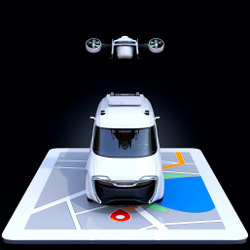
China’s e-commerce boom has generated a huge logistics demand, both in terms of express package delivery and on-demand food delivery.a
Chinese express firms delivered an estimated 40 billion parcels in 2017; up 28% from the previous year.2 Indeed, China’s on-demand delivery market exceeded over 30 million food orders daily by yearend 2017.
This growth in delivery services has created a healthy job market, with the number of employees in this sector up 130% from 2014 to 2017, according to the China Federation of Logistics and Purchasing. Last-mile delivery—the movement of goods from a transportation hub to final destination—has witnessed huge growth. Today there is an estimated three million couriers working in last-mile express delivery in China, and another one million couriers working for on-demand delivery services.
The country expects the express delivery industry to increase another 60% by 2020,3 requiring an even greater delivery force. However, the working population in China is aging; its numbers in steady decline since its peak in 2011 (a situation mainly caused by China’s 40-year-old “one-child policy,” which ended in 2016). This decline is expected to continue for at least another decade.
These two conditions have motivated the industry to seek a more efficient delivery solution—the autonomous delivery vehicle. But there are two dimensions of challenge—a profitable business model, and of course technology.
The Business Model of Autonomous Driving in Last-Mile Delivery
We have seen two major upgrades to the last-mile delivery business model: the warehouse is getting closer to the end users, and deliveries are merged into “multi-deliveries.”
In traditional logistics, the warehouse is a motionless unit used only for storage. Goods are transported from many other locations and then distributed to the multiple end users. The new express logistics, however, redefines the warehouse concept to be not only storage but also a mobile facility that relocates to serve many more users. Last-mile delivery is one such scenario applied to this “motion warehouse” concept, and the autonomous driving vehicle is one of its core technologies. The concept of motion warehouse is a revolution of logistics, which has combined the three major elements of retail business—people, goods, and ware-houses—into the new concept. The warehouse is aware of the needs of people, and provides the goods selection through the AI based big data analysis. In the new “motion warehouse” model, consumers can get the goods they want more accurately and quickly.
This approach extends the supply chain concept from the warehouse all the way to the end users. In a traditional logistic system, the connection is from the preposition warehouse directly to the end users. (A preposition warehouse is one in close proximity to the consumer). It could be an office building, or a small warehouse set up to serve a community that enables user delivery in 1–2 hours. However, with increasing population and demand, the “campus” model is emerging as an efficient way for even better user experience. Campuses’ aggregate delivery demands are always large so pre-allocation to customers and traditional (human) last-mile delivery is no longer the most efficient. By transporting a large number of packages together before the are allocated to customers, and delivering passively (users pick up packages at designated location) and proactively (carried directly to the end users), both user experience and efficiency are increased. Autonomous vehicles are key to achieving these benefits.
The Technology of Autonomous Driving in Last-Mile Delivery
Autonomous driving technology is not ready to replace human drivers in passenger vehicles. Many luxury vehicles may be equipped with advanced driver-assistance systems (ADAS)—including emergency braking, backup cameras, adaptive cruise control, and self-parking systems—but they are not fully autonomous. According to SAE International’s levels of vehicle autonomy as depicted in Figure 1, current ADAS functions mostly hover around levels 1 or 2. Technology is still far from realizing level 4—a truly driverless car.
Figure 1. SAE automation levels.
Two key challenges for fully autonomous passenger vehicles are trustworthiness and price. A typical autonomous passenger vehicle (as shown in Figure 2) is equipped with an array of state-of-the-art sensors and other technologies that can cost hundreds of thousand dollars.1 But even with such expensive equipment, autonomous vehicles are still far from reliable in terms of passenger safety. For example, last March a Tesla driver was killed when his car, set in “autopilot mode,” collided with a median barrier on the highway, causing the vehicle to catch fire.
Figure 2. Sensors on a typical autonomous passenger vehicle.
Table. Summary of California autonomous vehicle disengagement reports from 2017.
China just issued its first license for self-driving tests last March, and has not yet published any test result data, so we refer to autonomous vehicle disengagement reports issued by California’s Department of Motor Vehicles in 2017 (and summarized in the accompanying table). Waymo’s autonomous car required human assistance every 5,596 miles on average, which is the best among all tested vehicles. By comparison, a human driver on average has one accident approximately every 165,000 miles. Regulators will require autonomous cars prove much safer than current human behavior before drivers are no longer needed behind the wheel. Much more research and engineering efforts, possibly over a decade’s worth, is required to improve autonomous driving technology, including higher-resolution sensors, better algorithms, and faster computing chips.
Last-mile delivery, however, is a solid scenario illustrating how autonomous-driving technology has been successfully and safely employed. There are a few key differences between a delivery vehicle and a passenger vehicle. The last-mile delivery vehicle usually runs slowly, typically 20mph. A slow vehicle requires shorter perception distance, shorter braking distance, and less computing frame rate. Secondly, the last-mile delivery vehicle is typically smaller and lighter than passenger vehicles; this further decreases the risk of possible damage or harm when an accident happens. Finally, a delivery vehicle is free of passengers, therefore, it has fewer requirements for safety, planning, and control algorithms.
