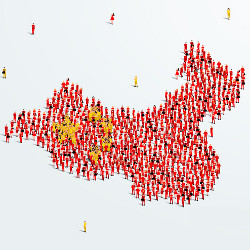
Mobile crowdsensing (MCS) presents a new sensing paradigm based on the power of user-companioned devices.11,12 It allows “the increasing number of smartphone users to share local knowledge acquired by their sensor-enhanced devices, and the information can be further aggregated in the cloud for large-scale sensing.”4 The mobility of large-scale mobile users makes MCS a versatile platform that can often replace static sensing infrastructures. A broad range of applications are thus enabled, including traffic planning, environment monitoring, urban management, and so on.
During the past decade, MCS has become a surging research topic in China. There are several reasons that precede this new sensing paradigm:
- Widespread new techniques. With the rapid advancement of mobile communication (4G/5G) and pervasive sensing techniques, it has been reported that sensor-rich smartphone users reached around 800 million in China by 2020, forming the largest ‘mobile’ population around the world. The prevalence of mobile devices in China builds a solid physical foundation for crowdsensing.
- Promotion by national research and development plans. China has put significant efforts into improving MCS through a series of key projects under its national R&D plans. These plans cover a series of major techniques and application areas, such as the Internet of Things (IoT), smart cities, as well as the next generation of artificial intelligence (AI 2.013).
- Particular developmental challenges and opportunities. As a developing country, China is undergoing a large-scale urbanization process. Numerous complex challenges have been raised, such as environment protection, transportation optimization, and urban management. The emergence of MCS opens up new opportunities to address these challenges.
The term “mobile crowdsensing” was coined in Ganti et al.’s work14 in 2011. In 2012, Yunhao Liu from Tsinghua University gave a thorough characterization of the definition for the first time, noting research challenges as well as MCS opportunities.11 Huadong Ma et al. from Beijing University of Posts and Telecommunications (BUPT) proposed several new metrics (for example, opportunistic coverage) to characterize the sensing opportunity and quality.12 The team led by Zhiwen Yu at Northwestern Polytechnical University (NPU) gave a thorough review of the challenges, the architecture, novel applications of MCS, and proposed the Visual Crowd-sensing (VCS) concept,3,4 which leverages built-in cameras of smartphones to attain informative/visual sensing of interesting targets. Tsinghua University and Shanghai Jiaotong University (SJTU) systematically study the general incentive mechanisms in MCS,6,20 as it is important to have the active participation of citizens. Zhang et al. from Peking University investigated Sparse Mobile Crowdsensing,16 which leverages the spatial and temporal correlation among the data sensed to reduce the required number of sensing tasks allocated.
Key applications and beyond.
Besides scientific investigation, many novel MCS applications (as shown in Figure 1) have also been developed in China to address the societal and developmental issues in different domains.
Figure 1. Representative MCS apps.
- Environment protection. Rapid urbanization usually results in severe air pollution problems (for example, PM2.5), especially for cities in developing countries like China. However, until now it has been difficult to obtain finegrained citywide PM2.5 status due to insufficient monitoring sites. Third-Eye,9 a crowdsensing application developed for fine-grained PM2.5 monitoring, is rooted in a joint research by BUPT and Microsoft Research Asia. It uses advanced deep learning algorithms to infer PM2.5 levels simply using the outdoor images taken by citizens’ smartphones. This helps government and researchers collect fine-grained data to better understand the causes and the propagation mechanism of PM2.5 pollution in modern cities. It has been integrated in Microsoft’s Urban Aira product.
- Indoor localization. Existing indoor localization techniques are mostly based on radio-based solutions, which usually require a site survey process to obtain detailed radio signals of interested areas. However, site survey proves costly in time and manpower, limiting its usage in practice. Researchers from Tsinghua University designed LiFS,17 which is a crowdsensing application that leverages smartphones to construct the radio map of floor plans.
