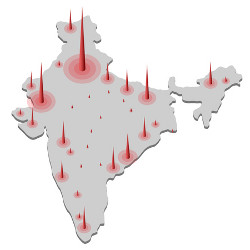
A number of models have been developed in India to forecast the spread of the coronavirus disease or COVID-19 in the country. While these have largely been variants of the classical susceptible-exposed-infectious-recovered (SEIR) compartmental model, other approaches using time-series analysis, machine-learning, network models, and agent-based simulations have also helped to provide specific insights into questions of policy. Model building has had to incorporate our evolving knowledge of the disease, including the appearance of new variants, immune escape leading to reinfections, time-varying non-pharmaceutical interventions, the pace of the vaccination program, and breakthrough infections. The predictive power of these models has been hampered by the lack of availability of quality data on infection and deaths as a function of age, the nature of social contacts, demography, and the clinical consequence of infection. An early emphasis on “ensemble models,” a thrust toward increased data availability, a greater engagement of modelers with the epidemiological and public health communities, and a more nuanced approach to communicating the limitations of modeling could have substantially increased the usefulness of models during the COVID-19 pandemic in India.
Most models were variants of the SEIR model where the individuals in the population move from S = susceptible to E = exposed to I = infectious to R = removed compartments. The time-evolution of the fraction of the population in each compartment is captured by a system of ordinary differential equations. Parameter estimation based on observables then enables the prediction of the future evolution of the pandemic.
Early in the pandemic, Bhatnager et al.19 concluded port-of-entry restrictions would achieve modest delays in the introduction of the virus, but would not be enough to prevent the COVID-19 outbreak in India. By incorporating mobility across cities using airline data, it quantified the effectiveness of quarantining symptomatic patients. Using age-stratified social contact patterns, Singh et al.23 highlighted the national three-week lock-down would not prevent a resurgence and advocated intermittent lockdowns and relaxations. Incorporating social behavioral patterns, Venkateswaran et al.25 projected a similar resurgence and advocated increased testing, tracing, and isolation to mitigate the pandemic. Modeling asymptomatic transmission, a key characteristic of COVID-19, and migration, Ansumali et al.5 concluded “herd immunity” is attained at 25% and that lockdown restricting migration is effective.
INDSCISIM15 (see Figure 1), a more sophisticated model with nine compartments and finer stratification for age-structured interaction, modeled lockdowns and other non-pharmaceutical interventions, testing variations, undercounting, and a range of age-specific infection fatality ratios. Recognizing significant spatial variation in the effective reproduction number, Ravinder et al.22 estimated the regionwise asymptomatic and undetected symptomatic COVID-19 infected fractions. District-level infection counts were estimated in Gupta et al.14 by taking into account mobility, disparate infrastructure capacities, and responses of the different states to the pandemic while Menon et al.20 studied the effectiveness of a few non-pharmaceutical interventions and Ghosh et al.12 provided 30-day shorter-term forecasts using a simpler SIS model.
Figure 1. The INDSCISIM model with several compartments, transitions, and associated transition rates. Given the large number of parameters, a Bayesian method was used to obtain optimal fits to reported cases and deaths.
The mechanistic ODE models in the works described here are large system limits of the stochastic agent-based models where the agents in the system interact with each other through their contact networks (household, workplace, schools, markets, transport spaces, among others). The interactions lead to the spread of infection in the population. Focusing on city-scale models for Bengaluru and Mumbai, Agarwal et al.4 explored the impact of various finer-grain unlocking scenarios. Considering a flu-like confounding illness coexisting with COVID-19, Gopalan et al.13 studied the impact of several testing policies and showed that location-based random symptomatic testing can capture the ground truth quite closely with very few daily tests. Recognizing that cities are spatially organized, Bhattacharyya et al.7 introduced a multilattice small world network for the city and showed local lockdowns restricted to the infected wards are effective compared to full-scale lockdowns. For such computationally intensive agent-based simulations, Agrawal4 and Kshirsagar et al.18 provide frameworks for parallelization and efficient implementation. A naïve implementation taking every edge’s effect in account would have taken O(N2) computations per time-step, where N is the size of the population. These frameworks, at the expense of not being able to tell from whom an infected individual caught the infection, reduce the computations to a manageable O(N) complexity per time-step. The mechanistic ODE models have O(1) complexity independent of the population size, and are hence scalable. The constant of course will depend on the number of compartments.
