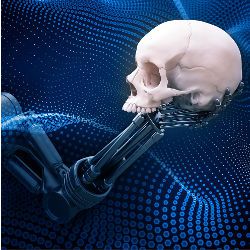
In January 2015, a host of prominent figures in high tech and science and experts in artificial intelligence (AI) published a piece called "Research Priorities for Robust and Beneficial Artificial Intelligence: An Open Letter," calling for research on the societal impacts of AI. Unfortunately, the media grossly distorted and hyped the original formulation into doomsday scenarios. Nonetheless, some thinkers do warn of serious dangers posed by AI, tacitly invoking the notion of a Technological Singularity (first suggested by Good8) to ground their fears. According to this idea, computational machines will improve in competence at an exponential rate. They will reach the point where they correct their own defects and program themselves to produce artificial superintelligent agents that far surpass human capabilities in virtually every cognitive domain. Such superintelligent machines could pose existential threats to humanity.
Recent techno-futurologists, such as Ray Kurzweil, posit the inevitability of superintelligent agents as the necessary result of the inexorable rate of progress in computational technology. They cite Moore’s Law for the exponential growth in the power of computer chips as the analogical basis for this claim. As the rise in the processing and storage capacity of hardware and other technologies continues, so, they maintain, will the power of AI expand, soon reaching the singularity.
These arguments for the concept of the singularity seem to us to be, at best, suspect. Moore’s Law concerns the growth of hardware processing speed. In any case, it will eventually run up against the constraints of space, time, and the laws of physics. Moreover, these arguments rely on a misplaced analogy between the exponential increase in hardware power and other technologies of recent decades and the projected rate of development in AI. Great progress is indeed being made in deep neural network learning (DL) that has produced dramatic improvements in the performance of some AI systems in speech recognition, visual object recognition, object detection, and many other domains.4 Dramatic increase in processing power (of GPUs for example) and in the availability of large amounts of data have been the driving force behind these advances. Nevertheless, the jump from such learning to superintelligence seems to us to be more than fanciful. In the first place, almost all these advances have been in the supervised setting where there are large amounts of training data. As the leaders of DL themselves point out,4 this situation is the exception rather than the rule—most data is unlabeled and calls for unsupervised learning. Furthermore, these recent advances have all been in narrow specialized tasks such as image recognition, not in more general learning tasks, which require complex reasoning. Nor do these algorithms work in a recursive self-improvement loop as conceived of by Good’s argument. Progress in deep learning and other areas of AI has not been exponential in any meaningful sense. It comes in irregular, and often unanticipated spurts, as is generally the case with breakthroughs in science and engineering. Unsupervised and general AI still remains a major open challenge. As pointed out in Bengio et al., "ultimately, major progress in artificial intelligence will come about through systems that combine representation learning with complex reasoning."4
Recent books by a mathematician10 and a philosopher5 have taken up variants of this view and issued their own warnings about the possible existential dangers that strong AI presents. Their main argument is more subtle. It is illustrated with a thought experiment involving the design of a machine with the narrowly defined goal of producing paper clips as efficiently as possible. Let us imagine this machine continually improves its ability to solve this narrow goal. Eventually, assuming the progress in AI continues indefinitely, the machine could set up subsidiary instrumental goals that serve its primary goal (maximizing paper clips). One of these instrumental sub-goals could conceivably be to utilize all other resources, including humans, to produce paper clips. The point of the thought experiment is to illustrate that even with a narrowly defined goal that is apparently benign, a superintelligent machine could adopt unforeseen instrumental sub-goals that are very dangerous, even to the point of posing an existential risk to humanity. Even though this is a thought experiment, both books betray a striking lack of engagement with the present state of technology in AI.
Shanahan11 offers a review of the present state of AI technologies and its future possibilites in the context of the singularity. He considers various technological approches toward superintelligence. On the one hand, there is the biology-based approach of trying to understand the human brain well enough to enable whole brain emulation. Kurzweil has also promoted this perspective, sketching fantasies of nano-devices traveling through the brain to map the whole connectome. Even if it were possible to fully decipher the brain’s "wiring," this does not entail that we will be able to reproduce human cognition and thought through the construction of computational models of this neural system, as Kurzweil seems to suggest, see the critique by Allen and Greaves.1 The nematode worm has a connectome small enough to be essentially fully mapped out in 2006, but this has produced little substantive understanding of its simple brain. Recently the "Human Brain Project," a billion-euro flagship project funded by the European Commission, ran aground because of an astonishing revolt by large number of Europe’s leading neuroscientists who called into question the validity of the project’s basic assumptions.
