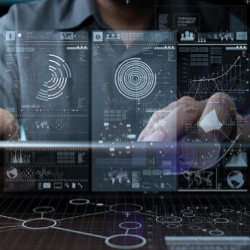
The healthcare system in Latin America (LATAM) has made significant improvements in the last few decades. Nevertheless, it still faces significant challenges, including poor access to healthcare services, insufficient resources, and inequalities in health that may lead to decreased life expectancy, lower quality of life, and poor economic growth.
Digital Healthcare (DH) enables the convergence of innovative technology with recent advances in neuroscience, medicine, and public healthcare policy.a In this article, we discuss key DH efforts that can help address some of the challenges of the healthcare system in LATAM focusing on two countries: Brazil and Mexico.
We chose to study DH in the context of Brazil and Mexico as both countries are good representatives of the situation of the healthcare system in LATAM and face similar challenges along with other LATAM countries. Brazil and Mexico have the largest economies in the region and account for approximately half of the population and geographic territory of LATAM.11
Brazil and Mexico each have a government-run public healthcare system that is universal in Brazil and fragmented in Mexico. Most Brazilians and Mexicans depend on the public healthcare system exclusively, and those who can afford it often pay for private health insurance. The demographics of Brazil and Mexico pose significant challenges to health. While life expectancy has experienced a significant increase in recent years, unhealthy lifestyles, poor environmental health, and low adherence to treatments have shifted causes of mortality toward chronic diseases. Thus, Brazil and Mexico are experiencing an increasing demand for uncompensated informal care that is higher than what is reported in developed countries;15 lower diagnostic and treatment costs are urgently needed.
Research efforts on DH in Brazil and Mexico involve a diversified composition of activities ranging from telehealth to mathematical modeling. Here we discuss several research projects showcasing how DH, using major computing science paradigms, may enhance the efficiency of healthcare delivery in a LATAM context.
Healthcare Analytics
Although healthcare analytics is a global trend,14 in LATAM it gains particular relevance given its specificities in demographic and socioeconomic aspects and in the adopted healthcare system. Healthcare analytics enables different projects tailored for the LATAM reality, such as the evaluation of public healthcare policies, big data analytics for public health research, or the data-driven analysis of the healthcare system.
A young child undergoing music therapy using Bendable Sound.
An example of an ongoing project in Brazil for the evaluation of public healthcare policies is the assessment of the impact of hospital-based breastfeeding interventions on infant health. Brazil has over three million births per year, 10% of which are premature births.b About 25% of the Brazilian hospitals implement at least one of the three initiatives that promote breastfeeding in maternal hospitals. This on-going project,3 led by the Institute of Scientific and Technological Communication and Information in Health/Fiocruz with the National Laboratory for Scientific Computing (LNCC) and CEFET-RJ as partners, assesses the impact on neonatal mortality of breastfeeding programs (alone or in combination), in particular considering that breastfeeding offers newborns increased protection against infectious diseases.2 This assessment is performed using spatial and temporal analyses that integrate epidemiological, statistical, and data science tools. To that end, the project analyzes more than 60 million deliveries and about 320,000 neonatal deaths in maternity hospitals in Brazil for over 20 years to better understand the cost-effectiveness of the combined adoption of these public policies promoting breastfeeding. The results thus help define integrated guidelines for the future implementation of these initiatives.
Healthcare analytics, pervasive healthcare, and artificial intelligence solutions have lowered diagnostic and treatment costs to enhance the efficiency of the healthcare delivery in LATAM.
Another ongoing project in Brazil focuses on data-driven analysis and it involves the evaluation of patient trajectories through the healthcare system by using time-varying graphs and process mining tools.12,13 To that end, this project, involving partner researchers from LNCC, the Federal University of Juiz de Fora, and the Brazilian Ministry of Health, comprises data of almost 60 million healthcare consultations or health procedures delivered to about 6.5 million unique patients in the city of São Paulo over two years (2014-2015). In a case study, the project analyzed the trajectories through the healthcare system of almost 25,000 pregnant women. The results promote understanding of how the system is being used, its bottlenecks, and where they emerge, thus offering knowledge to build upon for better management and resource allocation.
