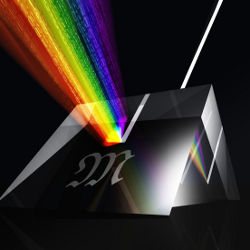
In 2006, AOL released a file containing search queries posed by many of its users. The user names were replaced with random hashes, though the query text was not modified. It turns out some users had queried their own names, or “vanity queries,” and nearby locations like local businesses. As a result, it was not difficult for reporters to find and interview an AOL user1 then learn personal details about her (such as age and medical history) from the rest of her queries.
Could AOL have protected all its users by also replacing each word in the search queries with a random hash? Probably not; Kumar et al.27 showed that word co-occurrence patterns would provide clues about which hashes correspond to which words, thus allowing an attacker to partially reconstruct the original queries. Such privacy concerns are not unique to Web-search data. Businesses, government agencies, and research groups routinely collect data about individuals and need to release some form of it for a variety of reasons (such as meeting legal requirements, satisfying business obligations, and encouraging reproducible scientific research). However, they must also protect sensitive information, including identities, facts about individuals, trade secrets, and other application-specific considerations, in the raw data. The privacy challenge is that sensitive information can be inferred in many ways from the data releases. Homer et al.20 showed participants in genomic research studies may be identified from publication of aggregated research results. Greveler et al.17 showed smart meter readings can be used to identify the TV shows and movies being watched in a target household. Coull et al.6 showed webpages viewed by users can be deduced from metadata about network flows, even when server IP addresses are replaced with pseudonyms. And Goljan and Fridrich16 showed how cameras can be identified from noise in the images they produce.
Key Insights
- Data snoopers are highly motivated to publicize or take advantage of private information they can deduce from public data.
- History shows simple data anonymization and perturbation methods frequently leak sensitive information.
- Focusing on privacy design principles can help mitigate this risk.
Naive aggregation and perturbation of the raw data often leave exposed channels for making inferences about sensitive information;6,20,32,35 for instance, simply perturbing energy readings from a smart meter independently does not hide trends in energy use. “Privacy mechanisms,” or algorithms that transform the data to ensure privacy, must be designed carefully according to guidelines set by a privacy definition. If a privacy definition is chosen wisely by the data curator, the sensitive information will be protected. Unfortunately, privacy definitions are not one-size-fits-all. Each application could have its own unique privacy requirements. Working independently, researchers from disparate fields rediscover similar privacy technologies, along with their weaknesses, new fixes, and other vulnerabilities. Our goal here is to synthesize some of the latest findings in the science of data privacy in order to explain considerations and best practices important for the design of robust privacy definitions for new applications. We begin by describing best practices, then explain how they lead to a generic template for privacy definitions, explore various semantic privacy guarantees achievable with this template, and end with an example of a recent privacy definition based on the template and apply it to privacy-preserving k-means clustering.
Desiderata of Privacy Definitions
When data is collected, the curator, with the aid of a privacy definition, puts it in a form that is safe to release. A privacy definition is a specification for the behavior of randomized and deterministic algorithms. Algorithms that satisfy the spec are called privacy mechanisms. The curator first chooses a privacy definition, then a privacy mechanism
satisfying the definition. The curator will run
on the sensitive data, then grant external users access to the output of
, or the “sanitized output.”
There is a long history of proposed privacy definitions, new vulnerabilities discovered, and amended privacy definitions developed only to be broken once again. As privacy concerns spread, parallel copies of this process are spawned in many research areas. Fortunately, current research has identified many best practices for engineering robust privacy protections for sensitive data. Although they can be formalized in a mathematically rigorous way, we present them at a more intuitive level, leveraging the following privacy definitions as sources of examples.
Definition 1 (∈-differential privacy9,11). An algorithm
satisfies ε-differential privacy if for each of its possible outputs ω and for every pair of databases D1, D2 that differ on the addition or removal of a single record,
.
Intuitively, ε-differential privacy guarantees that adding or removing a record from the data will have little effect on the output of a privacy mechanism
. For small ε, it means
will probably produce the same sanitized output regardless of whether or not Bob’s record is in the data.
