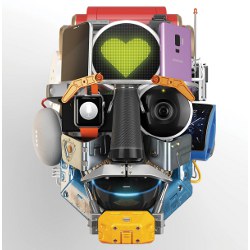
Today, people increasingly rely on computer agents in their lives, from searching for information, to chatting with a bot, to performing everyday tasks. These agent-based systems are our first forays into a world in which machines will assist, teach, counsel, care for, and entertain us. While one could imagine purely rational agents in these roles, this prospect is not attractive for several reasons, which we will outline in this article. The field of affective computing concerns the design and development of computer systems that sense, interpret, adapt, and potentially respond appropriately to human emotions. Here, we specifically focus on the design of affective agents and assistants. Emotions play a significant role in our decisions, memory, and well-being. Furthermore, they are critical for facilitating effective communication and social interactions. So, it makes sense that the emotional component surrounding the design of computer agents should be at the forefront of this design discussion.
Key Insights
- Systems that respond to social and emotional cues can be more engaging and trusted, enabling computers to perform complex tasks in a more socially acceptable manner.
- Emotionally sentient agents present the exciting potential for large-scale, in-situ experimentation and user experience testing. Large-scale analysis of affective data from everyday contexts is important for improving affective computing systems, and helping us learn more about human expression and well-being.
- It is important for designers to consider the specifications of emotionally aware systems. Learning purely from human-human behavior may not always be the most effective approach and an affective agent may raise users’ expectations of competence that the system may not possess.
Consider the following examples: Personal assistants (PAs) have become ubiquitous in our everyday computing lives. From well-known services like Amazon’s Alexa, Apple’s Siri, Microsoft’s Cortana, or Google Assistant, to chat bots for areas such as customer service and training, consumers are familiar with the concept of a computerized PA. We argue that for a PA to truly become valuable to the user, it must be natural to interact with and engaging. How do we design a PA that is liked, fun, and easy to work with, and most importantly, trustworthy? Several researchers have shown that an assistant that can sense a user’s social cues and affective signals along with her context, and respond appropriately, is valued more, considered more intelligent, and creates a greater desire by the user to interact with it.4,17
As they move into the digital era, healthcare and mental healthcare are seeing vast benefits from the influx of technology and machine learning. However, few systems effectively track the emotional health of their users—most of the time this is done via paper forms filled out before a doctor or therapy visit. The problem is that memory limits render these methods less effective over extended periods of time and are associated with demand effects (changes in behavior resulting from cues as to what constitutes appropriate behavior.) Computer programs can now track consumer and patient health, allowing for mining of that data for ideal intervention timing and personal reflection by the individual user of what makes them feel positive or not.24 Recent efforts have successfully used conversational agents to automate the assessment and evaluation of psychology treatments.25 Conversational agents could help with social support, wellness counseling, task completion, and safety, if they are designed with the ability to sense and manage affect and social interaction. This promising new direction could, for example, stave off rampant problems of loneliness in the elderly.31
Researchers have argued the relationship between a tutor and a learner plays an important role in improving educational results.39 New educational platforms (for example, EdX and Coursera) are asynchronous and distributed. Automated tutoring systems designed with the ability to understand students’ affective responses are very promising.11 There is also growing literature on using affective agents in training simulations, (for example, by the military), to improve realism, evoke empathy, and even stir fear.15 These simulations are critical for preparing soldiers, medical staff, and other personnel for the realities of combat zones and environmental catastrophes.
Affective computing brings new-found realism and immersion to entertainment applications, such as games, interactive media exhibits, and shows. In fact, companies have recently tracked their audience’s affective response as it was presented with variants of commercials and other kinds of entertainment during sporting events (for example, Affectiva, Inc. and Emotient, Inc.). This practice is becoming increasingly common in the areas of marketing and advertising to drive decision-making about marketing content (for example, what content works best, when and where to air advertisements).
