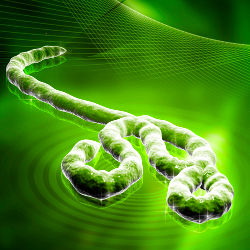
You cannot browse technology news or dive into an industry report without typically seeing a reference to “big data,” a term used to describe the massive amounts of information companies, government organizations, and academic institutions can use to do, well, anything. The problem is, the term “big data” is so amorphous that it hardly has a tangible definition.
While it is not clearly defined, we can define it for our purposes as: the use of large datasets to improve how companies and organizations work.
While often heralded as The Next Big Thing That Will Cure All Ills, big data can, and often does, lead to big blunders. Nowhere is that more evident than its use in forecasting outbreaks and spread of diseases.
An influenza forecasting service pioneered by Google employed big data—and failed spectacularly to predict the 2013 flu outbreak. Data used to prognosticate Ebola’s spread in 2014 and early 2015 yielded wildly inaccurate results. Similarly, efforts to predict the spread of avian flu have run into problems with data sources and interpretations of those sources.
These initiatives failed due to a combination of big data inconsistencies and human errors in interpreting that data. Together, those factors lay bare how big data might not be the solution to every problem—at least, not on its own.
Big Data Gets the Flu
Google Flu Trends was an initiative the Internet search giant began in 2008. The program aimed to better predict flu outbreaks using Google search data and information from the U.S. Centers for Disease Control and Prevention (CDC).
The big data from online searches, combined with the CDC’s cache of disease-specific information, represented a huge opportunity. Many people will search online the moment they feel a bug coming on; they look for information on symptoms, stages, and remedies. Combined with the CDC’s insights into how diseases spread, the knowledge of the numbers and locations of people seeking such information could theoretically help Google predict where and how severely the flu would strike next—before even the CDC could. In fact, Google theorized it could beat CDC predictions by up to two weeks.
The success of Google Flu Trends would have big implications. In the last three decades, thousands have died from influenza-related causes, says the CDC, while survivors can face severe health issues because of the disease. Also, many laid up by the flu consume the time, energy, and resources of healthcare organizations. Any improvement in forecasting outbreaks could save lives and dollars.
However, over the years, Google Flu Trends consistently failed to predict flu cases more accurately than the CDC. After the program failed to predict the 2013 flu outbreak, Google quietly shuttered the program.
David Lazer and Ryan Kennedy studied why the program failed, and found key lessons about avoiding big data blunders.
The Hubris of Humans
Google Flu Trends failed for two reasons, say Lazer and Kennedy: big data hubris, and algorithmic dynamics.
Big data hubris means Google researchers placed too much faith in big data, rather than partnering big data with traditional data collection and analysis. Google Flu Trends was built to map not only influenza-related trends, but also seasonal ones. Early on, engineers found themselves weeding out false hits concerned with seasonal, but not influenza-related, terms—such as those related to high school basketball season. This, say Lazer and Kennedy, should have raised red flags about the data’s reliability. Instead, it was thought the terms could simply be removed until the results looked sound.
As Lazer and Kennedy say in their article in Science: “Elsewhere, we have asserted that there are enormous scientific possibilities in big data. However, quantity of data does not mean that one can ignore foundational issues of measurement and construct validity and reliability and dependencies among data.”
In addition, Google itself turned out to be a major problem.
The second failure condition was one of algorithmic dynamics, or the idea that Google Flu Trends predictions were based on a commercial search algorithm that frequently changes based on Google’s business goals.
Google’s search algorithms change often; in fact, say Lazer and Kennedy, in June and July 2012 alone, Google’s algorithms changed 86 times as the firm tweaked how it returned search results in line with its business and growth goals. This sort of dynamism was not accounted for in Google Flu Trends models.
“Google’s core business is improving search and driving ad revenue,” Kennedy told Communications. “To do this, it is continuously altering the features it offers. Features like recommended searches and specialized health searches to diagnose illnesses will change search prominence, and therefore Google Flu Trends results, in ways we cannot currently anticipate.” This uncertainty skewed data in ways even Google engineers did not understand, even skewing the accuracy of predictions.
Google is not alone: assumptions are dangerous in other types of outbreak prediction. Just ask the organizations that tried to predict Ebola outbreaks in 2014.