Work in technology driven by AI generally seeks to solve particular tasks, rather than to model general human intelligence. It is often more efficient to perform these tasks by models that do not operate in the way that the human brain does just as we construct jets rather than machines that fly like birds. Shanahan also considers engineering AI approaches and casting them into a reinforcement learning framework. A robot is equipped with a reward function that it is programmed to optimize through interaction with the environment via a set of sensors. The agent takes actions and receives a payoff from the environment in response. It explores action strategies to maximize its payoff, and its final goal. This is perhaps the most suitable approach among today’s AI technologies within which to situate Bostrom’s thought experiment concerning the paper clip device whose reward function is the number of paper clips that it creates. Google’s DeepMind made headlines recently by demonstrating how a combination of deep learning and reinforcement learning could be used to build a system that learns to play Atari video games, and, even more recently, that beat the world champion at the game of Go. However, for more complex tasks, Shanahan and the Google DeepMind scientists agree that the science and technology currently associated with such an approach is in a thoroughly primitive state.
In fact much, if not all of the argument for existential risks from superintelligence seems to rest on mere logical possibility. In principle it is possible that superintelligent artificial agents could evolve, and there is no logical inconsistency in assuming they will. However, many other threats are also logically possible, but two considerations are always paramount in determining our response: a good analysis and estimate of the risk and a good understanding of the underlying natural or technological phenomena needed to formulate a response. What is the likelihood of super-intelligent agents of the kind Bostrom and Haggstrom worry about? While it is difficult to compute a meaningful estimate of the probability of the singularity, the arguments here suggest to us that it is exceedingly small, at least within the foreseeable future, and this is the view of most researchers at the forefront of AI research. AI technology in its current state is also far from a mature state where credible risk assessment is possible and meaningful responses can be formulated. This can be contrasted with other areas of science and technology that pose an existential threat, for example, climate change and CRISPR gene editing. In these cases, we have a good enough understanding of the science and technology to form credible (even quantitative) threat assessment and formulate appropriate responses. Recent position papers such as Amodel et al.2 ground concerns in real machine-learning research, and have initiated discussions of practical ways for engineering AI systems that operate safely and reliably.
By contrast to superintelligent agents, we are currently facing a very real and substantive threat from AI of an entirely different kind. Brynjolfsson and McAfee,6 and Ford7 show that current AI technology is automating a significant number of jobs. This trend has been increasing sharply in recent years, and it now threatens highly educated professionals from accountants to medical and legal consultants. Various reports have estimated that up to 50% of jobs in western economies like the U.S. and Sweden could be eliminated through automation over the next few decades. As Brynjolfsson and McAfee note toward the end of their book, the rise of AI-driven automation will greatly exacerbate the already acute disparity in wealth between those who design, build, market, and own these systems on one hand, and the remainder of the population on the other. Reports presented at the recent WEF summit in Davos make similar predictions. Governments and public planners have not developed plausible programs for dealing with the massive social upheaval that such economic dislocation is likely to cause.
Much, if not all of the argument for existential risks from superintelligence seems to rest on mere logical possibility.
A frequently mentioned objection to this concern is that while new technologies can destroy some jobs, they also create new jobs that absorb the displaced workforce. This is how it has always been in the past. So for example, unemployed agricultural workers eventually found jobs in factories. So why should this time be different? Brynjolfsson and McAfee argue that information technologies like AI are different from previous technologies in being general-purpose technologies that have a pervasive impact across many different parts of the economy. Brynjolfsson and McAfee and Ford argue that no form of employment is immune to automation by intelligent AI systems. MIT economist David Autor points to deep and long-term structural changes in the economy as a direct result of these technologies.3
One way in which AI-powered systems can improve production and services while avoiding massive unemployment is through a partnership of people and machines, a theme running through John Markoff’s book.10 He points out that the combination of humans and machines is more powerful than either one of them alone. The strongest chess player today, for example, is neither a human, nor a computer, but a human team using computers. This is also IBM’s cognitive computing vision, based on the Watson technology that defeated the human champions of "Jeopardy!" Today IBM is seeking to deploy Watson cognitive computing services in various sectors. For example, a human doctor aided by a Watson cognitive assistant would be more effective in diagnosing and treating diseases than either Watson or the doctor working separately.
While human-machine cooperation is a hopeful avenue to explore in the short to medium term, it is not clear how successful this will be, and by itself it is not an adequate solution to the social issues that AI automation poses. These constitute a major crisis of public policy. To address this crisis effectively requires that scientifically literate government planners work together with computer scientists and technologists in industry to alleviate the devastating effects of rapid technological change on the economy. The cohesion of the social order depends upon an intelligent discussion of the nature of this change, and the implementation of rational policies to maximize its general social benefit.
Join the Discussion (0)
Become a Member or Sign In to Post a Comment