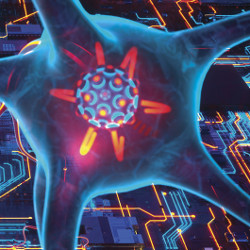
Artificial intelligence (AI) is a scientific field and a technology that is supported by multiple techniques—such as machine learning, reasoning, knowledge representation, and optimization—and has applications in almost every aspect of everyday life. We use some form of AI when we swipe a credit card, search the Web, take a picture with our cameras, give vocal commands to our phone or other device, and interact with many apps and social media platforms. Companies of every size and business model, all over the world, are adopting AI solutions to optimize their operations, create new services and work modalities, and help their professionals to make more informed and better decisions.
Key Insights
- AI ethics aims to identify and address several issues concerning the use of AI in our society, such as privacy, inclusion, robustness, transparency, fairness, and explainability, via technical, social, and sociotechnical methods.
- AI ethics has delivered principles, guidelines, tools, playbooks, educational modules, corporate policies, governance frameworks, standards, and regulations.
- Neurotechnologies collect and/or modify data from our nervous system and are rapidly being used in combination with AI.
- Lessons learned from AI ethics may offer useful insights to address neuroethical issues that may expand upon or introduce new concerns compared to AI.
Current Issues in AI Ethics
There is no doubt that AI is a powerful technology that has already imprinted itself positively on our ways of living and will continue to do so for years to come. At the same time, the transformations it brings to our personal and professional lives are often significant, fast, and not always transparent or easily foreseen. This raises questions and concerns about the impact of AI on our society. AI systems must be designed to be aware of, and to follow, important human values so that the technology can help us make better, wiser decisions. Let us consider the main AI ethics issues and how they relate to AI technology:
Data issues. AI often needs a lot of data, so questions about data privacy, storage, sharing, and governance are central for this technology. Some regions of the world, such as Europe, have specific regulations to state fundamental rights for the data subject—the human releasing personal data to an AI system that can then use it to make decisions affecting that person’s life.15
Explainability and trust. Often the most successful AI techniques, such as those based on machine learning, are opaque in terms of allowing humans to understand how they reach their conclusions from the input data. This does not help when trying to build a system of trust between humans and machines, so it is important to adequately address concerns related to transparency and explicability (see the online appendix at http://bit.ly/3CIgJ42 for examples of tools that provide solutions to these issues). For example, without trust, a doctor will not follow the recommendation of a decision-support system that can help in making better decisions for patients.
Accountability. Machine learning is based on statistics, so it always has a percentage of error, even if small. This happens even if no programmer actually made a mistake in developing the AI system. So, when an error occurs, who is responsible? To whom should we ask for redress or compensation? This raises questions related to responsibility and accountability.
Fairness. Based on huge amounts of data that surround every human activity, AI can derive insights and knowledge on which to make decisions about humans or recommend decisions to humans. However, we need to ensure that the AI system understands and follows the relevant human values for the context in which such decisions are made. A very important human value is fairness: We do not want AI systems to make (or recommend) decisions that could discriminate against or perpetuate harm across groups of people—for example, based on race, gender, class, or ability. How do we ensure that AI can act according to the most appropriate notion of fairness (or any other human value) in each scenario in which it is applied? See the online appendix for an example of an open-source library as well as for descriptions of multiple (though not exhaustive) dimensions of AI fairness. Like all ethics issues, fairness is a complex, socially influenced value that can neither be defined nor addressed by technologies alone.17
Profiling and manipulation. AI can interpret our actions and the data we share online to make a profile of us, a sort of abstract characterization of some of our traits, preferences, and values to be used to personalize services—for example, to show us posts or ads that we most likely will appreciate. Without appropriate guardrails, this approach can twist the relationship between humans and online service providers by designing the service to more clearly characterize our preferences and make the personalization easier to compute. This raises issues of human agency: Are we really in control of our actions, or is AI being used to nudge us to the point of manipulating us?
Impact on jobs and larger society. Since AI permeates our workplace functioning, it obviously has an impact on jobs, since it can perform some cognitive tasks that usually are done by humans. These impacts need to be better understood and addressed4 to ensure humans are not disadvantaged. As mentioned earlier, AI is pervasive and its applicability expands rapidly; any negative impacts of this technology could be extremely detrimental for individuals and society. The pace at which AI is being applied within (and outside) the workplace also brings concerns about people and institutions having enough time to understand the real consequences of its use and to avoid possible negative impacts.
