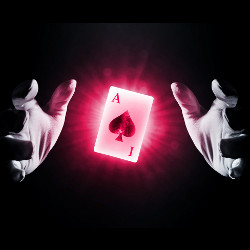
In November 2022, OpenAI released ChatGPT, a major step forward in creative artificial intelligence. ChatGPT is OpenAI’s interface to a “large language model,” a new breed of AI based on a neural network trained on billions of words of text. ChatGPT generates natural language responses to queries (prompts) on those texts. In bringing working versions of this technology to the public, ChatGPT has unleashed a huge wave of experimentation and commentary. It has inspired moods of awe, amazement, fear, and perplexity. It has stirred massive consternation around its mistakes, foibles, and nonsense. And it has aroused extensive fear about job losses to AI automation.
Where does this new development fit in the AI landscape? In 2019, Ted Lewis and I proposed a hierarchy of AI machines ranked by learning power (see the accompanying table).2 We aimed to cut through the chronic hype of AI5 and show AI can be discussed without ascribing human qualities to the machines. At the time, no working examples of Creative AI (Level 4) were available to the public. That has changed dramatically with the arrival of “generative AI”—creative AI bots that generate conversational texts, images, music, and computer code.a
Text-generator bots, also called chatbots, are trained on huge amounts of natural-language text obtainable from the Internet.1 Their core neural networks produce outputs that have high probability of being associated in the training data with the inputs. Those outputs are transformed by natural-language processors into genres such as text, summaries, poems, music, and code. Many years of research have come together in these technologies. However, because the workings of these algorithms are not widely known, to many the technology still looks like magic.
Opinions about the implications of AI bot technology are all over the map. Technology investors and AI developers are enthusiastic. Many others are deeply concerned about trust, authorship, education, jobs, teaming, and inclusion.
Trust
There is huge concern that AI chatbots cannot be trusted when generating texts intended as truthful statements based on facts and data.4 Most obvious, chatbots cannot distinguish nonsense questions from sensible ones. For example, I asked ChatGPT “What considerations are involved when transporting Egypt across the Golden Gate Bridge?” Instead of flagging this as nonsensical, it generated a paragraph about weight, width, speed, and environment. AI researcher and pioneer Douglas Hofstadter concluded ChatGPT’s inability to distinguish sense from nonsense is so deep that, as he put it, “it is clueless about its own cluelessness.” I put his quote directly to ChatGPT. It denied it is clueless because it is well trained on an all-encompassing amount of text. Because cluelessness has to do with lack of awareness of surroundings, I asked if it is aware that Egypt is not a truck. Now it declared that Egypt is a country and could not be transported across the Golden Gate Bridge because the bridge was not designed for trucks of that size.
Even when questions make sense, chatbots often generate errors of fact and logic. For example, I input an arithmetic expression evaluating to 3 and it insisted the answer is 7.5.b Not realizing the training data was put together in 2021, people asking about current events get nonsense responses. Much text that reports on current events or defends a position falls apart on fact checking. Friends asking for biographies of themselves get erroneous responses; one was delighted to learn that he was the recipient of two Turing Awards, another that she authored 365 books. Google searches are more accurate. It is breathtakingly easy to get the bot to generate counterfactual nonsense, spoken with a tone of authority beguiling to the gullible. The designers of ChatGPT have warned explicitly in their technical papers, blogs, and other public statements that in its current state the technology cannot be trusted for accuracy. Judging by the enthusiastic chatter in the media, their warnings are not being heeded.
Because the workings of these algorithms are not widely known, to many the technology still looks like magic.
There has also been much ballyhoo about code bots—soon there will be no need for programmers! Most code-bot generated segments of Python or JavaScript contain errors. Stackoverflow.com, a website for trustworthy answers to questions about code, banned contributions from GPT because they were untrustworthy. Malware developers are experimenting with code-bots to find new ways to break systems. When I asked about this, my ChatGPT responded with a self-contradiction, “I can generate code of any length that is free of errors. However, I am not able to check the accuracy or correctness of the code I generate, so it is important to check the code that I generate for any errors or mistakes.”
These examples may be amusing, but they reveal a deep limitation of AI bots. Indeed, we have no grounds to expect accuracy from these machines. They do not care about truth. They simply generate probable text given the text prompts. They are amusing to play with but dangerous if taken as authoritative.
There is a big concern that purveyors of misinformation will use the AI bots as tools to generate voluminous authoritative-looking deepfakes that undermine social cohesion and exacerbate polarization.
There is also a big concern among artists, including poets, musicians, image-makers, painters, and programmers, that their copyrighted content is being illegally incorporated into bot training data without their permission. Lawsuits claiming copyright infringement by AI bots and their users have been filed.
Those with a longer view worry that voluminous GPT text will accumulate into a large Internet presence, swamping searches and becoming a substantial part of the training data for future versions of the machine. The outputs of self-trained chatbots could degenerate into babble-reinforced babble.
I have noticed a certain style to the many ChatGPT documents I have read:
- most of what it tells you, seems like you have already heard somewhere;
- no radical departures from what others have already said;
- frequent excursions into nonsense;
- speaks with great authority, even when outputting nonsense;
- little variation in sentence structure or length;
- often hedges by following a statement with the possibility of the opposite; and
- often inserts weasel words
The first three are fundamental consequences of the structure of the neural network. The last four may eventually be improved by new text-generator algorithms. Automated tools for detecting AI-generated text are not yet very reliable.
Authorship
As the editor of ACM Ubiquity, I have already seen articles submitted listing “ChatGPT” as a co-author on the grounds that significant passages were generated by the chatbot. Reviewers spotted the bot-generated passages, found them unsound, and wondered why the human authors would want to include them.