How should a data curator choose ε? Consider a highly targeted query about an individual (such as asking if Bob’s record is in the data). For ∈-differential privacy, the most revealing privacy mechanism answers truthfully with probability eε/(1 + eε) and falsely with probability 1/(1 + eε).24 When ε is close to 0, both these probabilities are close to ½, and little information is provided; the mechanism is almost as likely to lie as respond truthfully; for example, when ε = 0.1, the true answer probability is ≈ 0.525, and when ε = 0.01, the probability is ≈ 0.502. We recommend choosing ε based on how close the curator wants this value to be to ½.
The Laplace mechanism is a popular mechanism for ε-differential privacy. Let f be a function that computes a vector of query answers on the data. To each query answer, the Laplace mechanism adds an independent Laplace random variable with mean 0 and standard deviation
, where S(f) is the global sensitivity of f – the largest possible change in f due to the addition of one record, or the maximum of ||f(D1) − f(D2)||1 over pairs of databases D1, D2 that differ in one record. Intuitively, the noise masks the influence of any single record on the result of f. Now consider:
Definition 2 (k-anonymity.34,35) Given a set Q of attributes, known as the quasi-identifier, a table is k-anonymous if every record in it has the same quasi-identifier values as k–1 other records. An algorithm satisfies k-anonymity if it outputs only k-anonymous tables.
K-anonymity defends against one type of attack called a “linkage attack”—joining an external dataset that associates an identity (such as name) with the quasi-identifier (such as ZIP code and age) to a k-anonymous table containing this publicly available quasi-identifier. Its goal is to prevent the matching of an identity to a single tuple in the k-anonymous table; clearly, there will always be at least k candidates in the join result. K-anonymity mechanisms usually operate by coarsening attributes (such as dropping digits from ZIP codes and changing ages to age ranges); see Figure 1 for two examples of k-anonymous tables.
Security Without Obscurity
The process of sanitizing sensitive data through a privacy mechanism
must follow Kerckhoffs’s principle21 and ensure privacy even against adversaries who might know the details of
, except for the specific random bits it may have used. Better yet, the mechanism
must be revealed along with the sanitized output.
The reasons for making the mechanism
publicly known are twofold: First, history shows “security through obscurity” is unreliable in many applications; and, second, the output of
must be useful. This sanitized output often takes the form of a dataset or statistical model and could be intended to support scientific research. In such a case, statistical validity is an important concern, and statistically valid conclusions can be drawn only when scientists know precisely what transformations were applied to the base sensitive data.
Likewise, privacy-mechanism designers should always assume attackers are smarter than they are. Just because the designer of a privacy mechanism cannot deduce sensitive information from the output of a piece of software, an adversary will also fail. A well-engineered privacy definition will overcome these disadvantages, protecting sensitive information from clever attackers who know how
operates. We explain how in subsequent sections.
Post-processing. A privacy definition determines the mechanisms that data curators can trust to not leak sensitive information. Let
be one such mechanism, and suppose
is some algorithm that can be applied to the output of
; for example, suppose
creates synthetic data from its inputs, and
builds a decision tree. Let the notation
denote the composite algorithm that first applies
to the sensitive data and then runs
on the sanitized output of
.
If
is trusted, should this composite algorithm
also be trusted? Intuitively, the answer is yes. It would be very strange if a data curator released privacy-preserving synthetic data but then claimed building statistical models from this data is a violation of privacy.
A privacy definition is closed under post-processing if
satisfies the constraints defining the privacy definition whenever
does. Differential privacy11 satisfies this property, but k-anonymity does not.23 Closure under post-processing has two important consequences: First, it ensures compatibility between a privacy definition and Kerckhoffs’s principle; for example, some algorithms that satisfy k-anonymity are susceptible to a so-called minimality attack.13,36 For each such k-anonymity mechanism
, it is possible to craft a post-processing algorithm
that takes the output of
, undoes some of its data transformations, and outputs a new dataset having records with possibly unique quasi-identifier values that are vulnerable to linkage attacks with external data. That is, the composite algorithm
does not satisfy the same conditions as
, or k-anonymity, and often reveals sensitive records.
By contrast, suppose an ε-differentially private algorithm
is applied to the data D, and the result
(D) is published. Given knowledge of
, a clever adversary can design an attack algorithm
and run it on the published data to obtain the result
(
(D)). Note
(
(D)) is the result of applying the composite algorithm
to the data D. Since ε-differential privacy is closed under post-processing, the composite algorithm
still satisfies ε-differential privacy and hence has the same semantics; the output of
is barely affected by the presence or absence of Bob’s (or any other individual’s) record in the database.