Control and value alignment. Although AI has many applications, it is still very far from achieving forms of intelligence close to humans (or even animals). However, the fact that this technology is mostly unknown to the general public raises concerns about being able to control it and align it with larger and sometimes disparate societal values, should it achieve a higher form of intelligence.34 Figure 1 lists these issues and links them to AI capabilities and methodologies.
Figure 1. Main AI ethics issues.
No single organization can address and solve all these issues alone, which is why AI ethics includes experts from many scientific and technological disciplines. Indeed, the AI ethics community includes AI experts, philosophers, sociologists, psychologists, lawyers, policymakers, civil society organizations, and more. Only by including all the voices—those that produce AI, those that use it, those that regulate it, those that are impacted by its decisions, and those that understand how to evaluate the impact of a technology on people and society—can we understand how to identify and address AI ethics concerns.
Technical solutions to AI ethics concerns, such as software tools and algorithms to detect and mitigate bias, or to embed explicability into an AI system, are certainly necessary. But they are not enough. Non-technical solutions, such as guidelines, principles, best practices, educational and reskilling activities,12 standards,18 audits, and laws,15 are also being considered and adopted. On top of these, there is the need to specify methodologies that operationalize AI ethics principles and create appropriate governance around them.
AI and Neuroscience
As we learn more about the nervous system and untangle the embodied and bidirectional interactions between our external environments and internal milieus, the need for new tools and capabilities increases. This is not only to meet the demands of basic bench, translational, and clinical neuroscience research by creating more advanced methods and materials for capturing neural signals and computing neural features, but also to provide novel therapies, develop new ways to restore or generate human functions, and create resources to augment and enrich our existing skills and experiences. At the same time, AI’s capabilities are continuously expanding, becoming more complex, efficient, and faster due to numerous advances in computing power.
AI, and especially machine learning, is increasingly being used in neuroscience applications. But the conceptual links between AI and neuroscience have been strong since the emergence of AI as a research field. The intertwined trajectories of the two fields are exemplified by goals of emulating and augmenting human intelligence via machines that can “learn,” common and often contentious brain-is-a-computer and computer-is-a-brain tropes (and associated colloquialisms such as, “I don’t have the bandwidth” or “my computer is out of memory”), and more recent computing techniques such as neural nets, neuromorphic algorithms, and deep learning. The associations between our minds and machines are only strengthening as AI becomes embedded into nearly every aspect of our lives—from our “smart” phones to our “smart” fridges and from our shopping habits to our social media. Neuro- or brain-inspired metaphors permeate our jobs, our homes, our transportation, our healthcare, and our interactions. Neuroscience-influenced AI applications are likely to increase as big tech and startup companies invest in neuroscientific research and insights to improve algorithms and associated capabilities.
The associations between our minds and machines are only strengthening as AI becomes embedded into nearly every aspect of our lives.
The inevitable movement beyond conjectural linkages into real-world interactions between computation and neuroscience has begun. Albeit indirectly, AI already pervasively interacts with our nervous systems by influencing, reinforcing, and changing our behaviors and cognitive processes. While the concept of an “extended mind” is not new (see Clark and Chalmers8), until relatively recently, humans were largely limited to extending their thoughts into the physical realm via representations such as symbols, writings, art, or spatial markers—stored on the walls of caves, on canvases, within books and diaries, or as signs in the environment. These tools and relics functioned as repositories of ideas, memories, directional aids, and external expressions of our internal selves. Now, however, we are extending the neural into the digital,19 and the more pervasive AI and digital technologies become, the more intertwined and almost inseparable they are with our nervous systems and associated abilities and psychology.20 Numerous studies now indicate that our use of smartphones, social media, and GPS has not only made us more dependent on these technologies but has also significantly influenced our attention,39 spatial navigation,10 memory capabilities,13 and even underlying neurophysiology.21
Neurotechnology
Simultaneously, the indirect and often theoretical links between AI and neuroscience are transforming into direct and tangible ones, from one-way extensions of our minds into digital spaces to bidirectional connections between nervous systems and computers. Over the last few decades, we have seen a rise in the development and deployment of devices—called neuro-technologies (neurotech)—that exploit advances in computing and the pervasiveness of AI to collect, interpret, infer, learn from, and even modify various signals generated throughout the entire nervous system (called neuro-data or neuroinformation). Neurotechnologies can interact with neurodata either invasively and directly through different kinds of surgical implants, such as electrodes or devices implanted into or near neuronal tissues, or they can interact non-invasively and indirectly through wearable devices sitting on the surface of the skin, picking up signals or proxies of those signals from the head, body, or limbs. Generally, neurotech is divided into three categories:
- Neurosensing, which essentially “reads” neurodata by collecting, monitoring, or interpreting it.