While these examples provide a description of the modeling work coming from India, some example models from the South Asian region include Chowdhury et al.,28 which is a neural network-based ANFIS and LSTM model for Bangladesh, Ali et al.,29 which is an autoregressive integrated moving average model for Pakistan, and Jayatilleke et al.,30 which is an SIR model for Sri Lanka with the contact rate modulated by a mapped stringency index. There are many more models for the region from researchers in other parts of the world that we have not discussed in this article.
Challenges
All the models described here relied on published data of infected, recovered, and deceased counts at city/district/state levels for calibration. Clearly, the model projections had significant variations among themselves and with reality. The key challenges for predictive power were:
- Data availability: Indian Council of Medical Research (ICMR) maintains a central repository with all cases data. Each state used this along with recovered and deceased data from hospital networks to publish district-wise daily numbers in varying formats. It was left to a voluntary effort, https://www.covid19india.org (see Figure 2), to provide the data in a standardized machine-readable format.
Figure 2. States of India reported via daily media bulletins.
- Data quality: The reported data were of varying granularity—some states reported at the district level and daily while some others did not. The metadata associated with the test cases were noisy because of significant variations in the nature of data collection (symptoms, vaccination, date and time of sample, test process variability, delay in reporting).
- Evolving response: The union government and the states’ responses continuously evolved as the countrywide lockdowns during March–May 2020 were followed by decentralized nonpharmaceutical and pharmaceutical interventions at the state level.
- Evolving testing capacity and policy: Detection of cases is highly dependent on testing capacity, which was seriously limited in the beginning but improved as the pandemic progressed. Further, testing policy resulted in varying tested numbers over time.
- Evolving virus: The virus continuously mutated resulting in newer variants with different transmission rates and immune escape properties.
Given these challenges, models varied significantly in their projections and performances. The heightened public attention on modeling coupled with the difficult task of communicating uncertainty quantification to the general public raised the expectations of an accurate match with reality to unrealistic levels. All these adversely impacted the adoption of models for devising effective public health responses.
Approaches
Selection. The variety of models with varying assumptions and projections led the Department of Science and Technology, Government of India, to consolidate the plethora of models into one robust ‘supermodel’ that could be “subjected to rigorous tests required for evidence-based forecasting, routinely practiced in weather forecasting communities.”9 The coordination team, see Vidyasagar et al.,26 zeroed in on one model,21 which was a variation on Ansumali et al.5 The ‘supermodel’ predicted the pandemic had peaked at the all-India level in late September 2020. The first wave and the number of modelers waned over the next few months.
Ensemble. Elsewhere, particularly in the U.S. and Europe, the respective centers for disease control took a different approach that fostered the development of ‘forecast hubs’16 and leveraged the modeling community’s expertise and enterprise. Such hubs served as platforms for modelers to submit models and projections for both the short-term and the long-term. The hub, which was launched in April 2020, collected forecasts from over 82 modeling teams into a data repository and made them easily accessible for comparison, evaluation, and guidance on response efforts. This “collect and aggregate” ensemble approach encouraged modelers to stay engaged and adapt their models to the evolving pandemic. (See the Scientific Advisory Group for Emergencies27 in the U.K. where modeling groups provided good quality monthly projections and influenced policy.)
New models November 2020–March 2021. Despite the zeroing in on the ‘supermodel,’ a few others emerged with a focus on the short-term. CSIR-4PI8 used machine learning techniques, IISC–ISI used a rudimentary log-linear fit model, and Ansumali et al.5 adapted to provide short-term forecasts. For the longer-term projections, the DST ‘supermodel’ also evolved into SUTRA3 relaxing the assumption that all symptomatic persons are detected and introducing a reach parameter to account for a growing “effectively involved” population. A PDE model was used in Ganesan et al.11 to address spatial heterogeneity.
Models varied significantly in their projections and performances.
Failure. Even though the alpha variant was active in the U.K. by the end of 2020, with cases tapering off in India, talk of hygiene hypothesis, and innate immunity, complacency had set in. When the second wave hit the country in April 2021 (see Figure 3), there was no warning from the modeling community. We did not anticipate the impact of variants, did not predict the greater severity of illness that affected hospitalization estimates, did not consider antibody waning, and did not fully comprehend the size of the susceptible population. It must be noted the immunization efforts in India began only in January 2021.
