, an online review, or even the specific sentences or paragraphs that make up these posts. In the empirical example presented in this article, documents refer to individual tweets on the Twitter platform. The process flow in Figure 1 shows the automatic steps that can be taken for real-time market segmentation.
Focusing on the process depicted in Figure 1, the first step is to collect the data and “clean” it. Standard text cleansing and preprocessing includes operations such as removing words that carry little meaning, like articles; removing numbers and punctuation; and transforming all text to lowercase characters. The main purpose of pre-processing textual data is to ensure the analysis can focus on the most relevant and meaningful words so that computing resources will not be wasted. (Interested readers can learn more about the process in ‘Text Preparation’ in Anandarajan et al.2)
Cleansed textual data is the input to a sentiment analysis, which generates a score for each of the documents in the data related to its sentiment polarity. Documents that contain many negative words—such as “hate” or “dislike”—yield negative scores in the analysis while documents that contain positive words—such as “love” or “like”—yield positive scores. A third possibility is documents that yield scores around zero, which means neutral sentiment. The sentiment analysis selected uses a “sentiment dictionary,” or lexicon, as a reference to determine which words are negative or positive, identify those words in each document, and compute the summation of negative and positive terms to yield an aggregate sentiment score for each individual document.19,40 Generating a sentiment score for each document is important because it can give practitioners an idea of the tone of each document across the continuum—from very negative to very positive.
The next step is to use an “emoji dictionary” to identify emojis embedded in the text. Containing a list of 1,024 different emojis, this dictionary was created by collecting all unique emojis over the entirety of documents and labeling each using the meaning set forth in the Unicode Consortium list at https://bit.ly/3C3dBhu. Identifying and quantifying emojis is also important, as they offer practitioners additional insight about the tone of each document and provide non-verbal cues. A pouting_face or thumbs_down emojis is clearly negative. A thinking_face can be used by individuals to express disbelief or skepticism.
Topic modeling integrates three data sources at the same time: the cleansed textual data, the sentiment analysis scores, and the emojis identified in the text. The suggested method uses Structural Topic Modeling (STM), a cutting-edge topic-modeling approach developed by Roberts et al.33 Topic modeling has been extensively used in management and related fields, although the most popular type employed is an older development called Latent Dirichlet Allocation (LDA).3,7 Examples of the many applications of topic modeling include the study of product dimensions that are relevant in online reviews, the study of online advertising, the study of consumer opinions, market-trend identification and product popularity studies, and social media content.26,27,28,31,41,45 Again, these applications are predominantly limited to LDA. The more novel STM has not been as widely used in management or related fields. However, some of its most recent applications include the study of customer complaints and customer satisfaction, job satisfaction, online COVID-19 information exchange, news contagion in the financial context, quality management, and a bibliometric analysis in information management.5,10,13,20,22,25,30,35,37
Unstructured data accounts for 80% to 90% of the total amount of data available in the digital world, seemingly mitigating the problem of data availability.
STM identifies topics contained in cleansed textual data, but the topic identification process is improved by incorporating additional non-text data—in this case sentiment scores and emojis—identified by the secondary tools. STM’s ability to incorporate additional data is what differentiates it from previous topic-modeling developments. The underlying assumption is that documents with negative sentiment scores containing emojis such as angry_face or thumbs_down—probably the case of Elizabeth—address different topics than documents with positive scores containing emojis such as clapping_hands or thumbs_up. Fresneda et al. provides empirical evidence of how topic identification can be enhanced by including sentiment scores in the topic-model analysis.16 Building on this novel approach, employing emojis as additional data offers topic detection an array of possibilities and nuances that goes beyond the simplistic sentiment score, which is merely an indication of global document polarity. Hence, both sentiment scores and emojis included in documents can significantly refine the topic identification and characterization process. With these three sources in place, STM automatically detects the most prevalent topics in each document. When the analysis is completed, topics can be presented as a list of associated words, or more technically speaking, as probability distributions over words.44
The next and final step is clustering analysis, using k-means clustering, which produces the actual segments. The idea is to group together those documents that address similar topics. The number of segments in the data is determined by a standard validation method, which uses average “silhouette” coefficient information for a possible range of clusters to identify the best-fitting cluster solution.34 Once the segments are formed, managers have plenty of information available about each of them, such as most prevalent topics, most frequent terms, most common emojis, and the average sentiment score. This information is also available for each individual document and can be grouped for each user profile, allowing practitioners to not only better understand each segment but also track the evolution and dynamics of individual users, and again, act accordingly.