- Neuromodulating, which “writes” data by changing the electrical activity, chemical makeup, and/or structure of the nervous system.
- Combinatorial or bidirectional, which can read and write neurodata, so to speak.
Neuromodulatory and combinatorial/bidirectional neurotech devices are areas where AI will be increasingly used and relied on to interpret neuro-data, infer and replicate proxies of neural signals in real time, and contribute to closed-loop systems for automatic and autonomous control of devices.
Figure 2 shows examples of invasive and non-invasive neurotechnology applications across neurosensing, neuromodulation, and combinatorial applications. These and other devices are being used for a gamut of applications spanning basic research, medicine, gaming, and more. For example, many of the invasive neurotechnologies are being applied in healthcare for neurological disorders including epilepsy (invasive EEG) and Parkinson’s disease (invasive neuromodulation and combinatorial). In contrast, many noninvasive technologies (including TMS and neuroimaging techniques such as fMRI) are being used to answer fundamental neuroscience questions. Noninvasive bidirectional neurotechnologies are being developed to be integrated into everyday processes, such as work and video games. In the example in Figure 2, a wrist-worn device senses peripheral neural signals, uses them to infer intended actions, and provides haptic sensory feedback to improve the experience and make it more intuitive. For an overview and to learn about many other examples, see the Royal Society’s 2019 report referenced in the online appendix.
Figure 2. Examples of invasive and non-invasive neurotechnology applications across three categories of neurosensing, neuromodulation, and combinatorial.
As neurotechnology is still emerging, the state of the art is constantly evolving, and the kinds of neurotech devices and applications present today run the gamut in terms of technological maturity, robustness, and scalability—from basic science and early translational or clinical research all the way to currently available consumer products. At present, scientists can invasively record from hundreds of neurons simultaneously. That is likely to soon become thousands with the advent and increasing adoption of interfaces such as neuralace, neural dust, and neural threads. Improvements in these interface capabilities, along with advancements in the materials used, will vastly change the future landscapes of computing and neuroscience. They will enable more accurate and specific recordings, enhanced signal-to-noise ratio, better efficacy and precision of targeted interventions, longer-lasting device functionality, and improvements to important safety considerations, including minimizing tissue damage and resisting corrosion from the internal corporeal environment, various bodily fluids, and device stimulation parameters.
Today, neurotech is most often developed or used within the clinical sciences and healthcare spaces to monitor and treat a gamut of chronic illnesses or injuries spanning neurological and psychological ailments, including Parkinson’s disease (deep brain stimulation16), chronic pain (spinal cord stimulation35), epilepsy (neuropace), depression (transcranial direct current stimulation25), and more. Some neurotech devices are beginning to restore movement and sensation in people with missing or damaged limbs;38 spinal cord severation;14 or those with sensory loss, impairments, or differences that individuals want to improve—for example, cochlear implants for deafness7 or retinal implants for certain kinds of visual impairments.5
Neurotechnologies can decode and project very specific and often rudimentary forms of thought, such as imagined handwriting,40 typing,27 and other kinds of intended and directed movements.28 They can also very crudely reconstruct conscious and unconscious mental imagery.24 These are being investigated for use primarily in medical contexts for people with communicative difficulties2 and functional movement issues28 but also more recently for the commercial space and to improve everyday work-life environments.32 Neuroscientists have also demonstrated the technological capability of brain-to-brain communication; that is, the ability to transfer sensory and perceptual experiences26 and memories11 directly between animals using invasive techniques, as well as to manipulate31 and control them.6 This capability is slowly and rudimentarily being developed and tested non-invasively between people for use in applications such as gaming and augmented reality (AR)/virtual reality (VR). Although applications are still emerging, there is evidence for widespread interest in neurotechnologies and/or neurodata collection for a variety of market sectors outside those described here, including but not limited to education, work, marketing, and military uses.
