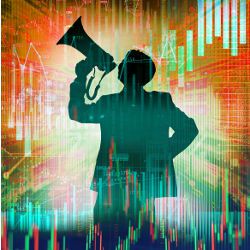
The transition of the largest online advertising platforms to auction-based automated real-time designs has transformed the advertising industry. The advertisers had an opportunity to design flexible goal-specific advertising campaigns, target focused small groups of consumers, and perform fast and efficient experimentations. At the same time, consumers can be exposed to a smaller number of higher quality advertisements. However, in order to achieve the design goals, such as optimal placement of ads that maximizes the sum of utilities of users or revenue generated by the auction platform, the auction needs to be carefully optimized. To optimize the auction, the auctioneer typically chooses a relatively small number of auction parameters that determine the allocation of items and prices based on submitted bids. Typical auction parameters include reserve prices, which determine the minimum bid and can correspond to any allocation. Another parameter used in sponsored search auctions is the quality score, which is a positive bidder-specific weight used to discount or inflate submitted bids before they are ranked. The setting of auction parameters requires a knowledge of advertisers’ preferences and consumers behavior, which can be acquired from data. This makes econometric inference from observed data of high importance for the design and analysis of online advertising auctions.
Key Insights
- Modern advertising platforms are automated systems powered by auctions that allocate and price advertising slots contemporaneously based on the bids submitted.
- Real-time auction-based systems require good predictions of user behavior, need to adapt to the changing marketplace, and require fast and robust methods for evaluating performance.
- These challenges can be addressed by applying econometric methods to the data from advertising platforms.
The structure of online advertising exchanges is becoming significantly complex, and requires multiple parameters to be input by auction designers. These parameters are required to yield consistent advertising allocations, relevant user ad experiences over time and catering ad placements to advertisers’ goals. This operational structure of online ad exchanges has been incorporated into the algorithmic implementation of advertising auctions, see Muthukrishnan,29 Aggarwal and Muthukrishnan,30 and Muthukrishnan31 for details of such an implementation. However, the increased heterogeneity and dynamics of the marketplace call for quick “on demand” adjustments of auction parameters in order to pursue auction platform revenue goals and relevant ad experiences for users. Recent evidence from the sponsored search advertising marketplace indicates that there are significant gains (both in terms of overall welfare and ad exchange revenue) from adopting data-driven designs. For example, Ostrovsky and Schwarz34 described the experiment on Yahoo!’s search engine where the advertisers `per-click values were recovered from observed bids. The distribution of recovered values was then used to set search query-specific reserve prices in the advertising auctions. The data shows a significant increase in the search engine’s revenue resulting from this switch.
As with other economic markets, advertising markets balance supply and demand. The webpage content attracts consumers and supplies their “attention.” Advertisers demand user attention since it converts advertised products and services to purchases. As in all economic markets, the equilibrium of demand and supply is driven by price. The dominant pricing model for advertising puts payment control on the side of advertisers and lets them determine their preferred prices. At the same time, consumers receive a “free” service. This feature has made advertiser behavior of direct importance for monetization and, therefore, has been studied in detail in recent years.
We survey the work devoted to the analysis of advertiser behavior, starting from market equilibrium models to more recent work where advertisers must adopt learning strategies to set their bids. We then discuss the “supply” side of the market, which to a large extent remains model-free. Recent empirical work has focused on determining actual advertisers’ value extracted from users. This work turned out to be significantly more delicate since user behavior is highly correlated with user characteristics, and thus, it is very difficult to distinguish the effect of advertising from the inherent propensity of particular users to purchase particular products. Finally, we describe recent changes in user behavior caused by the transformation of browsing and purchasing patterns generated by new devices, and new ways of accessing the Internet. We predict this trend will generate the need to do more in-depth consumer-side research of advertising markets.
The complexity of advertising markets highlights the importance of inference, as inference informs customized market-specific design and optimization of advertising markets. Here, we review existing and emerging approaches to inference for advertising auctions both aimed at the diagnostic analysis of advertising marketplaces and as an input to decisions regarding marketplace changes.