There are two major challenges in last-mile delivery, which autonomous driving can help: The distribution location of the package, and the delivery path. From past experience, when a delivery person arrived on location, the majority of time was spent waiting, especially when the planned delivery consisted of a large number of small packages destined for office buildings, campuses, and apartments. The waiting time for customers, and the handling time to delivery to customers, killed any efficiency of last-mile delivery. Moreover, the delivery person is paid by the number of packages delivered, meaning the company often pays a great deal of money for a trip to a single location, which kills any cost efficiency of last-mile delivery.
To be effective, last-mile delivery must determine the best route to dispense the most parcels. In a city, the best route is often not the shortest route, and road conditions constantly changed over time. An autonomous driving cart is similar to a larger “self-closing cabinet,” which can save both the average waiting time and the distribution time. On the other hand, equipment costs increase with the autonomous approach. Ideally, there would be a cost of only ¥1.5 per autonomous delivery compared with current ¥7-10 per delivery. Achieving this requires reducing costs of trial carts from ¥600,000 to ¥50,000 if the autonomous delivery (and vehicles) are in mass production.
Autonomous driving vehicles have major technology challenges, too. One obstacle is the behavior of the motion detection system when out in the real world. The algorithm may work perfectly in lab test conditions, but may not perform well when it is on the open road. Another difficulty with these vehicles is the range of vision when driving in dark or shadowing areas. Like the human eye, the range of the vision may vary in different levels of brightness; even with infrared light detection, the vehicle may not “see” in foggy and dusted environments.
How JD Uses the Vehicles in Delivery Scenarios
JD.com is the largest e-commerce platform by revenue, and offers a world-class set of online retail services to its legion of users, who now number close to 300 million in total. As a technology-driven company, JD.com has focused considerable effort in developing a robust and scalable retail platform that not only supports the company’s rapid growth but also allows it to provide cutting-edge technology and services to its partners and customers.
JD selected last-mile delivery practices as the first line of defense in its campaign to upgrade its logistics infrastructure. JD Logistics’ autonomous delivery vehicles will primarily be used for last-mile delivery in urban areas, carrying packages from dedicated stations to office buildings, pick-up stations, residential area convenience stores, and other locations. It was first used to support JD.com’s renowned two-hour express delivery service and will be rolled out across JD’s deliver network to be used for a wider range of applications. Autonomous driving vehicles will be loaded at delivery stations and will travel to pick-up points designated in advance by consumers. The recipient can collect the products they order simply by pressing a button on JD’s mobile app. JD’s delivery vehicle can also recognize the customer using face identification and deliver the product accordingly.
JD conducted its first trial in autonomous driving vehicles for last-mile delivery on June 18, 2017 at Renmin University, Beijing. The vehicle delivered about 10 packages in approximately six hours. JD subsequently deployed approximately 60 autonomous driving vehicles for last-mile delivery at Beijing, Xian, and Hangzhou for pilot AI-based package delivery. The city of Xian has been selected as the headquarters for JD’s fleet of vehicles. In December 2017, JD Group CEO Qiangdong Liu announced last-mile parcel delivery plans for 100 universities.
To be effective, last-mile delivery must determine the best route to dispense the most parcels. In a city, the best route is often not the shortest route, and road conditions constantly change over time.
As Figure 3 illustrates, the autonomous driving vehicles have been deployed and well tested in Beijing, Xian, and Hangzhou. Cities recently added include Tianjin, Guangzhou, Shanghai, Shenzhen, Changsha, Chengdu, Wuhan, and Suqian. User scenarios have also expanded from university campuses, to village areas, municipal areas, as well as industrial and institutional parks. Due to this wider reach, operations centers will be setup in Beijing to serve the north, and Changsha to serve the south.
Figure 3. The deployment progress of JD’s autonomous driving vehicle.
However, there are still many challenges for future fleet expansion; for example, deployment is currently not available to truly open, more rural environments and human interaction is still required to periodically adjust the autonomous driving path. In addition, the effort to build a fully autonomous driving vehicle network is heavily depended on how the technology evolves, moving from cloud computing to edge computing, the capability of sensor networks, and the level of intelligence in artificial intelligence. Moreover, laws and regulations covering autonomous vehicles on the open road can vary dramatically.
There are many pilot deployments extending the last-mile delivery programs, and as a result many customized last-mile delivery vehicles have joined the general-purpose fleet, such as the smart shopping cart to enhance the supermarket shopping experience; an model used for datacenter inspections; moving demo center vehicles used at conferences, and so on. As shown in Figure 4, JD does not intend to solve all the technology, business, and regulation limitations of these vehicles; instead, the focus is on carefully designing user scenarios that best fit the strengths of the current technology.
Figure 4. The use cases of the JD autonomous driving vehicle.
