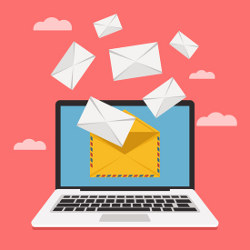
Vinton G. Cerf wonders "whether there is any possibility of establishing 'watcher networks'" in his October 2022 Communications "Cerf's Up" column. I must point out to all who have the same concern about "who will watch the watchers" that Philip K. Dick describes this problem in his story The Minority Report (see wikipedia https://bit.ly/2XlQcSA) where a group of three precogs (organic versions of AI) attempt to predict the future. It works often, but not always. So the question arises: How much authority are we willing to provide for AI and is the concept of three AIs working independently on the same problem a feasible solution. I agree with Cerf that we need to come up with a solution before the problem overwhelms us.
Tom Jones, Seattle, WA, USA
In the December 2022 Communications, there is a compelling column by Vinton G. Cerf, "On Truth and Belief," which exemplifies the growing worry about agreement, polarization, and the nature of truth. Even though I share the sentiments of Cerf, I believe his final assessment of the situation is counterproductive to his objectives, and by propagating this proposition, Cerf is diminishing the alternatives to the current state of affairs. As Cerf writes, "Perhaps critical thinking should be part of every educational curriculum from the earliest stages." There are two moments in this phrase worth discussing: criticism and education.
Let's start with the latter. A trend in contemporary politics is the attempt to solve societal issues by delegating them to the educational process. If there is any kind of habit, belief, skill, or competency that might be seen as fruitful for a society to come, the knee-jerk reaction is to add it to the educational curriculum. The solution is to make children acquainted with such an objective from early on, for example, to make the coming generation responsible for a political project of the current generation. This movement, however, represents a lack of responsibility of the current generation in taking the matter into their own hands. Perhaps, instead of proposing critical thinking in the educational curriculum, Cerf should propose to his readers the creation of reading groups, places for discussion, community centers, where the values and activities encompassed by his "critical thinking" can be exercised by people of his generation, our generation, thus taking the responsibility for fighting the erosion of agreement into our own hands.
More criticism, however, is possibly not what we need. Even though Cerf links criticism to agreement, he overlooks the fact that more and more criticism has become the very source of disagreement. Criticism, as the movement of finely analyzing arguments, their pre-supposition and their pragmatic consequences, even when well executed, has led toward debunking, skepticism, and suspicion. These are the same ingredients that brew conspiracy theories. The enlightened republic of letters is hardly returning in the age of digital media. If the option is to be a cynic critic, I believe agreement can only come in being non-critic, or perhaps post-critic. I posit the necessity of searching for new ways of engaging with discourse, truth and belief, instead of referring to the exhausted concept of criticism as a future possibility for agreement.
More criticism, however, is possibly not what we need.
In writing these final lines, Cerf has certainly embodied the opinions of many readers. These ideas, as I attempt to have briefly sketched, are not the optimal direction for the achievement of agreement and dialogue. By writing this letter, I hope to put these ideas to debate and perhaps ignite new thoughts on the considered matter.
Luiz do Valle Miranda, Kraków, Poland
Author's response:
Thanks to Tom Jones for his reference to The Minority Report—an important reminder!
Luiz do Valle Miranda seems to have mistaken my use of "critical thinking" for "criticism." Critical thinking is closest to the practices of science in which one asks important questions about the sources and quality of information/data and also tests theories with falsifying experiments. Critical thinking is what keeps us from taking as true, assertions that are without basis. I think all ages benefit from this kind of thinking. Critical thinking is not criticism in my dictionary.
Vinton G. Cerf, McLean, VA, USA
Research and Practice
The July 2022 issue of Communications has two advertisements on its first few pages. One raised the issue "Today's research driving tomorrow's technology" and the very next one spoke of a new book, Theories of Programming.
Reading further into the issue, I came to Jeanna Matthews' contribution challenging her readers to "consider reaching out with ideas for her column regarding Viewpoints." All of which caused me to consider the implications of all of that. An issue of great importance to me is the issue of the relevance of computing theory to computing practice. Does today's research really drive tomorrow's technology? Do the theories of programming make a contribution to practical programming? I do not know the answers to those questions, but I would sure like to.
It occurred to me that these are research questions, perhaps the most important research questions in the computing field. Does computing research/theory contribute to improvements in computing practice? If so, how? If not, why not? My own bias, as a dedicated practitioner, is that theory is all too often irrelevant to practice. But that is a personal bias, and I would like to see that bias evaluated in a proper way.
The last time I saw any data, readers and members of Communications and ACM are predominantly practitioners. And the authors of the content of Communications are predominantly theorists. That tends to mean that Communications is written by one audience but intended for another. Therein, of course, lies a problem.
I think answering my questions is a legitimate goal of the ACM flagship publication, Communications of the ACM. The question is, of course, who among our theorist authors is willing to explore these questions.
