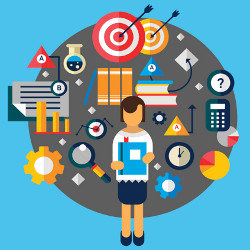
A 2014 IDC report predicted that by 2020, the digital universe—the data we create and copy annually—will reach 44 zettabytes, or 44 trillion gigabytes.10 With the explosive growth in organizational data, there is increasing emphasis on analytics that can be used to uncover the “hidden potential” of data. A 2014 Society for Information Management survey found analytics/business intelligence to be #1 among the top 15 most significant IT investments in the prior five years.12 It is not surprising that business analytics is increasingly central to managerial decision making within business functions: finance, marketing, human resources, and operations. For example, cash-flow analytics, shareholder-value analytics, and profit/revenue analytics are increasingly important aspects of the finance function. A 2017 survey of chief marketing officers found companies spend 6.7% of their marketing budgets on analytics and expect to spend 11.1% over the next three years.16 A 2017 Deloitte survey of HR managers found over 71% of the surveyed companies see people analytics as a high priority.3 Analytics is increasingly used in operations management for demand forecasting, inventory optimization, spare parts optimization, warranty management, and predictive asset maintenance. Acknowledging extreme deficiency of data literacy among today’s managers, by 2020, 80% of organizations will embark on data-literacy initiatives.15
Key Insights
- A variety of analytics applications are needed to support the various dimensions of managerial work in four business functions: finance, marketing, human resources, and operations.
- The future use of analytics in these functions will employ increasingly sophisticated types of analytics applications.
- To help business managers derive value from the data in the digital economy, analytics preparedness, as well as the design of data-literacy programs, will have to be function- and work-specific.
As analytics becomes central to decisions across finance-, marketing-, HR-, and operations-related work, it is also increasingly viewed as central to current and future continuous learning efforts within organizations. In the context of analytics-related continuous learning within different functions, managers need to better understand the trends in how different types of analytics applications are being and will be used for function-specific decision making. We thus focus on this research question: What current and future use of different types of analytics applications—static reports/interactive dashboards, descriptive analytics, predictive analytics, prescriptive analytics, and big data analytics—can help support different dimensions of managerial work—planning, implementing, and controlling—in four business functions? In 2015, we conducted a survey of 197 mid-level U.S.-based managers in finance (49), marketing (50), HR (49), and operations (49) functions on their current and future use of various analytics applications.
We found the current and future uses of various analytics applications for the four functions are different. Following our survey findings, we suggest analytics preparedness, as well as design of data-literacy programs within those functions, will need to match the use of each function. Practitioners can also use our findings to benchmark their respective organizations’ current and future use of analytics applications by business functions.
The rest of this article is organized as follows: We first characterize different types of analytics applications in the four functions, then describe three dimensions of managerial work. We present our findings on the current, or 2015, and future use, or five years out, or 2020, of different types of analytics applications to support the three dimensions. We then compare the current and future use of analytics separately in finance, marketing, HR, and operations functions, respectively.
Analytics Applications
Analytics applications are often characterized as static reports/interactive dashboards, descriptive analytics, predictive analytics, prescriptive analytics, and big data analytics. Static reports/interactive dashboards refer to database queries shown as reports and dashboards to users. Descriptive analytics employ summary statistics (such as mean, mode, and median) to characterize data and generate insights. Predictive analytics—using such tools as SAS Enterprise Miner, SPSS Modeler, and R—centers on data mining and machine learning techniques to better understand what will happen in the future based on historical data. Prescriptive analytics—simulation or optimization using such tools as @Risk and Crystal Ball—seeks to determine what should be done in the future. Big data analytics—the use of various types of data involving the Hadoop/NoSQL ecosystem—concentrates on uncovering hidden patterns and understanding previously unknown correlations, market trends, customer preferences, and other useful information from unstructured (non-tabular) data.