The second important consequence of closure under post-processing is how a data curator must express privacy definitions. Consider k-anonymity and ε-differential privacy. By analogy to database-query languages, the definition of k-anonymity is declarative; that is, it specifies what we want from the output but not how to produce this output. On the other hand, differential privacy is more procedural, specifying constraints on the input/output behaviors of algorithms through constraints on probabilities (such as P(
(D) = ω)). This is no coincidence; in order to achieve closure under postprocessing, it is necessary that the privacy definition impose conditions on the probabilities (even when
is deterministic) rather than on the syntactic form of the outputs.22
Composition. We introduce the concept of composition with an example. Suppose the 4-anonymous table in Figure 1 was generated from data from Hospital A, while the 3-anonymous table in Figure 1 was generated by Hospital B. Suppose Alice knows her neighbor Bob was treated by both hospitals for the same condition. What can Alice infer about, say, Bob’s private records? Bob corresponds to an anonymized record in each table. By matching ZIP code, age, and disease, Alice can deduce that Bob must have had a stroke. Each anonymized table individually might have afforded Bob some privacy, but the combination of the two tables together resulted in a privacy breach. The degradation in privacy that results from combining multiple sanitized outputs is known as “composition.”14
Self-composition. “Self-composition” refers to the scenario where the sanitized outputs are all produced from privacy mechanisms that satisfy the same privacy definition. Fundamental limits on a privacy definition’s ability to withstand composition are part of a growing literature inspired by the results of Dinur and Nissim7 who showed that the vast majority of records in a database of size n can be reconstructed when n log(n)2 statistical queries are answered, even if each answer has been arbitrarily altered to have up to o(
) error; that is, a distortion that is less than the natural variation of query answers that an adversary would get from collecting a sample of size n from a much larger population.
The privacy challenge is that sensitive information can be inferred in many ways from the data releases.
Despite such negative results that limit the number of times a private database can be queried safely, there can be a graceful degradation of privacy protections, as in the case of ε-differential privacy. If
1,
2, …,
k are algorithms such that each
i satisfies εi-differential privacy, then the combination of their sensitive outputs satisfies ε-differential privacy with ε = ε + … + ε;30 more formally, this privacy level is achieved by the algorithm
running mechanisms
1,
2, …,
k on the input data and releases all their outputs. The end result thus does not reveal any record deterministically while still satisfying differential privacy but with a linear degradation in the privacy parameter.
Self-composition has another practical benefit—simplifying the design of privacy-preserving algorithms. Complicated mechanisms can be built modularly from simpler mechanisms in the same way software is built from functions. By controlling the information leakage of each component individually, a privacy-mechanism designer can control the information leakage of the entire system. In the case of ε-differential privacy, the privacy parameter ε of the final mechanism is at most the sum of the privacy parameters of its components.4,30
Composition with other mechanisms. The data curator must also consider the effect on privacy when the mechanisms do not satisfy the same privacy definition. As an example,24,26 consider a database where each record takes one of k values. Let x1, x2,…, xk denote the number of times each of these values appears in the database; they are histogram counts. Let
1 be a mechanism that releases the sums x1 + x2, x2 + x3, …, xk–1 + xk. Note
1 does not satisfy ε-differential privacy. Moreover, the knowledge of any one count xi, combined with the output of
1, would reveal all the original counts. Now consider a mechanism
2 that adds noise drawn from a Laplace distribution, with variance 2/ε2, independently, to each histogram count, so its output consists of k noisy counts
, …,
. Mechanism
2 does satisfy ε-differential privacy;9 it is the Laplace mechanism mentioned earlier.
What is the effect of the combined release of the sanitized outputs of
1 and
2? From
, we have a noisy estimate of x1. From the quantity x1 + x2 and the noisy value
, we can obtain another independent estimate of x1. Combining x1 + x2, x2 + x3, and
we get yet another estimate. Overall, there are k independent noisy estimates of x1 that can be averaged together to get a final estimate with variance 2/(kε2), which is k times lower than what we could get from
2 alone. This example illustrates why there is a recent push for creating flexible privacy definitions that can account for prior releases of information (such as the output of
1) to control the overall inference.2,15,25
Convexity. Consider a privacy definition satisfied by two mechanisms,
1 and
2. We can create an algorithm
(p), or their “convex combination,” that randomly chooses among them; with probability p it applies
1 to its input and with probability 1–p it applies
2. Why consider mechanisms like
(p)? Convex combinations like
(p) could provide better worst-case error guarantees for some queries than either
1 or
2 for reasons similar to why mixed strategies may be preferred over pure strategies in game theory.