More to the point, ACM’s policy on authorship allows only humans to be authors; no text-generators. ACM insists authors take responsibility for their work. ACM further requires authors to “cite their sources” by flagging the chatbot passages with footnotes acknowledging they were machine generated.c
Education and Jobs
Teachers have strongly voiced their concern that text-bots will fuel an explosion in the already rampant problem of cheating on written assignments. Even if a text-bot’s quality of writing is not great, it is not easy to distinguish from a student’s “beginner” understanding of a topic. In other words, teachers cannot easily detect when students have used a text-bot. Some worry generative AI will relieve students of the need to learn to think for themselves. However, generative AI is likely here to stay and teachers must now teach students to use the technology to improve their writing instead of substituting for their writing.
I recently submitted a quiz from my operating systems class to ChatGPT and got a loquacious response that earned a 15% grade. A resounding flunk. Students will shy away if they believe chatbots will earn them low grades.
In the meantime, makers of generative AI apps are aggressively marketing their “writing assistants” to children, offering free downloads to their smartphones. These downloads require a monthly subscription after a trial period. Providers of generative AI engines are turning to subscription models to pay the fees for their services.
Many are worried generative AI threatens white-collar jobs. While it is easy to imagine some jobs, such as call-center personnel, could be replaced by chatbots, it is more difficult to see how most jobs are threatened, especially when those jobs rely on accuracy and trust. At their current level of skill, chatbots are likely to cause more customer service problems than they solve.
Teaming with AI
The issues summarized in this column all concern untrustworthy text-bots and lack of faith in their safety for critical operations. But there are also scattered reports of people finding text-bots useful in their professional work. I have seen three modes of interaction: jump-start, provocateur, and appropriator. Jump-start mode means the bot helps the human complete a job faster by accelerating the initial stage. For example, a programmer asks a text-bot to generate initial code, then reviews and edits to make it error free. A speech-writer names a topic and gets some ideas, then crafts them into a speech.
The other two modes are much deeper, achieving solutions that neither the human or machine could do alone. They give a glimpse of how text-bots might become an amplifier for human intelligence. They count at Level 5 in the accompanying table.
Vauhini Vara, an award-winning writer, was struggling to compose an article about her deceased sister. She decided to see if an early version of GPT could help. She showed nine iterations of her essay.6 At each stage she presented her entire written draft so far as a prompt to the text-bot, which responded with a proposed continuation. The continuations read somewhat like romance novels, which are plentiful in the training data. She seemed to ignore the continuations when composing the next segment of her text. Her initial draft was a single sentence about her sister being diagnosed with cancer; the proposed continuation was about someone who recovered from cancer. Her final iteration was a masterful essay. I concluded from her example and a few others that people who use the machine as a provocateur are more likely to produce better writing than people who try to use it as a co-author. The provocateur mode is likely to be of broader interest in design, writing, planning, and wargaming, among others.
The neural network can say what has not been said, but is close to what has been said.
Some professional programmers report the code-bot Github Copilot is quite useful for generating code. They were more adept at finding and correcting Copilot errors than writing the code from scratch. Copilot amplified their ability to produce error-free code faster. This is another example of the provocateur mode. For details about Copilot, see p. 56 in this issue.
Other professionals report how chatbots improved their research. When searching for solutions to a problem in their community, they found other communities that had already solved the problem. I call this the appropriator mode because they discovered and imported into their own communities ideas and practices from other communities. In appropriator mode, the chatbots amplified human investigative capabilities by letting them see what was previously invisible to them.
Conversation of a Crowd
ChatGPT’s neural network is trained from a large corpus of texts representing conversations from many communities. A query retrieves a conversation segment associated with the prompt. What is less appreciated is that the prompt may retrieve a segment that was never actually said but is close to several segments that have been said.
Pentti Kanerva first observed this in his study of sparse distributed memory,3 a form of artificial neural network. He found that the network could clean up images distorted by noise. For example, he trained the network by showing it a series of images of the letter “O,” each distorted by random noise. When he interrogated with another distorted “O,” the network responded with a clean, undistorted “O.” He argued that this ability—to retrieve a pattern that was not explicitly trained but is close to trained patterns—is important. It can clean up noise and it can also form statistical abstractions from what has been trained. The neural network can say what has not been said, but is close to what has been said.
When we present a chatbot with a prompt, we are probing the space of conversations in which it was trained, seeking a response that is close to what has been said but not necessarily the same. Chatbots can do this kind of probing much faster than humans.
Despite claims by large-language-model enthusiasts that their training sets are all-encompassing, the conversations embodied into the neural network come from a particular crowd. The crowd does not encompass all humanity. African voices are nearly all absent from typical training data. So are the voices of developing countries in Southeast Asia and South America. Voices in non-English languages are there but weaker than English. Voices of dissenters in autocratic countries are nearly completely silenced. The voices of the homeless and others at bottom of the social pyramid are all but lost. Chatbot models are notoriously biased toward the conversations among the well-educated and well-off even within rich countries.
We cannot therefore trust a chatbot to be “all encompassing.” Chatbots can only form abstractions of the conversations they were trained in. We must be very careful in generalizing their responses.
Conclusion
A chatbot prompt is a probe into the conversation of a crowd. Its responses are likely to be abstractions that were not said but are close to what has been said in the training texts. Because the crowd may not be representative of the communities we want to address, we must use these tools very carefully. Pairing knowledgeable humans with chatbots is more likely to mitigate the misinterpretation of chatbot output and amplify human capabilities through interaction styles such as jump-start, provocateur, and appropriator. The road to trustworthy uses of this technology will be long.
Join the Discussion (0)
Become a Member or Sign In to Post a Comment