As with any business process change, employing our recommended approach to unstructured data segmentation, as depicted in Figure 1, is not without its challenges. Deploying these tools may be difficult for firms that lack analytics talent with basic programming skills. The data’s size and complexity may constitute an additional challenge for organizations that lack the proper computational capabilities to handle and analyze unstructured data. Though we see these challenges mostly related to small and medium-sized corporations, an additional challenge in employing our suggested approach may be specifically related to larger corporations.
The process flow in Figure 1 was implemented using open source tools, such as R and Python programming languages. While these are state-of-the art, free tools accessible to everyone, many corporations employ commercial or proprietary software tools to implement tasks related to unstructured data, such as social-media monitoring, and they might be reluctant to change them for open source counterparts. While these software systems are powerful, they may lack the ability to implement some of the more cutting-edge, state-of-the-art methods that are becoming available frequently on open source software platforms. To illustrate the benefits of implementing this market-segmentation methodology, the next section offers an example of an application using Twitter data from a popular fast-food chain. Based on this empirical example, we will derive a set of additional implications about the implementation of unstructured data segmentation for companies.
An Empirical Example of Unstructured Data for Market Segmentation
In this section, we offer an idea of how market segmentation can occur using unstructured data. The data used in this example is publicly available on Kaggle.com and corresponds to customer support on Twitter from some of the largest corporations on that platform (https://www.kaggle.com). The selection of Twitter is based on data availability and, therefore, the replicability of our results since this unstructured data source is publicly available. The datasets are composed of customer service interactions between a company and Twitter users. Tweets from representatives of the firm were removed to focus the analysis on documents from the users themselves. The scope of the dataset is international, but the company selected for this empirical illustration is a U.S. firm, whose name we’ve removed from the dataset to maintain anonymity.
The empirical example corresponds to 22,427 tweets from users on a U.S.-based fast-food chain’s Twitter account. The analysis, which uses sentiment scores, emoji detection, STM, and k-means cluster analysis, yielded 43 different topics and six segments. The table in the online Appendix (see https://dl.acm.org/doi/10.1145/3478282) summarizes the most important features of each of those segments, including the number of documents in each segment, the most prevalent topics (presented by their most relevant associated words), some of the most common emojis used in the segment, and the average sentiment score for the segment. The Appendix also includes a few examples of the most representative Tweets in each segment, which are found to have the highest topical scores.
Table. Segment variation for User 118137.
The results reflect that customers who are disappointed with two of the products offered by this restaurant chain, queso and chips, form the core of Segment 1. Segment 2 is related to a Halloween promotion implemented by the restaurant, which seems to be appreciated and celebrated by customers, as reflected in the very positive sentiment score and emojis embedded in the text of the documents. Segment 3 contains customers reporting the location of the restaurant itself, probably because of promotions, reimbursements, missing items in an order, etc. (Note that reporting these locations yields neutral scores in the sentiment analysis, as expected.) Customers who are disappointed with either the assortment or amount of ingredients in the products constitutes Segment 4, reflected in both a negative sentiment score and negative emojis. Segment 5 is formed by customers who are apparently satisfied with their interactions with the restaurant chain. Fortunately for the company, this segment is the largest (74.4% of the total documents) and, as indicated in the included sample documents, there are clear indications of loyalty among these customers, although the sentiment score is only moderately positive. Segment 6 includes customers who experienced problems with either ordering online or using the restaurant app. If Elizabeth, the student in the introductory vignette, was part of the data and not a demonstrative, fictional character, her first tweet would be included in Segment 1 and her second tweet could be included in either Segment 4 or Segment 6.