Beyond these examples, emotionally intelligent systems are likely to impact retail, transportation, communications, governance, and policing. Computers are likely to replace human service professionals in many settings and emotion will play a role in these interactions. This wealth of examples illustrates the impact this technology might have on society. Careful design is therefore critical. Many people currently say they would not trust a machine with important decision-making (that is, money or health management), even when given evidence that machines can perform many tasks, such as data collection, numerical analysis, and planning more effectively than humans.a This further reinforces the need for research around systems that engender trust and personalized, emotional intelligence, so they might be considered more trustworthy, empathetic, socially appropriate, and persuasive. However, it will not always be appropriate to make affective systems. For instance, a PA could be considered valued if it performs essential functions, regardless of how natural it is to interact with. Consider human air traffic controllers and the highly analytical and symbolic way that they interact with airline pilots, as one example. Therefore, it is important to consider when it is appropriate to make technology emotion-aware.
As the basis of our position, we turn to a recent article written by Byron Reeves29 about interactive, online characters that might have several advantages over alternative system instantiations. Reeves claims that since the interactions humans have with media are fundamentally social, it is important for embodied agents to employ social intelligence to be successful. He makes the point that socially intelligent interfaces increase memory and learning and explicitly ground the social interaction. He argues that people implicitly react to these online characters (agents) as social actors. The agents could also increase trust in their interactions, which could be ever more important moving forward, as we incorporate the human-appropriate design aspects.
It has been 20 years since Rosalind Picard published her seminal book on the subject of affective computing.28 As with other areas of artificial intelligence (AI), however, progress toward her vision has ebbed and flowed. Smaller electronics have transformed wearable computing, enabling signals to be captured and analyzed on comfortable wrist-worn devices. Many consumer-grade smart watches now contain miniaturized physiological sensors that could be used for affect detection. Machine learning, including deep learning, has significantly improved computer-based speech and visual understanding algorithms, such as speech-to-text, facial expression recognition, and scene understanding.
As is the case with other forms of computer technology, there is danger of overhyping the capabilities of affective computing systems. Many of the compelling applications of affective computing have yet to be realized, in part because designing emotionally sentient systems is much more complex than simply sensing affective signals. Understanding and adapting to emotional cues is highly context dependent and relies on tacit knowledge. Compounding this, large, interpersonal variability exists in how people express emotions. Humans also have diverse preferences for how an agent responds to them. Personalization is very important to enable more compelling systems. The most successful affective agent is likely one that can learn about a person’s nuanced expressions and responses and adapt to different situations and contexts.
To do all of this, we must develop models of emotion that are amenable to computation. This is challenging, as emotions are difficult define, and the relationship between observed signals and states often requires a many-to-many mapping. Furthermore, human knowledge of emotion is predominantly implicit, defined by unwritten, learned social rules. These rules are also culturally dependent13 and not universal. Scientists have proposed numerous models of emotion, each with their own strengths and weaknesses. Nevertheless, the choice of defining emotions has significant implications for the design of a sentient system.
In this article, we describe the numerous benefits that emotion-aware systems can deliver for society. However, it would be negligent to downplay the significant ethical challenges and public concerns that surround the development of this technology. Practitioners should consider our proposals for safeguarding people and maintaining their trust.
To summarize, systems that respond to social and emotional cues are more engaging,9 build rapport better,16 and are more trustworthy.4,17 Unsurprisingly, researchers have also found them to be rated as more human-like and intelligent.33 However, as with physical appearance, there may not be a linear relationship between an agent’s emotional response and how likable it is. Specifically, an “uncanny valley”21 may exist for emotional expression. Humans are very adept at detecting behaviors that appear to be “off.”
We argue that for a PA to truly become valuable to the user, it must be natural to interact with and engaging.
Despite the number of challenges associated with building emotionally sentient systems, it remains a highly motivating goal. For anything other than simple tasks, emotionally intelligent agents have the potential to improve our health and quality of life. For just one example, these systems could help deliver mental health therapy to people struggling to access traditional care,b an area of increasing importance.
Here, we address the key design challenges in developing emotionally sentient systems, namely affect sensing, interpretation, and adaptation. While it would not be possible to survey each challenge in depth, we highlight the state-of-the-art research and discuss the most pressing opportunities facing researchers and practitioners.
