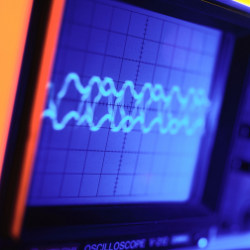
The history of invention is a history of knowledge spillovers. There is persistent evidence of knowledge flowing from one firm, industry, sector or region to another, either by accident or by design, enabling other inventions to be developed.1,6,9,13 For example, Thomas Edison’s invention of the “electronic indicator” (US patent 307,031: 1884) spurred the development by John Fleming and Lee De Forest in early 20th century of early vacuum tubes which eventually enabled not just long-distance telecommunication but also early computers (for example, Guarnier10). Edison, in turn, learned from his contemporaries including Frederick Guthrie.11 It appears that little of this mutual learning and knowledge exchange was paid for and can thus be called a “spillover,” that is, an unintended flow of valuable knowledge, an example of a positive externality.
Key Insights
- Knowledge spillovers from instrument technologies are exceptional and sustained over a long period of time.
- Knowledge spillovers from information technologies accelerate dramatically after 1970 and are closely related to instruments.
- Digital instruments—the intersection of information and instrument technologies—have been particularly generative in spurring invention in other sectors.
Information technologies have been a major source of knowledge spillovers.a Information is a basic ingredient of invention, and technologies that facilitate the manipulation and communication of information should also facilitate invention. Indeed, Koutroumpis et al.17 found that information technology patents receive more citations than patents in other technology sectors. Similarly, Klevorick et al.16 found that advances in information technologies can generate broader technological development by enhancing technological opportunities in adjacent industries.
Another technology sector that has been theorized as generating outsize spillovers are instrument technologies.b Instrument technologies include measuring equipment ranging from scales, rulers, chronometers, thermometers, and accelerometers to more complex devices such as interferometers, spectrometers, oscilloscopes, medical transducers, and electron microscopes. Despite arguments by historians of science that instrument technologies enabled industrial revolutions (for instance, see Baird2 and Price21), they have not been extensively studied. The studies that do exist are primarily qualitative descriptions of patterns of innovation rather than quantitative empirical analyses. For instance, Rosenberg22 illustrated how improvements in the instruments used for measuring, testing and manipulating materials also affect the results of industrial R&D. Aware of the scarcity of empirical research on instrument technologies, Rosenberg called for “more research [that] may powerfully illuminate the course of scientific progress in the twentieth century … [and that] … the scope of such research must be international.”
In this article, we undertake such an empirical study. While there are many studies on knowledge spillovers from specific technologies (such as broadband, see for instance Becchettiet et al.3 and Bertschek et al.5), there are few that have considered broader technological classes and economy-wide spillover effects. The study closest to ours is Hall and Trajtenberg12 who conducted analyses of patent citations to identify technologies that can be characterized as general-purpose technologies because of their generality and association with rapidly evolving technology sectors. Although we also conduct patent-level analyses, we are not interested in identifying general-purpose technologies. Instead, we seek to evaluate the impact of instrument and information technologies as sources of invention by investigating which technology sectors and fields are the most prolific sources of knowledge inputs into the invention activities of other sectors, and how these sources have changed over time. Thus, we are interested in identifying the evolution of the sources of invention, and in particular, the roles played by instrument and information technologies.
To do so, we examine how new technologies are disseminated and adopted across industries to characterize long-term shifts in the sources of technological change. We conduct a long-term descriptive analysis of cross-sectoral knowledge flows through analysis of patent citations. We take a big data approach and consider the entire technological progress of the world for more than the past century. We analyze the technology sectors of patented inventions and their prior art citations to describe the direction and volume of knowledge flows among between technology fields. This allows us to describe evolving relationships between technology fields that are difficult to discover with a short or industry-specific sample. Our methodology allows us to present an accurate image of large-scale technological trends in the economy. While specific inventions are impossible to predict, this approach can be used to pinpoint emerging areas of exceptional R&D productivity and impact. As such, our study provides the first quantitative empirical analysis of instrument and information technologies as a key source of knowledge for other fields of invention.