Word clouds were also built for each of the identified segments (see Figure 2). A word cloud is a graphical representation of term frequency, which determines the size of a term in the plot—that is, the larger the term in the graph, the more frequently it is used in the text. While term frequency does not typically equate to term importance, since topic modeling relies on term frequency and co-occurrence, the most frequent terms in a segment can be an important source of insight.
Figure 2. Word clouds for each segment.
The results suggest that Segments 1, 4, and 6 comprise dissatisfied customers, at a specific time, which may call for recovery actions from the fast-food company. Insights from unstructured data segmentation can help corporations not only spot specific issues faced by consumers but also investigate and resolve those issues, communicate solutions, and develop actions to mitigate or eradicate the issues that created customer dissatisfaction. As an example, the disappointing flavor of a product can lead to customer dissatisfaction in Segment 1. The organization can investigate and solve the issue (a disappointing flavor) and then communicate the solution—all based on the insight gleaned by studying Segment 1. Also, the organization can develop specific and customized recovery actions for a disappointing flavor, such as targeting a certain segment of customers—in this case, customers in Segment 1—with promotional free trials of the corrected product. Contrary to this, satisfied customers constitute Segment 5, a segment that may call for “loyalty-building” actions from the restaurant chain, such as coupons or appreciation with a membership program. Noteworthy, customers can only belong to a single segment at each point in time, as illustrated in the next section.
Capturing the customer journey across segments. The first portion of the empirical example showed how customers could be ascribed to specific segments depending on the documents—tweets—they posted. In other words, so far, the study has accounted for Elizabeth being pleased with the coupon from the fast-food chain (tweet 1) and being completely displeased when she discovered the unwanted ingredients in her order (tweet 2). In this section, we illustrate the ability of unstructured data segmentation to capture the dynamic nature of customers as they transition from one segment to another. Following the same example, now the study will show how Elizabeth would transition from the ‘pleased’ segment (tweet 1) to the ‘displeased’ segment (tweet 2) by capturing the time dimension (tweet 1 was posted on Monday night while tweet 2 was posted on Thursday afternoon). This ability differs significantly from the “snapshot” approach of traditional survey-based segmentation methods. To show this, one of the customers from the segmentation example implemented in the previous section was selected.
The example refers to a customer of the fast-food chain company, who is coded in the dataset as user 118137. The Table shows this user’s different posting dates, the actual tweet text, the resulting sentiment score, and emojis embedded in the text. This customer’s “journey” begins by being disappointed with one of the products the restaurant chain offers, and he or she is therefore ascribed to Segment 1. The next tweet relates to the same topic and continues to express this person’s disappointment in a milder way than in the previous document. But this person remained in Segment 1. Later, this customer participated in the chain’s Halloween promotion and, therefore, transitioned to Segment 2, also indicated using specific emojis—for example, a jack-o-lantern. The customer finally expressed gratitude and satisfaction on the company’s Twitter account with the smiling_face_with_smiling_eyes emoji. Hence, this customer’s journey ends in Segment 5. The segment variation for user 118137 is also shown in Figure 3.
Figure 3. Segment variation for User 118137 (SS: sentiment score, E: emojis used).
The ability to track how customers transition across segments, and the real-time ability to track how customers evolve over time, creates new and interesting possibilities for managers to more efficiently allocate resources. In fact, converting customers from segments with negative sentiment to segments with more positive sentiment can be an important tool for companies to strategically monitor service recovery efforts.