Platform Design and Advertiser Behavior
Bidder value inference. While the general auction platform design can be based on pure economic theory, platform optimization requires the knowledge of the bidders’ underlying preferences and the prediction of user action. This knowledge is derived from the auction outcomes data. The general problem of data-driven auction design can be formulated as the counterfactual inference—from observations of agent behavior in the platform, we want to predict how they will behave if the platform design is changed. With the new design, participating agents will re-optimize their strategies, and, thus, this new design leads to the change in bids. Counterfactual inference, therefore, requires the platform to anticipate strategic responses of bidders to the mechanism change.
The counterfactual inference is based on the economic model that characterizes agent behavior on the platform. Based on this model, one can use data to infer the “primitives” of the model (such as agent preferences) that are consistent with the observed behavior. The prediction for the new design will be based on computing bidders’ responses to the new auction mechanism, and the changed bids of the other bidders.
In an advertising auction model, the utility of a bidder (that characterizes the expected gain of the bidder from participating in an auction) is typically set equal to the click probability multiplied the bidder’s value per-click less the cost. The click probability depends on both the identity of the bidder and the placement of the ad. Therefore, it ultimately depends on the bid that determines where the ad is placed. In this model, we characterize each bidder by a single parameter that expresses this bidder’s value per click. Inference of bidders’ values per click is, therefore, one of the key ingredients of counterfactual inference. Another ingredient is the computation of the Bayes-Nash equilibrium of the auction.
The Bayes-Nash equilibrium of an auction is the set of bids and beliefs of bidders regarding the distribution of uncertainty in the auction such that the bids optimize bidders’ expected utilities, and the beliefs of bidders correctly capture the realized distribution of uncertainty. When bidders use Bayes-Nash equilibrium strategies and the equilibrium is unique, the values can be inferred directly from data by inverting bidders’ best response functions. The inferred bidder value makes the observed bid the best response to the observable empirical distribution of opponent bids. Athey and Nekipelov3 adopted this strategy to infer bidders’ values in sponsored search auctions. Sponsored links that appear beside Internet search results on major search engines are sold using real-time auctions. Advertisers place standing bids where they are associated with search phrases that form part or all of a user’s search query. Each time a user enters a search query, applicable bids are entered to an auction. The ranking of advertisements and the prices paid depend on advertiser bids as well as “quality scores” assigned for each advertisement and user query. These quality scores are traditionally set equal to the predicted probability of a user click on an ad. They vary over time because the statistical algorithms predicting quality produce different predictions based on the features of the user issuing the search query.
In theory, the allocation and payment functions in standalone position auctions are discontinuous with respect to bid, because the price only changes when the bidder goes up in the ranking with an increased bid. However, in ad auctions each bid is typically applied to many auctions. With sufficient uncertainty over auctions, the expected auction outcomes will be continuous. As a result, to accurately characterize the behavior of bidders in this setting, Athey and Nekipelov3 propose a model that has these properties.
The model proposed by Athey and Nekipelov3 deviates from the standard model of a Bayesian auction game where the values of the players are drawn from the distribution. The main reason for this deviation is that in real-world advertising auctions the sets of bidders are fixed and most bidders’ bids do not change over long time intervals. Athey and Nekipelov’s model rationalizes this observation. In the rest of the survey we adhere to the model of Athey and Nekipelov and assume the values of the bidders are fixed.
Consider the model where there are I bidders whose ads are eligible to be displayed to a user alongside the search results and to be placed in J available advertising positions. The user clicks on the ad i shown in the position j with probability Cij, where Cij = αj · si is the product of the ad-specific click probabillity si (for i = 1, …, I) and the advertising slot-specific click probability αj (for j = 1, …, J). The bidder-specific click probability si for the particular bidder i is a random variable whose realizations vary across search queries of user. The randomness of the bidder-specific click probability reflects different propensities of different users to click on a given ad.a The bidder-specific click probability si is referred to as the bidder’s quality score (or, simply, the score).
This description shows how an ad platform conducts a score-weighted auction. In each user query, the platform runs an auction where each advertisement i is assigned the score si, and bids are ranked in order of the product bi · si. The per-click price pi that bidder i in position j pays is determined as the minimum price such that the bidder remains in her position,
where bidder k is in position j + 1. Note that advertiser i‘s bid does not directly influence the final price she gets charged, except when it causes to change positions. In effect, an advertiser’s choice of bid determines which position she attains, where the price per click for each position is exogenous to the bid and rises with position. An auction with this type of pricing rule is called the (score-weighted) generalized second price auction.
