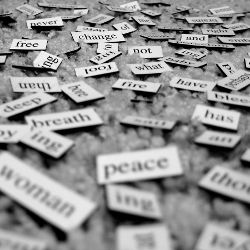
From the very early days of the World Wide Web, researchers identified a need to be able to understand the semantics of the information on the Web in order to enable intelligent systems to do a better job of processing the booming Web of documents. Early proposals included labeling different kinds of links to differentiate, for example, pages describing people from those describing projects, events, and so on. By the late 1990s, this effort had led to a broad area of computer science research that became known as the Semantic Web.1 In the past decade and a half, the early promise of enabling software agents on the Web to talk to one another in a meaningful way inspired advances in a multitude of areas: defining languages and standardsa to describe and query the semantics of resources on the Web; developing tractable and efficient ways to reason with these representations and to query them efficiently; understanding patterns in describing knowledge; and defining ontologies that describe Web data to allow greater interoperability.
Semantic Web Today
In fact, Semantic Web research and practice spanned the spectrum from focusing on expressivity and reasoning on the Web4 to providing an ecosystem of linked data that allows data resources to link to one another explicitly through shared naming and equivalence statements across repositories.2 Arguably, the far ends of this spectrum were ignoring the messiness of the real Web in the former case, and were not providing enough perceivable value because of lack of any organization or semantics in the latter. However, in between, there was a broad "sweet spot" where the work coming out of these communities has led to contributions that have gone beyond research and led to undeniable advances in the way that the Web works today:
- Over 2.5 billion Web pages have markup conforming to the schema.org format, which enables them to describe precisely the structured content on their sites using a shared vocabulary.b
- Linked data, in the form of structured, typed, and dereferencable links, powers media sites for organizations such as the BBC and New York Times; major libraries and museums around the world actively develop their content as linked data.
- Google, Yahoo!, Microsoft, Facebook, many other large Web companies as well as numerous research projects are developing large knowledge graphs, which define, structure, and link hundreds of millions of entities, to enhance search, to provide better advertising match, to improve the answers of their artificial personal assistants, and so on.
- Commercial database-management systems (for example, Oracle) provide native support for Semantic Web languages.
- Recommender companies are increasingly using semantics and semantic tagging to improve both the quality and accuracy of recommendations that they provide.c
- The World Health Organization is developing the main international terminology for diseases to be used by all United Nations member countries as an ontology to be usable on the Web.6
The list goes on.
Semantic Web Research in Transition
As the early research has transitioned into these larger, more applied systems, today’s Semantic Web research is changing: It builds on the earlier foundations but it has generated a more diverse set of pursuits. As the knowledge graphs mentioned previously increasingly use semantic representations, they have driven the functionality of a new generation of apps (mobile healthcare, mapping and shopping assistants, and others). As these applications became increasingly crucial to advertising and e-commerce, the representations they used became less formal and precise than many early Semantic Web researchers had envisioned.
As developers strive to provide structure and organization beyond just linking of data, they are not making very much use of the formal semantics that were standardized in the Semantic Web languages. Modern semantic approaches leverage vastly distributed, heterogeneous data collection with needs-based, lightweight data integration. These approaches take advantage of the coexistence of a myriad of different, sometimes contradictory, ontologies of varying levels of detail without assuming all-encompassing or formally correct ontologies. In addition, we are beginning to see the increased use of textual data that is available on the Web, in hundreds of languages, to train artificially intelligent agents that will understand what users are trying to say in a given context and what information is most pertinent to users’ goals at a given time. These projects are increasingly leveraging the semantic markup that is available on the Web; for example, the IBM Watson "Jeopardy!"-playing program made use of taxonomies and ontologies (such as DBpediad and YAGOe) to increase performance significantly.3
As the early research has transitioned into larger, more applied systems, today’s Semantic Web research is changing.
In addition to the increasing amount of semantically annotated information on the Web, a lot more structured data is becoming available. This data includes information from scientists and governments publishing data on the Web and the ever increasing amount of information available about each of us, individually and as societies—in the form of our social interactions, location and health data, activities, and interests. Harnessing this data, and understanding its diverse and often contradicting nature, to provide really meaningful services and to improve the quality of our lives, is something that researchers in both industry and academia are beginning to tackle. Statistical and machine-learning methods become more powerful and computational resources continue to improve. Thus, some of the semantic knowledge that researchers had to construct manually they can now learn automatically, tremendously increasing the scale of the use of semantics in understanding and processing Web data. While manually constructed formal ontologies may often (but not always) be required to form a backbone of semantics for the Web, much of the content that puts "meat" on those bones is "scruffy" and imprecise, often statistically induced. Indeed, the ontologies themselves might be learned or enhanced automatically. As the semantics, in a sense, becomes more "shallow," it could be more widely applicable.5 Consequently, our very understanding of the nature of the semantics that intelligent systems produce and leverage is changing, and with it, our vision for the future of the Semantic Web.