We first highlight the use of analytics in four functions—finance, marketing, HR, and operations—then describe the different dimensions of managerial work.
In finance, marketing, HR, and operations functions. Finance and accounting functions are the custodians and curators of financial data. As part of the accounting/finance function, collectively referred to as the “finance function” here, planning and budgeting set targets for revenue, expenditure, and cash generation, usually relying on spreadsheets.11 The objective of financial close reporting is to produce financial statements in “board book” forms and analyze financial targets. Finally, financial forecasting and modeling rely on advanced analytics, as in, say, profit-optimization capabilities that can help gauge profitability of different strategies. A 2011 research article from http://www.cfo.com/ reported deficiencies in current uses of analytics in finance, with approximately half of 231 companies surveyed in the U.S. reporting being less than “very effective” at incorporating information for strategic and operational decision making.
The marketing function has a long history of systematic use of data; for example, A.C. Nielsen measured product sales as early as the 1930s, geo-demographic data has been used since the 1970s, scanner panel data emerged in the 1980s, CRM software systems have been used since the 1990s, and user-generated content (such as online product reviews and blogs) in the 2000s produced large volumes of data.19 In 2004, Facebook pioneered an era of social network data, while smartphones with GPS capabilities (in 2007) prompted a flood of consumer-location data. With granular data, the marketing function increasingly employs analytics to develop and maintain customer relationships, personalize products and services, and automate marketing processes in real time, as through, say, recommendation systems and search marketing. More recently, images have been analyzed to classify facial features of models in advertisements.20
The HR function is primarily responsible for managing and rationalizing employment relationships through talent management1 and designing and overseeing performance appraisals.2 Major companies (such as Capital One and Dow Chemical) have used sophisticated simulation to better understand what will be required in terms of talent.1 Created during World War I, the “merit rating” system—tracking past performance through numerical scores—was developed to identify poor performers and used by 60% of U.S. companies by the start of World War II, a number that was closer to 90% in the 1960s.2 HR systems have traditionally used information concerning workers who are employed (as well as those not hired), hours worked, pay collected, and worker performance. More recent examples of HR analytics include workforce forecasting, human capital-investment analysis, and talent-value modeling.4
Operational priorities are often defined in terms of cost (such as productivity, capacity utilization, and inventory reduction), quality (such as reliability, durability, and serviceability), delivery time, and flexibility.18 Analytics is widely employed in the operations function; for example, anomaly analysis and proactive notifications can help avoid service outages; quick search across structured and unstructured data can help improve mean time to repair; supply-chain network optimization can help identify supply chain bottlenecks and support route and truckload optimization; and demand planning can help analyze customer segments in terms of channels, brands, and products down to the stock-keeping-unit level to develop models that shape demand and impact revenue.
In summary, various analytics applications are finding increasing use in a variety of business functions. In order to understand the current and future use of analytics in these functions, the next section defines managerial work in each function consistently.
In managerial work. Managerial work in business functions is often conceived as being multi-dimensional.6,7 One characterization of managerial work differentiates among planning, organizing, and controlling.7 Preventing managers from “uncritically extending the present trends into the future,” and “planning”—the first dimension—refers to integrating what the business is, what it will be, and what it should be. The “implementing” dimension refers to day-to-day organizing and daily “programming” activities. Over half of a manager’s time is spent on this dimension, making it central to managerial work.8,13 Finally, the process of work needs to be controlled, implying controls need to be embedded with respect to such aspects of performance as quality and efficiency; this dimension is often referred to as “controlling.”
Survey Methodology
Within each business function, we identified corresponding managerial tasks in three dimensions of managerial work: planning, implementing, and controlling. For example, to “develop product and market forecasts” is a planning-related task in the marketing function. We asked one manager in each function—finance, marketing, HR, and operations—to validate the managerial tasks for comprehensiveness. All managerial tasks we identified in the four functions are outlined in the online appendix “Managerial Work in Four Functions,” dl.acm.org/citation.cfm?doid=3274277&picked=formats.
