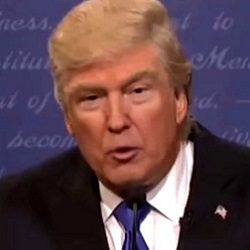
It has been said that the camera doesn’t lie. However, in the digital age, it is also becoming abundantly clear that it doesn’t necessarily depict the truth. Increasingly sophisticated machine learning combined with inexpensive and easy-to-use video editing software are allowing more and more people to generate so-called deepfake videos. These clips, which feature fabricated footage of people and things, are a growing concern in both politics and personal life.
“It’s a technology that is easily weaponized,” observes Hany Farid, a professor at the University of California, Berkeley.
“Today’s deep neural nets and AI algorithms are becoming better and better at creating images and video of people that are convincing and not real.”
Not only can deepfakes be used to depict a political candidate or celebrity saying or doing something he or she never said or did, they can depict false news events in an attempt to sway public opinion. And then there are the disturbing issues of blackmail and porn, including revenge porn. A number of deepfake videos have surfaced showing a person’s ex-partner nude or engaged in sex acts he or she did not commit. The person creating the deepfake video simply transposes the victim’s face onto the body of another person, such as a porn star.
Figure. Paul Scharre of the Center for a New American Security views a deepfake video made by BuzzFeed, which changed what was said by former U.S. president Barack Obama to what is spoken by filmmaker Jordan Peele (right on screen).
“Today’s deep neural nets and AI algorithms are becoming better and better at creating images and video of people that are convincing but not real,” says Siwei Lyu, professor of computer science and director of the Computer Vision and Machine Learning Lab (CVML) of the University at Albany, which is part of the State University of New York. As a result, researchers in digital media forensics, computer scientists, and others are now examining ways to better identify fake videos, authenticate content, and build frameworks to help thwart the rapid spread of deepfakes on social media.
“It’s a problem that isn’t going to go away,” Lyu says.
Deep Trouble
Deepfake technology bubbled to general public awareness in early 2018, when former U.S. president Barack Obama spoke out about the growing dangers of false news and videos. “We are entering an era when our enemies can make it look like anyone is saying anything at any point in time,” he stated in a video clip. Except it was not Obama actually making the video appearance; it was a deepfake created by comedian Jordan Peele in conjunction with online publication Buzzfeed. The goal was to help educate the public about the potential dangers of deepfakes.
Other examples abound. Parkland shooting survivor Emma González was depicted tearing up a copy of the U.S. Constitution, instead of a shooting range target. Celebrities such as Gal Gadot, Emma Watson, Hilary Duff, and Jennifer Lawrence have been inserted into porn scenes. A political party in Belgium depicted Donald Trump taunting Belgium for remaining in the Paris Climate Agreement. In India, a journalist who reported corruption in Hindu national politics found her image inserted into a fake porn video along with her home address and telephone number; she received numerous death and rape threats.
Deepfakes also can incorporate audio. For instance, crooks recently impersonated the voice of a U.K.-based energy company’s CEO in order to convince workers to wire $243,000 cash to their account.
Although photo, video, and audio manipulation techniques have been around for years (evolving from dodging and burning photos in the darkroom to using photo editing software to alter digital images), computer-altered video raises the stakes to a new level. “Advances in artificial intelligence technology have allowed for the creation of fake audiovisual materials that are almost indistinguishable from authentic content, especially to ordinary human senses,” said Jeffrey Westling, a Technology and Innovation Policy Fellow with the non-profit, non-partisan R Street Institute, at a U.S. House of Representatives hearing in June 2019.
Westling is not the only person sounding alarms. Says Matt Turek, program manager in the Information Innovation Office of the U.S. Defense Advanced Research Projects Agency (DARPA), “This can affect the political process, law enforcement, commerce and more. There are broad impacts if people can easily manipulate images and video … and significantly reduce society’s trust in visual media.”