Advertisers demand user attention since it converts advertised products and services to purchases.
Per-click values of bidders vi for i = 1, …, I are fixed for each bidder across user queries and are supported on [0,ῡ]. The pay-off of bidder i in a query where this bidder receives a click is the surplus vi – pi, where pi is bidder i‘s price per click defined by Equation (1).
While we assume all I bidders are eligible to be displayed in each user query, the actual sets of participating bidders in a real search query page will vary. For instance, some bidders may be excluded from certain queries based on the targeting settings (that is, advertisers may prefer to advertise in specific geographic locations). Suppose each bidder knows the entire set of bidders I, but can not observe the set of competitors in a given query and can not observe neither her own nor her competitors’ scores. In this case, bidders form beliefs regarding the distribution of scores of all bidders (since they affect the prices in individual user queries), and beliefs regarding the distribution of realizations of sets of their competitors across user queries. The standard assumption in the sponsored search auction literature (for example, Edelman et al.16 and Varian41) is that bidders have full information regarding each other’s bids. This reflects the idea of a high frequency environment where bidders can quickly determine the bids of their opponents, even if the auction platform does not explicitly provide that information. Bidder i then maximizes the expected pay-off (with per click value) with the bidder’s beliefs regarding the distribution of uncertainty of scores and sets of competitors. This pay-off (a.k.a. utility) can be expressed as,
where the expectation E is taken over the distribution of scores s1, …, sI, and the distribution of sets of bidders participating in the auction. We emphasize that the pay-off depends on the bid of the bidder i (bi) as well as the bids of all competing bidders (b-i) that may determine the rank and the price that bidder i pays depending on a realization of the set of scores.
Here, we consider the question of bidders’ per-click values that can be recovered from data that contains a large number of queries for a given set of potential bidders. For each query, the data available to the advertising platform contains the bids, a set of entrants, and the bidders’ scores relating to each user query.
In this case, we can define function Qi(·) to be equal to the probability of click on the ad of bidder i in a search query as a function of all bids. We also define function TEi(·) equal to the expected payment of bidder i in a search query as a function of all bids. The utility of bidder i can be written in terms of these functions as,
The best-responding bid maximizes the expected utility given the bids of opponent bidders (b-i). Athey and Nekipelov3 demonstrate that with sufficient smoothness and support size of the distribution of scores, functions Qi(·) and TEi(·) are strictly increasing and differentiable with respect to bids. As a result, the value per click for each bidder can be recovered using the necessary first order condition for optimality of the bid.
Functions Qi(·) and TEi(·) can be recovered directly from the datab (for example, using splines or any other appropriate method). Thus, the per-click value can also be recovered from a data using Equation (3). Smoothness, and monotonicity of Qi(·) and TEi(·) can be directly tested in data and has been verified using the auction data by Athey and Nekipelov.3
Equation (3) provides a simple practical method for estimating value per click. For each bidder we change bid by a small amount and then compute the change in the outcome of the auctions where the original bid of the bidder was applied. The evaluated change in expenditure will serve as an estimator for the derivative of TEi(·) function and the evaluated change in number of clicks will serve as an estimator for the derivative of Qi(·) function.
An advertiser’s choice of bid determines which position she attains, where the price per click for each position is exogenous to the bid and rises with position.
Evaluation of new auction mechanisms using data. The approach adopted in the empirical economics literature (see Bajari et al.4,5) provides a simple recipe for platform optimization subject to the inferred bidder preferences, assuming the bids constitute the Nash equilibrium (defined previously in terms of best responses and beliefs of bidders) under the new settings. To follow this recipe, first we estimate the components of the first-order condition (Equation 3) using data from historical realizations of auctions. Value per click can then be reverse engineered from the bids for each bidder. Second, we plug in the new proposed auction parameters (or consider a new auction format). For each set of bids we can simulate the auction outcomes under the new auction parameters. These new auction outcomes (prices and allocations for hypothetical bids under the new auction rules) will generate counterfactual functions
and
for each bid profile. Finally, assuming the system converges to the long-term Nash equilibrium under new auction rules, and the values of the bidders do not change, we can predict the new bids. To do so, we find the set of bids for all participating bidders such that the bid of each bidder maximizes her utility under the new auction rules given the bids of other bidders. Since the maximum utility will be attained at the point where its derivative with respect to the bid is equal to zero, finding the new equilibrium bids is reduced to solving the system of nonlinear equations for i = 1, …, I
using values vi inferred from the data (using Equation (3)) and solve for the set of bids
, …,
that makes all I equalities hold. Unlike Equation (3) where we use actual bids bi to obtain the values, in Equation (4) bids
are the outcome and the values are the input. We note that this strategy only works if functions
and
are strictly monotone and the equilibrium is unique.