Now, should we trust
(p) to protect privacy? It is reasonable to do so because the only thing
(p) does is add additional randomness into the system.22,23 We say a privacy definition is convex if every convex combination of its mechanisms also happens to satisfy that privacy definition. Convex privacy definitions have useful semantic properties we discuss in more detail in the next section.
Minimizing probabilistic failure. Consider a private record that can be expressed in one bit; that is, 1 if Bob has cancer and 0 otherwise. We add noise from a standard Gaussian distribution and release the result, which happens to be 10. If Bob’s bit is 1, then we are 13,000 times more likely to observe a noisy value of 10 than if Bob’s bit is 0. We have thus almost certainly discovered the value of Bob’s bit.
A naive implementation of a privacy-preserving algorithm may not satisfy the requirements of a chosen privacy definition.
One can argue that observing a noisy value this large is so unlikely (regardless of the value of Bob’s bit) that such a privacy breach is very rare and hence can be ignored. Such reasoning has led to relaxations of privacy definitions that allow guarantees to fail with a small probability δ.; one example is the relaxation (ε, δ)-differential privacy, which can produce more accurate datamining results.
Definition 3 (ε, δ)-differential privacy.10,11 Let
be an algorithm and
be its set of possible outputs.
satisfies (ε, δ)-differential privacy if for all subsets
⊂
and for all pairs of databases D1, D2 differing on the value of a single record,
.
The decision whether to always provide guarantees or allow privacy protections to fail with a small probability is application-specific and depends on the stakes involved. It is a privacy/utility trade-off having consequences with different levels of subtlety. For instance, let
be the algorithm that outputs ⊥ with probability 1–δ. and outputs the input dataset with probability δ. This
satisfies the more relaxed conditions of (ε, δ)-differential privacy. Similarly, consider an algorithm
* that returns the record of a randomly selected individual from the input dataset. If the number of records is N and if N > 1/δ then
* satisfies (ε, δ)-differential privacy yet always violates the privacy of some individual.
Worth noting is that ε-differential privacy and the relaxed (ε, δ)-differential privacy both offer the same high-level guarantee—the output distribution of a mechanism is barely affected by the value of any individual record. Still, privacy relaxation may consistently cause privacy violations, while the former will not. Reasoning about attackers can help data curators set parameter values that limit such information leakages14 and (as we discuss later) provide new perspectives on achievable guarantees.
Implementation concerns. As with many aspects of security, moving from theory to practice requires great care. In particular, a naive implementation of a privacy-preserving algorithm may not ensure privacy even though the algorithm is theoretically proven to satisfy the requirements of a chosen privacy definition. One problem arises from side-channels. Consider a program that processes a sensitive database and behaves as follows: If Bob’s record is in the database, it produces the output 1 after one day; if Bob’s record is not in the database, it outputs 1 right away. The output is the same, no matter what the database is. But by observing the time taken for a result to be output, we learn something about the database.18
Another concern arises when the theoretical algorithms base their security properties on exact computation that may be beyond the limits of digital computers.5,31 The most common example is the addition of noise from continuous distributions (such as Gaussian and Laplace). For most floating-point implementations, an analysis of the bit patterns yields additional information about the input data.31
Finally, many privacy mechanisms rely on a random number generator. A provably secure implementation of a privacy-preserving algorithm must be tailored to the quality of the randomness of the bits.8
A Generic Recipe
Privacy definitions that are convex, closed under post-processing, and require protections for all outputs of a mechanism
all have a similar format and can be written in terms of linear constraints,28 as in the following generic template:
Definition 4 (a generic privacy definition). Let D1, D2,… be the collection of possible input datasets. For some fixed constants
an algorithm
must satisfy the following conditions for every possible output the algorithm ω can produce:
To evaluate a proposed privacy definition, a good sanity check for current best practices is thus to verify whether or not the algorithm
can be expressed as linear constraints on the probabilistic behavior of algorithms, as in Definition 4; for example, k-anonymity does not fit this template,28 but with ε-differential privacy, there is a linear constraint
for every pair of datasets Dj1, Dj2 that differ on the presence of one rec-ord. We next discuss some semantic guarantees achievable through this template.
