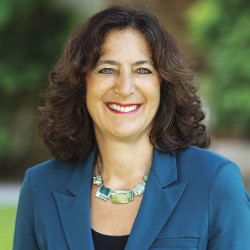
Communication is more important than ever, with everything from college to CrossFit going virtual during the COVID-19 pandemic. Nobody understands this better than 2020 Marconi Prize recipient Andrea Goldsmith, who has spent her career making the wireless connections on which we rely more capable and stable. A pioneer of both theoretical and practical advances in adaptive wireless communications, Goldsmith spoke about her work on multiple-input and multiple-output (MIMO) channel performance limits, her new role as the incoming dean at Princeton University's School of Engineering and Applied Science, and what's next for networking.
As an undergrad, you studied engineering at the University of California, Berkeley. What drew you to wireless communications?
After I got my undergraduate degree, I went to work for a small defense communications startup. It was a great opportunity, because I was working on really hard problems with people who had advanced degrees. We were looking at satellite communication systems and antenna array technology. I was really motivated to go back to graduate school because I wanted to learn more.
This was around the time that commercial wireless was starting to take off; cellular systems in particular.
By the time I went to graduate school, in 1989, they were starting to talk about second-generation cellular standards. There was a big debate about what the technology should be. I found that whole area fascinating, and it's what I ended up focusing on initially.
Later, after joining Stanford's Electrical Engineering department, you made groundbreaking advances in multiple-input and multiple-output (MIMO) channel performance limits.
We had looked at direction-finding techniques at the defense communications startup in the '80s, which exposed me to the MUSIC and ESPIRIT algorithms for direction-finding with multiple antennas. During graduate school, I spent two summers working at AT&T Bell laboratories with Gerry Foschini, whose work informed a lot of the early MIMO techniques, following up on the groundbreaking work of A. Paulraj at Stanford.
A few years after I came to Stanford, MIMO technology emerged as a really compelling one for capacity gain. So my group started looking at how to handle the dynamic adaptation of multiple antenna systems. We'd been working on dynamic adaptation of single antenna systems, and that was a natural area to expand into.
More recently, you've begun to explore deployments in the millimeter wave band, and in particular, millimeter-wave massive MIMO technologies. Can you talk about your work in that area?
Millimeter-wave is a really interesting spectral band to explore for commercial wireless. The biggest attraction is the amount of spectrum that's available—tens of gigahertz of spectrum. We have to find ways to utilize that, especially given how much of the lower bands are already occupied.
But millimeter-wave communication is challenging even at relatively short ranges, because it's very inefficient.
If you have a single, omni-directional antenna, the power falls off relative to one over the frequency squared. So when you go up to these very high frequencies, you have tremendous fall-off, because the omni-directional antenna is sending out energy in all directions. When you steer that energy in a particular direction, you can get a lot of the energy back, and there are different techniques. You can use antenna designs like horn antennas, for example, to get this directivity. But the beauty of MIMO is that you use software and electronic steering techniques to dynamically point the energy exactly in the direction that you want it to go, depending on where the receiver moves. That's in theory. In practice, it's hard to do because any interference scatters the energy in all directions. Millimeter-wave is much more sensitive to interference because it requires this directional steering in order to get reasonable performance.
What are some of the techniques you've explored?
There are many open questions. We've done some work looking at the fundamental capacity limits of massive MIMO arrays that adapt to time-varying channels. We started with perfect conditions, where you can estimate the channel perfectly and feed it back instantaneously. Of course, that's a very idealized setting. In a typical massive MIMO setting, you need to measure the antenna gain from tens or even hundreds of antenna elements at the transmitter to every one of the antenna elements at the receiver. That's much more challenging. So we've also looked at techniques for situations where you can't do that kind of dynamic adaptation. What if you estimated the channel imperfectly—how would you deal with interference? What if you stopped trying to do any kind of channel estimate and did blind MIMO decoding? We've also looked into adapting the antenna arrays to meet the requirements of different applications, because some applications don't require such high-performance gains.
So you're trying to match what you're doing at the physical layer with the requirements at the application layer.
The next generation of wireless networks needs to support a much broader range of applications. The goal of each generation of cellular has always been getting to higher data rates, but what we're looking at now are low-latency applications like autonomous driving, and networks so far have not really put hard latency constraints into their design criteria. If you exceed the latency constraints on your video or audio applications, it just means that quality is poor, or maybe the connection is dropped. That's not acceptable for a real-time autonomous vehicle application. Networks also need to be able to support soft constraints on energy consumption for low-power Internet of Things devices, which might run off a battery that can't be recharged.
"There's a price to be paid for machine learning, in terms of computational complexity and latency."
Let's talk about machine learning, which you found can trump theory in equalizing unknown or complex channels.
I was very skeptical of jumping onto the bandwagon of machine learning, but when you don't have good models, machine learning is an interesting tool for figuring out the end-to-end optimization of a system. We first applied machine learning when we were working on molecular communication: using molecules instead of electromagnetic waves to send ones and zeros. We used an acid for one and a base for zero and sent it out through a liquid channel. The way the signal propagates is by diffusion, and there's no good channel model for that. You also need to equalize it, because the chemicals sit around in the channel for a long time. If you send a lot of ones, then the channel has too much acid in it, and when you send a base, it will get destroyed by the acid.
In that situation, you found that machine learning worked better than any existing techniques.
That's right. Later, we started looking at machine learning more broadly for channel equalization on traditional wireless channels. The optimal technique is the Viterbi algorithm, and we found that it can't be beat under ideal conditions, where you know the channel perfectly and you have no complexity constraints. But when you relax those perfect assumptions, it turns out that machine learning can do better.
Of course, it's not always the case that you should go to machine learning as soon as you move away from perfect conditions. There's a price to be paid for machine learning, in terms of computational complexity and latency. But to me, the meta-lesson is that having domain knowledge plus some knowledge of machine learning is much more valuable to solving domain-specific problems than having very deep knowledge of machine learning, but not really understanding the specific problem you're trying to solve. We understood the problem of equalization well, so we were able to take this tool and use it very efficiently to reach a solution.
You were recently appointed dean of Princeton University's School of Engineering and Applied Science. What are some of your goals?
Princeton already has a strong group of people who are working on wireless communication and networking. For my own research, I'm excited to work with these Princeton colleagues, as well as researchers in nearby wireless groups at NYU and Rutgers. There's been a resurgence of interest in wireless lately, and in bridging the digital divide in the pandemic, so it's a very exciting time to be working in the field.
I join Princeton at a time when it is growing the size of its engineering faculty by almost 50%, building an entirely new neighborhood with new buildings for all its engineering departments and interdisciplinary institutes, and also building a separate part of campus dedicated to innovation, entrepreneurship, and forging stronger ties with industry. I'm really excited to be the incoming dean at such a transformational time for Princeton Engineering.
Join the Discussion (0)
Become a Member or Sign In to Post a Comment