While once relegated to the realm of science fiction, the merging of machine, body, and psyche is on the horizon due to the technological advancements enabled by neuroscience and AI. However, in most of the state-of-the-art examples summarized above, additional research and an extensive amount of work is needed before these neurotechnologies can be reliably or ethically implemented. For instance, considerable improvements are required in terms of the ease and speed of acquisition and analysis of neurodata (for future scalability), the standardization of methods, the feasibility and accessibility of neurodevices (for example, to make them more intuitive, less cumbersome, more affordable, and more adaptable to differences across human bodies), the size and diversity of neuro datasets to build future representative models,30 and the validation of existing results to establish robustness and replication.
Neuroethics
Given the important implications of neurotech on society, the relative immaturity of their techniques and inferences, the increasing hype and misinformation around their abilities, and the growing direct-to-consumer push of their capabilities, there are concerns that the commercialization of neurotech and the commodification of neurodata is moving at a speed and scale that could proceed without proper policies and regulations in place to protect future consumers. Likewise, if history is any indication of the future, the increase in cautionary tales from AI applications resulting in community harms and reactionary mitigation strategies only strengthens the need to develop proactive guardrails within an AI-enabled neurotech space.
However, for agreed-upon standards and best practices to be put in place, we must first understand the ethical concerns associated with neurotechnologies and how they compare to those seen in AI. The study of ethical principles and implications related to the development, deployment, and use of neurotechnologies (and associated neuroscience research and neurodata) is commonly referred to as neuroethics, a relatively nascent but growing field of inquiry emerging in the late 1990s and early 2000s out of medical and bioethics.22 Neuroethics is critical of the assumptions and intentions underlying neurotech and neuroscientific findings. It is also concerned with questions about neurotech’s impact on human self-understanding and the downstream effects of changes in this fundamental understanding on our biology, our psychology, and our society.
AI already pervasively interacts with our nervous systems by influencing, reinforcing, and changing our behaviors and cognitive processes.
The ethical considerations surrounding neurotech are still being researched; there is yet so much to learn about the nervous system and about how, and the extent to which, neuro-technology will influence humanity. However, there are at least eight core neuroethics issues that consistently emerge which could pose significant societal, technical, and legal challenges. These are briefly defined in the following list of concepts, rights, and values that could be impacted by using neurotechnology:
- Mental Privacy: A condition met when one’s neurodata is free from unconsented observation, intrusion, interpretation, collection, or disturbance by third parties or unauthorized neuro-tech devices.
- Human Agency and Autonomy: The ability to act or think with intention, in the absence of coercion or manipulation, with sufficient information to make rational decisions regarding one’s mind and body.
- Human Identity: The subjective, complex, and dynamic embodiment of various aspects of human reality, including but not limited to biology, culture, ecology, lived experiences, and historic socio-political situations—which together give rise to each person’s unique ideas of meaning, relatedness to others and the world, and conceptions of self and ownership of life. This phenomenon is simultaneously unique and literally inscribed within the nervous system while also being influenced and constructed by external forces, such as communal/societal needs.
- Fairness (including issues of access): Equitable and just treatment of individuals or communities irrespective of their choice or ability to use neurotech or not or to participate in neurodata collection or not, as well as in manners regarding availability of neurotech; access to neurotech benefits; and/or participation in neurotech design influence, neurotech solutions, and neurodata interpretations.
- Accuracy: The correctness of neurodata measurements, interpretations provided by neurotech, or code generated by neurotech for the purpose of modifying the nervous system.
- Transparency: The quality of being clear and open about the capabilities of neurotech, the use of neurodata, and any inferences drawn from either (related to explicability as well as informed consent).
- Security: A set of technologies, standards, and procedures which protect neurodata and data inferred from neurotech against access, disclosure, modification, or destruction by unauthorized users.
- Well-being: A prioritized state of physical and mental satisfaction (including the health, safety, happiness, and comfort of individuals and/or communities) achieved through both the avoidance of negligence and the prevention of harm (broadly defined), injury, or unreasonable risk of either in the design or implementation of neurotech (or the usage of associated neurodata). This also includes elements of psychological safety as well as larger societal and environmental considerations, such as cultural or community conservation or avoidance of toxic or non-degradable waste.