Implications for Companies
In the “Big Data and Market Segmentation” section, the study called managers’ attention to three important advantages of unstructured data segmentation, including the availability of data, its dynamism, and its inexpensiveness. In light of the empirical analysis, we see additional takeaways for companies:
Managers can implement smarter actions from unstructured data segmentation results. Empirical analysis suggests that satisfied customers, at a specific time, constitute Segment 5, which may call for “loyalty-ensuring” actions from the company targeting these customers. Yet, the analysis also suggests that segments 1, 4, and 6 correspond to customers who are dissatisfied at a specific time, which may instead require recovery actions from the company. The online appendix and Figure 2 suggest that these segments may demand completely different recovery actions, as the reason of dissatisfaction is radically different among the three segments. While Segment 1 features customers who are disappointed with two specific products (queso and chips), Segment 6 is formed by customers who experienced problems ordering online or using the restaurant’s application. Hence, unstructured data segmentation can inform managers to help develop better, more tailored actions to respond to these specific challenges. As an illustrative example, customers in Segment 1 can receive free samples of improved queso and chips once the product issue is resolved, whereas customers in Segment 6 can be strategically redirected to in-store ordering through in-store purchase discounts while the online/app ordering issues are fixed.
The success of managers’ actions can also be tracked with unstructured data segmentation. Companies can capitalize on the dynamism of unstructured data segmentation as an additional tool to track the effectiveness of marketing actions and campaigns. For illustration purposes, let us assume that the Halloween promotion in the empirical example is intended to be a recovery action for disappointed customers. Companies might be interested in assessing the success of this promotion by tracking customer migration from Segments 1, 4, and 6 (displeased), through Segment 2 (the Halloween promotion), and into Segment 5 (pleased), shown in the second portion of the empirical analysis for customer 118137.
Unstructured data segmentation calls for more flexible marketing planning. Unstructured data segmentation is dynamic, but it also implies a shift in the current market segmentation paradigm, as it is based on consumer-generated data. Practitioners do not delineate the variables included in the analysis to perform segmentation. Instead, the analysis is implemented on what matters to consumers. Managers need to plan and structure flexible means to respond to the dynamism of this segmentation and to consumers’ ever-changing tastes, needs, and challenges.
Unstructured data segmentation can complement or replace traditional segmentation. Organizations that may not have implemented segmentation in the past due to very limited resources can think of this approach as an alternative to other segmentation methods that may require unavailable resources. Companies with established methods that may already implement segmentation can think of unstructured data segmentation as complementary to their existing approaches. Again, analyzing consumer-generated data drastically changes the managerial viewpoint, as it is implemented on what is significant to consumers and not the other way around. Complementing existing segmentation approaches with unstructured data segmentation helps organizations to ensure important topics for consumers will not be omitted, while businesses can continue pursuing their own market research goals through traditional, established methods. Regardless of the choice, the deployment of new unstructured data-segmentation capabilities may impact the current organizational structure.9,18
Unstructured data segmentation has additional potential applications beyond segmentation. The suggested approach has other potential application areas, such as (new) product development or consumer experience improvement. Managers can capitalize on the results of the analyses to develop better products (for instance, addressing the product issues suggested by Segment 1 of the empirical illustration) and improve consumer interactions with a company (for example, solving the ordering and app problems suggested by Segment 6 in the empirical section).
Prepare your company for future developments in unstructured data segmentation. Future developments might incorporate other types of data, beyond text and emojis, contained in that text. There are many other forms of unstructured data, such as images, audio, and video, which might play a role in the future development of unstructured data segmentation, giving organizations more nuanced results. Although the suggested methodology can be readily extended to many unstructured data sources, such as online consumer reviews, other social media platforms such as Instagram or YouTube rely more on other forms of unstructured data, such as images and videos respectively. This promises exciting future research in unstructured data segmentation. Future developments may impact even more organizational structures and accentuate the analytics talent problem.9,23
Discussion
Segmentation is a key component to better position products and identify opportunities in the market.24 Market segmentation plays a critical role in adapting the marketing mix for distinct groups of customers, and it is therefore a crucial tool for managers. While traditional segmentation may find unstructured data segmentation approaches puzzling, the growing availability of such data, and particularly textual data, highlights the need for practitioners to start capitalizing on segmentation methods that can incorporate text and other meaningful types of data, such as emojis, in the analysis.36 If organizations often implement market segmentation based on what is available, then the use of unstructured data is primed to take over as the next frontier of market segmentation.8,38
Join the Discussion (0)
Become a Member or Sign In to Post a Comment