Emotion Sensing
Affect sensing and tracking in and of itself has benefits. For example, one could track how the emotions of an individual change over time to understand his emotional triggers.24 In most cases, however, users would want a system that adapts and responds to affective cues in an intelligent way, such as a computer game designed to change in difficulty based on the players’ emotions (for example, Nevermind by Flying Mollusk, Inc.). Furthermore, it is likely that people will desire systems that respond with the appropriate emotion if interaction is required.27
Sensing affective states is an integral part of designing emotionally sentient systems. For more than 25 years, computer science methods have been applied to visual, audio, and language data to infer emotion. In many cases, this involves detecting subtle signals amongst high-dimensional data. While verbal and nonverbal cues both contain rich information about a person’s emotional state, researchers have found significant improvement in the automated understanding of nonverbal behavior by combining signals from numerous modalities (such as speech, gestures and language).10 Though the aim of this article is not to survey affect sensing methods, it is important to discuss them, as they influence many practical design considerations. For example, how should designers choose the appropriate types of sensor signals to measure emotions? What is the best way to fuse signals from different modalities? How can you tell if sensor measurements are sufficiently accurate for a given use case? How can a system distinguish between emotional expression and other social cues? In this section, we will discuss the detection of signals. In the next, we discuss how they might be modeled and interpreted.
Verbal. Linguistic patterns and word choice can tell us a lot about a user’s affective state. Linguistic style matching occurs between people in natural social interactions.26 Typically, style matching is a sign of rapport or bonding between individuals. People may even alter their speaking style without being consciously aware of it over the passage of time with an interactant.
The LIWC software is a package that enables automatic extraction of linguistic style features26 by capturing the frequency of use of words from different categories. For example, positive, negative, and functional words turn out to be especially important. Matching a person’s linguistic style (for example, through word choice) is perhaps one of the simplest ways an agent can be designed to emotionally bond with a person. For unembodied chatbots, this is one of a small set of techniques that could be used. There are numerous packages available for text and speech sentiment analysis, and they are simple to apply. One can design a system that analyzes speech or text for verbal sentiment with a speech-to-text engine. Designers should be aware that these systems might not capture the full complexity of human language. Though many of these systems are trained on large-scale corpora that are available to researchers (for example, Tweets), they may not always generalize well to other domains (like email messages).
Nonverbal. Facial expressions, body gestures, and posture are some of the richest sources of affective information. We use automated facial action coding and expression recognition systems to measure these signals in videos. Automated facial action coding can be performed using highly scalable frameworks,23 allowing analysis of extremely large datasets (for example, millions of individuals). These analyses have revealed observational evidence of cross-cultural and gender differences in emotional expression23 that for the first time can actually be quantified. Depth-sensing devices like the Kinect sensor significantly advanced pose, gesture, posture and gait analysis making it possible to design systems that used off-the-shelf low-cost hardware. Designers now have access to software SDKs for automated facial and gesture coding that are relatively simple to integrate into other applications. These can even be run on resource-constrained devices enabling mobile applications of facial expression analysis, such as mobile agents that respond to visual cues.
Acknowledging expressions of confusion or frustration from a user’s face is one practical way that an agent could make use of facial cues to the benefit of the interaction. Within a known context (that is, an information-seeking task) it is possible to detect these types of negative expressions when they occur. Generally, responses to incorrectly detected affective states will not frustrate the user if they are able to understand the reasoning that the agent used.24
The use of a camera or microphone for measuring affective signals (whether in public or private settings) is a particularly sensitive topic, especially if subjects are not aware the sensors are present and active. Designers need to carefully consider how their applications may ultimately influence social norms about where and when video and audio analysis and recording is acceptable.