Our results highlight, first, that spill-overs from instrument technologies, as anticipated by historians of science, are exceptional and sustained over a long period of time. We also find the spill-overs from information technologies accelerate dramatically after 1970 and that they are closely related to instruments. In fact, the intersection of information and instrument technologies, which we call “digital instruments,” has been particularly generative in spurring invention in other sectors.
Second, we conceptualize these exceptionally generative classes of technologies as “invention machines” due to their critical roles in the processes of invention in many sectors of the economy. We suggest that both information and instrument technologies should be considered as types of “Turing machines of invention:” Per Rosenberg, information technologies enable the manipulation of information, whereas instrument technologies enable the manipulation of physical matter (chemical substances, artifacts, physical processes, biological organisms). Thus, they are not only general-purpose technologies that can be utilized in many different sectors but also general invention technologies that facilitate the discovery of other technologies. Together, instrument and information technologies as digital instruments have been used to automate a wide range of industrial processes since early 1970s. They constitute an essential combination whose fundamental nature in technological change has thus far gone unnoticed.
Finally, we suggest that digital instruments constitute the core of industrial systems and other “smart” systems, often known as the Internet of Things (IoT). The evolution of these technologies began decades ago and reflects a convergence of industrial instrumentation with digital communication technologies. While the processing and transmission capacity of such systems has multiplied in recent years, the core ideas and technologies have existed for a long time. We suggest the continuing coevolution of instrument and information technologies will generate very powerful invention machines for the coming decades, spurring a potential technological flourishing in the adopting sectors. We now describe our methodology to analyze the patent database and present our empirical results primarily through visualizations.
Method
Given the difficulty in measuring knowledge flows between firms, patent citations have long been considered proxies for the flow of knowledge from the inventors whose patents are cited to the inventors making the citations. Much economic research has attempted to measure and assess the implications of such spillovers by analyzing citations made in patent documents to predecessor inventions. To verify this measurement strategy, Jaffe et al.14 surveyed the meaning of patent citations and concluded that a substantial part (but by no means all) of such citations involve actual flows of knowledge. Thus, patent citations are a noisy but meaningful indicator of knowledge spillovers in an economy. However, patent citations must be used carefully, as citations can be added not only by the inventors, but also by the patent attorneys and the patent examiners involved with the patent application, with the final decision ultimately lying with the patent examiner.8 That said, patent data remains a valuable, even if imperfect, tool with which to measure how new technological knowledge is disseminated in the economy.
Our data source is PatStat, a comprehensive resource from the European Patent Office covering more than 170 publication authorities (patent offices), 88 million awarded patents, 160 million citations, and more than 200 control variables covering the period from 1850 to 2018. In this article, we consider the four primary PatStat technology sectors (mechanical engineering, chemistry, electrical engineering, and instruments), resulting in 32 fields (details are provided online, see Appendix 1 at https://dl.acm.org/doi/10.1145/3377476).c,d Our analysis is based on a simple count-data model of the number of citations received by each patent, controlling for several confounding factors that may influence our estimates. The basic model is the following:
Ci is the sum of all citations received by patent i, Fkt is a binary variable equal to 1 for patents that belong to field k and were published in year t, and 0 otherwise. This model reports estimators at the field-year level conditional on a set of controls. These controls are included in Xi, the vector of patent characteristics, and εi is the error term. βkt captures the number of citations received by each field and year, all other things being equal. Our analysis is done at the patent level allowing for maximum degree of flexibility in the estimates.
Given our analytic interest in instrument and electrical engineering technologies, we exploit the fact that patents can be classified to multiple patent sectors. Building upon the growing impact of electrical engineering patents after the 1970s (see Figures 1 and 2 and Koutroumpis et al.17), we use a differences-in-differences model to measure the change in spillovers to other sectors that originate from instruments before and after 1970. We construct Sik as the sum of citations that originate from sectors j other than k (k ≠ j), with Sik = Σk≠j Cik < Ci, where Ci is the sum of all citations received by patent i. The simple model from Eq. 1 now becomes:
Figure 1. Total patents, citations, and spillovers by technology sector (1920–2010).