Good and bad disclosures. Even when published data allows an analyst to make better inferences about Bob, Bob’s privacy has not necessarily been violated by this data. Consider Bob’s nosy but uninformed neighbor Charley, who knows Bob is a life-long chain-smoker and thinks cancer is unrelated to smoking. After seeing data from a smoking study, Charley learns smoking causes cancer and now believes that Bob is very likely to suffer from it. This inference may be considered benign (or unavoidable) because it is based on a fact of nature.
Now consider a more nuanced situation where Bob participates in the aforementioned smoking study, the data is processed by
, and the result ω (which shows that smoking causes cancer) is published. Charley’s beliefs about Bob can change as a result of the combination of two factors: by him learning that smoking causes cancer, and since Bob’s record may have affected the output of the algorithm. This latter factor poses the privacy risks. There are two approaches to isolate and measure whether Charley’s change in belief is due to Bob’s record and not due to his knowledge of some law of nature—”counterfactuals”12,25,33 and “simulatability.”2,15,29
Privacy via counterfactuals. The first approach12,25,33 based on counterfactual reasoning is rooted in the idea that learning the true distribution underlying the private database is acceptable, but learning how a specific individual’s data deviates from this distribution is a privacy breach.
Consider pairs of alternatives (such as “Bob has cancer” and “Bob is healthy”). If the true data-generating distribution θ is known, we could use it to understand how each alternative affects the output of
(taking into account uncertainty about the data) by considering the probabilities Pθ(
outputs ω | Bob has cancer) and Pθ(
outputs ω | Bob is healthy). Their ratio is known as the “odds ratio.” It is the multiplicative factor that converts the initial odds of Bob having cancer (before seeing ω) into the updated odds (after seeing ω). When the odds ratio is close to 1, there is little change in the odds, and Bob’s privacy is protected.
Why does this work? If the reasoning is done using the true distribution, then we have bypassed the change in beliefs due to learning about laws of nature. After seeing ω, the change in Charley’s beliefs depends only on the extent to which ω is influenced by Bob (such as it was computed using Bob’s record).
What if the true distribution is unknown? To handle this scenario, the data curator can specify a set
of plausible distributions and ensure reasoning with any of them is harmless; the corresponding odds ratios are all close to 1. A counterfactual-based privacy definition would thus enforce constraints like Pθ(
outputs ω | Bob has cancer) ≤ eεPθ(
outputs ω | Bob is healthy) for all possible ω, for various pairs of alternatives and distributions θ. When written mathematically, these conditions turn into linear constraints, as in the generic template (Definition 4).
Privacy via simulatability. The second approach,2,15,29 based on simulatability, is motivated by the idea that learning statistics about a large population of individuals is acceptable, but learning how an individual differs from the population is a privacy breach. The main idea is to compare the behavior of an algorithm
with input D to another algorithm, often called a “simulator,”
with a safer input D‘; for example, D‘ could be a dataset that is obtained by removing Bob’s record from D. If the distribution of outputs of
and
are similar, then an attacker is essentially clueless about whether ω was produced by running
on D or by running
on D‘. Now
does not know anything about Bob’s record except what it can predict from the rest of the records in D‘ (such as a link between smoking and cancer). Bob’s record is thus protected. Similarly, Alice’s privacy can be tested by considering different alterations where Alice’s record is removed instead of Bob’s record. If
can approximately simulate the behavior of
no matter what the true data D is and no matter what alteration was performed, then every individual record is protected.
Privacy definitions based on simulatability are generally more complex than those based on counterfactuals. To check whether
satisfies the definitions, it is often necessary to find the appropriate simulator
. However, in some cases, the privacy definitions can also be expressed using linear constraints, as in the generic privacy definition template.
Counterfactuals vs. simulatability. The differences between counterfactual and simulatability approaches depend on the nature of the data that must be protected. When the data records are independent of each other, properties of the data-generating distribution and properties of a population are essentially the same (due to the law of large numbers), in which case both approaches provide similar protection.
Data correlations. A difference arises when there is correlation between individuals. First, we consider a scenario when counterfactuals would be more appropriate. Suppose a database contains records about Bob and his relatives. Even if Bob’s record is removed, Bob’s susceptibility to various diseases can be predicted from the rest of the data because it contains his family’s medical history. The general goal of privacy definitions based on simulatability is not to hide this inference but to hide how Bob’s actual record differs from this prediction. On the other hand, if we include probabilistic models of how diseases are passed through genetics, then privacy definitions based on counterfactuals will try to prevent predictions about Bob and his family. Intuitively, this happens because the actual family medical history is not a property of the data-generating distribution but of a sample from that distribution. Since the family medical history is correlated with Bob’s record, it would allow better predictions about how Bob deviates from the data-generating distribution; hence, it must be protected as well.