Importantly, these concerns are not mutually exclusive and are considerably interrelated. For example, obtaining informed consent to collect neurodata would involve mental privacy, human autonomy and agency, transparency, and data security assurance. Likewise, well-being is met when all other concerns are sufficiently addressed. Many of these concepts also invoke previously established bioethical and medical principles related to beneficence, nonmaleficence, dignity, and justice, indicating that they are relevant to a broader range of applications outside of neurotech. In practice, responding to these concerns will often mean answering challenging questions on a contextual, socially and historically informed, case-by-case basis, as the extent of risk changes depending on:
- The neurodata of interest
- How the neurodata is being treated—for example, is it being read, written, or both?
- Whose neurodata is being collected (by whom and for what purposes)
- The overall literacy of participants and end users in the space—for instance, do they understand the neurotech’s capabilities or how sensitive their neurodata is?
- The location of the discussion or application—for example, the specific impacted community, cultural norms and values, societal expectations, associated politics and regulations, economic or financial contexts, or any environmental considerations if applicable.
Ethics in the Age of AI and Neurotech
When considering the previous list in the specific context of AI ethics, it’s clear that neurotech poses familiar ethical challenges, and both largely converge along issues of value alignment and transparency. Areas such as these, where the fields’ ethical issues overlap, are important to highlight because they suggest that some of the existing solutions or strategies (technological and otherwise) used by AI might be applied to neurotech applications to mitigate these specific concerns. However, neurotech also poses risks that may not be sufficiently covered by existing AI regulations, governance frameworks, best practices, or company policies, and this may indicate the need to update or develop new preventative strategies, policies, and solutions. The remaining neuroethics considerations fall under this kind of divergence, as they highlight challenges that while potentially shared with those posed by AI, may also be appreciably different, magnified, or expanded given the potential capabilities of neurotech and the sensitivity of neurodata.
For example, addressing mental privacy, human identity, and human agency and autonomy may be considerably more challenging in neurotech than in AI. These are of particular concern, given that neurotech could one day both directly collect neurodata and write new information into our nervous systems, all potentially without being detected. This contrasts with the current AI technologies humans interact with, which may only indirectly influence our nervous systems and associated thoughts or behaviors—or do so more slowly or at a level that is multiple steps removed. Additionally, the majority of our nervous system’s signals are unconscious and outside our awareness or control, making it technically challenging to precisely pinpoint the kinds of data neurotech collects, interprets, or modulates in the first place. Data choice, collection, and curation, however, are likely less difficult to determine or control in an AI application.
Likewise, it may also be difficult to establish what kind of neurodata we consent to share, and it might be plausible to provide neurotech with private information unknowingly or unintentionally. This is also true with most behavioral data that AI systems have access to today, but we call attention to this because there still exists a presumption of privacy within one’s own mind that seems inaccessible to others or technology. Yet, this assumption may no longer be a certainty with advances in neurotechnology. Furthermore, the fact that some neurotech can directly modify ongoing neural activity and directly feed (or write) data into the nervous system in real time raises questions about how we can better protect and ensure bodily/mental autonomy and decision capacity. This includes the potential for changing (purposefully or not, quantifiably or not) the integrity of our mental processes, including our conceptions of identity. Because neurotech may one day be able to directly influence a person’s behavior, thoughts, emotions, memories, perceptions, or relationships between these phenomena, it poses challenging questions about free will, cognitive liberty, agency, and notions of self-hood that AI may not yet have had to truly address to this extent, although there is a legacy of bioethical research showing that identity has been a fundamental concern in other biotech spaces, such as organ transplants and pharmaceutical enhancements.
Likewise, fairness is a substantial component of AI ethics and some of the same issues surrounding equitable access and inclusion in design and interpretation are also in neuroethics. But neurotech may one day allow us to directly infer and act on neurodata that we are unaware of (for example, unconscious biases or repressed memories) or cannot control, as well as significantly augment or change our mental and physical abilities. Thus, the risk to fairness is greater with neurotech, as these kinds of capabilities could perpetuate existing inequities and biases or create new avenues for discrimination or malicious targeting that are even harder to see because they are quite literally hidden inside us. Additionally, underlying and unchallenged assumptions about what constitutes “normative” neurodata or which neurotech outcomes are considered desirable may also be biased against people with hidden disabilities or neurological differences (a concern that has also been put forth when discussing genetic technologies and biometrics, among others. Normative assumptions may also contribute to complex cultural and intra-community dilemmas for similar reasons.