Figure 2. Patent citations by sector, field, and year.
where FSector,t takes the values Sector = [electrical, mechanical, chemical], Fsector,t ∗ FInstruments,t is a binary variable equal to 1 for patents that list technology sectors in electrical, mechanical or chemical and instruments sectors published in year t, and 0 otherwise. The Postyear=1970 binary variable is 1 for years after 1970 and 0 otherwise. The interaction of Postyear=1970 by the selected FSector,t ∗ FInstruments,t measures the post 1970 effect in the treated groups. We report estimators at the field-year level using the same set of controls included in Xi, the vector of patent characteristics including tech sector fixed effects. εi is the error term and βkt reflects the number of citations received by each field and year, all other things being equal.
There are several factors that may influence patent citation counts. First, the number of citations is linked to the procedures followed by publication authorities (national or regional patent offices) that oversee the application and grant process. This can change over time as new processes within the patent offices affect the ways they attribute citations. Second, prior art citations have been rising in recent years thus introducing a secular trend. We therefore control for year and patent office effects allowing direct comparisons across jurisdictions and over time. Third, a patent may belong to a family of inventions that are submitted to multiple patent offices. The size of such a patent family can affect the visibility of the invention and hence increase the likelihood of the patent being cited. We compute and control for the numbers of patents that belong to each family and each “extended” family.e Fourth, technology sectors have varying publication and citation patterns. We control for the total number of inventions granted (annual patent flows) and the total number of citations within each patent class each year. These metrics correct for potentially inflated citation counts in sectors with more inventions (hence with a higher likelihood of being cited) and sectors that cite patents and non-patent literature more extensively than others. Further, we control for the citations made by patent examiners and the number of claims to capture the extent and scope for protection sought. Lastly, we capture seasonal effects with controls for the month of publication.
All these controls reassure us about the validity of comparisons over time, across patent offices, and across technology fields. Our assumption is that patents submitted in a patent office, at the same time, within the same field, and with the same family size will be treated equally by the authorities. Given that recently published patents have a shorter window of observations, to avoid a systematic bias, we only consider results with a varying cut-off year ranging from 2005 to 2018 in our analysis. (In the online appendix, Figure 1 presents the average number of citations received by all patents in the years after publication; https://dl.acm.org/doi/10.1145/3377476). We also only focus on priority patents (the patent with the first application filing date) and attribute all citations for subsequent applications (across patent offices) to them. This latter choice along with the increasing patenting activity in recent years—for which we explicitly control—reduces our sample to approximately 54 million (from 88 million in total).f
Results
Relative influence. To understand the overall long-term pattern of knowledge spillovers via prior-art citations of patented inventions, we first look at the spillovers from all technology sectors (but not within technology sector) across 10 decades, from the 1920s to the 2010s.g We present these results in Figure 1.
Panel A in Figure 1 presents the total patents by technology sector and illustrates the growth of patents over the past century. It clearly shows, with the exception of the 2000s, that the most common patents awarded are mechanical engineering technologies, ranging from 422,504 in the 1920s to 4,623,680 in the 2010s. Panel B in the figure presents the total citations by technology sector and illustrates that mechanical engineering technologies were the most cited patents up until the 1970s, when electrical engineering technologies became dominant. Citations of electrical engineering patents rose from 501,283 the 1970s to peak at 8,138,699 in the 2000s. Panel C in the figure presents the total cross-sector spillovers (that is, excluding the same sector citations) by technology sector and illustrates that mechanical engineering technologies were the main source of sectoral spillovers up until the 1970s, when instrument technologies (rising from 5,365 to 24,674 in the 2010s) and electrical engineering technologies (rising from 10,651 to 28,856 in the 2000s), became the main source of cross-sector spillovers. Put differently, Figure 1 highlights a shift from mechanical and chemical technologies to electrical and instrument technologies as the main source of cross-sector spillovers since the 1970s.