- Refined urban management. Smart city development relies on urban and community dynamics monitoring to provide essential information. Google launched the Waze service,b a successful MCS application in the traffic system domain. It allows drivers to share roadside events (for example, road work, traffic jams, accidents) and aggregate them on the digital map for navigation. In 2021, Didi Chuxing,c the leading mobile transportation company in China, launched a new application called “Long-distance Eye,” which allows citizens to share visual information of roadside events (for example, photos or short videos about traffic accidents) through their smartphones or dashcams. This allows for better driving plans and reduces traffic congestion. Digital Chinad is a famous smart city service provider. In 2013, they released the “Integrated Citizen Service Platform,” which allows citizens to instantly report various urban management issues they encounter (for example, pavement/sidewalk damage). A similar application is the SeeClickFixe in the U.S., which allows people to report non-emergency neighborhood issues to local governments.
- Event sensing in cities is crucial for identifying emergency/unusual events and maintaining public safety. Researchers from NPU in China have developed InstantSense,1 which leverages peoples’ photographs to obtain detailed event reports in real time.
Toward Heterogeneous Crowdsensing
MCS is a human-centered sensing paradigm, but some places are not accessible for human beings. In recent years, with the development of IoT and edge computing techniques, there are more pervasive devices equipped with sensing and computing capabilities. With the integration of these capabilities, the next-generation MCS (MCS 2.0)—Heterogeneous Crowdsensing (HCS)—is proposed. There are several distinctive features that characterize HCS (as shown in Figure 2).
Figure 2. Heterogeneous crowdsensing (MCS 2.0).
Heterogeneous participants. HCS aims to build an extended sensing and computing space with heterogeneous participants, including humans, machines, and environmental sensors. The pervasive machines involve various robots, smart vehicles, and so on, while environmental sensors consists of sensor networks.
Collaborative sensing. The sensing capabilities of different participants are distinct. Though humans are skilled in mobile sensing, environmental sensors have advantages for constant sensing in sparsely populated regions. HCS studies collaborative sensing techniques to adaptively select and aggregate the complementary sensing power from diverse participants to complete complex sensing tasks.
Augmented intelligence. A distinctive feature of HCS is that both human and machine participation is involved in the large-scale sensing process. The coexistence of human intelligence and machine intelligence, however, must be orchestrated in an appropriate and optimal manner to enhance both.
Enabling Techniques
MCS is heading toward the HCS generation, and there are several enabling techniques that help achieve this goal.
Energy-efficient collaborative sensing. MCS 2.0 leverages pervasive devices as additional participants for crowdsensing. However, energy concerns for such resource-constrained devices are emerging regarding their limited power supply and high computational needs.15 To address this issue, collaborative sensing becomes an important technique. There are currently two major methods under investigation. The first method eliminates the dependency of on-device batteries by mingling heterogeneous energy-harvesting mechanisms to harvest energy from surrounding objects.2 The second way is to replace traditional energy-consuming sensors with energy-efficient sensors regarding the spatio-temporal dependencies.8
Hybrid human-machine intelligence (HHMI). In 2017, the Chinese government issued the AI 2.0 plan.13 According to the plan, breakthroughs should be made in several basic theories of AI, including the hybrid human-machine intelligence (HHMI). HHMI aims to integrate human intelligence into an AI system to complement machine capabilities throughout its life cycle. However, the implementation of HHMI is still being explored. First, a set of theory is required for defining human and machine functions and characteristics. Second, criteria should be set to estimate the opportunity of human-machine cooperation during the continuous learning cycle. Third, the evaluation methods of the HHMI system from the perspective of both humans and machines is also an important but difficult issue. As a representative case of HHMI, a human-feedback identification model is proposed,19 which can continuously update the tree-based incremental learning model with human guidance.
HCS aims to build an extended sensing and computing space with heterogeneous participants, including humans, machines, and environmental sensors.
