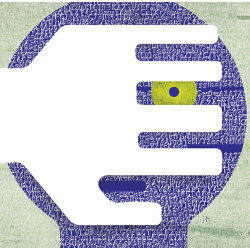
The digital economy relies on the collection of personal data on an ever-increasing scale. Information about our searches, browsing history, social relationships, medical history, and so forth is collected and shared with advertisers, researchers, and government agencies. This raises a number of interesting privacy issues. In today’s data protection practices, both in the U.S. and internationally, "personally identifiable information" (PII)—or, as the U.S. Health Insurance Portability and Accountability Act (HIPAA) refers to it, "individually identifiable" information—has become the lapis philosophorum of privacy. Just as medieval alchemists were convinced a (mythical) philosopher’s stone can transmute lead into gold, today’s privacy practitioners believe that records containing sensitive individual data can be "de-identified" by removing or modifying PII.
What is PII?
For a concept that is so pervasive in both legal and technological discourse on data privacy, PII is surprisingly difficult to define. One legal context is provided by breach-notification laws. California Senate Bill 1386 is a representative example: its definition of personal information includes Social Security numbers, driver’s license numbers, financial accounts, but not, for example, email addresses or telephone numbers. These laws were enacted in response to security breaches involving customer data that could enable identity theft. Therefore, they focus solely on the types of data that are commonly used for authenticating an individual, as opposed to those that violate privacy, that is, reveal some sensitive information about an individual. This crucial distinction is often overlooked by designers of privacy protection technologies.
Any information that distinguishes one person from another can be used for re-identifying data.
The second legal context in which the term "personally identifiable information" appears is privacy law. In the U.S., the Privacy Act of 1974 regulates the collection of personal information by government agencies. There is no overarching federal law regulating private entities, but some states have their own laws, such as California’s Online Privacy Protection Act of 2003. Generic privacy laws in other countries include Canada’s Personal Information Protection and Electronic Documents Act (PIPEDA) and Directive 95/46/EC of the European Parliament, commonly known at the Data Protection Directive.
Privacy laws define PII in a much broader way. They account for the possibility of deductive disclosure and—unlike breach-notification laws—do not lay down a list of informational attributes that constitute PII. For example, the Data Protection Directive defines personal data as: "any information relating to an […] natural person […] who can be identified, directly or indirectly, in particular by reference […] to one or more factors specific to his physical, physiological, mental, economic, cultural, or social identity."
The Directive goes on to say that "account should be taken of all the means likely reasonably to be used either by the controllera or by any other person to identify the said person." Similarly, the HIPAA Privacy Rule defines individually identifiable health information as information "1) That identifies the individual; or 2) With respect to which there is a reasonable basis to believe the information can be used to identify the individual." What is "reasonable"? This is left open to interpretation by case law. We are not aware of any court decisions that define identifiability in the context of HIPAA.b The "safe harbor" provision of the Privacy Rule enumerates 18 specific identifiers that must be removed prior to data release, but the list is not intended to be comprehensive.
PII and Privacy Protection Technologies
Many companies that collect personal information, including social networks, retailers, and service providers, assure customers that their information will be released only in a "non-personally identifiable" form. The underlying assumption is that "personally identifiable information" is a fixed set of attributes such as names and contact information. Once data records have been "de-identified," they magically become safe to release, with no way of linking them back to individuals.
The natural approach to privacy protection is to consider both the data and its proposed use(s) and to ask: What risk does an individual face if her data is used in a particular way? Unfortunately, existing privacy technologies such as k-anonymity6 focus instead on the data alone. Motivated by an attack in which hospital discharge records were re-identified by joiningc them via common demographic attributes with a public voter database,5 these methods aim to make joins with external datasets harder by anonymizing the identifying attributes. They fundamentally rely on the fallacious distinction between "identifying" and "non-identifying" attributes. This distinction might have made sense in the context of the original attack, but is increasingly meaningless as the amount and variety of publicly available information about individuals grows exponentially.