Speech prosody. With the rise of conversational interfaces (such as Cortana and Siri) nonverbal speech signals present an increasingly valuable source of affective information. As with facial coding, there is a strong focus on designing systems that work outside of lab-based settings. Numerous companies have related software development kits (SDK) and application programming interfaces (API) (for example, BeyondVerbal, audEERING, Affectiva) that provide prosodic feature extraction and affect prediction. As with facial expressions, there is likely to be some level of universality in the perception of emotion in speech (a similar set of “basic” emotions) but a great amount of variability will exist across languages and cultures. Many of these “non-basic” states will be of greater relevance in everyday interactions.
Physiology and brain imaging. While expressed affective signals are those that are most used in social interactions, physiology plays a significant role in emotional responses. Innervation of the autonomic nervous system has an impact on numerous organs in the body. Computer systems can measure many of these signals in a way that an unaided human could not. Brain activity (for example, electroencephalography (EEG), functional near infra-red (fNIR)), cardiopulmonary parameters (for example, heart and respiration rates and variability) and skin conductance all can be used for measuring aspects of nervous system activity. Although wearable devices have only had partial adoption, there are several compelling approaches for measuring cardiovascular (heart) and pulmonary (breathing) signals using more ubiquitous hardware. The accelerometers and gyroscopes on a cellphone can be used to detect pulse and breathing signals, and almost any webcam is sufficient to remotely measure the same. While people are experienced at applying social controls to their facial expressions and voice tone, they do not have the same control over physiological responses, meaning measurements may feel more intimidating and intrusive to them. One should be cognizant of these concerns in the design of agents, as they are likely to influence how the agents are perceived, from how likable they are, to how trustworthy they are.
Design challenges for adoption. Despite the advances in sensing emotions, there remain many challenges in basic objective measurement. Many of these measurement approaches have not been characterized, or simply fail in natural settings. For example, facial expression recognition may be reliable for videos with simple behaviors and when the face is frontal to the camera, but, in the case of out-of-plane head rotation and co-occurring facial actions, recognition can perform poorly. Physiological sensing approaches are seriously hampered during physical activities. As machine learning and affective computing research advance, objective measurement techniques will improve. In the meantime, practical systems can still be deployed based on automated facial and speech analysis. However, designers need to take these limitations into account.
One challenge with real-world systems that respond to emotions is that expressions of emotion are often very subtle or sparse. This may mean that it is challenging to develop automated detection systems with high recall (that is, the fraction of emotion responses detected) and low false positive (alarm) rates. In social interactions, many nonverbal behaviors (for example, smiling) will be more frequent than when people are alone. Thus, it may be more practical to design systems that respond to both social and emotional cues.
The sparsity and lack of specificity within unimodal cues (that is, a facial expression) are key reasons why multimodal affective computing systems have been found to be consistently better than unimodal ones.10 In some settings (for example, call center analysis) the availability of visual cues might be limited. In others, various modalities might not available. The most effective systems will be those that leverage the most information, both about the individual and the context she is in.
Large interpersonal variability exists in nonverbal behaviors. Thus, person-specific models can bring many benefits. Longitudinal studies are needed for this type of modeling. To date, such studies have been few and far between. We need to design new mechanisms for incentivizing individuals to interact with a system or to be passively monitored for extended periods of time. Ultimately, the most successful affective computing technology will be able to build personalized models that leverage online learning to update over time.
Emotion Labels
One of the most significant choices in designing an affective computing system is how to represent or classify emotional states. Emotion theorists have long debated the exact definition of emotion, and many models and taxonomies of emotion have been proposed. Common approaches include discrete, dimensional, and cognitive-appraisal models; other approaches include rational, communicative and anatomic representations of affect.22
Discrete models. Discrete categorizations of emotion posit that there are “affect” programs that drive a set of core basic emotions and the associated cognitive, physiological, and behavioral processes.39 There are several categorizations that have been proposed, but by far the most commonly used set is the so-called “basic” list of emotions of anger, fear, sadness, disgust, surprise, and joy. These states can be represented as regions within a dimensional space. In practice, the challenge with discrete models of emotion arises from the state definitions. Even “basic” states do not occur frequently in many situations. Designers must a priori consider which states might be relevant and/or commonly observed in their context.