Robert L. Glass, Nordland, WA, USA
Editor-in-Chief's response:
Robert Glass raises several important questions in his letter. Let me respond with several of my own.
First, what is a CS theoretician? Aside from the actual field of theory, most computer scientists that I know build systems and run experiments. Many of them go even further and try to turn their ideas into tangible products by creating startups. The dividing line between research and practice in our field seems fuzzier and less well defined than in other scientific disciplines, perhaps because we create what we study. For example, the book Glass calls out (Theories of Programming) is the collected works of Tony Hoare, who invented both Quicksort and null pointers and produced seminal work on programming language semantics. Practitioner? Researcher? Theoretician?
Second, why are more practitioners not members of ACM (and readers of Communications)? To me, this is the existential question for ACM, given that its membership has held constant for more than a decade while the number of people with CS degrees has grown exponentially. Why have our students not joined ACM? Is there a better organization, magazine, or website for keeping up to date with our fast-changing field? Do practitioners not feel a need to stay up to date? Or, is ACM/Communications not relevant to the professional interests of practitioners?
These are all important questions, and I welcome the readers' perspectives on them.
James Larus, Editor-in-Chief, Communications of the ACM, Lausanne, Switzerland
Let's Build on Cybersecurity Technology Success
Moshe Y. Vardi's November 2022 Communications editorial "Accountability and Liability in Computing" about today's cyber-insecurity is to be applauded for distinguishing the success of "technical solutions" from "the market-failure issue." I have long made much the same distinction as reported in the May/June 2022 IEEE Security & Privacy blogpost "High Assurance in the Twenty-First Century", but with a very different conclusion regarding "technical solutions." Vardi asserts that 75 years into the computer age we still do not know how to build secure information systems. In contrast, I note that during the past 50 years the Security Kernel technical solution has "been a technology success, and a market failure."
What is the evidence of technology success?
What is the evidence of technology success? Five years ago, my November 2016 Communications Viewpoint "Cyber Defense Triad for Where Security Matters" pointed out "Security for cyber systems built without a trustworthy operating system (OS) is simply a scientific impossibility." The tech industry (like Microsoft) has asserted that this technology is "not able to cope with systems large enough to be useful." I responded that "this quite widely spread assertion has been repeatedly disproven by counterexamples from both real systems and research prototypes." Those included Security Kernels for SACDIN Minuteman missile control, NSA's BLACKER Internet cryptographic system, and commercial GEMSOS for primary IT for the Pentagon. The Viewpoint noted Security Kernel technology was applied for successful "deployments of highly secure systems and products, ranging from enterprise 'cloud technology' to general-purpose database management systems (DBMS) to secure authenticated Internet communications". With respect to security, it points out "At least a half-dozen security kernel-based operating systems have been produced that ran for years (even decades) in the face of nation-state adversaries without a single reported security patch."
It is disappointing Vardi did not even acknowledge, let alone discuss, this contradicting evidence. I realize there are a couple of "sacred cows" that may also discourage authors from mentioning this technology: its demand for specific hardware support and its tension with open source ideology. Although segmentation and protection ring hardware were introduced for Multics security more than 50 years ago, Intel included them for security in their still ubiquitous x.86 architecture.
So why should we care that a highly respected expert source such as a Communications editorial did not mention a contradictory perspective? It is hardly surprising that an author emphasizes what is deemed supportive to a particular advocated direction—in this case "Accountability and Liability in Computing." The voice of such experts significantly influences the perception of "a lack of technical solution … which disincentivizes those who may be able to fix serious security vulnerabilities from doing so." As I discuss in some detail in the IEEE Security & Privacy blogpost, it is most important that designers and manufacturers know they can build highly secure information systems on a Security Kernel-based trustworthy operating system, as has been repeatedly done in the past. This is affordable, commercially available technology with demonstrated good performance.
Roger Schell, Pacific Grove, CA, USA
Author's response:
My Communications columns are one-page contributions. Under these constraints, there is no room for a scholarly review. Rather each column aims at making one point. In the column in question the point was the lack of accountability in computing due to lack of liability. I do not think that Schell and I are in disagreement. He argues that technical foundations already exist to build secure information systems, while I argued the slow progress in cybersecurity is due to market failure, which disincentivizes those who may be able to fix serious security vulnerabilities from doing so.
Moshe Y. Vardi, Senior Editor, Communications of the ACM Houston, TX, USA
What Is Expectability in Prediction Modeling?
In the November 2022 Communications India region special section "Hot Topics" contribution, "Toward Explainable Deep Learning," Vineeth Balasubramanian emphasized the necessity of achieving high levels in all three components of any algorithm: explainability, interpretability, and transparency. These components are even more crucial in health care compared to other domains. The computational models that are often widely used play important roles in decision support in, for example, liver allocation and cardiovascular risk assessment.