To apply k-anonymity or its variants such as l-diversity, the set of the so-called quasi-identifier attributes must be fixed in advance and assumed to be the same for all users. It typically includes ZIP code, birth date, gender, and/or other demographics. The rest of the attributes are assumed to be non-identifying. De-identification involves modifying the quasi-identifiers to satisfy various syntactic properties, such as "every combination of quasi-identifier values occurring in the dataset must occur at least k times."6 The trouble is that even though joining two datasets on common attributes can lead to re-identification, anonymizing a predefined subset of attributes is not sufficient to prevent it.
Re-identification without PII
Any information that distinguishes one person from another can be used for re-identifying anonymous data. Examples include the AOL fiasco, in which the content of search queries was used to re-identify a user; our own work, which demonstrated feasibility of large-scale re-identification using movie viewing histories (or, in general, any behavioral or transactional profile2) and local structure of social networks;3 and re-identification based on location information and stylometry (for example, the latter was used to infer the authorship of the 12 disputed Federalist Papers).
Re-identification algorithms are agnostic to the semantics of the data elements. It turns out there is a wide spectrum of human characteristics that enable re-identification: consumption preferences, commercial transactions, Web browsing, search histories, and so forth. Their two key properties are that (1) they are reasonably stable across time and contexts, and (2) the corresponding data attributes are sufficiently numerous and fine-grained that no two people are similar, except with a small probability.
The versatility and power of re-identification algorithms imply that terms such as "personally identifiable" and "quasi-identifier" simply have no technical meaning. While some attributes may be uniquely identifying on their own, any attribute can be identifying in combination with others. Consider, for example, the books a person has read or even the clothes in her wardrobe: while no single element is a (quasi)-identifier, any sufficiently large subset uniquely identifies the individual.
Re-identification algorithms based on behavioral attributes must tolerate a certain "fuzziness" or imprecision in attribute values. They are thus more computationally expensive and more difficult to implement than re-identification based on demographic quasi-identifiers. This is not a significant deterrence factor, however, because re-identification is a one-time effort and its cost can be amortized over thousands or even millions of individuals. Further, as Paul Ohm argues, re-identification is "accretive": the more information about a person is revealed as a consequence of re-identification, the easier it is to identify that person in the future.4
Lessons for Privacy Practitioners
The emergence of powerful re-identification algorithms demonstrates not just a flaw in a specific anonymization technique(s), but the fundamental inadequacy of the entire privacy protection paradigm based on "de-identifying" the data. De-identification provides only a weak form of privacy. It may prevent "peeping" by insiders and keep honest people honest. Unfortunately, advances in the art and science of re-identification, increasing economic incentives for potential attackers, and ready availability of personal information about millions of people (for example, in online social networks) are rapidly rendering it obsolete.
The PII fallacy has important implications for health-care and biomedical datasets. The "safe harbor" provision of the HIPAA Privacy Rule enumerates 18 attributes whose removal and/or modification is sufficient for the data to be considered properly de-identified, with the implication that such data can be released without liability. This appears to contradict our argument that PII is meaningless. The "safe harbor" provision, however, applies only if the releasing entity has "no actual knowledge that the information remaining could be used, alone or in combination, to identify a subject of the information." As actual experience has shown, any remaining attributes can be used for re-identification, as long as they differ from individual to individual. Therefore, PII has no meaning even in the context of the HIPAA Privacy Rule.
Beyond De-identification
Developing effective privacy protection technologies is a critical challenge for security and privacy research. While much work remains to be done, some broad trends are becoming clear, as long as we avoid the temptation to find a silver bullet. Differential privacy is a major step in the right direction.1 Instead of the unattainable goal of "de-identifying" the data, it formally defines what it means for a computation to be privacy-preserving. Crucially, it makes no assumptions about the external information available to the adversary. Differential privacy, however, does not offer a universal methodology for data release or collaborative, privacy-preserving computation. This limitation is inevitable: privacy protection has to be built and reasoned about on a case-by-case basis.
Another lesson is that an interactive, query-based approach is generally superior from the privacy perspective to the "release-and-forget" approach. This can be a hard pill to swallow, because the former requires designing a programming interface for queries, budgeting for server resources, performing regular audits, and so forth.
Finally, any system for privacy-preserving computation on sensitive data must be accompanied by strong access control mechanisms and non-technological protection methods such as informed consent and contracts specifying acceptable uses of data.
Join the Discussion (0)
Become a Member or Sign In to Post a Comment