Next, we examine a situation where simulatability-based privacy definitions are more appropriate. Consider a social network where many profiles of individuals are public. Private information about individuals is often predictable directly from the public profiles of their friends and contacts.37 Even if Bob’s profile is private, it is easy to collect information that is correlated with Bob. Here, privacy definitions based on simulatability are applicable, allowing data curators to process the social network data with algorithms
that create outputs from which it is difficult to tell if Bob’s record was used in the computation.
Data constraints. One difficulty in designing privacy definitions is accounting for public knowledge of constraints the input database must satisfy. Constraints may correlate the values of different records, arising due to, say, functional dependencies across attributes or prior exact releases of histograms. Correlations arising from constraints provide inference channels attackers could use to learn sensitive information. A privacy definition must thus account for them; for example, while Census data records must be treated confidentially, certain coarse population statistics must, by law, be released exactly in order to determine the number of Congressional Representatives for each state. More generally, if a histogram H of the data has been released exactly, how can a data curator choose a privacy definition, and hence constraints on
to account for the information in the histogram so any subsequent data release via
is able to ensure privacy? Complete solutions to this problem are open but appear to be easier for approaches based on counterfactuals if we use data-generating distributions that are conditioned on the histogram, or P(D|H).25 For approaches based on simulatability, there is more of a challenge since data-alteration techniques consistent with previously released information must be developed; recall, they provide the guarantee that an attacker would not be able to reliably determine whether the original dataset or altered dataset was used in the computation. It is important to note, too, that constraints on the input data, and especially those arising from prior releases, can be exploited for better utility.
Interpretations of differential privacy. These two approaches for defining semantics for privacy definitions also provide two ways of interpreting ε-differential privacy. The simulatability argument shows an algorithm satisfying ε-differential privacy provides the following protection: an attacker cannot detect whether
was run on the original data or on altered data from which any given record was removed.2,14 This is true no matter how knowledgeable the attacker is, as long as the data alteration is consistent with what is known about the data; if not, additional leakage can occur, as explained in the earlier discussion on composition with other mechanisms. From a different perspective, the counterfactual argument shows an algorithm
satisfying ε-differential privacy prevents an attacker from learning how an individual differs from the data-generating distribution precisely when all records are independent.25
Example: Blowfish
We illustrate this discussion with Blowfish,19 a new class of privacy definitions that follows the generic privacy template in Definition 4. Like differential privacy, Blowfish definitions satisfy a number of desirable properties we outlined earlier, including Kerckhoffs’s principle, self-composition, convexity, and closure under post-processing. The privacy goals of Blowfish definitions have both a counterfactual and a simulatability interpretation. In addition to satisfying these properties, Blowfish definitions improve on differential privacy by including a generalized and formal specification of what properties of an individual in the data are kept private and by accounting for external knowledge about constraints in the data. Blowfish thus captures part of the privacy design space. In the rest of this section, we describe how data owners can use Blowfish to customize privacy protections for their applications.
Blowfish definitions take two parameters: privacy ε (similar to differential privacy) and policy P = (
) allowing data curators to customize privacy guarantees. Here,
is the set of possible record values,
is a set of publicly known constraints on the data, and
is the set of all possible datasets consistent with
. Specifying
allows a data curator to create privacy definitions that can compose with prior deterministic data releases, thus avoiding some of the difficulties discussed earlier in the section on desiderata. To simplify the discussion, we set
to be the single constraint that the dataset has n records, in which case
=
; for more complicated constraints, see He19 on Blowfish and Kifer and Machanavajjhala25 on Pufferfish frameworks.
