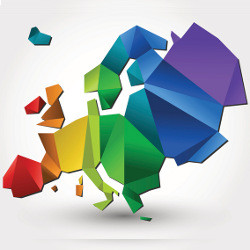
Making a high-quality gear cannot be learned simply from an Internet search. You may find guidelines, papers, rules, lectures, and videos. However, applying this general knowledge to a specific production process and dealing with uncertainties and disruptions requires special know-how, most of which resides in people’s heads and networks and is acquired to a large extent through “learning by doing.”
Over 10 years ago, the vision of Industry 4.05 was announced at the Hannover Fair 2011 as part of the German/European High-Tech Strategy and adopted internationally by the Japanese Industrial Value Chain Initiative, the Advanced Manufacturing Initiative in the U.S., the Chinese Made in China 2025 strategy, the South Korean Manufacturing 3.0, and the U.K.’s High-Value Manufacturing Catapult research center. This “fourth industrial revolution” follows the earlier stages of mechanization (steam engine), mass production (assembly lines), and IT-based electronic automation. Core elements of Industry 4.0 include a reference architecture (RAMI 4.0) for networking increasingly autonomous IoT devices, and cyber-physical production systems (CPPS) where simulations (“Digital Twins”) help monitor, predict, and control physical production systems. Moreover, personalized and context-specific “assistants” and related organization forms should enable “new work” settings.
Yet, the vision of Industry 4.0 is far from being realized. In the research cluster “Integrative Production Technology for High-Wage Countries” (2006–2018),2 the mapping of interdisciplinary models together with advanced mathematical techniques for model-order reduction not only resulted in much more realistic models, but also accelerated their simulation by up to five orders of magnitude. Meanwhile, data-driven analytics and machine learning (ML) entered the production landscape. The Excellence Cluster “Internet of Production” (IoP, 2019–2025)4 aims at a new level of digital collaboration with data, models, and knowledge in production. The core idea is establishing a “worldwide lab” (WWL) for cross-domain learning, breaking down current data silos (see Figure 1). A WWL combines data analytics, domain-specific models, and expert knowledge, and sharing of the gained know-how for a large industrial domain.
Figure 1. The worldwide lab of production is a breeding ground for innovation and cross-domain learning. Source: Martin Riedel.
To create real added value from the gigantic amounts of data that exist in all areas of production, a complete Digital Twin seems completely unrealistic due to the size of high-resolution databases, network overload, security, and data sovereignty concerns. Instead, the IoP approach circumscribes a near real-time digital representation of the WWL by a large collection of interrelated Digital Shadows (DS) as depicted in Figure 2. As task- and context-dependent, purpose-driven, aggregated, and persistent datasets, DS encompass a complex reality from multiple perspectives in a more compact fashion and with better performance than a fully integrated Digital Twin. From a production management perspective, DS can be interpreted as mediators between the vast amounts of heterogeneous data and detailed production engineering models with the needed granularity level.8
Figure 2. Concept of the Digital Shadow in production. Source: Cluster of Excellence “Internet of Production.”
DS research involves several CS disciplines.1 From a data management and AI perspective, a DS is an extended database view, comprising a defining reduced mathematical or ML model (view definition query) and its partial stored answer (materialization).6 From a model-driven software engineering perspective, traceability/provenance and cross-view interrelationships between different DS need to be managed. To complete the infrastructure,7 process mining creates and interprets DS on large-scale event sequences. HCI considers user interfaces to DS, and communication engineering studies DS as wrapped objects of transportation, protection, and storage in a network.
In the IoP cluster, more than 30 IoP institutes from different disciplines, such as computer science, engineering, material science, economics, and social sciences, as well as more than 50 industrial partners contribute competence in an iterative exploration and validation process, studying over a dozen interdisciplinary use cases. Application examples in aircraft turbine production, steel rolling, plastics and textile engineering, as well as electric car life-cycle engineering improve traditional KPIs such as quality, cost or efficiency, but also address important sustainability goals such as waste avoidance, energy saving, and CO2 reduction. Initial industrial uptake is happening by SMEs and start-ups, but also in the context of industry platforms such as the Volkswagen “Industrial Cloud,” the BMW “Open Manufacturing Platform,” or the alliance-driven “ADA-MOS” Industrial IoT platform. They enable new engineering solutions, for example, a new form of condition monitoring for a wide field of engineering applications.
The vision of Industry 4.0 is far from being realized.
In the future, the value of real production data—labeled by means of a suitable standard—will increase drastically. To create and fairly capture this added value, the world of production in academia and industry must advance toward a trustworthy WWL. Beyond integrating engineering and CS/AI research, this requires more socioeconomic research in considering DS as social objects of human work, and as the units of trade in business models. In summary, we understand the concept of Digital Shadows as an important novel computer science abstraction for representing and sharing CPPS knowledge in future production environments.
Join the Discussion (0)
Become a Member or Sign In to Post a Comment