Operating system for crowdsensing. Regarding the vast crowdsourcing field, Amazon Mechanical Turkf has become the most successful online crowdsourcing company in the world. The core of it is a generic framework that supports various online crowdsourcing tasks (for example, translation and image labeling). Different from online crowdsourcing, MCS represent unique features such as spatio-temporality and pervasive sensing. To support rapid design and development of MCS applications in different domains, numerous platforms and frameworks are emerging. Existing MCS platforms usually address specific issues from a certain perspective, and different platforms are not compatible with each other. In 2019, researchers from NPU in China addressed this issue by drawing on the idea of ubiquitous operating systems (OS) and proposed a novel OS (CrowdOS 1.0),10 which is an abstract software layer running between the native OS and the application layer. Based on an in-depth analysis of the complex relationship among crowdsensing tasks, participants, and data, they built the OS kernel with three core modules: the Task Resolution and Assignment Framework (TRAF), the Integrated Resource Management module (IRM), and the Task Result Quality Optimization (TRO) module.
To meet the new characteristics of MCS 2.0, a new version of CrowdOS is being developed, to deal with the new challenges such as heterogeneous resource management, scheduling, and collaboration, as shown in Figure 3. The 2.0 version further enhances the platform’s compatibility and extendibility for heterogeneous MCS. As an open source MCS platform, CrowdOS shares the application installation packages, related algorithm modules, source codes, and various project documents from its website (www.crowdos.cn). To date, there have been more than 20,000 visits from 20+ countries. Based on CrowdOS, we are now collaborating with researchers from around the world (for example, Rutgers University in the U.S., Ulster University in the U.K., Waseda University in Japan) and Trustieg (the biggest open source software community in China) to launch an open source competition on innovative MCS applications. This is believed to be the first competition on open source MCS software development over the world.
Future Trends
Though we have witnessed significant development of MCS in China in recent years, there are still several issues to be tackled to further promote its growth in the MCS 2.0 era.
Strengthen the theoretical foundation. Though MCS has received much achievements at the technological level, the theoretical foundation of it is largely lagging behind. The basic scientific issues, such as the emergence of crowd intelligence (that is, the wisdom of crowds), crowd cognition mechanisms, and hybrid human-machine intelligence, are still not sufficiently explored. Therefore, one fundamental task is to study these theoretical problems and using the mechanisms/principles to improve MCS technique development.7
Embracing new technologies. The future of MCS must be aware of new technologies and applications. The emergence of AI, AIoT (AI in IoT), and Blockchain brings new opportunities and benefits to MCS. For instance, AIoT leverages compression and edge-intelligence methods to enable the usage of deep learning algorithms in resource-constrained devices.21 Blockchain, however, paves the way for trust and secure interaction among independent agents.5,18
Deployment of killer apps of MCS. MCS is still limited to a supporting role in China and not placed in a dominate position to deal with major developmental issues. By aggregating the benefits of human, machine, and IoT devices, the next generation of MCS will present grand opportunities in key national economical areas such as smart industry and social governance. Therefore, the Chinese MCS community should maintain close collaboration with industry researchers and government managers to investigate killer MCS apps to be deployed.
Conclusion
Mobile crowdsensing has rapidly developed in China during the last decade. The pervasive use of smart/wearable devices as well as the large-scale sensing requirements from different fields drives its development. Numerous enabling techniques are explored or being developed, including task allocation and worker selection, incentive mechanisms, crowdsourced data management, privacy/security protection, as well as collaborative sensing and human-machine intelligence. The availability of a generic crowdsensing platform such as CrowdOS also facilitates the research and development of MCS applications. Recently, the Chinese government released the “Fourteenth Five Year Plan and the 2035 Vision of China,” where the development of “Digital Economics” and “Digital China” has become a core mission. MCS is expected to play an important role in the coming digital economics era.
Acknowledgment
This work was partially supported by the National Key R&D Program of China (2019YFB2102200), and the National Science Fund for Distinguished Young Scholars (61725205, 62025205).
Join the Discussion (0)
Become a Member or Sign In to Post a Comment