For each managerial task, the survey respondent—a finance, marketing, HR, or operations manager—could choose one or more of the following types of analytics applications currently in use for conducting that task: none, static reports/interactive dashboards, descriptive analytics, predictive analytics, prescriptive analytics, and big data analytics. None indicated analytics was not used for that managerial task. They also indicated their future use of the various analytics applications for each task.
A total of 197 individuals responded to the survey from the four functions; 49 HR, 49 finance, 49 operations, and 50 marketing. Respondents had, on average, 7.9 years of work experience in their current position and 15.3 years of total work experience. Respondents worked in organizations that, on average, employed 900 full time equivalent employees. As reported in Table 1, the respondents were distributed among small- (22%), medium- (38%), and large-size (36%) organizations.
Table 1. Survey respondents ranked by organization revenue.
Figure. Current and future use of analytics applications for managerial work.
Survey Findings
We first explore the current and future use of various analytics applications for three dimensions of managerial work—planning, implementing, and controlling—then compare the current and future use of various analytics applications for each function separately.
Our overall results are outlined in the figure here as different dimensions of managerial work along the y-axis—planning, implementing, and controlling—and business functions—finance, marketing, HR, and operations—along the x-axis. For planning-related managerial work, static reports/interactive dashboards are currently, as in Figure 1a, the most commonly used analytics applications. The current use of static reports/interactive dashboards applications was 56.1%, 44.8%, 35.0%, and 36.4% for planning-related work in finance, marketing, HR, and operations, respectively. On the other hand, the current least-used analytics application was prescriptive analytics—4.9%, 5.7%, and 7.9% for planning-related work in finance, marketing and operations functions, respectively—with the exception of the HR function where the least-used analytics application for planning-related work was big data analytics (5.5%). With respect to current implementing- and controlling-related managerial work, static reports/interactive dashboards were again the most frequently used, and prescriptive analytics was least-frequently used. Static reports/interactive dashboards and prescriptive analytics are generally the most- and least-frequently used, respectively, analytics applications for managerial work.
The prominence of static reports/interactive dashboards is not surprising considering it was the traditional view of analytics in organizations in the 1990s, and organizations have since then invested in various business-intelligence and reporting initiatives.17 Static reports/interactive dashboards tend to have less rigorous technical (mathematical) sophistication, requiring little training in use and adoption. In contrast, the lower current use of more advanced prescriptive analytics can potentially be attributed to the mathematical skills (such as linear programming) required to understand and use them. Among other analytics applications, the survey results suggest big data analytics is the most frequently used in the marketing function, with 22.4%, 15.8%, and 18.7% for planning-, implementing-, and controlling-related marketing work. This is perhaps due to the need to better manage customer relationships with (big) data generated outside organizations on social media platforms and the long history of using analytics for marketing decisions.19 Predictive analytics applications are most frequently used for planning-, implementing-, and controlling-related HR work, with 21.5%, 12.7%, and 13.0%, respectively.
Comparing current and future use (see Figure 1b), it seems that, in general, static reports/interactive dashboards will still be the predominant analytics application in the near future. The exceptions include the projected use of descriptive analytics for planning- and implementing-related HR work (26.7% and 26.6%, respectively) and the use of big data analytics for controlling-related marketing work (25.2%). However, compared to current use, our respondents indicated the dominance of static reports/interactive dashboards will diminish in the future, in the range of 10.0 percentage points to 20.5 percentage points (henceforth referred to as “points”). This implies there is indeed an increasing trend toward using more advanced types of analytics applications—descriptive, predictive, prescriptive, and big data.
In the following paragraphs, for each business function we compare current and future use of different types of analytics applications with respect to a specific type of managerial work—planning, implementing, and controlling. For each such type of work, we highlight the greatest negative and positive difference in yellow and green (background), respectively, in Table 2, Table 3, Table 4, and Table 5. We excluded “None” from this consideration. Based on Likelihood Ratio Chi-square tests, we note statistically significant differences in each table. This test assumes a null hypothesis that the distribution of responses for using a particular analytics application is the same between the current and the future. An asterisk in the difference indicates there is a significant change in the number of respondents who reported their intention to use a specific analytics application in the future (five years out) vs. their current use of the same analytics application.
