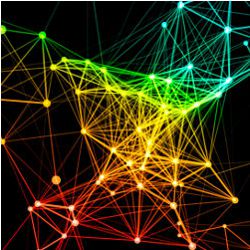
Leslie Lamport, known for his seminal work in distributed systems, famously said, “A distributed system is one in which the failure of a computer you didn’t even know existed can render your own computer unusable.” Given this bleak outlook and the large set of possible failures, how do you even begin to verify and validate the distributed systems you build are doing the right thing?
Distributed systems are difficult to build and test for two main reasons: partial failure and asynchrony. Asynchrony is the nondeterminism of ordering and timing within a system; essentially, there is no now.10 Partial failure is the idea components can fail along the way, resulting in incomplete results or data.
These two realities of distributed systems must be addressed to create a correct system. Solving these problems often leads to solutions with a high degree of complexity. This in turn leads to an increase in the probability of human error in either design, implementation, or operation. In addition, the interaction of asynchrony and partial failure leads to an extremely large state space of failure conditions. Because of the complexity of distributed systems and the large state space of failure conditions, testing and verifying these systems are critically important. Without explicitly forcing a system to fail, it is unreasonable to have any confidence it will operate correctly in failure modes.
Formal verification is a set of methods that prove properties about a system. Once these methods have been used, a system gets a gold star for being provably correct.
Formal specification languages. Individual systems and protocols can be verified using formal methods such as TLA+ and Coq.16 These are formal specification languages that allow users to design, model, document, and verify the correctness of concurrent systems. Every system under test requires the definition of the program, an environment including what kinds of failures can happen, and a correctness specification that defines the guarantees the system provides. With these tools a system can be declared provably correct.
Amazon Web Services (AWS) successfully used TLA+ to verify more than 10 core pieces of its infrastructure, including Simple Storage Service (S3).8 This resulted in the discovery of several subtle bugs in the system design and allowed AWS to make aggressive performance optimizations without having to worry about breaking existing behavior.
The typical complaint about formal specification languages is that learning them is difficult and writing the specifications is tedious and time consuming. It is true that while they can uncover onerous bugs, there is a steep learning curve and a large time investment.
Model checking. Another formal method—model checking—can also determine if a system is provably correct. Model checkers use state-space exploration systematically to enumerate paths for a system. Once all paths have been executed, a system can be said to be correct. Examples of model checkers include Spin,11 MoDIST,14 TLC,7 and MaceMC.6
Given the multitude of inputs and failure modes a system can experience, however, running an exhaustive model checker is incredibly time and resource consuming.
Composite. Some of the objections to and costs of formal verification methods could be overcome if provably correct components composed with one another to create provably correct systems. However, this is not the case. Often components are verified under different failure models, and these obviously do not compose. In addition, the correctness specifications for each component do not compose together to create a correctness specification for the resulting system.1 To prove the resulting system is provably correct, a new correctness specification must be created and the tests must be rerun.
Creating a new correctness specification for each combination of components does not scale well, especially in the microservice-oriented architectures that have recently become popular. Such architectures consist of anywhere from tens to thousands of distinct services, and writing correctness specifications at this scale is not tenable.
Verification in the Wild
Given that formal verification is expensive and does not produce components that can be composed into provably correct systems without additional work, one may despair and claim the problem seems hopeless, distributed systems are awful, and we cannot have nice things.
However, all is not lost! You can employ testing methods that greatly increase your confidence the systems you build are correct. While these methods do not provide the gold star of verified provable correctness, they do provide a silver star of “seems pretty legit.”
Monitoring is often cited as a means for verifying and testing distributed systems. Monitoring includes metrics, logs, distributed tracing systems such as Dapper12 and Zipkin,2 and alerts. While monitoring the system and detecting errors is an important part of running any successful service, and necessary for debugging failures, it is a wholly reactive approach for validating distributed systems; bugs can be found only once the code has made it into production and is affecting customers. All of these tools provide visibility into what your system is currently doing versus what it has done in the past. Monitoring allows you only to observe and should not be the sole means of verifying a distributed system.
Canarying new code is an increasingly popular way of “verifying” the code works. It uses a deployment pattern in which new code is gradually introduced into production clusters. Instead of replacing all the nodes in the service with the new code, a few nodes are upgraded to the new version. The metrics and/or output from the canary nodes are compared with the nodes running the old version. If they are deemed equivalent or better, more nodes can be upgraded to the canary version. If the canary nodes behave differently or are faulty, they are rolled back to the old version.
Canarying is very powerful and greatly limits the risk of deploying new code to live clusters. It is limited in the guarantees it can provide, however. If a canary test passes, the only guarantee you have is the canary version performs at least as well as the old version at this moment in time. If the service is not under peak load or a network partition does not occur during the canary test, then no information about how the canary performs compared with the old version is obtained for these scenarios.
Canary tests are most valuable for validating the new version works as expected in the common case and no regressions in this path have occurred in the service, but it is not sufficient for validating the system’s correctness, fault tolerance, and redundancy.
All is not lost! You can employ testing methods that greatly increase your confidence the systems you build are correct.
Unit and integration tests. Engineers have long included unit and integration tests in their testing repertoires. Often, however, these tests are skipped or not focused on in distributed systems because of the commonly held beliefs that failures are difficult to produce offline and that creating a production-like environment for testing is complicated and expensive.