Dimensional models. The most commonly used dimensional model of affect is the circumplex—a circular, two-dimensional space in which points close to one another are highly correlated. Valence (pleasantness) and arousal (activation) are the most frequently selected descriptions used for the two axes of the circumplex, however, the appropriate principal axes are still debated. Another model uses “Positive Affect” (PA) and “Negative Affect” (NA) each with an activation component. Dimensional models are appealing, as they do not confine the output to a specific label but can be interpreted in more continuous ways. For example, in some applications, none of the “basic” emotions labels may apply to an observed emotional response, but that response will still lie somewhere within the dimensional space. Nevertheless, a designer will still need to carefully consider which axes are most appropriate for their use case.
Appraisal models. Cognitive-appraisal models consider the influence of emotions on decisions. Specifically, emotions are elicited and differentiated based on a person’s evaluation of a stimulus (that is, an event or object). In this case, a person’s appraisal of a situation will affect their emotional response to a stimulus. People in different contexts experiencing the same stimulus will not necessarily experience the same emotion.
Appraisal models employ a more formalized approach to context. This is very important, given that only a very small number of behaviors are universally interpretable (and even those theories have been vigorously debated). It is likely that a hybrid dimensional-appraisal model will be the most useful approach.
Although academics have been experimenting with computational models of emotion extensively, there are no commercially available software tools for recognizing emotion (either from verbal or nonverbal modalities) that use an appraisal based model of emotion. Incorporating context and personalization into assessment of the emotional state of an individual is arguably the next big technical and design challenge for commercial software systems that wish to recognize the emotion of a user.
Emotional Agents
Several articles have been written on the benefits of conversational agents for more naturalistic human-computer interactions.7,8 This research movement partly came from a belief that traditional WIMP (windows, icons, mouse, and pointer) user interfaces were too difficult to navigate and learn14 and not natural enough. Here, we focus on the addition of emotional sentience to the agent to explore what additional benefits might be achieved with the addition of intelligent affect sensing and appropriate agent-based responses.
Dialogue systems. The first examples of affective agents were dialogue systems. In the 1960s, Eliza was an agent capable of limited natural language understanding37 that simulated a psychotherapist. Recently, chat systems have become popular and are being used in many forms, from mental health therapy to customer support. The practical application of these dialogue systems has been made possible by advancements in natural language processing (NLP). The barrier to create bots is now much lower, as illustrated by a 14-year-old boy who created his own homework reminder bot.c Many emotional cues are nonverbal, and therefore require an agent to have the ability to express nonverbal emotion. More recent dialogue systems, such as Xiaoicedd), leverage text-to-speech technology, allows for a greater range of expression through voice prosody. Yet, the effective synthesis of nonverbal cues is still a very challenging problem. Currently, realistic synthesis of voice tone requires thousands of lines of dialogue to be recorded. Generative machine learning methods may eventually help replace the need for this type of labor-intensive data collection and provide realistic voice synthesis.
Virtual agents. While most present day virtual, conversational personal assistants do not rely on emotional recognition or delivery (for example, Siri, Cortana, and others), there has been a large literature examining personality and other emotional components of conversational agents, as well as the social and personal benefits that accrue from their use. Starting with the work by Reeves and Nass30 in their landmark book, The Media Equation, a communication theory was laid out that suggested humans treated computers and other forms of media as socially as they would another human during conversation. They also claimed that this response from humans is automatic (that is, without conscious effort). Reeves and Nass argued that people respond to what is present in new forms of media, and their perception of reality, as opposed to what they know to be true (for example, this is a computer). This allows users to be able to assign a personality to a conversational agent, among other things. Through a series of studies, Reeves, Nass and their colleagues showed that politeness, personality, emotion, social roles, and form all influence how humans treat and respond to all kinds of media, including computer systems. Researchers in the tutoring community11 have shown that emotionally sentient systems enhance the effectiveness of human-computer interaction, and that the lack of emotional responsiveness can reduce performance. Kraemer19 has provided ample evidence of the benefits of socio-emotional benefits of pedagogical conversational agents.
