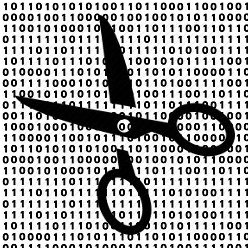
As science comes to depend ever more heavily on computational methods and complex data pipelines, many non-tenure track scientists find themselves precariously employed in positions grouped under the catch-all term "data science." Over the last decade, we have worked in a diverse array of scientific fields, specializations, and sectors, across the physical, life, and social sciences; professional fields such as medicine, business, and engineering; mathematics, statistics, and computer and information science; the digital humanities; and data-intensive citizen science and peer production projects inside and out of the academy.3,7,8,15 We have used ethnographic methods to observe and participate in scientific research, semi-structured interviews to understand the motivations of scientists, and document analysis to illustrate how science is assembled with data and code. Our research subjects range from principal investigators at the top of their fields to first-year graduate students trying to find their footing. Throughout, we have focused on the multiple challenges faced by scientists who, through inclination or circumstance, work as data scientists.
The "thorny problems" we identify are brambly institutional challenges associated with data in data-intensive science. While many of these problems are specific to academe, some may be shared by data scientists outside the university. These problems are not readily curable, hence we conclude with guidance to stakeholders in data-intensive research.
The Janitors of Science
Within data-intensive science, it is a truth universally acknowledged that a dataset in need of analysis must first be cleaned. This dirty job falls to the data scientist. Though the computational machinery of science has allowed new forms of scientific inquiry—and new kinds of scientists—to be developed, the machinery is fickle and only accepts pristine datasets. Yet the process of cleaning datasets is often hidden or rendered invisible by disciplinary and organizational divisions.14 While even the simplest dataset must be massaged prior to use, the problem multiplies when instrument calibration degrades or automated pipelines are changed without notice. One interviewee suffered an instrument malfunction during a remote sensing experiment. Unknowingly, one in an array of sensors failed out of calibration range during a field study, but the automated pipeline continued to generate data, which had to be painstakingly cleaned in the following weeks. In scientific fields that produce comparatively small amounts of data, cleaning is often done manually in a spreadsheet, and problems spotted visually, but with bigger data comes bigger spills that require bigger cleanups.
Continuing Education in Science
Early champions of "big data" infamously predicted an "end of theory,"1 arguing that with enough data and computation, all research questions become simply an abstract problem of data processing. In contrast to this anti-disciplinary discourse, we see academic data scientists struggling to master the subject expertise necessary to make competent decisions about how to capture, process, reduce, analyze, visualize, and interpret research data. Domain scientists work closely with data scientists to model scientific problems, relying on common understanding to develop a team's data pipeline and computational infrastructure. As a result, the integrity of the research process can rest with data scientists. In such settings, data scientists must develop "interactional expertise"5 by learning how to speak the jargon and conceptual vocabulary of a given discipline, and, more cogently, learning to ask the right questions of disciplinary scientists. Interactional expertise is not a skill that is readily taught in formal settings, particularly in traditional disciplinary degree programs. In response, data scientists gain interactional expertise in the fields in which they work by tactics such as making vocabulary lists of disciplinary jargon, quizzing colleagues in the hallway before a meeting, attending department seminars, taking classes, and reading literature of multiple domains.
The Overwhelmingness of Openness
Data-intensive science is increasingly tied to practices of, and policies for, "open science."12,13 Open science spans open access publications, open datasets, open analysis code, open source software tools, and much more. The concept spreads over a myriad of tools, platforms, frameworks, and practices that change often. Conflicts arise between tools that are built on open source ecosystems and controlled by a mix of public and private entities, ranging from file formats to high-performance computing infrastructures. Managing so many overlapping mechanisms can be overwhelming, especially when data scientists are hired to take the burden of maintaining infrastructures off the backs of domain researchers.11 Today's scientific training may provide solid fundamentals for early career work, but rarely provides the skills necessary to keep pace with a fast-changing, complex ecosystem. Research groups face difficult trade-offs between migrating to new tools and maintaining old packages, versions, and formats that work well enough—and are often embedded in legacy systems that must be maintained. These trade-offs can place data scientists in uncomfortable mediating positions, similar to when they must translate between different disciplines.