In a 2014 study, Yuan et al.15 argue this conventional wisdom is untrue. Notably, the study shows that:
- Three or fewer nodes are sufficient to reproduce most failures;
- Testing error-handling code could have prevented the majority of catastrophic failures; and,
- Incorrect error handling of nonfatal errors is the cause of most catastrophic failures.
Unit tests can use mock-ups to prove intrasystem dependencies and verify the interactions of various components. In addition, integration tests can reuse these same tests without the mock-ups to verify they run correctly in an actual environment.
The bare minimum should be employing unit and integration tests that focus on error handling, unreachable nodes, configuration changes, and cluster membership changes. Yuan et al. argue this testing can be done at low cost and it greatly improves the reliability of a distributed system.
Random model checkers. Libraries such as QuickCheck9 aim to provide property-based testing. QuickCheck allows users to specify properties about a program or system. It then generates a configurable amount of random input and tests the system against that input. If the properties hold for all inputs, the system passes the test; otherwise, a counterexample is returned. While QuickCheck cannot declare a system provably correct, it helps increase confidence that a system is correct by exploring a large portion of its state space. QuickCheck is not designed explicitly for testing distributed systems, but it can be used to generate input into distributed systems, as shown by Basho, which used it to discover and fix bugs in its distributed database, Riak.13
Fault-injection testing causes or introduces a fault in the system. In a distributed system a fault could be a dropped message, a network partition, or even the loss of an entire data center. Fault-injection testing forces these failures to occur and allows engineers to observe and measure how the system under test behaves. If failures do not occur, this does not guarantee a system is correct since the entire state space of failures has not been exercised.
Game days. In October 2014, Stripe uncovered a bug in Redis by running a fault-injection test. The simple test of running kill −9 on the primary node in the Redis cluster resulted in all data in that cluster being lost. Stripe referred to its process of running controlled fault-injection tests in production as game day exercises.4
Jepsen. Kyle Kingsbury has written a fault-injection tool called Jepsen3 that simulates network partitions in the system under test. After the simulation, the operations and results are analyzed to see whether data loss occurred and whether claimed consistency guarantees were upheld. Jepsen has proved to be a valuable tool, uncovering bugs in many popular systems such as MongoDB, Kafka, Elastic-Search, etcd, and Cassandra.
Netflix Simian Army. The Netflix Simian Army5 is a suite of fault-injection tools. The original tool, called Chaos Monkey, randomly terminates instances running in production, thereby injecting single-node failures into the system. Latency Monkey injects network lag, which can look like delayed messages or an entire service being unavailable. Finally, Chaos Gorilla simulates an entire Amazon availability zone going down.
As noted in Yuan et al.,15 most of the catastrophic errors in distributed systems were reproducible with three or fewer nodes. This finding demonstrates that fault-injection tests do not even need to be executed in production and affect customers in order to be valuable and discover bugs.
Once again, passing fault-injection tests does not guarantee a system is correct, but these tests do greatly increase confidence the systems will behave correctly under failure scenarios. As Netflix puts it, “With the evergrowing Netflix Simian Army by our side, constantly testing our resilience to all sorts of failures, we feel much more confident about our ability to deal with the inevitable failures that we’ll encounter in production and to minimize or eliminate their impact to our subscribers.”5
Lineage-driven fault injection. Like building them, testing distributed systems is an incredibly challenging problem and an area of ongoing research. One example of current research is lineage-driven fault injection, described by Peter Alvaro et al.1 Instead of exhaustively exploring the failure space as a model checker would, a lineage-driven fault injector reasons about successful outcomes and what failures could occur that would change this. This greatly reduces the state space of failures that must be tested to prove a system is correct.
Conclusion
Formal methods can be used to verify a single component is provably correct, but composition of correct components does not necessarily yield a correct system; additional verification is needed to prove the composition is correct. Formal methods are still valuable and worth the time investment for foundational pieces of infrastructure and fault-tolerant protocols. Formal methods should continue to find greater use outside of academic settings.
Verification in industry generally consists of unit tests, monitoring, and canaries. While this provides some confidence in the system’s correctness, it is not sufficient. More exhaustive unit and integration tests should be written. Tools such as random model checkers should be used to test a large subset of the state space. In addition, forcing a system to fail via fault injection should be more widely used. Even simple tests such as running kill −9 on a primary node have found catastrophic bugs.
Efficiently testing distributed systems is not a solved problem, but by combining formal verification, model checking, fault injection, unit tests, canaries, and more, you can obtain higher confidence in system correctness.
Acknowledgments
Thank you to those who provided feedback, including Peter Alvaro, Kyle Kingsbury, Chris Meiklejohn, Flavio Percoco, Alex Rasmussen, Ines Sombra, Nathan Taylor, and Alvaro Videla.
Related articles
on queue.acm.org
Monitoring and Control of Large Systems with MonALISA
Iosif Legrand et al.
http://queue.acm.org/detail.cfm?id=1577839
There’s Just No Getting around It: You’re Building a Distributed System
Mark Cavage
http://queue.acm.org/detail.cfm?id=2482856
Testing a Distributed System
Philip Maddox
http://queue.acm.org/detail.cfm?id=2800697
Join the Discussion (0)
Become a Member or Sign In to Post a Comment