A further line of research emphasizes that embodied agents offer several advantages over non-embodied dialogue systems. An agent that has a physical presence means that the user can look at it. Cassell8 has written a lot about this, including how the representation of the agent and its modalities have greater benefits than the early dreams of ubiquitous computing36 and its goal of embedded (invisible) interaction. Central to her argument is that it is important to realize how humans interact with each other. The human body allows us to “locate” intelligence—both the typical domain knowledge required, but also the social and interactional information we need about conversational parameters such as turn-taking, taking the floor, interruptions, and more. In this vision, then, an embodied social agent who converses with the user requires less navigation and searching than traditional user interfaces (because you know where to find information). Multimodal gestures, such as deixis, eye gaze, speech patterns, and head nods and other, nonverbal gestures are external manifestations of social intelligence which support trustworthiness.3 For instance, early research has shown that to attain conversation clarify, people rely more on gestural cues when their conversations are noisy.32 From this perspective, embodied social agents might be a more natural way for people to interact with computation.
So, conversational agents provide a mental model for the user to start with. Well-designed or anthropomorphic features can then help to create a framework of understanding around how to work with these agents. Specifically, conversational agents can provide affordances for available interaction qualities, capabilities, and limits. Our argument is that if designers can tap into users’ natural affinity for social interaction with an agent, this will also lead to higher levels of affinity for, and interaction with, that agent. This will eventually lead to trust. If we design agents to not only behave as we expect them to, but also to adhere to social norms and values, then we can amplify trust.12
Today, research focusing on virtual assistants, both embodied and not, has achieved positive results: improving users’ task performance,29 establishing trust and likeability in a real estate transaction context,3 improving naturalness of interactions with appropriate emotions,29 and in advancing tutorial systems.11,39 This can largely be attributed to the findings that humans respond to these systems socially, even when they are not. Adding emotional intelligence should only enhance this natural, social response, but more research is needed.
The issue of “social caretaking”6 (that is, using emotional agents to care for the young, infirmed, or elderly) is a new field under investigation. It has been found that proactive, affective agents can help elderly users feel more comfortable with the technology, and can even ease loneliness to some degree.31 Also, work by Lucas et al.20 shows the real promise in using conversational agents in clinical interviews. They obtained more honest responses from patients with increased willingness to disclose, since the patients felt more comfortable talking with an agent than a human in certain circumstances. While researchers in this line of work have shown the benefits of agents, they have also pointed out that humans will engage in racism, lie, feel envy, and more toward emotional agents. Thus, this is a key area to continue exploring, as we get better at designing emotional systems.
However, there have been concerns raised that the appearance of these embodied emotional agents lack naturalness, especially with nonverbal gestures and cues such as inaccurate eye gaze or emotional facial gestures.2 If humans begin to mimic or model their interactions with an agent who doesn’t emote appropriately, it could result in negative emotional learning. This issue is of most concern in the social caretaking scenarios mentioned above, and especially with children, who model behavior through social learning.1 While the affective modeling community is making great strides in creating more natural, human-like embodied agents with real, human-like communication patterns,39 we have a lot to do to allay these concerns.
Robots. Physical systems have advantages over virtual agents. The most obvious is that robotic systems can perform physical actions and tasks in the real world. They can put an arm around a person to comfort them or move an object or make a meal. Again, in this domain, research is revealing the benefits of robots that express affect appropriate to each situation, such as asking for something politely or apologizing after making a mistake. Researchers have found that robots showing human-like expressions and positive politeness are more able to get humans to assist them and that robots that show sorrow or sadness after making a mistake are viewed as more intimate, especially if the users thought the robots were acting autonomously.17 Hammer et al.18 report on several studies that look at the acceptability of social robots by older adults. They found that attributes like appearance, intellectuality, friendliness, and kind-heartedness are important for acceptability. In addition, robot companions may be viewed more positively if they emulate situationally appropriate social behavior.
These systems will always suffer from imperfect reliability and a superior design principle involves exposing transparency about the outcome and involving the human in the reparation.