Figure 3. COVID-19 active cases in India.
Newer efforts. Spurred by the failure, the modeling effort was renewed. Several mitigation strategies for Karnataka with inclusion of mobility, antibody waning, vaccination, and its interplay with non-pharmaceutical interventions, were studied in Adiga et al.1 to provide longer-term projections including the possibility of a third wave and strategies for allocating vaccines across districts. INDSCISIM, SUTRA, the PDE model,11 and CSIR-4PI8 were revived, along with Dukkipati et al.,10 another machine-learning model based on the Hawkes process. However, the ensemble framework remained to be done.
Some Newer Approaches
In view of the severity of the second wave in April 2021, the need for an early warning system was acutely felt. Toward this, Athreya et al.6 built an early-warning system that considered district and state healthcare infrastructure capacity and provided warnings of upcoming surges when the cases were still low. It used an elementary first-order method that relied only reported data from a district and called out a warning when the local growth rate exceeding the recovery rate. Since the goal was to report up-surges quickly, the averaging window was kept small which led to false alarms. Nevertheless, the approach provided useful guidance to the state of Karnataka on the surging districts (see Figure 4). An alternative projection method was based on a comparison of the speed of the omicron variant’s surge with the delta variant’s surge in South Africa. While South Africa and India have very different social contact structures, the advantage of omicron over delta could be taken to be an invariant biological factor applicable to both South Africa and India. This suggested a way to project the omicron peaks in India (see Figure 5) and provided a means to assess the impact of weeknights and weekend curfews.2 Alongside these efforts, an Indian version of the forecast hub16 collated projections from CSIR-4PI, IISc-ISI log-linear models, machine learning models, time-series models, and a version of SUTRA (see Figure 6).
Figure 4. Early warning system for Karnataka built in early January 2022. See Athreya et al.6 for details of the method and https://www.isibang.ac.in/~incovid19/ for current status in Karnataka.
Figure 5. The model for COVID-19 cases due to Omicron variant in Bengaluru Urban district17 using a simple compartmental model.
Figure 6. India forecast hub16 has been developed to provide a common platform for modeling and forecasting teams to contribute short-term COVID-19 incident case forecasts for the states of India. The goal is to enable effective communication of available forecasts to both the public and the policymakers.
Roadmap for the Future
We have surveyed a subset of computational epidemiological modeling efforts for COVID-19 arising out of India. Our main message is we should have a National Forecast Hub featuring an ensemble of models with regular updates from the modelers. The differing assumptions and the varying predictions, all made available in one place, will enable better communication of uncertainty and a greater understanding of the applicability and the limitations of the individual models.
We should have a National Forecast Hub featuring an ensemble of models with regular updates from the modelers.
On another front, states/union-territories share testing data with ICMR. However, access to this (600+ million tests) is limited, resulting in only a few research outcomes and publications. The data is anticipated to be noisy. But the country has considerable expertise in statistical and machine learning to mine this data and gather insights. Further, this can be integrated with serosurvey data, genome sequencing, clinical data from the National Center for Disease Control, and mobile health data from platforms such as Aarogya Setu to build meaningful predictions and design better-targeted data-driven and evidence-based public health responses. These could include the treatment protocol, sampling strategy for sequencing and testing, non-pharmaceutical interventions, and vaccination strategies. We should also revisit previous waves to validate the models. To enable all this, it is imperative that India commits to data independence and to making data publicly available in a standardized format post anonymization.
We have not addressed many important items: assessing deaths due to COVID-19, sampling, testing, and sequencing protocols for sentinel surveys for enabling timely alerts, and biosurvelliance through continuous wastewater testing. Modeling will be of enhanced quality if these are incorporated.
Acknowledgments. This work was partially supported by the Centre for Networked Intelligence, the Institute of Eminence grant at the Indian Institute of Science to support an Indo-U.S. COVID-19 response effort, the SERB-MATRICS grant, and the CPDA grant at the Indian Statistical Institute.
Join the Discussion (0)
Become a Member or Sign In to Post a Comment