Yet it is not only fake news that is the problem. Trust in real news and videos is diminished as well when there is a high degree of uncertainty about what constitutes reality.
Concerns are especially high as the 2020 U.S. presidential election approaches. The rapid spread of false news on social media—such as a May 2019 doctored video of Nancy Pelosi that made the House Speaker appear intoxicated and slurring her words—have demonstrated the power of fake images and social media. The Pelosi video, which was viewed more than 2.5 million times on Facebook and shared many times by prominent political leaders, was actually dubbed a “cheapfake” video because the sound was simply slowed to about three-quarters speed.
Image Is Everything
Deepfakes potentially represent the next frontier in propaganda wars. They could depict fake murders or frame a person for a crime he or she did not commit, they could provide falsified evidence of a weapons system that does not exist, and they could be used to stage news events that never happened, such as immigrants rioting in order to sow discord. They could also be used to falsify evidence in a automobile crash and other events. “Right now, deepfakes tend to be around people, but many other possibilities are plausible,” Turek says.
Driving deepfake videos is a growing array of easily downloaded programs with names like AI Deepfake and Deep-Nude, that allow users to plug in images and synthesize fake content. This includes face-swapping, lip-syncing, and a technique called puppet-master that allows a person to manipulate a video with their own movements and expressions. The computer maps the targeted area, say the face, and transposes that face onto another person’s head. A generative adversarial network (GAN) compares the two sets of images or video—the system synthesizing content attempts to fool a second system that’s inspecting it. The second machine learning system indicates when it meets a threshold of appearing real. It’s then a matter of syncing audio and video. “You provide the images and the machine does the heavy lifting,” Farid says.
“There are broad impacts if people can easily manipulate images and video … and significantly reduce society’s trust in visual media.”
Over the last few years, computer scientists have fed more and more images into these GANs, so they are able to produce increasingly ultrarealistic fake images. Powerful image manipulation techniques that were once solely the province of movie studios producing scenes in films like Forrest Gump and The Matrix are filtering onto the desktop and into apps. Farid points out these applications don’t require enormous computing resources to accomplish the task. In fact, it often is possible to focus on a specific portion of the body. For example, when Peel created the Obama deepfake video, he only had to manipulate the area around the mouth, and use his voice to impersonate the president’s.
For now, there’s been plenty of alarm and, except for some documented cases of revenge porn, more hype than reality. However, as Lyu points out, “Awareness is a form of inoculation. The goal is not to stifle innovation—there are a number of positive and innocuous uses for the technology—it’s to create an environment where it is not misused and abused.”
Spotting Fakes
It often takes a trained eye to spot irregularities in deepfake videos—and human detection is not totally effective, even among experts. That is leading researchers down a path that focuses on machine detection, forensics, authentication, and regulation. Lyu, a pioneer in deepfake detection research, says many approaches, including those he has developed, focus on a basic reality: “A fake video is generated by an algorithm, while a real video is generated by an actual camera. As a result, there are clues and artifacts that can usually be detected. In many cases, there is image warping, lighting inconsistencies, smoothness in areas, and unusual pixel formations.”
DARPA also is working to elevate detection methods through advanced forensics algorithms that are part of its Media Forensics (MediFor) program. It, too, taps machine learning—much of its research involves the use of GANs. A convolutional neural network (CNN) trains a recurrent neural network (RNN) to spot abnormalities and anomalies. In practical terms, a computer might examine pixels in a photo or video and determine whether the laws of physics were violated in the making of the video. “Is lighting consistent, are shadows correct, does the weather or lighting match the date the video was captured?” Turek asks.
Of course, battling deepfake algorithms with detection algorithms using CNNs, RNNs, and other methods ultimately leads to a perpetual machine-learning cat-and-mouse game.
Another area of research revolves around the use of digital watermarks, which could be embedded in news content, business videos, and other materials where a high level of trust is required. However, a big problem with visual watermarks, Lyu says, is that they can be manipulated easily, and veracity is often based on the goodwill of the user. “It’s ultimately a voluntary system and those creating fake videos aren’t likely to use them,” he says.