Research using data-driven analysis and mathematical models to analyze epidemiological data has also been active in Mexico. One ongoing project led by the Institute of Research in Applied Mathematics and Systems at the National Autonomous University of Mexico (UNAM), including collaborations with the Mexican Ministry of Health, has explored the use of time-dependent epidemiological models to make reliable predictions on the evolution of influenza6 and the COVID-19 pandemics. Results indicate the potential of the sanitary measures in reducing outbreaks.
Overall, these projects contribute to evaluating public healthcare policies to eventually cope with healthcare disparities that are common in LATAM due to an unequal distribution of economic income and assets.
AI for Healthcare
Artificial intelligence (AI) has shown a growing impact in key categories of healthcare mainly involving the screening and the early detection of different health disorders. Screening is a strategy adopted by health authorities in order to identify a health problem at initial stages and enable early intervention. However, current methods used as the gold standard for screening present multiple limitations including high false positive classification rate or using data that is subject to clinicians’ interpretation and often inaccurate and biased. Furthermore, due to having limited equipment in LATAM hospitals, specialized exams for screening including imaging exams, like mammography, magnetic resonance (MR) or computed tomography (CT) are hardly available; so there is a huge need for alternative low-cost exams.
Moving in this direction, a research project, developed by VisualLab at Fluminense Federal University (UFF), proposes thermography image processing using AI techniques for breast cancer screening. Breast cancer is the leading cause of cancer deaths among women in Brazil and Mexico, reaching almost 30% of cancer deaths in both countries. Since cancerous tissue temperature is generally higher than healthy surrounding tissues, thermography has been considered a promising screening method for breast cancer detection, by generating images that reveal the heat distribution on the skin surface.9 VisualLab has developed image analysis methods to assess the risk of breast cancer, using dynamic infrared thermography,16 a method for monitoring the dynamic response of skin temperature after thermal stress. Breast images of UFF’s University Hospital patients were analyzed to build time series. Those temperature time series are then clustered by the k-means algorithm and evaluated by clustering validation indices. Indices values are treated as features and submitted to a feature selection step. As the last step, classification is performed considering the feature vector for each patient. The method was tested with 1,400 images from 70 different patients (35 healthy and 35 with breast anomalies) acquired with a FLIR SC620 thermal camera. Among several classifiers, the best results were achieved by K-Star, obtaining 98.57% accuracy using tenfold cross-validation.17
The Neuroimaging Laboratory at Universidad Autónoma Metropolitana-Iztapalapa, a leading group on image and signal processing for healthcare in Mexico, has also shown how image processing can be used for the screening and monitoring of cognitive decline by identifying anatomical and functional connectivity of the brain from electroencephalogram recordings.1
As deep learning makes inroads in medical image processing, being able to explain the results of a machine learning algorithm—a field referred to as explainable AI, or XAI—becomes paramount and has become an important trend for DH systems. For example, an ongoing project, developed by MídiaCom Lab at UFF, involves the use of AI for early detection of patients with mild cognitive impairment (MCI)4 to promote early interventions and avoid dementia. As patients attending public hospitals in Brazil usually do not have MR or CT imaging exams, the proposed dynamic decision model uses clinical data, including symptoms and neuropsychological tests. Periodically an ML process is run and evaluates different kinds of AI techniques, combining discretization and balancing methods applied to the available patient dataset, in order to select an updated decision model with the best performance. The model was trained and tested with 319 patients from the Center for Alzheimer’s Disease at the Federal University of Rio de Janeiro, and the Center for Attention to Health of the Elderly at UFF, achieving 94% accuracy. In addition to indicating the diagnostic probability, the proposed decision model also informs the physician about which test results have most influenced the system decision and which additional tests can be made to increase diagnostic probability.