Another well-known study also looked at users’ reactions to interactions with a robot after good or mistaken task performance and whether or not the robot responded emotionally.17 These researchers were interested in the question surrounding unexpected behaviors from robots during collaborative tasks, which are extremely likely to occur. There is currently very little research on the topic. These researchers thought an affective interaction might be more useful and trust-enabling than a more efficient, less human-like interaction. What they found was a humanoid robot that expresses emotions, for instance apologizing via speech and nonverbal gestures, is much preferred over one without these skills, despite taking more time on the task and making errors. They also found the robot that exhibited more human-like, emotional signals might make humans more likely to feel empathetic toward the robot, and not want to hurt its feelings. Most importantly, the humans trusted these robots more because of their increased transparency and feedback in communication and emotional expression. These findings suggest that robots that express human-like, polite, emotional signals can significantly mitigate dissatisfaction when errors or other problems occur during human-agent interaction. These findings could also result in good design guidelines for designers of human-robot or other kinds of human-agent conversational systems. These systems will always suffer from imperfect reliability and a superior design principle involves exposing transparency about the outcome and involving the human in the reparation. As the authors point out, however, juxtaposing reliability with expressiveness is challenging and the design of an error-free system is unlikely in the near term.
And of course, there is concern about the uncanny valley, as it has been shown that if robots look too human-like, but do not match social expectations in terms of behavior, then people do not like and might distrust these systems even more. Anti-robot sentiment, in addition, could be a real concern. People may feel threatened by the proliferation of robots and the appearance that robots will not care for humans, act morally or ethically.
Future Affective Systems
The deployment of intelligent agents is widespread on mobile devices and desktops. However, most agents that have been designed with some emotion sentience have been limited to constrained experimental settings. While “cognitive” agents can often perform effectively with NLP alone, emotionally sentient agents require multimodal sensing capabilities and the ability to express emotion in more complex ways, which has been very challenging to achieve in real-world settings. However, given the review here, it is likely the next frontier on which these assistants/agents compete with one another will be their ability to emotionally connect with their users.
Social robotics that have basic facial expression recognition (for example, Pepper, Softbank Inc.) are now on the market. These devices are likely to elicit a richer set of emotions than the typical interaction with a cognitive agent designed for information retrieval. As such, they present the exciting potential for large-scale, in-situ experimentation and user experience testing. Large-scale collection and analysis of affective data is important for improving affective computing systems, and deployment of systems in everyday contexts is one way to achieve this, with the obvious caveats raised earlier.
Robots can express rich emotion, in addition to having customized hardware for sensing affective signals. Leonardo5 is an example of a robot with a face capable of near human-level expression. Commercially available robots, such as Cozmo (by Anki, Inc.), have engines for expressing limited physical emotional behaviors. However, robotics such as these are unlikely to be ubiquitous in the near-term. The most common emotional agents are still likely to be virtual. These agents need not have human appearance; abstracted representations of characters can still communicate significant amounts of emotional information. We can return to perhaps the most famous robot of all—R2-D2—that was scripted to successfully convey many emotions through colors and sounds. Agents such as Cortana could use similar abstractions to both convey emotions and elicit emotion from their users; physical motion is not a prerequisite for complex emotional expression.
It is also important for designers to understand that learning purely from human-human behavior may not always be the most effective approach.35 Considering how to present and sense information is important when a user is trying to complete tasks that already require considerable cognitive processing.
Embodied social agents can help express and regulate emotion, which is important in every social interaction. We know that emotional intelligence is a key factor in intellect and can strongly influence behavior. Per Reeves,29 research shows that negative experiences with technology are much more strongly remembered and actionable than are positive ones, so automated systems need to consider negative interactions in design, as ignoring these negative incidents could lead to the same bad feelings, or worse, rejection of an automated system. Embodied social agents are a preferred way to deal with these kinds of experiences. Facial expressions, for example, can signal what responses are appropriate, or when more information is needed. This can be much faster than just using words or text alone. Likewise, intelligent social agents can be used to display important social and cultural manners, whose influence should not be ignored in design as well. Reeves’ overall point, much like that of Cassell’s,3,9 is that embodied, social agents that respect human-to-human interaction protocols, simply can make user interfaces easier to use, if designed appropriately.