Battling deepfake algorithms with detection algorithms using CNNs, RNNs, and other methods ultimately leads to a perpetual machine-learning cat-and-mouse game.
Researchers also are exploring ways to embed more sophisticated certificates or tokens into videos to authenticate them using cryptographically signed hashes that are stored on a blockchain. One startup, San Diego-based Truepic, is now working with chip maker Qualcomm to extract a signature from an image so it can be verified later. Another firm, U.K.-based Serelay, also has created a verification process to which a number of insurance companies have signed on already, in order to verify claims. Farid, who is a consultant for Truepic, says these validation systems could prove particularly valuable for users of social media sites, where there currently is minimal oversight and high levels of false news circulating because it is highly profitable to these organizations.
Farid believes social media sites also need to do a better job of inhibiting the spread of false news, including deepfakes. “They cannot wash their hands of all responsibility. When they know something is fake—and it has been clearly proven—they need to put monetization aside and do something to curb the spread.”
The Legal Picture
Not surprisingly, deepfakes are also testing the legal system and prompting the U.S. Congress, states, and other entities to take action. For example, the “Malicious Deep Fake Prohibition Act of 2018” (S.3805) would introduce penalties for those who create, with intent to distribute, fake videos that “facilitate criminal or tortious conduct.” In September 2019, Texas passed a law specifically prohibiting deepfake videos aimed at harming candidates for public office or to influence elections. A month later, California had passed a ban on sharing deepfake videos within two months of an election; the state also enacted a separate bill that makes it easier to sue over deepfake porn videos.
“Laws must be updated to protect against clear cases of digital harassment, such as revenge porn, but government entities must avoid legislating for or against specific features because the technology is evolving rapidly,” says Albert Fox Cahn, executive director of the Surveillance Technology Oversight Project at the Urban Justice Center, a not-for-profit organization that promotes privacy and civil rights.
However, not everyone agrees that new laws are needed. Electronic Frontier Foundation civil liberties director David Greene noted in a February 2018 online post: “If a deepfake is used for criminal purposes, then criminal laws will apply. This includes harassment and extortion … There is no need to make new, specific laws about deepfakes in either of these situations.” In civil cases, he noted, the tort of False Light invasion of privacy is applicable. “False light claims commonly address photo manipulation, embellishment, and distortion, as well as deceptive uses of non-manipulated photos for illustrative purposes.”
The one thing everyone can agree on is that a framework of fairness is essential. While it is impossible to stamp out every piece of false news and every deepfake video, it’s entirely possible to rein in the chaos.
Concludes Cahn: “It’s really difficult to have meaningful discussions and debates as a society when we can’t even agree on the underlying facts. When you consider that deepfake videos have the potential to deceive huge numbers of people, you wind up in a very dark place … a place that could significantly disrupt society and create a great deal of instability.”
Li, Y. and Lyu, S.
Exposing DeepFake Videos by Detecting Face Warping Artifacts. Computer Vision Foundation, November 1, 2018, http://bit.ly/2pbASwP
Long, C., Basharat, A., and Hoogs, A.
A Coarse-to-fine Deep Convolutional Neural Network Framework for Frame Duplication Detection and Localization in Forged Videos, Computer Vision Foundation http://bit.ly/33GP7ZL
Güera, D. and Delp, E.J.,
Deepfake Video Detection Using Recurrent Neural Networks, 2018 15th IEEE International Conference on Advanced Video and Signal Based Surveillance (AVSS). 27–30 Nov. 2018, https://ieeexplore.ieee.org/abstract/document/8639163
Koopman, M, Macarulla, R., and Geradts, Z.
Detection of Deepfake Video Manipulation, Proceedings of the 20th Irish Machine Vision and Image Processing conference, August 29–31, 2018, http://bit.ly/2NGd7qh
Join the Discussion (0)
Become a Member or Sign In to Post a Comment