I would like to propose a fourth component denoted as "expectability." When a first machine learning prediction model is trained using a first subpopulation, and a second machine learning prediction model is trained using a second subpopulation, an expected behavior is observed if each model performs the best on the subpopulation it was trained on. For example, an unexpected behavior of the first machine learning model would be if the model yielded higher discrimination performance and/or closer-to-optimal calibration performance compared to the second model when deployed on the subpopulation the second model was trained on. Expectability would be achieved if any model trained on a certain subpopulation would always perform the best on similar subpopulations compared to any other models.
In analyses applied on the Explorys Life Sciences Dataset (an electronic medical record repository with over 21 million patients), we realized the widely used risk assessment tool in cardiology, the Pooled Cohort Equations, does not always behave as expected.1 I led this research effort in collaboration with IBM Research and the Broad Institute of MIT and Harvard. The research revealed that one of the four equations, the one designed to predict outcomes on the Black men subpopulation, achieves the best calibration performance compared to the other three models when deployed on the three subpopulations it was not designed to function on. We explored the spectrum of possibilities to explain this unexpected behavior and realized that several factors affecting calibration performance must be considered when developing risk assessment tools, which include variability between models such as the total number of coefficients, the size of the coefficients, and how close the coefficients are to zero in each model. Additional considerations must include skewness assessment, which relies on measures such as prevalence and predicted risk scores of patients in different risk ranges of the training and testing sets.
Any future prediction models must include assessments of expectability.
Any future prediction models must include assessments of expectability to evaluate expected behavior, using a variety of subpopulations and performance measures. High levels of expectability may even be most crucial because a model that performs well could be explainable, interpretable, and transparent; however, that model would still be suboptimal if it behaves unexpectedly.
Uri Kartoun, Cambridge, MA, USA
Author's response:
Thank you for sharing this interesting perspective. The notion of "expectability" of machine/deep learning (ML/DL) models is, beyond doubt, critical. The DL research community has been viewing this from different perspectives such as model error calibration4 (also see https://bit.ly/3PwdodG) conformal prediction (we have a book on this topic ourselves2), robustness,3 out-of-distribution generalization,5 transfer learning7 and domain generalization.6 However, your point of view is interestingly complementary to these explorations. Machine learning has always focused on the objective of "generalization performance," viz, the capability of the model to perform on data that it has not seen before. This has led to deliberate efforts on making a model do well on subpopulations that it did not see while training. It may be an interesting follow-up discussion to see when one would need expected calibration on subpopulations, and when one would need model generalization on other subpopulations. Either way, your point is an important one—and the closest topic I can think of within my limited knowledge is in terms of calibration/conformal prediction.
More generally speaking, while explainability (and related terms) are intended to hedge predictions and support the reasoning behind decision making, "expectability" is possibly a property of the model predictive performance itself. One could view these as different dimensions of the Quality-of-Service (QoS) levels an ML/DL model ought to support. When one views ML/DL as a paradigm of data-driven automation akin to its predecessor—software development—it is perhaps timely and critical for real-world ML/DL systems to have a rigorous life cycle with requirement specifications, development, evaluation, verification and quality control phases—especially in risk-sensitive and safety-critical applications.
Vineeth N. Balasubramanian, Kandi, India
Factoring Impact
Thanks for an interesting discussion of the pros and cons of virtual meetings, as we have suffered them for the past nearly three years as described in the November 2022 Communications "The Impact of Virtual Meetings." The News item lacks a discussion of how difficult virtual meetings are to the lip-reading deaf. I am such a person.
Often, low Internet speed, a speaker's slow Wi-Fi connection, or inefficient platform software make the refresh rate of the video of the speaker too low for lip-reading. Some platforms make the speaker's head a thumbnail, too small for lip-reading, in order to give maximum space to the speaker's slides. AI-generated captioning is offered as the solution to not being able to read lips. However, for all but the clearest, newscaster-like speakers, AI-generated captions are useless for understanding what a speaker is saying. So, I am often left guessing what a speaker is saying.
One virtual conference I attended used a platform that was low refresh rate by design—whose motto was "Audio is king in the world of online videos"—and that showed all slide-showing speakers' heads as thumbnails. The conference's solution was to provide me with AI-generated captioning. However, among all the lectures I attended, I was able to understand only one, a keynote, delivered by an unusually clear native English speaker, for whom the AI-generated captions happened to be useful.
Needless to say, I prefer in-person meetings and conferences.
I wonder how accessible virtual meetings are to the blind, with bit-mapped images of slides that are unreadable by software that converts text to voice, and the fact that all voices now emerge from one loud speaker rather than from different places in a room.
Daniel Berry, Waterloo, ON, Canada
Author's response:
Thanks for the comment. You raise a great point. It seems like there is a lot of work to be done making virtual meetings accessible to all. The story looks closely at why companies may or may not want to make virtual work the standard. But managers should be also considering the quality and nature of virtual work, too, before mandating such policies.
Logan Kugler, Tampa, FL, USA
Join the Discussion (0)
Become a Member or Sign In to Post a Comment