Such projects illustrate how ML and image processing can be used for early, low-cost non-invasive breast cancer, Alzheimer’s, and MCI screenings in LATAM. The projects from Brazil are part of the research network of the National Institute of Science and Technology in Medicine Assisted by Scientific Computing, covering a diverse set of activities related to DH.
Pervasive Healthcare
For more than a decade, pervasive healthcare (PH) research in LATAM has focused on the development and pilot-testing of pervasive sensing solutions, natural user interfaces, and remote care management. Several researchers have proposed mobile and wearable sensing platforms, involving either user or environment instrumentation, to collect patient data that is relevant for clinical case assessment and could be used to train machine learning models for the early detection and diagnosis of different disorders and diseases. A recent trend is to use digital markers to quantify physiological and behavioral data collected through wearable and mobile sensing, or through the tracking of interaction patterns with digital devices.
One example is an ongoing project from a Mexican autism network that has deployed the first living laboratory10 actively using PH technology in the everyday activities of a specialized school-clinic. The network is currently being led by the Ensenada Center for Scientific Research and Higher Education (CICESE) and includes academic institutions like Centro de Enseñanza Técnica Y Superior University and UNAM, and different public and private schools located in northwest Mexico. This project explores if elastic displays can uncover force-related gestural interactions that can be used as a digital marker to support autism screening.
The elastic display BendableSound, developed at the Mobile and Ubiquitous Computing Lab at CICESE-Mexico, runs a 3D background of space-based elements that play sounds when users touch them.5 A study of children with autism and neurotypicals who used BendableSound uncovered atypicalities in the amount of force and gestural interactions used by children with autism. Machine learning models using the more dominant features and the more relevant gestures show good performance in classifying children with autism from neurotypicals with 97.3% of precision and recall.
Socially assistive robots are interactive, intelligent systems that employ hands-off social interaction strategies, including the use of speech, facial expressions, and communicative gestures, to assist in a particular healthcare context.
Other research initiatives in LATAM have proposed the use of telemedicine, social media, and crowdsourcing for remote care management, as Brazil and Mexico have large territories with remote rural and unplanned metropolitan areas that are hard to reach. This research shows how PH can be used to improve patient-clinician communication and support remote patient monitoring. Telehealth consultations, whose regulation faced a long history in Brazil and Mexico, was suddenly regulated in Brazil and was being offered to those socially isolated at home during the pandemic of 2020.c
PH research in LATAM has also been actively studying the use of natural user interfaces to support therapeutic interventions. Solutions range from the use of brain-computer interfaces to the use of gesture therapy,18 a term coined at the Robotics Lab at the National Institute for Astrophysics, Optics, and Electronics to reduce the gap between cost and accessibility, lowering therapeutic costs and lessening the burden of informal caregivers in LATAM.
A success story is the use of socially assistive robots (SARs) to assist in the care of older adults with dementia. SARs are interactive, intelligent systems that employ hands-off social interaction strategies, including the use of speech, facial expressions, and communicative gestures, to assist in a particular healthcare context.
The robot Eva, developed at the Mobile and Ubiquitous Computing Lab at CICESE-Mexico, is an open source SAR using conversational strategies for people with dementia as proposed by organizations such as the Alzheimer Association.7 Eva relies on knowledge about the user, including their capabilities and preferences, to personalize and guide cognitive stimulation therapy using activities such as music, reminiscence, cognitive games, and relaxation. It incorporates new knowledge from the user after each session of use. While following a somewhat limited, but personalized script, the robot can autonomously lead a therapy session with minimal conversation breakdowns. The robot has been evaluated with individuals with mild to moderate dementia for the last three years in a geriatric residence. The results show older adults with dementia learn to interact with Eva with no previous training in one or two sessions. After nine weeks of using Eva, older adults significantly decreased their neuro-psychiatric symptoms associated with dementia and increased their quality of life.8 Some of the technical challenges facing the widespread use of social robots for dementia include developing proper adaptation strategies based on disease progression and inferencing user mood. In addition, the continuous use of a conversational agent could be used to assess the course of the disease and the effectiveness of interventions through paralinguistic or verbal analysis.