By bringing attention to these thorny problems, we aim to promote further discussion of the role of data science both inside and outside of data-intensive science.
Scarcity of Career Paths
Despite the rapidly growing need for data scientists in scientific research collaborations, these roles can lack specific job descriptions, and therefore a career path.7 Data scientists are often part of a research personnel pool that moves from project to project within a university. Few of these jobs lead to faculty positions or other secure career tracks. Even in scientific enterprises that invest in computational infrastructure for data, we rarely find career advancement systems that include data-specific tracks. Those exceptions we have encountered occur outside university departments, such as large-scale, globally distributed research projects with significant division of labor. The scarcity of career paths for those with combined expertise in a scientific domain and information technology results in a profound loss of research capacity for universities. Whether individuals entered academic data science jobs as a career choice or as a byway en route to a faculty post, the lack of perceived upward mobility is resulting in departures for industry or other sectors.
Managing Infrastructures for the Long Term with Short-Term Funding
Scientific infrastructures accrete over long periods of time. Laboratories are constructed, equipment acquired, staff hired and trained, software and tools developed, journals and conferences launched, and new generations of scientists educated and graduated. Data scientists are increasingly responsible for maintaining the continuity of essential knowledge infrastructures, yet projects may outlast individual grants, leaving data scientists to operate in conditions of uncertainty about the long-term future of the infrastructure they build.9 This uncertainty poses complex challenges, both in terms of anticipating the needs of future users and of sustainability. In some scientific fields, the project life cycle unfolds on the scale of decades, in distinct stages such as initial conception, setting scientific goals, designing data management systems, constructing instruments and facilities, collecting data, processing data through pipelines, and releasing "science ready" data to the community. Builders of scientific infrastructure must make decisions in the present that will affect what data is collected and made available for decades, opening up some potential avenues of inquiry and closing down others.2 Data-intensive science is plagued by the tyranny of small decisions; choices optimal in the short term may create a thorny nest of complications five or 10 years later.
Untangling Thorny Problems
The data-intensive science problems we have outlined here are intertwined with the organizational and funding of science within the university system.6 They only exist, and can only be addressed, within these larger institutional and political constraints. The specific circumstances of data science activities vary widely between and within the physical, life, biomedical, and social sciences; engineering, humanities, and other fields. Scientific practices in all of these fields are in flux, requiring new tools and infrastructures to handle data at scale, and grappling with new requirements for open science. Some individuals choose data science jobs in universities, but often the job finds them. Learning data science may be an investment that leads to a productive career, but all too often, time spent as the "data person" or "computer person" on the science team is labor not spent on dissertations, publications, or the scientific research that launches a tenure-track career.
These scientific environments have high personnel turnover rates, with individuals working in data science capacities through sequential post-doctoral fellow or grant-funded research scientist positions, or leaving for jobs in the corporate sector. Labor statistics are unlikely to capture the growth or turnover rate of these positions in science because the work is hidden behind so many different job titles. It is difficult to assess the damage to scientific progress when trusted data scientists move on to other institutions, as the losses may become apparent only months or years later. No matter how well code is documented, no paper trail can substitute for the rich domain expertise and tacit knowledge of those who conducted the science.4,10
By bringing attention to these thorny problems, we aim to promote further discussion of the role of data science work both inside and outside of data-intensive science. Our list of problems is by no means exhaustive and our proposed remedies by no means complete. We offer our vignettes in the spirit of diagnosis and invite data scientists working in other fields, disciplines, and industries to contribute their own sets of thorny problems and solutions. We have written from the point of view of academic science as one permutation of data science, a term that escapes easy definition even as it advances. Much work remains.
Join the Discussion (0)
Become a Member or Sign In to Post a Comment