Failing to Foresee Ebola
Headlines across the globe screamed worst-case scenarios for the Ebola outbreak of 2014. There were a few reasons for that: it was the worst such outbreak the world had ever seen, and there were fears the disease could become airborne, dramatically increasing its spread. In addition, there were big data blunders.
At the height of the frenzy, according to The Economist (http://econ.st/1IOHYKO), the United Nations’ public health arm, the World Health Organization (WHO), predicted 20,000 cases of Ebola—nearly 54% more than the 13,000 cases reported. The CDC predicted a worst-case scenario of a whopping 1.4 million cases. In the early days of the outbreak, WHO publicized a 90% death rate from the disease; the reality at that initial stage was closer to 70%.
Why were the numbers so wrong? There were several reasons, says Aaron King, a professor of ecology at the University of Michigan. First was the failure to account for intervention; like Google’s researchers, Ebola prognosticators failed to account for changing conditions on the ground. Google’s model was based on an unchanging algorithm; Ebola researchers used a model based on initial outbreak conditions. This was problematic in both cases: Google could not anticipate how its algorithm skewed results; Ebola fighters failed to account for safer burial techniques and international interventions that dramatically curbed outbreak and death-rate numbers.
“Perhaps the biggest lesson we learned is that there is far less information in the data typically available in the early stages of an outbreak than is needed to parameterize the models that we would like to be able to fit,” King told Communications.
“In the future, I hope we as a community get better at distinguishing information from assumptions,” King says.
That was not the only mistake made, says King. He argues stochastic models that better account for randomness are more appropriate for predictions of this kind. Ebola fighters used deterministic models that did not account for the important random elements in disease transmission.
“In the future, I hope we as a community get better at distinguishing information from assumptions,” King says.
Can We Ever Predict Outbreaks Accurately?
It is an open question whether models can be substantially improved to predict disease outbreaks more accurately.
Other companies want to better predict flu outbreaks after the failure of Google Flu Trends—specifically avian flu—using social media and search platforms. Companies such as Sick-weather and Epidemico Inc. use algorithms and human curation to assess both social media and news outlets for flu-related information.
These efforts, however, run the same risks as previous flu and Ebola prediction efforts. Social media platforms change, and those changes do not always benefit disease researchers. In fact, says King, data collection may hold the key to better predictions.
“I suspect that our ability to respond effectively to future outbreaks will depend more on improved data collection techniques than on improvement in modeling technologies,” he says.
Yet even improvements in data collection might not be enough. In addition to internal changes that affect how data is collected, researchers must adapt their assessments of data to conditions on the ground. Sometimes, as in the case of avian flu, not even experts understand what to look for right away.
“The biggest challenge of the spring 2015 outbreak [of avian flu] in the United States was that poultry producers were initially confused about the actual transmission mechanism of the disease,” says Todd Kuethe, an agricultural economist who writes on avian flu topics. “Producers initially believed it was entirely spread by wild birds, but later analysis by the USDA (U.S. Department of Agriculture) suggested that farm-to-farm transmission was also a significant factor.”
No matter the type of data collection or the models used to analyze it, sometimes disease conditions change too quickly for humans or algorithms to keep up. That might doom big data-based disease prediction from the beginning.
“The ever-changing situation on the ground during emerging outbreaks makes prediction failures inevitable, even with the best models,” concludes Matthieu Domenech De Celles, a postdoctoral fellow at the University of Michigan who has worked on Ebola prediction research.
Lazer, D., and Kennedy, R.
(2014) The Parable of Google Flu: Traps in Big Data Analysis. Science. http://scholar.harvard.edu/files/gking/files/0314policyforumff.pdf
Miller, K.
(2014) Disease Outbreak Warnings Via Social Media Sought By U.S. Bloomberg. http://www.bloomberg.com/news/articles/2014-04-11/disease-outbreakwarnings-via-social-media-sought-by-u-s-
Erickson, J.
(2015) Faulty Modeling Studies Led To Overstated Predictions of Ebola Outbreak. Michigan News. http://ns.umich.edu/new/releases/22783-faulty-modeling-studiesled-to-overstated-predictions-of-ebola-outbreak
Predictions With A Purpose. The Economist. http://www.economist.com/news/international/21642242-why-projectionsebola-west-africa-turned-out-wrong-predictions-purpose
Join the Discussion (0)
Become a Member or Sign In to Post a Comment