The final component of the policy is G = (
, E), or the “discriminative secret graph.” The vertices in G are the possible values a record can take. Every edge (x, y) ∈ E describes a privacy goal with both counterfactual and simulatability interpretations. From the simulatability viewpoint, changing a single record from x to y (or vice versa) will not cause a significant change in the probability of any output. From the counterfactual viewpoint, if records are independent, an attacker could estimate the odds of a new record having value x vs. y, but estimated odds about any individual in the data would not differ significantly from this value. Using this graph G, we define the concept of neighboring databases, then formally define the Blowfish framework:
Definition 5 (G-Neighbors). Let P = (
, G,
) be a discriminative secret graph. Two datasets D1, D2 ∈
are called G-neighbors if for some edge (x, y) ∈ E and some dataset D ∈
, D1 = D ∪ {x} and D2 = D ∪ {y}.
Definition 6 ((ε, P)-Blowfish Privacy). Let P = (
, G,
) be a policy. An algorithm
satisfies (ε, P)-Blowfish privacy if for all outputs ω of the algorithm
and all G-neighbors D1, D2 we have
.
This privacy definition clearly matches the generic template of Definition 4. We now examine some policies and their applications.
Full domain. Consider a policy PK = (
, G,
) where K is a complete graph, and every pair of values in the domain
are connected. The result is that two datasets are neighbors if they differ (arbitrarily) in any one record. (ε, PK)-Blowfish privacy is equivalent to a popular variant of differential privacy11 that requires
for all ω and for all pairs of datasets D1, D2 that differ (arbitrarily) in the value (rather than presence/absence) of one record.
Partitioned. Let us partition the domain
into p mutually exclusive subsets, with
= {P1, …, Pp}. Consider a graph
= (
, E), where any two values x, y are connected by an edge if and only if x and y appear in the same partition. Each connected component of
is thus a clique corresponding to one of the Pi. Now, two datasets D1 and D2 are neighbors if D2 can be obtained from D1 by replacing the value of one record with a new value belonging to the same partition. For example, let
be the set of all disease outcomes, partitioned into three subsets: healthy cases, communicable diseases, and non-communicable diseases. Let us use the graph
corresponding to this partition in our Blowfish policy. An algorithm
satisfying Definition 6 comes with the guarantee that the probabilities of its outputs do not change substantially if one communicable disease is replaced with another communicable disease or a healthy case with another healthy case, or a simulatability interpretation.
Learning population statistics is acceptable, but learning how an individual differs from the population is a privacy breach.
What about replacing a noncommunicable disease with a communicable disease? Can the algorithm’s output probabilities be significantly different in such a case? The answer is yes. In fact, this policy allows algorithms to publish the exact status of each individual—healthy, contagious, or noncontagious—and approximate histograms of each disease. However, specific details (such as which person has which contagious disease) are protected. Such behavior may be desirable in certain health-care applications where some facts must be disclosed but further details kept confidential.
Distance threshold. Many applications involve a concept of distance between records; for instance, the distance between two age values can be the absolute difference, and the distance between two points on a plane can be the straightline Euclidean distance or the Manhattan distance along a grid. Given a distance metric d, one can define a discriminative secret graph Gd,θ in which only nearby points are connected. That is, (x, y) ∈ E only when d(x, y) < 0 for some threshold q; for example, if
is the set of all points on Earth, and d is the orthodromic distance between pairs of points, we can set θ = 10 miles, so valid record locations are connected to other valid record locations that are within 10 miles of each other. In general, if an individual’s location x (in dataset D1) was changed to another point y (resulting in a neighboring dataset D2), then an algorithm satisfying Blowfish with this policy will have the guarantee that for all outputs ω
An adversary may thus be able to detect the general geographic region of a target individual but unable to infer the location with a resolution better than 10 miles. Such a relaxed notion of privacy is reasonable when dealing with location data; individuals may not want disclosure of their precise locations but be less worried about disclosing their information at a coarser granularity (that may be obtained from other sources). As we show later, data output by mechanisms that satisfy such relaxed notions of privacy permit data mining results with greater accuracy than if data is generated using mechanisms that satisfy the stricter notion of differential privacy.
Attribute. Let
be a multi-attribute domain with m attributes
= A1 × A2 × …, × Am. Consider a graph Gattr,c connecting any two values x and y that differ in at most c attribute values. A Blowfish policy with this graph is useful for location traces and genome data. For the former, attributes correspond to locations of an individual at different times. Neighboring datasets thus differ in at most c locations of a person, hiding the specific details about every sequence of c consecutive locations of an individual. In the genome case, an attribute corresponds to a specific position on the genome. Under this policy, an algorithm’s output would be insensitive to changes to a block of up to c positions on the genome.
