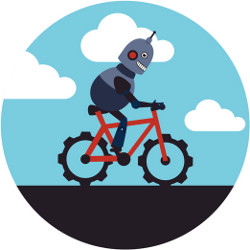
In his 2019 Turing Award Lecture, Geoff Hinton talks about two approaches to make computers intelligent. One he dubs—tongue firmly in cheek—”Intelligent Design” (or giving task-specific knowledge to the computers) and the other, his favored one, “Learning” where we only provide examples to the computers and let them learn. Hinton’s not-so-subtle message is that the “deep learning revolution” shows the only true way is the second.
Hinton is of course reinforcing the AI Zeitgeist, if only in a doctrinal form. Artificial intelligence technology has captured popular imagination of late, thanks in large part to the impressive feats in perceptual intelligence—including learning to recognize images, voice, and rudimentary language—and bringing fruits of those advances to everyone via their smartphones and personal digital accessories. Most of these advances did indeed come from “learning” approaches, but it is important to understand the advances have come in spheres of knowledge that are “tacit”—although we can recognize faces and objects, we have no way of articulating this knowledge explicitly. The “intelligent design” approach fails for these tasks because we really do not have conscious theories for such tacit knowledge tasks. But, what of tasks and domains—especially those we designed—for which we do have explicit knowledge? Is it forbidden to give that knowledge to AI systems?
Figure. “Human, grant me the serenity to accept the things I cannot learn, data to learn the things I can, and wisdom to know the difference.”
The polymath Polanyi bemoaned the paradoxical fact that human civilization focuses on acquiring and codifying “explicit” knowledge, even though a significant part of human knowledge is “tacit” and cannot be exchanged through explicit verbal instructions. His “we can know more than we can tell” dictum has often been seen as a pithy summary of the main stumbling block for early AI efforts especially in perception.
Polanyi’s paradox explains to a certain extent why AI systems wound up developing in a direction that is almost the reverse of the way human babies do. Babies demonstrate aspects of perceptual intelligence (recognizing faces, voices and words), physical manipulation (of putting everything into their mouths), emotional intelligence, and social intelligence, long before they show signs of expertise in cognitive tasks requiring reasoning skills. In contrast, AI systems have demonstrated reasoning abilities—be they expert systems or chess—long before they were able to show any competence in the other tacit facets of intelligence including perception.
In a sense, AI went from getting computers to do tasks for which we (humans) have explicit knowledge, to getting computers to learn to do tasks for which we only have tacit knowledge. The recent revolution in perceptual intelligence happened only after labeled data (such as cats, faces, voices, text corpora, and so forth) became plentiful, thanks to the Internet and the World Wide Web, allowing machines to look for patterns when humans are not quite able to give them explicit know-how.
Lately though, Polanyi’s paradox is turning into Polanyi’s revenge both in research and practice of AI. Recent advances have made AI synonymous with learning from massive amounts of data, even in tasks for which we do have explicit theories and hard-won causal knowledge.a This resistance to accept any kind of explicit knowledge into AI systems—even those operating in tasks and environments of our design—is perplexing. The only “kosher” ways of taking explicit knowledge in deep learning systems seem to be to smuggle them in through architectural biases, or feeding them manufactured examples. Anecdotal evidence hints that industry practitioners readily convert doctrine and standard operating procedures into “data” only to have the knowledge be “learned back” from that data. Even researchers are not immune—a recent paper in Nature Machine Intelligence focused on how to solve Rubik’s Cube by learning from billions of examples, rather than accept the simple rules governing the puzzle. There are policy implications too. Many governmental proposals for AI research infrastructure rely exclusively on creating (and curating) massive datasets for various tasks.
The current zeal to spurn hard-won explicit (and often causal) knowledge, only to try to (re)learn it from examples and traces as tacit knowledge, is quixotic at best. Imagine joining a company, and refusing to take advice on their standard operating procedures, and insisting instead on learning it from observation and action. Even if such an approach might unearth hidden patterns in how the company actually works, it will still be a wildly inefficient way to be an employee. Similar concerns will hold for AI assistants in decision support scenarios.
A common defense of this “learning first” trend is the asymptotic argument that since we humans—with an essentially neural basis of their brains—have managed to develop shared representations and ability to communicate via explicit knowledge, AI systems based purely on learning may well be able to get there eventually. Perhaps. But it is quite clear that we are far from that point, and a misguided zeal to steer away from AI systems that accept and work with explicit knowledge is causing a plethora of problems right now.
Indeed, AI’s romance with tacit knowledge has obvious adverse implications to safety, correctness, and bias of our systems. We may have evolved with tacit knowledge, but our civilization has been all about explicit knowledge and codification—however approximate or aspirational. Many of the pressing problems being faced in the deployment of AI technology, including the interpretability concerns, the dataset bias concerns as well as the robustness concerns can be traced rather directly back to the singular focus on learning tacit knowledge from data, unsullied by any explicit knowledge taken from the humans. When our systems learn their own representations from raw data, there is little reason to believe that their reasoning will be interpretable to us in any meaningful way. AI systems that refuse to be “advised” explicitly are taking the “all rules have exceptions ” dictum to the “what are rules?” extreme, which flies in the face of civilizational progress, and seriously hinders explainability and contestability of machine decisions to humans in the loop.
How confident can we be of a medical diagnostic system using AI, when it shares little common knowledge beyond raw data with the presiding physician? This is no longer a hypothetical. Just recently, a paper in JAMA Dermatology showed that a commercially approved AI system for melanoma detection was easily mislead by surgical skin markings next to benign moles. Wittgenstein was alluding to this at some level, when he remarked “if a lion could speak, we could not understand him.”
At least part of the problem, in terms of public perceptions, is our own very human romance with tacit knowledge, which continues despite the fact that the progress of civilization depended on explicit knowledge. We tend to romanticize je ne sais quoi and ineffability (no one ever impressed their life mate by “explaining” their love with a crisp numbered list of falsifiable attributes!). This very human trait makes feats of AI systems that learn without being told all that much more fascinating to us (nevermind their inscrutability and attendant headaches).
While we are easily impressed at computer performance in tasks where we have no conscious models and explicit knowledge (for example, vision, speech), there are also many domains, especially those designed by humans, where we do have models and are willing to share them! Indeed, the hallmark of human civilization has been a steady accumulation of such explicit knowledge. After all, many animals have perceptual abilities that are more acute than we humans have, but we got much farther because of our ability to acquire and use explicit knowledge, rather than learn only from observation. It is important for AI systems to be able to take such knowledge when it is readily available, rather than insist on rediscovering it indirectly from examples and observation. There should be no shame in widening the pipeline between humans and AI systems and accepting readily offered knowledge, be it explicit norms and rules, causal models or shared vocabulary.
Of course, combining learning and explicit knowledge in a fully principled way continues to be an open problem. Often the explicit knowledge may only provide an initial bias that gets refined through learning. To do this effectively, we will need to go beyond ways to smuggle knowledge through model architectures. While we are busy trying to make headway on that problem however, we should at least resist the temptation to stigmatize acquisition and use of explicit knowledge.
We found it to be fruitless to insist on explicit knowledge for tacit tasks such as face recognition. It will be equally futile to ignore readily available explicit knowledge and insist on learning/recovering it from examples. Our machines must have the wisdom to know when to take advice and when to learn.
Join the Discussion (0)
Become a Member or Sign In to Post a Comment