Table 2. Current vs. future use of analytics applications in finance management.
Table 3. Current vs. future use of analytics applications in marketing.
Table 4. Current vs. future use of analytics applications in HR management.
Table 5. Current vs. future use of analytics applications in operations management.
In finance management. With respect to planning-related finance work, static reports/interactive dashboards (56.1%) and predictive analytics (12.1%) were the top two analytics applications currently used (see Table 2). In the future, static reports/interactive dashboards (41.7%) and descriptive analytics (15.5%) will be popular applications. Comparing current to future use of analytics applications for planning-related finance work, the respondents in general indicated a significant increase in the use of advanced analytics applications in the future—descriptive (by 7.4 points), prescriptive (by 7.0 points), and big data analytics (by 4.9 points). On the other hand, static reports/interactive dashboards are projected to see the greatest drop in use (by 14.4 points).
With respect to implementing-related finance work, static reports/interactive dashboards alone account for almost two-thirds of the current analytics applications used (63.0%). Comparing current and future use of analytics applications, prescriptive analytics has the most significant increase in use (by 5.3 points), while static reports/dashboards are expected to have the most significant decrease in use (by 14.0 points).
For controlling-related finance work, static reports/interactive dashboards were the dominant application being used (64.4%) and will continue to be dominant in the future (54.1%). In the future, our respondents indicated an overall increase in the use of different types of advanced analytics applications, though the increase is only significant for prescriptive and big data analytics, by 5.0 and 3.7 points, respectively. Much like planning-and implementing-related finance work, static reports/interactive dashboards are projected to see the greatest drop in use (by 10.3 points).
By percentage points, prescriptive analytics will experience the greatest increase in implementing- and controlling-related finance work, by 5.3 and 5.0 points, respectively, and is a close second for the greatest increase in planning-related finance work (by 7.0 points) behind descriptive analytics. Our findings suggest educating finance managers in prescriptive and descriptive analytics applications will be imperative for success in their job performance. While the finance function is quantitative by nature, prior findings recognized it trailed other functions (such as marketing, operations, and HR) in its use of analytics.5 More recently though, Davenport and Tay5 provided an example of how Intel has begun initiatives in forecasting revenue and predicting impairments in its capital investments. Our findings suggest the finance function may be moving beyond static reports toward using advanced analytics more broadly.5,11
In marketing management. With respect to planning-related marketing work, our respondents indicated current use to be the highest for static reports/interactive dashboards (44.8%) followed by big data analytics (22.4%) (see Table 3). In the future, a significantly greater share of planning-related marketing work will likely involve descriptive (14.7%), predictive (20.2%), and prescriptive analytics (14.7%), with a concomitant decrease in the use of static reports/interactive dashboards (by 20.5 points).
With respect to implementing-related marketing work, static reports/interactive dashboards are currently the most frequently used analytics application (42.4%). The dominance of this type of application will decrease by 15 points in the future. On the other hand, predictive and big data analytics will see the greatest increases in the future, with overall usage at 20.4% and 23.4%, respectively.
Controlling-related marketing work follows similar current usage trends as the other two dimensions—planning and implementing. The use of static reports/interactive dashboards will again significantly decrease in the future (by 18.5 points), and this will, it seems, be replaced by a significant increase in the use of predictive (by 16.4 points) analytics. The use of prescriptive (by 4.6 points) and big data (by 6.5 points) analytics are also projected to increase significantly.