Last June, JD’s autonomous driving vehicles hit the open road in Beijing’s Haidian District (Figure 5). These courier robotic vehicles can perform 360-degree environmental monitoring, automatically avoid roadblocks and pedestrians, and can react to traffic lights. It can independently stop at a distribution point, send delivery information to the user, and the user can pick up the packages through face recognition, input code, or clicking on the JD app link from their mobile phone. The vehicle can store up to 30 containers, and can travel 15k/h. As a result, last-mile deliveries can upgrade from 100–200 orders a day, to 1,000 orders per day.
Figure 5. A JD courier robot on a road in Haidian District, Beijing.
Production difficulties still persist: the fault tolerance of the object recognition technology, the business processing for package returns from the end user to the warehouse, and the reliability of the vehicle (especially rounding corners) are three major challenges that must be resolved. In the early production period, human interaction may still be necessary. However, we believe when more road test data is accumulated and analyzed, the accuracy and efficiency of these vehicles will be fully achieved.
How Meituan Uses the Vehicles in Delivery Scenarios
Meituan is the world’s largest e-commerce platform for local services. Meituan’s service covers over 200 categories, including catering, on-demand delivery, car sharing, bicycle sharing, hotel and travel, movie, entertainment and lifestyle, and spreads over 2,800 counties, districts, and cities in China. In 2017, Meituan served 310 million active consumers and 4.37 million active merchants on the platform.
One of Meituan’s services is its on-demand food delivery known as Meituan Waimai. By May 2018, Meituan Waimai was delivering 21 million orders per day and had hired 600,000 food-delivery riders. The service is usually within three kilometers, with tight time limits of 30 minutes. The fulfillment process includes three phases: 1. The courier goes to the restaurant to pick up the food, usually by walking through a shopping mall to get to the restaurant; 2. The courier transports the food next to consumer’s building; 3. The courier walks or takes an elevator to the consumer (See Figure 6). In practice, each phase takes approximately one-third of the total delivery time.
Figure 6. Diagram of an on-demand delivery for Meituan’s autonomous vehicle.
Different phases need different types of vehicles. In phase 1 and phase 3, we use an indoor robot, as shown in Figure 7. This robot is 0.5m by 0.8m; its small size allows for easy entrance to shopping malls and office buildings. It does localization based on WiFi fingerprint and vision SLAM. It can also communicate through Zigbee to an elevator control module, thus can go up and down the buildings. The robot receives order information from the cloud scheduling system, runs to the merchant following the scheduled route, opens the top cover automatically so the merchant can put the food inside. When approaching its destination, the robot sends a text message to the user’s mobile app, and then the user can pick up the food using the password code included in the text message.
Figure 7. Meituan’s indoor delivery robot.
In phase 2, a larger and faster autonomous delivery vehicle is used for street transportation, as shown in Figure 8. The vehicle measures one-meter wide and two-meters long, with maximum speed of 40km/h and maximum load of 10 orders. It uses the same technology as an autonomous passenger vehicle, including lidar, camera, and GNSS receiver. It can detect pedestrians, bicycles, automobiles, and other obstacles, and can also react to traffic lights.
Figure 8. Meituan’s outdoor delivery vehicle.
Challenges and Opportunities
There are many challenges for the large-scale deployment of autonomous delivery vehicles: The technology is not mature yet, the entire ecosystem must be further developed to make it more reliable, and the costs much shrink.
Moreover, our living infrastructure is not yet ready for autonomous driving. Many communities have locked or gated entrances, which require manual operation using a key or access card. Many buildings have revolving or swing doors, which are easy for humans to use but very difficult for robots. Elevators are rarely robot-ready. In fact, we must talk to building owners to get a permit to install a communication module in every elevator. These frustrations must be handled before we can fully enjoy autonomous vehicle deployment.
Government regulation of autonomous vehicle is a critical concern. While these delivery vehicles run at a fairly slow speed, most regulators consider “slow” a grey area, fitting between high-speed passenger vehicles and bicycles. Government regulations are not ready to handle pure level 4 (driver-free) vehicles like autonomous delivery vehicles. What happens if the autonomous vehicle is involved in an accident or a traffic violation? Who is responsible? Closed environments such as common in last-mile delivery can be used as pilot scenarios for learning that enable more complex, open road scenarios. This suggests two autonomous driving vehicles development methodologies: find a killer-level use case to drive the business model; or find the best technology and build the ecosystem.
Fortunately, this somewhat immature technology is acceptable for slow-speed delivery vehicles. The lack of infrastructure support may prevent us from mass deployment in some situations, but there are still many suitable scenarios for first-stage deployment. As for government regulation, the Chinese government is among the most supportive for high-tech innovations like autonomous vehicles.
The Future of Autonomous Delivery Vehicles
China’s e-commerce boom brings a huge volume of logistics demand, both for express package delivery and for on-demand food delivery. The last-mile delivery is a perfect use case for autonomous driving technology.
Large-scale deployment of autonomous vehicles still depends on technology maturity and governing regulations. Nevertheless, these issues are not showstoppers. There are many pilot scenarios with government support, helping the industry step into the water of autonomous driving in order to accumulate the data and real-world experience needed to improve its technology.
Join the Discussion (0)
Become a Member or Sign In to Post a Comment