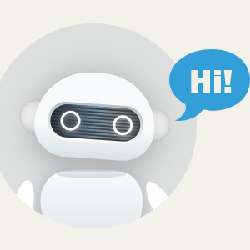
The idea of conversing with a computer is nothing new. As far back as the 1960s, a natural language processing program named Eliza matched typed remarks with scripted responses. The software identified key words and responded with phrases that made it seem as though the computer was responding conversationally.
Since then, such conversational interfaces—also known as virtual agents—have advanced remarkably due to greater processing power, cloud computing, and ongoing improvements in artificial intelligence (AI) and machine learning.
Today, chatbots are popping up at websites and in smartphone apps—even if they sometimes sputter, stammer, and fail. The same technology is helping robots, smart speakers, and other machines operate in a more humanlike way.
"Chatbots and conversational interfaces are becoming a realistic proposition," says Scott Likens, New Services and Technology Leader at professional services firm PricewaterhouseCoopers.
Research firm Statistica reports that the worldwide market for chatbots will grow more than six-fold between 2016 and 2025, from $190.8 million to $1.25 billion. U.K. research firm Juniper Research reports that chatbots could save companies $3.6 billion on healthcare alone by 2022.
Says Bassam Salem, founder and CEO of AtlasRTX, a chatbot software firm, "There is a growing acceptance and desire to use chatbots and digital assistants, particularly among young people."
Talk is Cheap
Developing chatbots that can communicate effectively with humans is a daunting task. A chatbot system must converse in a realistic way, while delivering relevant and useful information. A problem with early chatbots is that they relied on keywords and simplistic scripts to generate responses. In contrast, today's systems—relying on the same underlying technology that powers digital assistants like Siri, Cortana, and Alexa—tap machine learning and natural language algorithms to continually learn and generate relevant responses. What's more, accuracy increases and responses become more refined as the system digests additional data.
Yet, designing functional conversational interfaces requires more than merely tossing data science and AI at the task. Chatbots often stumble over the nuances of human interaction; replicating the way people talk and react is difficult. "A chatbot not only has to understand your questions, but also deliver appropriate responses based on an array of factors," says S. Shyam Sundar, founder and co-director of the Media Effects Research Laboratory at Penn State University, as well as the university's James Jimirro Professor of Media Effects.
According to Sundar, smarter and better conversational interfaces require a deeper, broader understanding of how to embed linguistics and behavioral elements into software. "In order to respond appropriately, these systems must understand turn-taking that is common in human conversations, provide tiny empathy and sympathy cues, process what a person is trying to accomplish, and take the right action," he explains.
The idea is to develop chatbots that communicate like a real person without appearing too real. At the center of this challenge is the concept of the "Uncanny Valley," which posits that as a robot or digital interface becomes more lifelike and takes on some human characteristics, people will tend to accept it and even like it; however, if the same interface becomes too realistic, humans will reject it. "There must be some distinguishing factors, so that it does not come across as eerily human," Sundar explains.
The Final Word
For now, chatbots remain linguistic toddlers; they're able to handle fairly basic interactions, but struggle with more complex words, situations, and scenarios. Making matters worse, they often are unable to recognize when they don't know something, or when they are taking the wrong direction or dispensing useless information. Nevertheless, "Algorithms are improving. We're moving from a question-and-answer phase to a more intelligent and complex conversational level," Likens points out.
Over the next few years, both supervised and unsupervised machine learning promise to transform chatbots into much better conversationalists. As humans annotate documents and transcripts to "fill in gaps" and address the nuances of human-machine interaction, chatbot performance will improve, Likens says. Yet there's also a need to design user interfaces to accommodate the way people use chatbots. This means better recognition of context and linking to documents, videos, and other content, as well as incorporating the use of live support agents when they are needed.
Understanding anthropomorphic components is crucial, Sundar says. His research shows that a high level of interactivity can compensate for the somewhat impersonal nature of a chatbot. A human name, mimicking a user's speech patterns, and acting more like a real person can also ratchet up acceptance, but can also raise user expectations for interactivity. "It's necessary to find the right level of humanness to match the interactive potential of a chatbot and deliver the appropriate anthropomorphic cues," he explains.
The ultimate goal, of course, is to develop conversational interfaces, including chatbots, which can tackle a wide array of tasks; sensing a user's needs, emotional state, and feelings, and even translating words across languages in real time.
Concludes PricewaterhouseCoopers' Likens, "Conversational interfaces represent a natural evolution in how we use devices."
Samuel Greengard is an author and journalist based in West Linn, OR, USA.
Join the Discussion (0)
Become a Member or Sign In to Post a Comment