Moreover, neurotech interfaces may exacerbate or compound issues we currently see with AI: Device sensors do not adequately account for different hair types or skin colors, instructions and interfaces are designed in a way that widens instead of bridges the current digital or technological divides, devices are not available to or affordable for all, or neurotech benefits are not equitably distributed when appropriate. Issues of fairness are at stake not only when we consider neurotech devices that might purposely replace abilities/sensations and restore baseline functionality, but also at extremes when we consider the capability of neurotechnology to augment functions or abilities beyond those available in humans. Fairness also underlies the very conceptualizations used in this paper, as many of the ethical concerns and values listed are heavily influenced by Western norms and pedagogy, and neurodata is often produced and curated within Western European origins.30 This means that some concepts may not resonate well with or apply to different cultures, social standards, or global contexts. To make neurotech governance fairer, there needs to be a better understanding of how the social and technical concerns pertaining to neurotechnology differ, are redefined, or get reprioritized across local, national, and international communities.
Commercialization of neurotech and the commodification of neurodata are moving at a speed and scale that could proceed without proper policies and regulations in place.
At the very least, many of the issues outlined here regarding neurotech will likely be compounded with those of other technologies, including AI, due to their overlap and societal relevance. For example, AI and data science regularly contend with data privacy and de-identification practices. Depending upon the technology and data format, neurodata can be used to reasonably identify someone9 in ways that go beyond traditional PII or demographic aggregates. While this property is not unique to neurodata (it also applies to genetics and other bio-metric data types), it presents a challenge that many AI methods are not equipped to handle. It remains to be seen whether neurodata may be more technically challenging to de-identify than other data forms. Similarly, existing technological harms to well-being are complicated by neurotech applications. Like many of the technologies we use today, neurotech will also require elements that impact materials sourcing, resource allocation, energy consumption, and supply-chain operations.
However, some neurotechnologies may also have understudied interactions with the environment that AI may not have to face. For example, many medical neurotechnologies (positron emission tomography and some CT or MRI scans, for instance) require contrast agents to be able to visualize certain kinds of neurodata. Some of these contrasts, such as flourine-18, are radioactive and do not degrade in the environment while other agents, such as gadolinium, are toxic to the environment and humans over time as concentrations build. Unfortunately, due to poor waste management practices and overt pollution, they are increasingly being found in wastewater, groundwater, rivers, and oceans,33 eventually making their way into our drinking water and the food we consume—for example crops, livestock, aquatic life. This has obvious implications for the general well-being and health of our planet and our communities and only adds to the list of existing negative environmental impacts caused by technology.
Research regarding ethical concerns elicited by neurotech and AI as well as the need for action in this space is not new nor are discussions illustrating the intersections of AI with neurotech in methods and applications.36,37 However, to our knowledge, we are the first to summarize and compare the core ethical issues between both technologies, as well as offer guidance and lessons learned from the specific perspective of a tech company that actively participates in both spaces. The initial comparison of ethical concerns between neurotech and AI is summarized in the Table. Note, however, that this table may not be exhaustive; additional considerations and differences may emerge as both AI and neurotech unfold.
Figure 3 provides an example of the kinds of ethical concerns that might arise in a bidirectional neuro-tech application that also relies on AI. Specifically, the two application scenarios refer to a system that aims to prevent epileptic seizures in one example or stop Parkinson’s tremors in a different example. A neurotech component is used to read and deliver signals from/to the brain, and an AI system provides classification and prediction capabilities based on incoming data to influence the outgoing neurotech action. These types of closed-loop adaptive neurotechnologies, combined with AI, are not too far off; they are currently being developed and honed for both Parkinson’s disease3 and intractable epilepsy.23 Importantly, Figure 3 illustrates that ethical considerations are associated with both the technology capabilities (on the right) and the context in which they are embedded and used (on the left). For example, issues of fairness can be found throughout the entirety of the application, whether that is:
Figure 3. Example of a closed-loop combined neurotech and AI application scenario, and the relevant ethical concerns across contextual and technical considerations.
- Ensuring there is a representative group of patients enrolled in the trial and involving them in co-creation of treatment goals.
- Including a diverse set of engineers and designers in creating the technology and associated methods.
- Testing hardware and software on a representative set of patients to make sure neural signals are collected in the same way across individuals in future trials.
- Mitigating potential bias in the AI algorithms that learn the signals and adapt the stimulation.
- Verifying that associated neuro-modulatory effects are equitable across different groups of patients and that certain populations are not disproportionately impacted by its side effects.
- Obtaining feedback and input from a diverse set of patients, caretakers, and clinicians as part of one’s assessment of the technology’s impact.