Monotonicity of payment and allocation functions along with the uniqueness of the Nash equilibrium allow us to use numerical continuation methods to find the new equilibria. The numerical continuation approach to finding this new equilibrium is based on transforming the set of conditions Equation (4) into system of ordinary differential equations. Athey and Nekipelov3 show that solving this system of differential equations is equivalent to finding the new equilibrium (that is, it will not diverge or generate new extraneous solutions). The solution of numerical approximation of ordinary differential equations will yield the new equilibrium.
Although formulating the equilibrium computation problem in terms of solving the system of differential equations is mathematically elegant, the process can be complicated since it requires many calls to functions
and
, which need to be computed from the data for each evaluation. This problem is further accentuated in most advertising markets where the large number of eligible bidders participating in each auction leads to difficulties in computing the new equilibrium, even when sufficient conditions hold for existence and uniqueness of the Nash equilibrium.
A significant simplification in equilibrium settings can be achieved when the object of interest is not the entire vector of bids, but a specific lower dimensional auction outcome, such as revenue or welfare. When a simple outcome is defined, the entire vector of counter-factual equilibrium bids is no longer of direct interest, which effectively reduces the scale of the computational problem. Next, we consider this approach in application to social welfare in an auction.
The price of anarchy bounds. While equilibrium-based analysis can be widely applied, it is largely based on the idea that bidders use equilibrium strategies and the corresponding set of bids constitutes a Nash equilibrium. A common assumption used in the economics literature is the equilibrium is unique in a given market. In practice, however, it is impossible to guarantee that sufficient conditions for Nash equilibrium uniqueness are satisfied in a given auction mechanism. For instance, the non-monotonicity of either the payment or allocation function leads to several possible values for each click that are consistent with observed bids. Then the simple equilibrium-based analysis is no longer valid.
The economics literature has developed an approach to the analysis of data from outcomes of games with multiple equilibria and games in non-equilibrium settings (for example, see Aradillas-Lopez et al.1 and Beresteanu et al.6). The approach is to directly consider the optimality conditions for the players in these new settings. In auctions such optimality conditions lead to sets of possible values of bidders referred to as identified sets. This approach, however, has proven to be very difficult with reported computational time significantly exceeding those in the equilibrium framework even for simple games (see Ciliberto and Tamer13).
Koutsoupias and Papadimitriou23 have developed a theoretical framework for analyzing simple auction outcomes such as revenue and welfare. The framework produces bounds for these outcomes comparing them to the welfare-maximizing allocation over all feasible realizations of bidders’ values. This approach is referred to as the price of anarchy approach, which is defined as the ratio of the worst-case objective function value of the outcome of a game (such as Nash equilibrium outcome) and that of an optimal outcome. It turns out the price of anarchy can be established under minimal assumptions on the underlying preferences for many commonly used auction mechanisms (for instance, see Roughgarden36).
There are two difficulties with practical application of the price of anarchy bounds for a given game. First, these bounds can be quite conservative. Given they are derived taking the worst case equilibrium and the value profile, these bounds can be large. However, the values of players and the equilibria that generate the worst-case outcomes may not occur in reality. Even when the data from the mechanism is available, the price of anarchy bounds do not take it into account. Second, the price of anarchy is mechanism-specific and has so far been derived on the case-by-case basis.
Hoy et al.20 bridge the gap between the robust but coarse theoretical price of anarchy bounds and precise but difficult to compute identified sets. The idea is to integrate data directly into the price of anarchy style analysis that can be applied to large classes of existing auction mechanisms. This new concept is called the empirical price of anarchy.