These efforts represent projects showing PH can make digital healthcare solutions increasingly available in the homes, geriatric residences, and in the school-clinics in LATAM.
Challenges and Trends
DH research is representative in Mexico and Brazil due to the particularities of their demographics, culture, economic, and geographic territory. In this article, we showed how healthcare analytics, AI, and PH may improve their healthcare system. Here we discuss major challenges as a springboard for a new focus on DH in LATAM.
Health data collection is very challenging and expensive. The LATAM context imposes significant shortcomings to collect and maintain large public datasets with consistent and reliable information. Current key challenges involve the homogenization of diverse levels of data quality and accuracy as well as the integration of data fragments from diverse sources. The key to showing the current value of such large datasets involves the challenging process of converting big data into valuable insights which may be limited due to the low number of cases, class imbalance, and nonuniform misclassification in current AI models. Risks associated with the tensions of collecting data in the lab versus in naturalistic conditions have resulted in solutions divorced from practical applications when working with real-world scenarios resulting in poor performance due to using unreliable data.
The feasibility of the collection of health data during everyday activities will only be possible through the use of innovative technology that individuals are using on a daily basis. This opens up opportunities for the creation of natural and intuitive forms of interaction and innovative devices to ease data gathering and the analysis of large datasets in naturalistic conditions. Development challenges involve exploring novel input and output mechanisms through custom-built wearable and sensing devices, the use of natural user interfaces to measure novel gestural interactions, and techniques to indirectly infer health data. In LATAM, healthcare clinics and hospitals have limited equipment, making it difficult to deploy innovative technology without an appropriate information technology backbone; understanding what infrastructure and architectures are needed to use built-in sensors and innovative technology will help healthcare providers deal with troubles of upscaling and scalability.
Moreover, general DH solutions have not been developed to treat the diverse healthcare needs of Latin American citizens. Most DH solutions have typically offered a “one-size-fits-all” solution in which personalization of healthcare services to the particular needs and capabilities of its patients has been largely neglected. A current trend in AI involves the development of predictive and adaptive decision models to be used in different healthcare scenarios. Applying a particular model to a different set of data will not be able to adapt itself; however, one of the main drawbacks of these models is that they do not take into account the context of its use and of that of their users.
Descriptions of the development and empirical study of DH in concrete scenarios are scarce and urgently needed. The actual deployment of DH in the healthcare system infrastructure poses a variety of social, cultural, legal, and policy-based issues. The area’s average investment in healthcare is below that of developed countries.d In particular, clinical and computing methods for the development and evaluation of the impact of DH solutions in concrete scenarios are also different. The low number of participants enrolled in pilot studies and the lack of replication studies have also reduced the possibilities of convincing clinicians and the pharmaceutical industry to invest in transforming DH solutions that come out of research projects into commercial products, and are also limiting research in healthcare analytics and AI.
At the time of writing, Brazil and Mexico, like the rest of the world, were facing the outbreak of the novel coronavirus (SARS-CoV-2). At the center of the resulting health, economic, and social crisis, an urgent call for actions on the application of information and communication technologies to healthcare has emerged, in addition to the more obvious demands for research on epidemiological surveillance and models, drug and vaccine development, and multi-omics analysis. Some initiatives are investigating the feasibility of detecting COVID-19 through AI-based lung image analysis or how AI may accelerate the process of in silico drug design. These are just some examples out of many possible ones. Despite the hard outcomes, this crisis has put computing applied to healthcare in the front line, overcoming a tipping point and boosting DH in Brazil and Mexico, with innovative technology playing a key role in enabling better and low-cost healthcare services and assistance.
Acknowledgments
M. Tentori thanks the support of CONACYT and MSR. A. Ziviani thanks the support of CNPq and FAPERJ. D.C. Muchaluat-Saade thanks the support of CNPq, FAPERJ, INCT-MACC and CAPES PRINT. J. Favela thanks the support of CONACYT.
Join the Discussion (0)
Become a Member or Sign In to Post a Comment