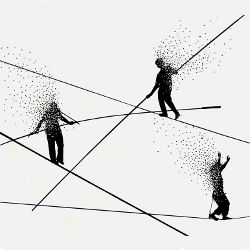
For years, the key ethic for safe, sustainable data sharing was anonymization. As long as a researcher or organization took steps to anonymize datasets, they could be freely used and shared. This notion was even embedded in law and policy. For example, laws like the Health Insurance Portability and Accountability Act (HIPAA) Privacy Rule and the European Union’s Data Protection Directive facilitate sharing of anonymized datasets with fewer if any restrictions placed upon datasets that contain personal information.
But it turns out that "anonymization" is not foolproof. The possibility of correctly identifying people and attributes from anonymized datasets has sparked one of the most lively and important debates in privacy law. In the past 20 years, researchers have shown that individuals can be identified in many different datasets once thought to have been fully protected by means of de-identification.a,7 In particular, a trio of well-known cases of re-identification has called into question the validity of the de-identification methods on which privacy law and policy, like the HIPAA privacy rule, relies. A governor and Netflix and AOL customers were all accurately identified from purportedly anonymized data. In each case, an adversary took advantage of auxiliary information to link an individual to a record in the de-identified dataset.
The failure of anonymization has been widely publicized. But the debate over how to proceed in policy and practice remains stalled. In order to find the right path, the perfect cannot be the enemy of the good. Anonymization must be conceptualized as a process of minimizing risk instead of a state of guaranteed safety.
A Crisis of Faith and Scientific Discord
The possibility of correctly identifying people and attributes from de-identified datasets has sparked a crisis of faith in the validity of de-identification methods. Do these methods still protect data subjects against possible privacy harms associated with revealing sensitive and non-public information? Certainly, there is widespread skepticism about de-identification techniques among some leading privacy scholars and most of the popular press, which in turn undermines the credibility of the exemptions for de-identified data in regimes like HIPAA. This is of obvious concern because it not only creates legal and regulatory uncertainty for the scientific research community but may even discourage individuals from contributing data to new research projects. (It also heightens consumer mistrust of e-commerce firms offering their own dubious "guarantees" of anonymization, thereby reinforcing the "privacy is dead" meme.)
The community of computer scientists, statisticians, and epidemiologists who write about de-identification and re-identification are deeply divided, not only in how they view the implications of the auxiliary information problem, but in their goals, methods, interests, and measures of success. Indeed, we have found that the experts fall into two distinct camps. First, there are those who may be categorized as "pragmatists" based on their familiarity with and everyday use of de-identification methods and the value they place on practical solutions for sharing useful data to advance the public good.1 Second, there are those who might be called "formalists" because of their insistence on mathematical rigor in defining privacy, modeling adversaries, and quantifying the probability of re-identification.6 Pragmatists devote a great deal of effort to devising methods for measuring and managing the risk of re-identification for clinical trials and other specific disclosure scenarios. Unlike their formalist adversaries, they consider it difficult to gain access to auxiliary information and consequently give little weight to attacks demonstrating that data subjects are distinguishable and unique but that (mostly) fail to re-identify anyone on an individual basis. Rather, they argue that empirical studies and meta-analyses show that the risk of re-identification in properly de-identified datasets is, in fact, very low.
Formalists, on the other hand, argue that efforts to quantify the efficacy of de-identification "are unscientific and promote a false sense of security by assuming unrealistic, artificially constrained models of what an adversary might do."6 Unlike the pragmatists, they take very seriously proof-of-concept demonstrations of re-identification, while minimizing the importance of empirical studies showing low rates of re-identification in practice.
This split among the experts is concerning for several reasons. Pragmatists and formalists represent distinctive disciplines with very different histories, questions, methods, and objectives. Accordingly, they have shown little inclination to engage in fruitful dialogue much less to join together and find ways to resolve their differences or place de-identification on firmer foundations that would eliminate or at least reduce the skepticism and uncertainty that currently surrounds it. And this makes it very difficult for policy makers to judge whether the HIPAA de-identification rules should be maintained, reformed, or abandoned.