Here, we discuss the idea behind the empirical price of anarchy for auction settings where bidders adhere to playing full information equilibrium best responses in the auction mechanism A, but where those best responses are not unique (for example, due to non-monotonicity of allocations and prices in the auction). We assume, like in the model of Athey and Nekipelov,3 the auction parameters are random while the profile of bidders’ values is fixed and we use V = (v1, …, vI) to denote that profile. For a given profile of values auction A can have multiple Nash equilibria that constitute set Σ(A, V). Combined with the distribution of auction parameters across user search queries, an equilibrium (which is an element of Σ(A, V) ) generates the distribution of observable auction outcomes (allocations and payments of bidders) D(·) across those user queries.
If the set of all equilibria Σ(A, V) is not a singleton, then there will be many equilibrium distributions of outcomes D(·) that correspond to value profile V. Conversely, for each distribution of auction outcomes D(·) there may be multiple value profiles of the bidders that could have generated that distribution of outcomes. Our next step is based on exploring this information to make statements regarding the counterfactual welfare (the sum of bidder utilities and the revenue of the auctioneer) in auctions.
We define the Empirical Bayesian Price of Anarchy (EPoA) as the worst-case ratio of welfare of the optimal allocation to the welfare of an equilibrium in a given auction A, taken over all value profiles of the bidders V and Nash equilibria that could have generated the observed distribution of auction outcomes D(·). The notion of EPoA allows us to provide bounds on the (unobserved) welfare of the optimal allocation (OPT) and the welfare of the auction A that is actually implemented,
Thus, the EPoA is the characteristic of an auction that allows us to measure the efficiency of the current auction mechanism without estimating (the set of) values of the bidders.
The empirical price of anarchy (and, subsequently, the bound for optimal welfare) is defined for the true distribution of auction outcomes D(·). Then, the idea is to replace the true distribution of auction outcomes with empirical distribution
of auction outcomes observed in the data. Given that the empirical distribution is a consistent estimator of the true distributionc the bound for the welfare constructed using
will bound the actual auction welfare with probability approaching one (as we get more samples from the distribution of auction outcomes D(·)). We call
the estimator for EPoA.
We note that even the estimator for EPoA is defined as a potentially complex constrained optimization problem. It turns out that it is possible to avoid solving this problem by invoking the revenue covering approach. The revenue covering approach is based on establishing the ratio of the actual auction revenue and maximum payment that can be extracted from participating bidders in equilibrium. This ratio can be used to establish a simple bound for EPoA. We now describe this approach in more detail in application to the sponsored search auction.
Consider the sponsored search auction model with uncertainty as we described in detail. We can define the average price per click for bidder i with bid bi as ppci(bi) = TEi(bi)/Qi(bi). The typical function that provides the expected number of clicks as a function of the bid Qi(bi) in the sponsored search auction is continuous and monotone. As a result, we can construct its inverse b =
(z) that specifies the bid that is needed to get the expected number of clicks z. Then, the average price per click can be redefined in terms of expected clicks as ppci(z) = ppci(
(z)), which is the average price per click that bidder i getting z clicks pays. Function τi(z) =
(z) is called the threshold because it corresponds to the minimum price per click that bidder i needs to pay to get z clicks in expectation. The cumulative threshold for agent i who gets Qi expected clicks is
can be interpreted as the total payment that bidder i would have paid, had she purchased each additional click at the average price of previously purchased clicks when she purchases a total of Qi clicks.
DEFINITION 1. Strategy profile σ of auction A (defining the mapping from bidders’ values into their bids) is μ-revenue covered if for any feasible allocation
Auction A is μ-revenue covered if for any strategy profile σ, it is μ-revenue covered.
The inequality Equation (6) can be defined in expectation over the realizations of possible equilibrium profiles and all thresholds corresponding to the realizations of bid profiles. Then, the expected revenue from the auction can be measured directly by the auction platform (as a sum of payments of bidders per auction over the observed auction realizations). The thresholds can be computed via simulation from the observed auction realizations given that the allocation and pricing rule is controlled by the auction platform, and thus empirical equivalents of TEi(·) and Qi(·) can be computed for each bid bi. As a result, the platform can compute the revenue covering parameter for given auction mechanism A.
The next result, developed in Syrgkanis and Tardos,37 takes revenue covering parameter and provides the EPoA for the auction mechanism A.
THEOREM 1. The welfare in any μ-revenue covered strategy profile σ of auction A is at least
-approximation to the optimal welfare. In other words, EPoA
.