Answering queries with Blowfish. Recall that adding Laplace noise with 0 mean and
standard deviation to a function f (where S(f) is the sensitivity of f) ensures ε-differential privacy. Blowfish, with a policy P = (
, G,
) is also compatible with additive Laplace noise and requires an often smaller standard deviation of
where S(f, G) is the policy-specific global sensitivity of f—the largest difference ||f(D1) − f(D2)||1 over all datasets D1, D2 that are G-neighbors.
Consider a multidimensional record domain
= A1 × A2 × …, × Am where each attribute is numeric. Let qsum denote the function that sums all the records together; that is, for each attribute, it computes the sum of the values that appear in the data. Let ai and bi denote the maximum and minimum values in attribute Ai. The global sensitivity S(qsum) of qsum is
. The policy-specific global sensitivity of qsum under Blowfish policies is usually much smaller. In the case of the distance threshold policy Gd,θ with d being the L1 Manhattan distance, S(qsum, Gd,θ) is only θ. Consider a single attribute domain
Age
and further suppose the age values range from 0 to 100. The global sensitivity of qsum is 100. The policy-specific sensitivity of qsum under GL1,5 is only 5. If, instead, the policy used a partition graph
that partitions age into ranges (such as {0 – 10, 11 – 20, 21 – 30,…,91 – 100}), then the policy-specific global sensitivity is only 10. Finally, with the attribute policy, S(qsum, Gattr, 1) = maxi(ai − ci.
K-means clustering. For a specific data-mining result, consider an application of Blowfish to k-means clustering.
Definition 7 (K-means clustering). Given a set of n vectors {x1, …, xn}, the k-means clustering problem is to divide these n records among k clusters S = {S1, …, Sk}, where k ≤ n, so as to minimize the objective function
where
is the mean of cluster Si.
The iterative (non-private) k-means clustering algorithm initializes a set of k centroids {μ1, μ2,…, μk}, one for each cluster. These centroids are iteratively updated in two steps: assign each xj to the cluster with the nearest centroid, and set each centroid μi to be the mean of the vectors of its corresponding cluster. The algorithm terminates after a certain number of iterations or when the centroids do not change significantly.
Each iteration (the two steps) are easily modified to satisfy ε-differential privacy4,30 and Blowfish.19 These steps require access to the answers to two queries: qhist, which returns the number of points in each cluster, and qsum, or the sum of the points in each cluster. As discussed earlier, qsum can be answered through the Laplace mechanism. Analogously, qhist can be answered with the Laplace mechanism because it has global sensitivity S(qhist) = 1 (for differential privacy) and policy-specific global sensitivity S(f, G) = 2 for all Blowfish policies discussed here. The policy-specific sensitivity of the qsum query under Blowfish policies is typically much smaller than its global sensitivity so we would thus expect more accurate clustering under the Blowfish privacy definitions.
Figure 2 confirms this improvement in utility. For the clustering task, we used a small sample of the skin-segmentation dataset,3 or 1%, which is approximately 2,500 instances, in order to make the problem challenging. Each instance corresponds to the RGB intensities from face images, and each intensity ranges from 0 to 255. The x-axis is the privacy parameter ε, and on the y-axis (note the log scale) we report the error incurred by the privacy-preserving algorithms. We measure the error as the ratio between the squared error (Equation 1) attained by the privacy-preserving algorithms to that achieved by the non-private k-means algorithm after 10 iterations that was sufficient for the convergence of the non-private algorithm. The Laplace mechanism for ε-differential privacy incurred the most error. Using the Gattr,1 policy already reduces the error by at least a factor of 1.5. The error is further reduced when using GL1,θ, for θ ∈ {256, 128, 64, 32}. It is interesting to note the error does not increase monotonically as we increase θ – GL1,128—an improvement of 3x and 2x over differential privacy for ε ≤ 0.5 and ε > 0.5, respectively. One explanation is that small amounts of error can help avoid local minima while clustering.
Conclusion
Privacy definitions are formal specifications an algorithm must satisfy to protect sensitive information within data. Our experience shows that designing robust privacy definitions often requires a great deal of subtlety. Our goal is to present some of the major considerations in this design process, along with example privacy definitions and resulting privacy mechanisms. We hope this discussion inspires additional curiosity about the technical nature of privacy.
Acknowledgment
This work is supported by the National Science Foundation under Grants 1054389 and 1253327.
Join the Discussion (0)
Become a Member or Sign In to Post a Comment