We now look at the relative influence of the four primary technology sectors, namely instruments, electrical engineering, mechanical engineering, and chemistry (Figure 2). Although the four technology sectors display distinct citation profiles, the pattern changes for all of them in the early 1970s.h As anticipated, we observe that instruments are the most widely cited sector both within and across other industrial activities, exhibiting the highest mean numbers of citations per patent for the entire period. The most striking changes within instrument technologies appear to both measurement and medical instruments, which consistently receive more citations than the mean of all sectors for the entire period of study. Also of interest are control instruments that become more influential after the 1970s. Control instruments typically relate to the manipulation and management of machinery.
For electrical engineering technologies, we observe that prior to 1970 this technology sector follows the mean, after which it begins to increase more rapidly, peaking just after the 2000s. Within electrical engineering, most technology fields are above the mean post 1970, except for basic communication processes. Of note are the fields of computer technology, digital communication, audiovisual technology and telecommunications, which exhibit significant spillovers. Considering the other technology sectors, while mechanical engineering was consistently above the trend prior to 1970, and chemistry technology sectors closely followed the general trend, soon after 1970 their influence starts to decline to below trend.
Figure 3 presents the spillovers for all patent technologies from the 1950s to the 2000s.i This shows the direction and size of the relative cross-sectoral citations. As the number of patents increases significantly with time, so do the level of spillovers across decades. For example, the maximum spillovers from a technology field range from 17,642 in the 1950s to 513,275 in the 2000s. We clearly show over this time period a shift from mechanical and chemistry technologies as the main source of cross-sector spillovers to electrical and instrument technologies, with the effect beginning in the 1970s.
Figure 3. Spillovers for all patent technology fields by decade, 1950–2010.
Taken together, the patterns presented in Figures 1,2,3 correspond to a shift from mechanical and chemical technologies to electrical technologies. They are also aligned with findings by Jovanovic and Rousseau15 and Koutroumpis et al.17 regarding the ICT revolution commencing about this time.
Cross-sector and cross-field spillover effects. Table 1 presents our regression analyses of the technology sectors that instrument patents are co-listed with. We define as “digital instruments” those patents that list both electrical engineering and instrument sectors, and “mechanical instruments” those patents that list both mechanical engineering and instrument sectors. Similarly, “chemical instruments” list both chemistry and instrument sectors.
Table 1. Spillovers for instruments cross-referenced with other patent sectors, before and after 1970.
We find that instrument technologies appear to have generated substantial and sustained knowledge spillovers over several decades regardless of the underlying technological base. Overall, chemical instruments (coefficient 1.632) are the most influential, followed by mechanical instruments (1.394) and digital instruments (1.320). When we consider citation patterns after 1970 (X Post), more specifically considering the ICT revolution, we find that spillovers from digital instruments increase by 0.388 citations after 1970 (column 1), while those from mechanical instruments (-0.034) and chemical instruments (-0.080) drop after 1970 (columns 2 and 3). This suggests that instrument technologies have become increasingly digitized post 1970.
Given the important inflection point of 1970 in the transition to digital technology-based instruments, we now focus on post 1970 spillovers and investigate how each instrument field interacts with the main sectors, namely, electrical engineering, chemistry, and mechanical engineering technologies. Table 2 presents these coefficients.
Table 2. Spillovers from instrument technology fields (sub-sectors) interacted with other patent sectors after 1970.