The Next 10 Years
As we look at the next decade of the Semantic Web, we believe these trends will continue to fuel new demands on Web researchers. Thus, these trends lead us to formulate a new set of research challenges. We believe the objective of the next decade of Semantic Web research is to make this vast heterogeneous multilingual data provide the fuel for truly intelligent applications.
Bringing a new kind of semantics to the Web is becoming an important aspect of making Web data smarter and getting it to work for us.
Achieving this objective will require research that provides more meaningful services and that relies less on logic-based approaches and more on evidence-based ones. We note the rubrics listed here are not all that different from the challenges we faced in the past, but the methods, the scale, and the form of the level of representation languages changes drastically. We present questions under each of the rubrics to guide this research.
- Representation and lightweight semantics: Semantic Web standards that were developed by the World Wide Web Consortium fueled early research on the Semantic Web, enabling scientists not to worry about the underlying representation languages and to publish resources that provide linking between many open databases expressed in standard formats.f However, the world of semantics on the Web also increasingly encompasses representations in non-standard (and sometimes proprietary) formats. This diversity also applies to how formal the representations are. New questions that emerge include: How do we leverage these diverse representations? What is a broader view of what constitutes semantics on the Web? How do we coordinate the diverse components of structured knowledge that are defined by various parties and that must interact in order to achieve increasingly intelligent behavior? How do we define lightweight, needs-based, "pay-as-you-go" approaches for describing knowledge? What are the languages and architectures that will provide this knowledge to the increasingly mobile and application-based Web?
- Heterogeneity, quality, and provenance: It is a truism that data on the Web is extremely heterogeneous. Web resources drastically vary in size, underlying semantics, and of course, quality. A dataset precise enough for one purpose may not be sufficiently precise for another. Data on the Web may be wrong, or wrong in some context—with or without intent. Provenance has already been recognized as critical to applications using data on the Web. This heterogeneity raises a variety of questions to explore: How do we integrate heterogeneous data and particularly how can we understand which data can be integrated to what degree? How can we represent and assess quality and provenance of the data? How do we evaluate whether the quality of a particular source is sufficient for a given task?
- Latent semantics: Obviously, there is a lot of semantics that is already on the Web, albeit mostly in text, or in data that machines cannot readily interpret. To complement formally developed ontologies, we must be able to extract latent, evidence-based models that capture the way that users structure their knowledge implicitly. We need to explore these questions: How much of the semantics can we learn automatically and what is the quality of the resulting knowledge? As ontologies are learned or enhanced automatically, what is the very meaning of "formal ontologies"? How do we develop some notion of approximate correctness? Do similar or different reasoning mechanisms apply to the ontologies that are extracted in this way? How do crowdsourcing approaches allow us to capture semantics that may be less precise but more reflective of the collective wisdom?
- High volume and velocity data: While the challenges of the growing "Internet of things" are just starting to emerge, already we see scientists and developers trying to come to grips with the problems caused by the high volume and velocity of the sensory data that is streaming to the Web. New research must explore these questions: How do we triage the data in motion to determine what to keep and what we may choose, or need, to allow to be lost? How do we deploy simple decision-making agents in such applications, and what are the semantic needs of such agents? How can our applications integrate constantly changing sensor data with fixed data of long duration and high-quality semantic provenance?
In short, bringing a new kind of semantics to the Web is becoming an increasingly important aspect of making Web data smarter and getting it to work for us. We believe our fellow computer scientists can both benefit from the additional semantics and structure of the data available on the Web and contribute to building and using these structures, creating a virtuous circle. The techniques of the early Semantic Web research have defined many of the parameters that we need in order to understand these new approaches and have provided important data resources to the community exploring how to build new Web-based applications. Continued research into Web semantics holds incredible promise, but only if we embrace the challenges of the modern and evolving Web.
Join the Discussion (0)
Become a Member or Sign In to Post a Comment