Our findings suggest marketing work is leading the use of big data analytics applications and is expected to continue in the future. Comparing current to future use, there is a general shift toward more advanced analytics applications—predictive, prescriptive, and big data—for various tasks in the marketing function, with predictive analytics experiencing the greatest percentage points increase. This suggests data-literacy programs will need to provide marketing managers with ways to incorporate predictive analytics in their work. The marketing function involves the greatest projected drop in the use of static reports/interactive dashboards among all the functions. This is consistent with prior research that suggests recommendations, geo-fencing, search marketing, and retargeting, or types of advanced analytics, are increasingly being embedded into the marketing function.19
In HR management. With respect to planning-related HR work, static reports/interactive dashboards are currently the most used (35.0%), with predictive analytics generally being the second most used (21.5%) (see Table 4). In the future, descriptive analytics will experience a significant increase (by 7.7 points), matching a similar decrease in static reports/interactive dashboards (by 10 points). We also found the use of big data analytics is expected to increase significantly (by 4.4 points).
Implementing-related HR work has a similar current usage pattern as planning-related HR work in terms of leading current and future analytics applications. In this dimension of HR work, the use of static reports/interactive dashboards will decrease significantly (by 13.2 points), along with a significant increase in the use of descriptive analytics (by 9.5 points). Additionally, the projected use of predictive analytics will significantly increase in the future (by 8.8 points) to 21.5%. While prescriptive analytics will generally continue to be the least frequently used analytics application in the future, its use will nonetheless significantly increase in the future (by 4.6 points).
Similar to implementing-related HR work, for controlling-related HR work, the data indicated similar significant increases in descriptive, predictive, and prescriptive analytics (by approximately 6 points) for controlling-related HR work and a concomitant decrease in projected use of static reports/interactive dashboards (by 11.9 points).
Overall, descriptive analytics will play a greater role in HR work, as it is expected to experience the greatest point increase for planning and implementing and be tied for the second greatest increase for controlling. This makes it an important analytics application for future data-literacy programs in HR. Given that, in 2014, 12% of U.S.-based companies, including Adobe, Juniper Systems, Dell, and Microsoft, had dropped traditional one-time annual employee appraisals, the increased use of descriptive analytics for more frequent performance appraisals is not surprising.2 Our respondents also indicated increasing use of advanced analytics applications for HR work, or predictive and prescriptive analytics, and these increases were significant for implementing and controlling dimensions. Predictive analytics applications include predicting an employee’s or a job candidate’s personality and future work performance based on their Facebook profile.14
In operations management. Static reports/interactive dashboards are the most used current analytics application for planning-related operations work (36.4%) (see Table 5). In the future, such use will decrease significantly, by 11.4 points to 25%. Predictive analytics will have a significant and almost-matching increase (by 12.3 points) and be the second most used application for planning-related operations work (21.5%). Prescriptive and big data analytics will also increase significantly, by 5.0 and 6.3 points, respectively.
With respect to implementing-related operations work, static reports/interactive dashboards were the most used analytics application (41.5%), but their use is expected to decrease significantly in the future (by 13.9 points). This decrease will be replaced primarily by a significant increase in the use of predictive analytics (by 9.4 points); additionally, future use of prescriptive and big data analytics will also increase significantly, by 3.0 and 5.7 points, respectively.
Given that analytics is increasingly being embedded in managerial work, “one size fits all” cannot be employed for domain-specific decision making.
In controlling-related operations work, while static reports/interactive dashboards are the most (42.2%) used today, this use is projected to decrease significantly by 12.3 points to 29.9%. Predictive analytics in controlling-related operations work will experience a significant (and the greatest) percentage-point increase in use (12.4 points) to 21.6%, and prescriptive and big data analytics will both increase significantly as well, by 4.4 and 5.1 points, respectively.