These divergent views might lead us to different regulatory approaches. Those that focus on the remote possibility of re-identification might prefer an approach that reserves punishment only in the rare instance of harm, such as a negligence or strict liability regime revolving around harm triggers. Critics of anonymization might suggest we abandon de-identification-based approaches altogether, in favor of different privacy protections focused on collection, use, and disclosure that draw from the Fair Information Practice Principles, often called the FIPPs.
These problems with the de-identification debate are frustrating sound data use policy. But there is a way forward. Regulators should incorporate the full gamut of Statistical Disclosure Limitation (SDL) methods and techniques into privacy law and policy, rather than relying almost exclusively on de-identification techniques that only modify and obfuscate data. SDL comprises the principles and techniques that researchers have developed for disseminating official statistics and other data for research purposes while protecting the privacy and confidentiality of data subjects. SDL can be thought of in terms of three major forms of interaction between researchers and personal data: direct access (which covers access to data by qualified investigators who must agree to licensing terms and access datasets securely); dissemination-based access (which includes de-identification), and query-based access (which includes but is not limited to differential privacy).5
Adopting the SDL frame for the de-identification debate helps to clarify several contested issues in the current debate. First, the most urgent need today is not for improved de-identification methods alone but also for research that provides agencies with methods and tools for making sound decisions about SDL. Second, the SDL literature calls attention to the fact that researchers in statistics and computer science pursue very different approaches to confidentiality and privacy and all too often do so in isolation from one another. They might achieve better results by collaborating across methodological divides. Third, the legal scholars who have written most forcefully on this topic tend to evaluate the pros and cons of de-identification in isolation from other SDL methods. Debates focusing exclusively on the merits or demerits of de-identification are incomplete. SDL techniques should be part of most regulators’ toolkits.
The Way Forward: Minimizing Risk
Most importantly, SDL can be leveraged to move de-identification policy toward a process of minimizing risk. A risk-based approach would seek to tailor SDL techniques and related legal mechanisms to an organization’s anticipated privacy risks. For example, if the federal agency administering the HIPAA Privacy Rule (Health and Human Services) fully embraced a risk-based approach, this would transform the rule into something more closely resembling the law of data security.4 Such an approach would have three major features:
Process-based: Organizations engaged in releasing data to internal, trusted, or external recipients should assume responsibility for protecting data subjects against privacy harms by imposing technical restrictions on access, using adequate de-identification procedures, and/or relying on query-based methods, all in combination with legal mechanisms, as appropriate.
Contextual: Sound methods for protecting released datasets are always contingent upon the specific scenario of the data release. There are at least seven variables to consider in any given context, many of which have been previously identified in reports by the National Institute of Standards and Technology (NIST) and others. They include data volume, data sensitivity, type of data recipient, data use, data treatment technique, data access controls, and consent and consumer expectations.
Statistical Disclosure Limitation can be leveraged to move de-identification policy toward a process of minimizing risk.
Tolerant of risk: The field of data security has long acknowledged there is no such thing as perfect security. If the Weld, AOL, and Netflix re-identification incidents prove anything, it is that perfect anonymization also is a myth. By focusing on process instead of output, data release policy can aim to raise the cost of re-identification and sensitive attribute disclosure to acceptable levels without having to ensure perfect anonymization.b
Organizations sharing data should be required to provide "reasonable data release protections." The tenets of reasonable, process-based, data-release protections would look similar to those of data security: assess data to be shared and risk of disclosure; minimize data to be released; implement reasonable de-identification and/or additional data control techniques as appropriate; and develop a monitoring, accountability, and breach response plan.
These requirements would be informed by the nascent industry standards under development by NIST and others, including accepted de-identification and SDL techniques as well as a consideration of the risk vectors described here.2 Of course, those who engage in unauthorized re-identification are also culpable and it might be worthwhile to supplement contractual or statutory obligations not to engage in re-identification with severe civil (or even criminal) penalties for intentional violations that cause harm.3 It is important that any such statutory prohibitions also include robust exemptions for security research into de-identification and related topics.
A risk-based approach recognizes there is no perfect anonymity. It focuses on process rather than output. Yet effective risk-based data release policy also avoids a ruthless pragmatism by acknowledging the limits of current risk projection models and building in important protections for individual privacy. This policy-driven, integrated, and comprehensive approach will help us better protect data while preserving its utility.
Join the Discussion (0)
Become a Member or Sign In to Post a Comment