Here we again see the confluence of electrical engineering with instrument technologies, with electrical engineering strongly co-listed with optical (0.242) and measurement (0.212) instruments (columns 1 and 2). These results are suggestive of digital sensor technologies. However, we also find positive effects for optical instruments (column 1) co-listed in the chemistry sector (0.306), suggestive of laser technologies, and for medical technology instruments (column 5) co-listed in the chemical (0.838) and mechanical engineering (1.012) sectors.j
Given the importance of electrical engineering to instruments post-1970 (Table 2), we carry out a similar analysis of the fields of the electrical engineering sector. Table 3 reports the interactions of the electrical engineering fields with the instrument fields post 1970. Of note is the strong interaction of optical instruments (column 1) with all electrical engineering fields except for basic and digital communication. Table 3 also shows strong interaction of measurement instruments (column 2) with semiconductors (0.246), electrical machinery (0.245), computer technology (0.129), and basic communication (0.113). Both are highly suggestive of the technologies that comprise the digital sensors that typify industrial connected devices. There is also evidence of the digitization of medical instruments (column 5) with the strong interaction of electrical machinery (1.368) and computer technology (0.512), and the digitization of control systems (column 4), with a strong interaction effects with IT management methods (0.443) and computer technology (0.157).
Table 3. Spillovers for instrument technology fields interacted with electrical engineering technology fields after 1970.
Conclusion and Limitations
To investigate the hypothesized spillover effects of instrument and information technologies,2,16,22 we analyzed the entire global history of patenting from 1850 to 2018 to detect long-term patterns of knowledge spillovers via prior-art citations of patented inventions. We found that information and instrument technologies generate the most substantial and widespread spillovers of knowledge used in other technology fields. For this reason, we call them “invention machines.” The greatest spillover impact is generated by digitized optical and measurement instruments.
Digital instruments form the technological base of industrial connected devices, such as smart buildings (including “smart” lighting, heating, ventilation, and air conditioning and physical security systems), process sensors for manufacturing, and real-time location and sensing devices for healthcare. Digital instruments also comprise the sensors a standard smartphone has—accelerometer, gyroscope, magnetometer, GPS, barometer, proximity sensors, and ambient light sensors—without which many of the functions of the phone cannot work.k There were approximately 8.4 billion of these types of connected devices in 2017,l and by 2020, it is estimated there will be between 30 to 50 billion connected devices.19
Thus, we argue the convergence of digital technologies and instrument technologies is likely to bring about the next generation of invention machines. Advanced digital communications make it possible to simultaneously and immediately utilize information in a wide variety of contexts. As such, digital instruments will allow the observation and manipulation physical, chemical, biological, and social processes in connected industrial activities in a vast set of contexts. One can view these technologies as key enablers for the “Second Machine Age” vision of the future of Brynjolfsson and McAfee.7 Therefore, we suggest the emergence and adoption of the digital instruments in coming years is likely to generate a flurry of invention in many if not most technology fields.
The increasing reach of digital instruments and knowledge spillovers will potentially speed up the rate of invention both within industries and within firms. As the onslaught of networked automation may continue to create industrial value but also societal upheaval via creative destruction of jobs, occupations, and organizations, it is interesting to note that the set of technologies that fundamentally enables this, instruments, has gone relatively unnoticed in the economics and management of technology.
Despite the volume of data analyzed, this study is subject to several limitations. First, patents represent only a subset of technological knowledge that may also appear in other forms and channels including non-patent literature, tacit or organizational knowledge, open innovation, and software. Capturing these links would strengthen the interpretation of spillovers and could also help explain or even predict the launch of new inventions and technologies. We consider this along with a parallel research of academic and open source software a promising area of future research.
Second, we can identify influential patents ex-post but have not created a robust method that predicts the new patents that will appear. One approach for this could combine the spectrum of possible applications of a patent using our baseline specification and machine-learning techniques to analyze a very granular dataset.
Third, there is a long literature linking the inventor capacity, networks, legislation and resource allocation to the subsequent success of inventions.4,16,20,23 Our work has not utilized this type of information to predict the commercial success of various inventions, and we believe some fruitful research can be undertaken here.
Acknowledgments. This work was supported by EPSRC project EP/K039504/1 and the EIT ICT Labs. We thank Paul Romer, Shane Greenstein, Eric Brynjolfsson, Chris Forman, Kristina McElheran, Frank Nagle, Avi Goldfarb, Achim Luhn, the NBER Digitization workshop participants (2016) and the Academy of Management Big Data Symposium (2016) participants for their comments.
Join the Discussion (0)
Become a Member or Sign In to Post a Comment