We note the operations function had a similar pattern of current and future analytics application use and pattern of change across all three dimensions of work. Predictive analytics is poised for the greatest share increase, and data-literacy programs will need to support this type of analytics application for operations work in the near future. The increased use of predictive analytics in operations management is potentially due to its greater use in product life-cycle management, asset management, inventory management, and service management. For example, optimal maintenance schedules can be set to reduce downtime, and alerts can be received about any imminent failure. As another example, Volvo conducts predictive, machine learning-driven analytics across petabyte-scale datasets to discern breakdown and failure rates.a
Conclusion
While widely recognized that analytics increasingly influences work in various business functions, no prior research has methodically examined how various types of analytics applications support different dimensions of managerial work in four business functions. Given that analytics is increasingly being embedded in managerial work, “one size fits all” cannot be employed for domain-specific decision making.15 Even within a business function, the prevalence of analytics applications might differ by the associated dimensions of work—planning, implementing, and controlling. Future data-literacy programs will thus need to be designed differently for each business function.
Our survey included almost 200 U.S.-based business managers to provide a nuanced perspective on the current and future use of different types of analytics applications for supporting managerial work in four business functions within an organization. Here, we offer some concluding comments regarding our findings. First, compared to current use, we found indications of significant increases in future use of advanced analytics applications. Consistent with prior studies, the survey findings suggest the diversified use of analytics applications is on the rise within all four functions. As different types of data become available for decision making (such as time-series scanner panel data, text-based user-generated content, social network data, and consumer location data19) our findings suggest that, in the future, managers will need to be engaged in different types of analytics applications as a core aspect of their responsibility.
Second, in general, we found static reports/interactive dashboards are and will continue to be a frequently used analytics application for all four functions. Our survey findings suggest managers need to continue to develop competency in their use of static reports/interactive dashboards, and business intelligence initiatives. However, their use will decrease significantly—in the range of 10 percentage points to 20.5 percentage points. This is consistent with a Gartner report that found spending on traditional business intelligence has been decreasing since 2015, with concomitant broad and pervasive deployment of self-service analytics.9 This decrease is happening as software for advanced analytics applications is becoming easier to use, and, with increased data literacy, business functions are starting to discover how more-sophisticated analytics applications can help managers accomplish their respective work. For example, dedicated applications that can predict financial riskiness of individuals based on their mouse movements while completing loan applications are now being used by the finance function.b
Third, we found the functions will differ as to which will be most used in the future. We found that the managerial work in the finance function will continue to rely on static reports/interactive dashboards the most, with projected future use at 41.7%, 49%, and 54.1% for planning-, implementing-, and controlling-related work, respectively. On the other hand, reliance on static reports/interactive dashboards is relatively lower for marketing (between 23.8% and 27.4%), HR (between 25% and 28.5%), and operations (between 25% and 29.9%) functions. HR will have static reports/interactive dashboards, descriptive analytics, and prescriptive analytics applications as the top three analytics applications. Marketing and operations can expect similar future use patterns, with static reports/interactive dashboards, predictive analytics, and big data analytics applications as their top-three analytics applications. These patterns suggest data-literacy programs and associated analytics investments will need to be function- and work-specific in the future.
Fourth, we found the functions are changing at different rates in terms of their expected future use of analytics applications for different dimensions of work. The finance function will see the greatest point increases in prescriptive analytics for implementing-related work. On the other hand, the HR function will see the greatest point increase in descriptive analytics for all dimensions of work. Both marketing and operations functions will experience the greatest point increases in predictive analytics across all dimensions of work. Our data indicates across all dimensions of managerial work, the finance function lags in today’s use of an analytics application nine times, while that lag for HR, marketing, and operations occurs four, one, and one time, respectively. However, in the future, the finance function will lag six times, while HR, marketing, and operations will lag four, five, and zero times, respectively. These results suggest some functions are more quick to embrace the capabilities of analytics to help them accomplish their work.
Finally, these survey results provide a benchmark for different functions in their current and future use of different types of analytics applications. Our findings can be used as a starting point for discussion within organizations about their current and anticipated future use of analytics to support different types of managerial work.
Acknowledgments
We thank Andrew A. Chien, Robert D. Austin, and the anonymous reviewers for providing very helpful comments that improved the quality of this article.
Join the Discussion (0)
Become a Member or Sign In to Post a Comment