In the near-term machines are unlikely to understand all of the complex social norms that humans typically follow or detect the emotional states of people with high precision and recall. Therefore, agents will, on occasion, exhibit socially inappropriate behavior. Ideally, an intelligent system should be designed so that it can learn from these mistakes, or at the very least apologize when a mistake is detected. In a week-long study, we found that people were generally delighted when the computer accurately reflected their mood and quite forgiving when it did not.24 However, for a commercial system that will be used for more than two weeks, a user’s patience could be tested by a system which regularly makes mistakes and cannot be corrected or learn online.
Designing systems that can measure, often passively, and log affective signals presents ethical challenges. As with any technology, there is the possibility that it will be abused. Much of the hardware used for sensing affective signals is small and ubiquitous (for example, microphones or webcams). Even measurement of physiological signals can be performed using these devices and does not require contact with the body. Thus, people may not be aware that an agent is measuring and responding to their emotional state.
As described in Becker et al.,2 our ability to render emotional expressiveness in agents is extremely limited today, though this is improving quickly. Still, it should be cautioned that embodied agents and robots will never experience the physiological reactions nor the actual emotions that they project (for example, a racing heart or relaxation). The question then becomes one of how humans react to this limited display of emotionality and our obvious understanding that these agents are not human. Much more experimentation must be done to identify the uncanny valley and find design sweet spots, where more natural expression abilities and ease of use don’t crossover into negative experiences.
There is a danger that a person could be manipulated by agents that can interpret their emotional state. People tend to trust agents that appear more attractive, for example, even when they are not reliable.38 Deception of this kind must be avoided. If we are to be interacting with computer agents more and more, there is a likelihood that we will change our behavior to mimic that of the system, much as humans do.26 Other evidence supports this idea, such as data showing that people are changing how they think as a result of using Internet search engines. Specifically, children, who have extensive interactions with an agent that cannot accurately mimic human emotional cues and understanding, may end up “imprinting” these social agents’ behaviors and styles of interaction. Another undesirable outcome would be that children grow-up treating agents rudely and that these behaviors leak into human interactions. Designers should study and consider how to minimize the chance of these negative scenarios.
Finally, an affective agent may raise the users’ expectations of competence or common sense that the system may not possess. In circumstances where this could lead to frustration, or other negative outcomes, it might not be appropriate to make a system respond to affective signals.
Conclusion
While research and development of emotionally sentient computer systems is already 50 years old, only recently have these systems been adopted for real-world applications. Agents that sense, interpret, and adapt to human emotions are impacting healthcare, education, media and communications, entertainment, and transportation. However, there remain fundamental questions about the design principles that should govern such systems. From the types of signals that are measured, to the model of emotions that is employed, to the types of tasks they perform and the emotions they express, there are fundamental research questions that still need to be answered.
Agents can take many forms, from dialogue systems to physically expressive humanoid robots. While intelligent agents are widespread on mobile devices and desktops, those that have been designed with emotional sentience have been limited to constrained experimental settings. However, one could argue the deployment of emotionally sentient systems is at a tipping point. The next major advancement in development will be spurred by large-scale and longitudinal testing of these systems in real-world settings. This will in part be made possible by the increasing adoption of intelligent assistants (for example, Apple’s Siri, Microsoft’s Cortana, Amazon’s Alexa, or Google Assistant) and in part by the availability of social robots.
We have highlighted current design challenges that are limiting adoption of these systems, including, how to account for large interpersonal variability, sparsity, many-to-many mappings between behaviors and emotions, and how to create a system that avoids social faux pas. There are ethical issues raised by emotionally sentient systems and this needs very serious, careful design consideration.
Figure. Watch the authors discuss this work in the exclusive Communications video. https://cacm.acm.org/videos/designing-emotionally-sentient-agents
Join the Discussion (0)
Become a Member or Sign In to Post a Comment