The estimator for the EPoA is then obtained by replacing the true revenue covering parameter μ with its estimator recovered from the data. As a result, this approach allows one to establish the bounds for auction welfare bypassing complex computations required in the approaches previously used in the economics literature by combining statistical inference and results from the algorithmic game theory. The Syrgkanis and Tardos37 approach may potentially be applied to other bounds, for example, comparing the welfare of a given auction mechanism to the welfare of another auction mechanism (instead of the welfare of the optimal allocation). We believe that such an analysis is an important direction for future research.
Advertising markets with learning agents. Real-world advertising platforms are complex systems with thousands of bidders who compete in the same auction with bidders dynamically changing their bids, entering and exiting auctions. In this case, information requirements for bidders to derive their Bayes-Nash equilibrium profiles are truly impractical since they are required to form beliefs over the actions of all of their thousands of opponents, as well as the dynamic adjustment of auction parameters by the advertising platform.
In practice, most bidders in these large advertising platforms use algorithmic tools that allow them to automatically and dynamically update their bids for multiple ads and advertising campaigns. The algorithmic solutions implemented in these tools take the advertisers goals (in terms of yield of auction outcomes) as inputs, and adjust bids using dynamic feedback from the auction outcomes. Such implementations can be associated with algorithmic learning, where the bidding strategy is treated as the goal of online statistical learning procedure.
Recent work by Blum et al.,8,9 Blum and Y. Mansour,10,11 Caragiannis et al.,12 Hartline et al.,19 Kleinberg et al.,22 Roughgarden,36 and Syrgkanis and Tardos37 shows that some of the worst case outcome properties of full information pure Nash equilibria extend to outcomes when all players use no-regret or low-regret learning strategies, assuming the game itself is stable. The assumption that players use low-regret learning to adjust their strategies is attractive for a number of reasons. First, low-regret learning outcome generalizes the Bayes-Nash equilibrium assumption. Rather than assuming that at any time players’ actions form a Bayes-Nash equilibrium, it is only necessary to assume that each player has no-regret for any fixed action over a longer time period. Thus, this assumption reduces the requirement to gather player aggregate behavior over multiple runs of the game rather than the constraint of optimality in each game. If behavior of all agents is stable over the time period, this exactly fits the Nash equilibrium assumption; however, this also allows evolving play. Second, there are many well-known learning strategies that guarantee players achieve no regret, including the weighted majority algorithm2 also known as Hedge,17,26 and regret matching18 just to name a few simple ones.
Real-world advertising platforms are complex systems with thousands of bidders dynamically changing their bids, entering and exiting auctions.
In the context of bidders using algorithmic strategies, we cannot directly estimate their preferences from observed actions. While in Nash equilibrium, bid is the expression of bidder’s value per click, but with algorithmic strategy, the bid is solely the outcome of the algorithm and thus the link between the bid and the value can be lost if the algorithm only approximately optimizes the bidder’s objective function. In this case there may be a range of possible values that are compatible with the observed bids.
Nekipelov et al.33 use the concept of ε – regret learning to study online learning in sponsored search auctions. In online learning settings the regret of a given learning algorithm measures the difference between cumulative loss achieved by predictions given by the algorithm and loss of the best predictive function (a.k.a. “expert”) from a given reference set. This concept can be applied to learning in repeated sponsored search auctions. The loss function will be associated with the negative utility in the sponsored search auction described earlier (that is, the loss minimization will correspond to the utility maximization). Nekipelov et al.33 suggest set the strategies that adhere to a constant bid over the sequence of auctions. This idea is motivated by the empirical observation of Microsoft’s Bing advertising platform, where a large fraction of bidders do not change bids over the lifetime of their advertisements. Then, the average regret of bidder i over T periods against the expert that sets the constant bid b’ can be evaluated as,
where index t correspond to the time period. The sequence of bids
achieves εi-average regret if for any expert b’ (from the bid space) Regreti(b’) ≤ εi. Based on this definition Nekipelov et al.33 introduce the notion of a rationalizable set. We note that if a given sequence of bids
has εi-average regret, then the value per click vi of bidder i and the average regret εi satisfy the set of inequalities
for each b’. Since bid sequences are observed in data, and the components of bidder utility (expected clicks and expected payment) can be simulated, these inequalities impose restrictions on pairs (vi, εi). The set of these pairs is the rationalizable set is denoted as
Join the Discussion (0)
Become a Member or Sign In to Post a Comment