Additionally, some ethical issues are not common throughout the process but appear only within certain contexts. For example, neuroethics concerns around human identity might be most likely to appear later in the application, after prolonged neuromodulation, and seen only through interactions with patients (see the online appendix for a real-world example). All these considerations highlight the importance and necessity of methods such as participatory design and the associated inclusion of multi-stakeholder considerations from the start—not only to help create neuro-tech that is useful for and wanted by individuals, communities, and societies at large but also to aid in identifying issues and problems before and as they arise.
Once we identify the issues around the combined use of AI and neuro-tech, how can we address them? As explained, some issues are or could potentially be greatly expanded compared to AI, so we may need to deploy updated or even new solutions and mitigation or prevention strategies to address them, both technical and not—for example, social, political, institutional, and economic approaches. However, the good news is that we do not need to start from scratch. A lot of foundational work has been completed over the past five or so years to begin addressing many AI ethics issues. We’ve constructed and used multi-stakeholder approaches to identify the concerning issues and their impacts; specified best practices, principles, and guidelines; built technical solutions which may be considered, re-used, or updated for neurotech or neurodata (for example, federated learning practices or de-biasing techniques); adopted educational/training methodologies; created governance frameworks and international standards; and even defined hard laws based on AI ethics considerations, such as the very recent one by the European Commission15). While doing all this, we have learned several lessons, identified challenges, listed the failures, and reported on successful approaches. By “we,” we mean the whole society, not just AI experts: experts from many scientific disciplines, business leaders, policymakers, and civil society organizations. Additionally, the international neuroethics community has created more than 20 ethical guidelines, principles, and best practices—compiled by the Institute of Neuroethics (IoNx)—from which we can and should draw.
Therefore, we can and should exploit this knowledge and the developed capabilities to accelerate the path toward addressing the issues raised by the combination of AI and neurotech. The first step is to clearly map the relationship between common and magnified issues, which we started doing in the previous sections and have summarized in the Table. Then, we will be able to update and augment current AI ethics frameworks and actions to cover many if not all the expanded issues. To fully understand the current state of the art in neurotech, the real implications on humans and society, and places of intersection with existing regulatory and governance strategies, we need to involve experts from other disciplines, such as neuroscience and neuroethics, that are now rarely found in existing AI ethics initiatives.19 What is considered to be “multi-stakeholder” must be greatly expanded if we want to correctly identify the issues in this broader technological/scientific context, define the relevant principles and values, and then build the necessary concrete actions. If we are to understand the breadth of neurotech applications and potential issues, conversations and considerations will need to not only include experts from neuroscience, ethics, and computer science/AI disciplines but also experts from sociology and anthropology, medicine, science and technology studies, law, business, human-computer interaction, international politics, and more. Moreover, these efforts will also require the expertise of individuals and communities most likely to use or be affected by neurotechnology, as well as those who may be excluded from use or access to the tech or who have been historically disadvantaged by technology or under-considered in tech discussions. Their needs and lived experiences should be considered as additional forms of expertise that are essential to understanding and measuring impacts of neurotechnology, as well as creating better technology and reducing harms.
Table. AI vs. neurotech ethics issues.
Conclusion
With this article, we wanted to point out the co-evolution of AI and neurotech and their potential convergence points to those who are actively thinking and working in AI and AI ethics. Our intention has been to initiate a more general conversation and clear a path to addressing the identified neuroethics issues. We hope that by identifying the core ethical issues at stake in neurotech, comparing these issues with those in AI, and highlighting places where existing AI ethics initiatives and tools may suffice or may warrant new or different approaches, we can encourage interdisciplinary and cross-stakeholder collaborations between multiple fields and communities to engender timely and concrete actions that minimize the negative impact of this emerging technology.
AI is already a powerful and often positive technology in our lives. Combined with neurotech, it will bring huge new benefits in healthcare, work, leisure, and more. But as we know, greater power comes with greater responsibilities. Knowledge should advance at the same pace as wisdom and awareness of human values and societal forces, so that technological progress can benefit all of us. Given that neurotechnologies are still emerging, there is an opportunity to continue to learn from the past, think proactively about potential issues, and develop preventative technical, legal, societal, and educational solutions before problems arise.
Figure. Watch the authors discuss this work in the exclusive Communications video. https://cacm.acm.org/videos/ai-and-neurotechnology
Join the Discussion (0)
Become a Member or Sign In to Post a Comment